In my last post, I briefly discussed the two standard approaches to modelling of extremes events, block maxima and threshold models. Both models were introduced with the assumption of observations being independent and identically distributed. This assumption of temporal independence is unrealistic as most extreme events occur over several consecutive observations. This may make you question the appropriateness of the two models I have mentioned previously and rightly so!
Stationarity
Stationarity is more realistic for many physical processes. This allows variables to be mutually dependent but the stochastic properties remain the same throughout time. So, the distribution of X_1 is the same as that of X_{41}. When extreme observations of a process exhibit short-range dependence, i.e. several consecutive observations are classified as extreme, they are said to form a cluster. In order to fit the models previously mentioned, we need some way to obtain observations from clusters which we can then deem independent. This is called declustering.
A more precise definition is as follows:
Declustering corresponds to a filtering of the dependent observations to obtain a set of threshold excesses that are approximately independent.
Inference for clusters of extreme values of a time series usually requires the identification of independent clusters of exceedances over a high threshold. There are many different methods used to “decluster” a stationary series. The choice of declustering scheme can have a significant effect on estimates of within-cluster characteristics and return levels.
A common approach is to identify independent clusters of exceedances above a high threshold, to evaluate the characteristic of interest for each cluster and to form estimates from these values. The two most common methods used to identify clusters are blocks and runs declustering. Runs declustering, for example, assumes that exceedances belong to the same cluster if they are separated by fewer than a certain number of observations below the threshold, known as the run length. The potential problem then arises in the selection of this run length parameter as this choice is somewhat arbitrary.
Peaks over Threshold
The standard approach to declustering is called Peaks over Threshold (POT). Once a definition of clusters has been decided, the maximum excess in each cluster is recorded and these cluster maxima are then assumed to be independent. The GPD can then be fitted to these independent cluster maxima.
However, there are some problems with this approach.
- It is sensitive to the choices made in the definition of a cluster, i.e threshold and run length selection.
- Again, as with the block maxima approach, there is a significant wastage of information by only considering the maximum of each cluster in the modelling procedure.
- This method can also incur significant bias in the estimates of parameters and subsequently, in the return level estimates.
- Cluster characteristics cannot be investigated when working with this filtered set of independent extremes.
Return levels
Return level estimation is very important when modelling extreme data. The n-return level is the level we expect to exceeded every n observations. These return level estimates are used in the design specifications of preventative measures such as sea-walls and thus, significant bias in these estimates could cause serious problems. Damage can occur that could’ve been prevented such as the flood damage at Newlyn, pictured below.
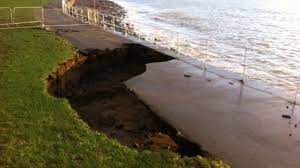
An Interesting Approach
One method that really caught my eye which aims to reduce this bias to negligible levels is a declustering scheme developed by Lee Fawcett and David Walshaw. The authors take a rather unusual approach to dealing with the dependence present in the series. They, simply, ignore it!
Initially, Fawcett and Walshaw propose continuing with the incorrect assumption of independence between exceedances of a high threshold. They use all threshold exceedances in the modelling procedure, dealing with the problem of waste in other methods. This approach of using all exceedances also manages to significantly reduce the bias in parameter and return level estimates.
The only problem with this approach is that due to the incorrect assumption of independence, standard errors associated with model parameters are underestimated. To deal with this, the authors use a method developed by R.L. Smith, which allows them to inflate standard errors to more realistic levels using a relatively simple adjustment.
Thus, the authors manage to deal with the problem of dependence by initially, ignoring it and accounting for it afterwards. This approach works well where the goal is to improve return level estimation.
The authors also developed another approach in which they used all threshold exceedances again but on top of that, explicitly modelled the temporal dependence structure with the goal of improving estimation of cluster characteristics. This approach adds a large degree of complexity to the modelling process and so is really only justified in the case where cluster characteristic are of interest.
Conclusion
There are many approaches to deal with dependence in time series extremes, some more complicated than others. I think the goal of the researcher should dictate the types of methods used. If the goal is to improve return level estimation, then the simpler approach of ignoring dependence and accounting for it later seems like a good idea. However, if the aim is to gain insight into the dependence structure of a process and look at within-cluster characteristics, then explicit modelling of dependence might be needed. I will reference another interesting approach below which is more concerned with cluster characteristics. The approach developed by Christopher A. T. Ferro and Johan Segers, relies on a particular cluster characteristic called the extremal index and is based on the limiting distribution of times between exceedances.
As I’ve said, there are many other methods out there with a variety of goals which help to deal with the added complexity caused by the removal of the unrealistic assumption of temporal independence in extreme observations. I have only briefly outlined one approach which looked to improve on the standard POT method. I hope you’ve enjoyed reading this post and gotten a taste for how dependence is dealt with in an EVT setting.
Thanks for reading! References below and as always, feel free to leave a comment or contact me through the contact form if you’d like to discuss any part of this.
Inference for clusters of extreme values – Ferro & Segers
Improved estimation for temporally clustered extremes – Fawcett & Walshaw
Markov chain models for extreme wind speeds – Fawcett & Walshaw