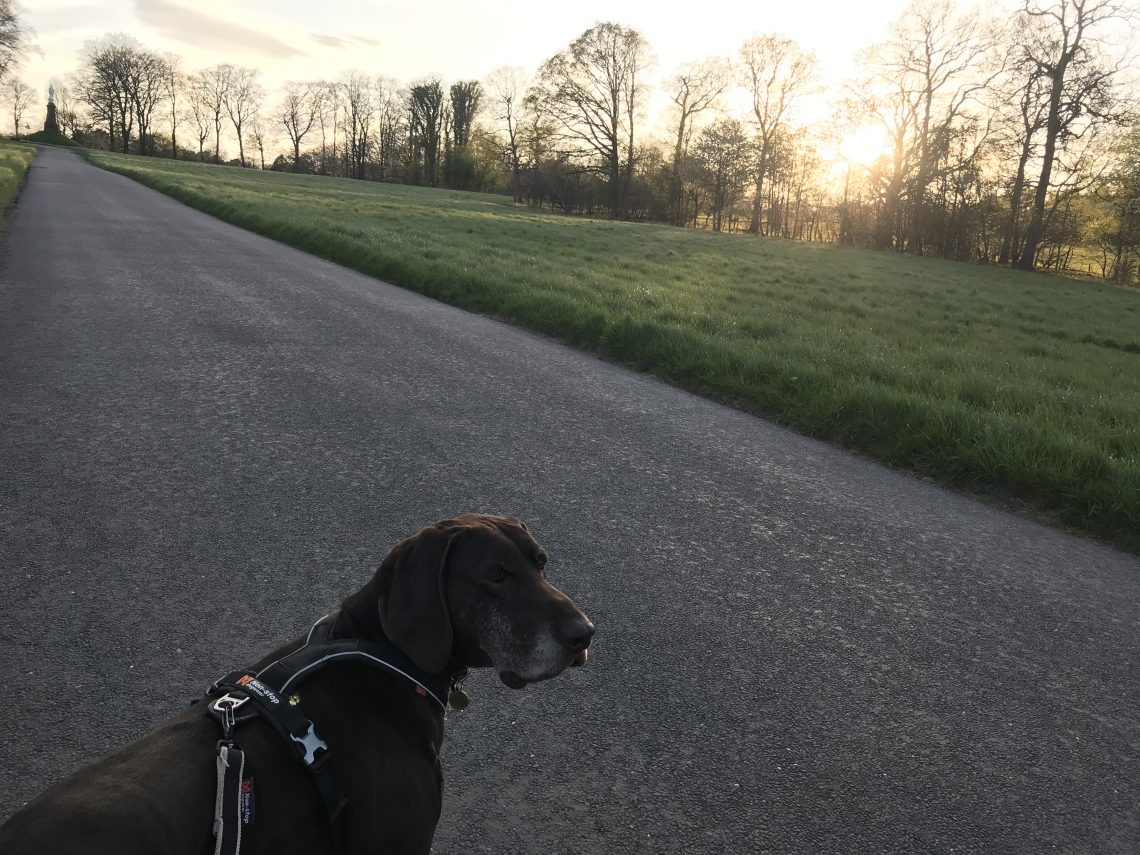
Dealing with Imputation Uncertainty
This post tackles a popular method that helps you understand the amount of variability you have introduced to your analysis through replacing missing data with estimated values. This variability is known as Imputation Uncertainty.
If you haven’t read my first two posts on Missing Data, it might be worth taking a look before you read this. You can find the first post here, and the second, here.
I had some misgivings about imputation before I learnt about methods to quantify imputation uncertainty.
My misgivings centred around the fact that with imputation we are sort of making the data up (in a statistically rigorous fashion, of course!). But even so, how happy could we be with our analysis after imputing?
It turns out we can use a method that gives us insight into how much variability is down to the fact that we have imputed missing data.
This can help us to understand how confident we can be in our statistical analysis, given that it is based in part on missing data.
One popular method that gives us a measure of imputation uncertainty is Multiple Imputation.
How do we do Multiple Imputation?
- Firstly, we create an imputed data set using any method that involves taking draws from a predictive distribution.
- We repeat this, to create M imputed data sets.
- We can analyse these data sets, to come up with estimates of parameters we are interested in.
- We can then combine these estimators. There are also formulas that we can apply to calculate within imputation variance, across imputation variance, and overall variance.
- These can give us an idea of how much of the variability in our estimates is down to the imputation process.
Multiple Imputation isn’t the only method that can help us with Imputation Uncertainty. You can read more about them in some of the references below.
Further reading
You can find out more about Imputation Uncertainty in Chapter 5 of the below book. Multiple imputation is discussed in Chapters 5 and 10.
Little, R. J. A. and Rubin, D. B. (2020). Statistical analysis with missing data. Wiley Series in Probability and Statistics. Wiley, Hoboken, NJ, third edition.
This paper below contains a nice summary of Multiple Imputation and goes on to discuss the issue of variable selection. In other words, it considers what to do if your different imputed data sets imply that different variables are valuable and should be kept in a statistical model, while others should be discarded.
Wood, A. M., White, I. R., and Royston, P. (2008). How should variable selection be performed with multiply imputed data? Statistics in Medicine, 27(17):3227-3246.
And here is a long report I wrote as part of my studies at STOR-i on the broader topic of Missing Data: click here to read. It discusses Imputation Uncertainty, and other issues, in more depth.

2 Comments
Pingback:
Pingback: