Making Random Things Better: Optimisation of Stochastic Systems
At the STOR-i Annual Conference earlier this year, Professor Christine Currie presented two interesting applications of optimisation, where the elements of the system are subject to uncertainty. Optimisation involves finding a value that maximises or minimises a function, this is often a complicated and random function. In many real-life situations, it is optimistic to assume all data elements in the system are known quantities and that none are subject to uncertainty. Christine outlined examples in the passenger transportation industry and in healthcare where the inputs and outputs of the system are uncertain.
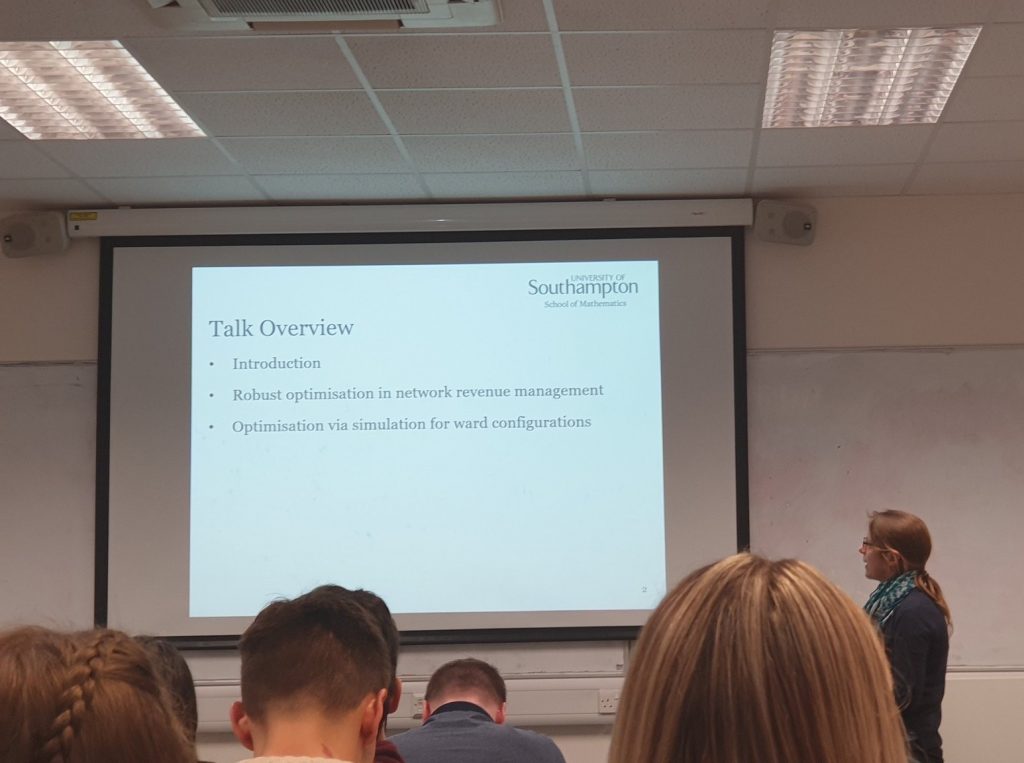
Christine is Associate Professor of Operational Research in Mathematical Sciences and Director CORMSIS (the Centre for Operational Research, Management Science and Information Systems) at the University of Southampton. Additionally, she is Editor-in-Chief for the Journal of Simulation. Christine’s research is concerned with simulation optimisation, mathematical modelling of epidemics, optimal pricing and applications of simulation in healthcare.
Airline Revenue Management Example
Firstly, Christine delivered an optimisation example for network revenue management of tickets for an airline. This is modelled as an optimisation problem because the aim is to maximise revenue subject to capacity, demand and sales. It is difficult to set prices for these tickets since it is uncertain how many will be sold, we say there is stochastic demand. If it was certain that there would be a high demand for a certain flight, then it is likely that the airline will set higher prices to maximise revenue. To complicate this further, airlines often sell different classes and packages of tickets, so it must be decided at what prices different seats will be sold. Since the seats have no value once the plane departs, we have a perishable inventory and it is fundamental to sell all tickets in order to maximise revenue.
Whilst the demand is uncertain, bounds can be assumed and a probability distributed assigned to it. Therefore, Christine suggests maximising the minimum revenue or minimising the maximum regret instead of just maximising revenue. This approach uses the bounds and distribution of demand, so it is not required to know demand exactly.
Healthcare Example
Christine provided us with a different example within healthcare to illustrate the idea of subset selection. Here, patients are waiting for transport to an acute hospital ward before rehabilitation. The ward is set up with an arbitrary number of bays and within each bay, there is an arbitrary number of beds but each bay must be single sex. These make up the constraints for the optimisation problem where the primary objective is to minimise waiting time. Additionally, the secondary aim is to maximise bed utilisation and minimise patient transfers around the ward.
Clearly, this quickly turns into a complicated optimisation problem. This requires a complex decision to be made with multiple objectives and a large number of options (including patients and beds). Christine’s work suggests providing the system expert with a subset of solutions rather than a single optimal solution, this avoids a situation where the expert is presented with a solution they cannot implement. Therefore, rather than a single solution, it is proposed that a subset of the system is chosen such that we are within some proportion of the optimum solution with some probability.
I thoroughly enjoyed listening to applications of optimisation in real-life situations; it was interesting to see how Christine approached the challenges of uncertainty and complexity. To read more about Christine’s research you can visit her website. Additionally, please read my blog post about the STOR-i conference and all of the talks to find out more.