Recently, I was interested in the application of data science. It is well known that data science could be used in personalised treatment, advertising, beating credit card fraud, etc. To my surprising, data science has already been used in the retail industry for long years. In the competitive retail industry, how could data science do? Let’s look at two real examples in the supermarket.
Grocery store know pregnancy
In 2003, an angry man went to his local supermarket ‘Target’ to complain with the manager that his teenage daughter received a personally addressed flyer from this supermarket. And the flyers were advertising maternity products, babywear, baby furniture, nappies and infant formula.
“are you trying to encourage my daughter to get pregnant?”
Some weeks later, the store manager rang back to apologise once again. It turns out his teenage daughter was, in fact pregnant, and she hadn’t told her parents yet.
– How Companies Learn Your Secrets
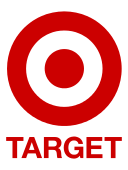
So, how did Target knows the daughter gets pregnant before his father? The answer is to find the correlation through Association analysis. Briefly speaking, correlation is a statistical term, and it measures the degree of relevance. Target supermarket researchers found that pregnant women are more likely to purchase a certain type of products. This means there is a high correlation between pregnancy and purchasing a certain type of products. This blog illustrates the correlation with a simple example.
Statisticians in Target uses the customers’ shopping habits and physical condition to find the relationship. Shopping habits are usually fixed, but customers will make changes when some events happen. For example, customers purchase more toilet rolls and hand sanitisers than usual during Covid-19. Pregnant women usually switch from scented soap to unscented soap and start to buy supplements such as calcium, magnesium and zinc. With such customers habit prediction models, Targets’ annual sales rise from 44 billion dollars to 67 billion dollars between 2002 and 2010, which indicates the success of prediction models based on association analysis.
Beers and diapers
This reminds me of another real example in the retail industry – ‘beer and diapers’. Walmart found that men are more likely to purchase beers and diapers together at once shopping. Similarly, they use the association analysis to study the correlation among shopping history and find out beers and diapers are commonly purchased together. Thus, you may find the beer aisle is always next to the diapers.
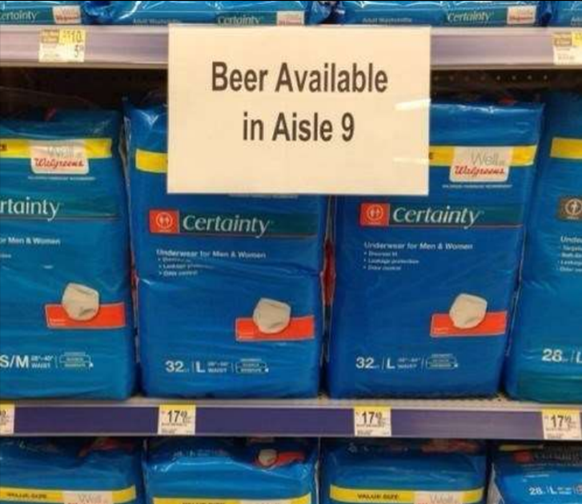
Furthermore…..
As most of us already know, the location of the aisle is designed exactly by statistical research. But it is too outdated. Nowadays, supermarkets are using data science to do more things. For example, they use data science to help them make good promotion methods; they predict the sales of certain products (like umbrellas, ice creams and so on) based on the weather to prepare enough stock. Besides, they also use data science to manage inventory based on the prediction of the next day. Watching BBC documentary ‘Supermarket secret‘, you will be amazed how much data science they have been used to provide a more convenient shopping environment to attract you!!
However, data science will not always true?
Such models are based on typical rules; as long as user fit their rules (e.g., purchasing unscented soap and supplement), they may get the result like pregnancy. Although such a model is blatant and crude, it indeed helps retailers make better decisions resulting in high profits.
However, is Targets’ model always true? A woman may start to buy supplements and unscented soaps when she becomes allergic and want to improve her physical condition by supplements. Still, the model thinks she has a high probability of being pregnant. In other words, pregnant women are more likely to purchase supplements and unscented soaps, but purchasing supplements and unscented soaps don’t mean pregnant. That’s the difference between correlation and causality. And such causality somehow relates to the prediction ability of the model.
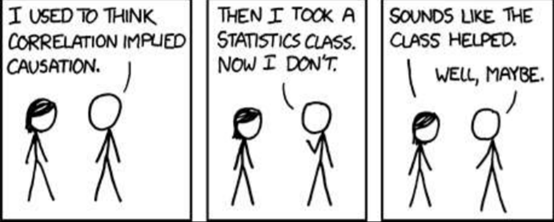
We could easily get the correlation result as it just the mathematics. However, the result only indicates correlation instead of causality without context. For example, in summer, the sales of ice cream will increase, and simultaneously sharks become active. We could easily get the high correlation result between ice cream and shark attacks. However, does this relationship make sense? That is to say that there’s no causal relationship in either direction — neither of these things causes the other, even indirectly.
So, the useful analysis will need both highly correlated and causal relationship. And high causality to some extent is related to the high prediction ability of the model.
In conclusion, we have introduced two famous examples about data science in retail industry. And we also recommend a good BBC documentary. Finally we introduce that data science will not always be true due to the causality. When searching data science in the supermarket, I found these blogs are very useful:
https://towardsdatascience.com/how-data-science-and-ai-are-changing-supermarket-shopping-e47f63f4b53f This blog talks about more generally how data science and AI changes supermarket
https://towardsdatascience.com/correlation-and-causality-a-beer-and-diaper-story-27a064f4f995 It talks the difference between correlation and causality.
https://livebook.manning.com/book/machine-learning-in-action/chapter-11/#:~:text=Association%20analysis%20is%20the%20task,items%20that%20frequently%20occur%20together. It talks about the association analysis in a mathematical way.
https://towardsdatascience.com/association-analysis-explained-255823c1cf9a It explained the association analysis in detail and in a technical way with R code.