Background
I earned a BSc in Mathematics and Applied Mathematics from Huazhong University of Science and Technology in 2020. I then obtained MSc degrees in Financial Math and Statistics from King’s College London and the University of Warwick. During my Financial Math degree, I specialized in machine learning for solving PDEs and focused on Deep Pricing of American options in the CEV model. In my Statistics degree, I developed a strong interest in Bayesian Statistics and MCMC methods, which deepened my desire for further research.
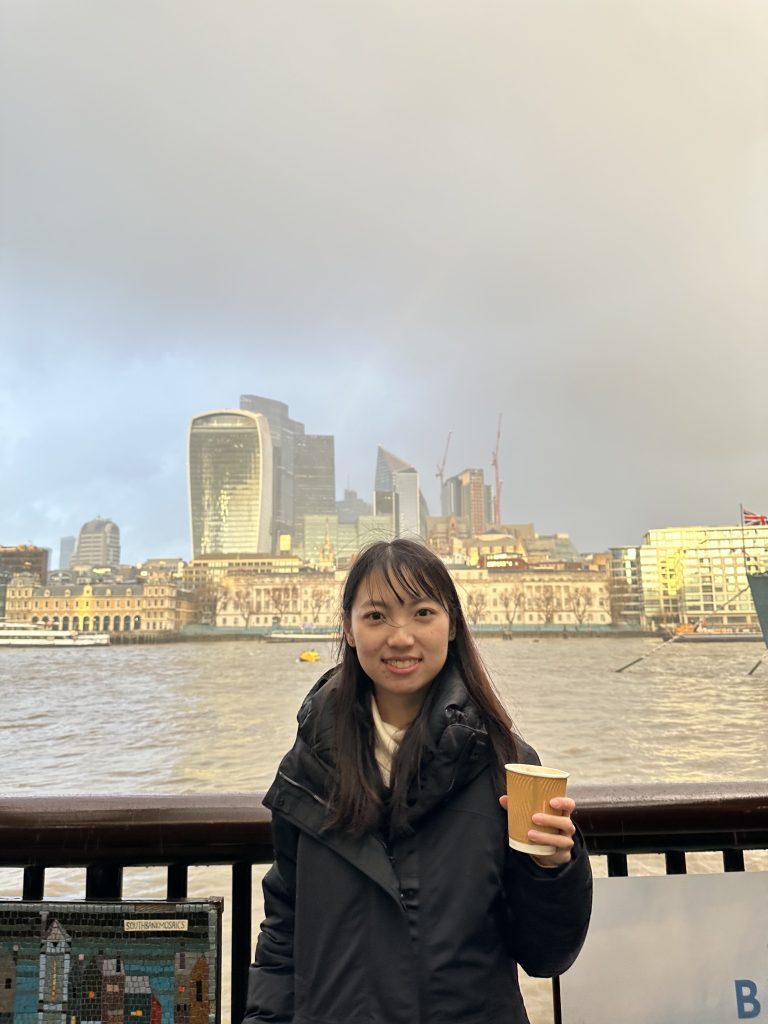
I appreciate the focus on teamwork and problem-solving within STOR-i. Moreover, I eagerly anticipate the opportunity to collaborate with industry, allowing me to witness how theoretical concepts and methodologies are applied in practical contexts. Additionally, the prospect of delving into diverse topics during the MRes year, enabling me to pinpoint my specific research interests, excites me. I am eagerly anticipating the day when I can embark on my independent research journey.
Experience
My passion for Statistics and Financial Mathematics initiated from my studying experience in Financial Mathematics at King’s College London. The knowledge that I gained during the Risk Neutral Valuation module made me realize that one of the most important and basic models in pricing of options, the Black-Scholes-Merton model, is based on the assumptions of ideal conditions of the market. However, the real financial market may not satisfy these assumptions, so I am interested in option pricing outside of the Black-Scholes-Merton model. Besides, my experience at the Econophysics module inspired my interest in applying statistical method in
finance. Precisely, by looking at historical data, it can be found that the probability distribution of equity prices and their tails showing that they are not Gaussian but power law, which contradicts the assumption of perfect market in many financial models.
During the final project at King’s College London, I built a deep neural network to approximate the pricing function mapping from the parameters like stock prices, strike prices and time to maturity to the prices of American put options computed by the Finite Difference Method and did model calibration using this neural network. Then I learned that machine learning can be used to enable the rapid computations of option prices, which is further essential to model calibration and the computation of risk of option portfolios. In conclusion, my current research interests mainly involve the application of machine learning and other statistical methods in finance.
To further explore these interests, I began my second MSc program in Statistics at University of Warwick. The experience here not only strengthened my basic knowledge of statistics like Linear Regression, Probability and Hypothesis Testing, but also widened my insight into simulation-based techniques like the Monte Carlo method. The content of Monte Carlo module sparked my new interest in researching Monte Carlo Markov Chains (MCMC) and a variety of sampling algorithm like the Gibbs Sampler. Therefore, besides my interest in Financial Mathematics, I would like to leave some space for more possible research interests in MCMC.