In my last post I promised an overview of my two research topics. We were encouraged to choose one topic from Statistics and the other from Operational Research. Today we will focus on the more statistical topic which I was introduced to by Emma Eastoe.
In statistics we are often interested in determining the most likely behaviour of a system. The usual way to do this would be to fit a model to the observations from the system. This can be done by finding a family of distributions that approximately describes the shape of the data. This family of distributions (or model) will have certain parameters. The observations can then be used to estimate the value of these parameters which maximises the probability of that set of observations occurring. In some situations however, the normal behavior of a system is of less concern to us and we are instead interested in the maximum (or minimum) outcome that we would expect to observe over an extended period of time. For example, if a local council is considering investment in flood defences they are not interested in the average height of the river but only in the events where the volume of water would exceed the river’s maximum capacity and cause flooding.
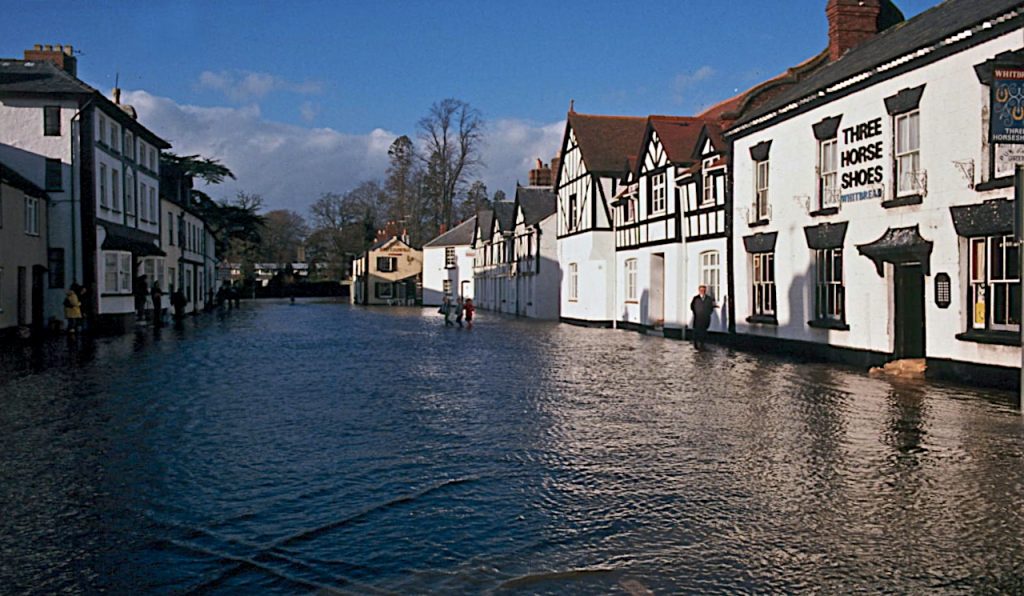
The problem here is that we are considering very unusual events that any distribution which was fitted to the entire set of observations would be unable to reliably estimate. We therefore require models that can be fitted to just the extreme events. There are two main approaches to consider: the Block Maxima Model and the Threshold Excess Model. Each of these approaches can by characterised by their different way of classifying an event as extreme.
- Block Maxima Model: Here we partition the data into equal sections and then take the maximum data-point in each block to be an extreme event. The distribution of these maxima belongs to a specific family of distributions called the Generalised Extreme Value Family.
- Threshold Excess Model: This approach considers all events that are above a certain threshold to be extreme. It can be shown that for a sufficiently high threshold these values will follow a Generalised Parito Distribution.
In both models we have an important decision to make. For the Block Maxima Model we must choose a block length and in the Threshold Excess Model we must set a threshold. These decisions play a very similar role in that they determine the number of points we have to fit our model to. If the block size is set too large or the threshold too high we will not have enough points to fit our distribution which will result in greater variance in the result. On the other hand if the block size is too small or the threshold too low the resulting points will not be well approximated by the Extreme Value or Parito distribution respectively.
Sometimes the data we are looking at is multidimensional. For example, if we want to describe extreme storm events for applications in shipping we may have data for wind and rain. These different variables may depend on each other or could be completely independent. Having more that one dimension imposes another difficulty – what do we want to consider as an extreme event. Do we need extreme values for wind and rain or is just one of the variables being extreme enough for an event to be considered extreme? Both the Block Maxima and Threshold Excess approaches can be extended to consider higher dimensions.
In my next post I will talk about my Operational Research topic: Optimal Patrolling.