If you’ve ever taken a statistics class, you might’ve been taught both the frequentist (or, as some call, classic) and Bayesian approaches to inference. But which one is the best? If there is a best one… In this post I’m going to give you a brief explanation about the two methodologies and then, by the end, maybe you’ll have all the cards to choose the one that suits you the best. Who knows if you’re a frequentist or a Bayesian person? Let’s start.
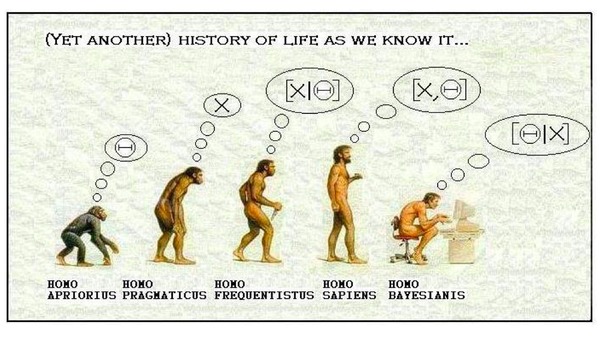
Frequentist Approach
The classical approach to inference focuses more on the data generating process. It makes predictions on the underlying truth of the experiment using only data from the current experiment. So, if you choose to use a frequentist methodology, you’ll start by making assumptions about the process that generated the data. Then, you’ll produce as many replications you wish and you’ll finalise by trying to build some evidence for what the parameter, say \theta , is or is not.
How can you do that? Mainly, through hypothesis testing and p-values. You can check here a very interesting post about this that my friend Maddie wrote. Briefly, a p-value is a probability (that you calculate) of obtaining an effect at least as extreme as the one in the data you have, assuming that H_0 is true. If you have a small value, it is less likely that the results are completely random and hence it is more significant. Analogously, a large value will be less significant and so there is a higher chance of your results to be random.
But what is good and what is bad in the classic way? As I, at least, like to hear the bad news first, let’s start with the critiques. First, we can argue that it’s ad-hoc and doesn’t really have a deductive logic behind: the p-value depends on the setup of the experiment and that’s it. Also you’ve to fully specify these experiments ahead of time. The last point of criticism I’ll mention may not be a downside of the method but ignorance. Often people wrongly interpret the p-value saying that, if we have a p-value of 0.05 , then the probability of H_0 is 5\% . But this is not true. A significance level of 0.05 means that, if H_0 is true, then 5\% of the time H_0 will be rejected due to randomness. Let’s now pass to the “good news”. Given a p-value, you either reject or don’t reject H_0 , hence it’s objective. Furthermore, what was also a downside, it’s also an upside. The fact that we have to specify the experiment beforehand may help control the research bias, for example.
Bayesian Approach
Probability is orderly opinion, and that inference from data is nothing other than the revision of such opinion in the light of relevant new information
Thomas Bayes
The philosopher Thomas Bayes is mostly known for formulating the very basis of Bayesian inference, Bayes’ Theorem:
P(A \mid B)=\frac{P(B \mid A)P(A)}{P(B)},where A and B are events.
But how exactly does Bayesian inference work? Well, first you’ll define a prior distribution (informative or non-informative) which includes your subjective beliefs about the parameter \theta . Then, you’ll gather data and update your prior with the data using Bayes’ theorem. With this you’ll obtain your posterior distribution, which is the distribution that represents your updated beliefs after having observed data. Finally, you’ll analyse this distribution and summarise it. For that you can use to the mean, median, quantiles, it’s your choice.
Moreover, as before, there are also pros and cons for using this method. Starting again with the “bad news”. The major critique to Bayesian inference is precisely the fact that you have subjectivity in the prior. As there isn’t a method for choosing a prior, you may end up choosing a prior that you believe in and I may end up choosing one completely different, for example. Thus, there’s a chance for us to reach different posteriors distributions and hence different conclusions. Not ideal, huh? Some also argue that, regardless of knowing the true case, a hypothesis is either true or false so it shouldn’t be assigned a probability. However, assigning a probability to the hypothesis also constitutes an advantage since it’s what it’s needed to make decisions (besides being easier to communicate a result). Moreover, having subjectivity on the choice of priors also allows for performing a sensitivity analysis, where you can study the effects of choosing different prior. A major benefit, I would say, is the fact that conclusions lie in a probability distribution rather than a point estimate.
But what to choose?
Both have advantages and disadvantages. In one hand, a frequentist approach is less computationally intensive than a Bayesian approach. On the other hand, having an informative prior will ease some issues that we may encounter in classical inference. Be careful, that this might not happen if you take non-informative priors.
So, are you a frequentist or a Bayesian statistician? In reality, it won’t really matter the methodology you choose. The important thing is to understand and correctly interpret the results you obtain in your study. So, adopt the one you fancy the most.
I hope you found this post interesting. You can find more about this subject in the links below
- https://infotrust.com/articles/bayesian-vs-frequentist-methodologies-explained-in-five-minutes/
- https://ocw.mit.edu/courses/mathematics/18-05-introduction-to-probability-and-statistics-spring-2014/readings/MIT18_05S14_Reading20.pdf
- https://agostontorok.github.io/2017/03/26/bayes_vs_frequentist/
I’ll finish by citing a description of both methodologies I found here while writing this post. See you later!
Frequentist Reasoning
I can hear the phone beeping. I also have a mental model which helps me identify the area from which the sound is coming. Therefore, upon hearing the beep, I infer the area of my home I must search to locate the phone.
Bayesian Reasoning
I can hear the phone beeping.Now, apart from a mental model which helps me identify the area from which the sound is coming from, I also know the locations where I have misplaced the phone in the past. So, I combine my inferences using the beeps and my prior information about the locations I have misplaced the phone in the past to identify an area I must search to locate the phone.