Infinitely Many Doctors? Hmmm...
6th March 2016
I am currently in the process of putting together a literature review on one of the research topics that were presented to us in January
and February. The topic I have chosen to look at is Infinite Server Queuing Theory and looking at its applications within Health Care
Services. The topic was presented by Dr. David Worthington and he
talked about some of the work done in the past, particularly by PhD students he has worked with. Queuing Theory is the mathematical study
of modelling queues that form when people, objects or tasks (all referred to as customers) are waiting for a service (of some description)
to happen. They are treated as stochastic processes and so probability theory plays a large role. There are many different types of queuing
system though they are generally described by three properties and denoted by $A/B/S$ where:
- $A$ determines how the customers arrive at the queue;
- $B$ specifies the probability distribution behind the service times;
- $S$ is the number of servers that can administer the service;
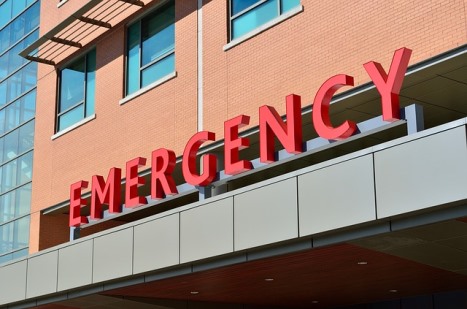
The arrival process is often taken to be a Poisson process, that is, the time between arrivals come from an Exponential distribution with
rate $\lambda$ and are independent. This is denoted by an $M$. There are other arrival processes that can arise, but for this blog we will
focus on the Poisson process case. The service time can come from any non-negative probability distribution.
For the case I am studying, $M_t/G/\infty$, there are infinitely many servers, a time varying Poisson arrival process and a general
service time distribution. This may seem like a rather useless thing to study. There are no systems at all that have infinitely many
servers or infinite capacity. So what is the point of wasting time on these?
However, there are a number of benefits to this system, and insights can be gained into how a system runs. The first benefit is that it is
actually mathematically tractable. There have been many papers written about the properties of the $M_t/G/\infty$ queue. Suppose that the
number of servers busy (which equals the number in the system) is a random variable $Q(t)$ with expectation $m(t)$. The primary ones are by
Eick, Massey and Whitt (see references). In these papers, the central theorem of interest contains the following:
For each $t$, $Q(t)$ has a Poisson distribution with mean
$$m(t) = \mathbb{E}\left[\int_{t-S}^t\lambda(u)du\right] = \mathbb{E}[\lambda(t-S_e)]\mathbb{E}[S].$$
$S_e$ is a random variable with cdf given by the stationary-excess of the service time $S$. The remarkably simple formula allows one to
calculate the expected number of people being served at any one point. More importantly, the fact that $Q(t)$ comes from a Poisson
distribution means that its full distribution is specified. Thus, if the arrival rate, $\lambda(t)$, and the service time distribution is
known for a system, then one can calculate the probability of ever exceeding a specific threshold. The papers also give explicit formulae
for particular values of $m(t)$ under particular situations. The main use of this is to help with
capacity planning, ie. Working out how many resources you need to cover a particular percentage of time. This idea was mentioned in the
context of Police Cars in my blog post Problems with Police Cars and Pricing. Although the model does not
include the effects of hitting the limit of servers available, it does give an indication of when the pressure is applied and the magnitude
of that pressure, which can then be used to work out how many servers one needs.
In a paper by Massey and Whitt, the theorem was generalised to a network of queues, each with infinitely many servers. The main difference
being that $Q_i(t)$ at point $i$ in the network has mean dependent on its aggregated arrival rate (that is, from both outside and elsewhere in
the network).
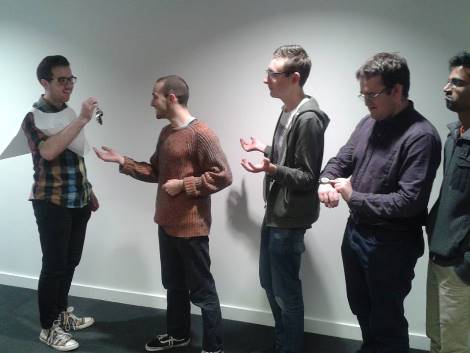
A few of the STOR-i crew queuing up. Note that this wouldn't have happened if there were infinitely many servers!
You may still be wondering what this has to do with Health Care. Well, one particular example comes from a paper by N Izady and D Worthington.
Their idea was to model A&E as a network of queues with different resources, but each with infinitely many servers (Doctors or Nurses). Then,
they used a so called Square Root Staffing Law to find the true number of servers $s_i(t)$:
$$s_i(t) = \bigg\lceil m_i(t) + \beta\sqrt{m_i(t)} \bigg\rceil$$
where $\beta$ is a quality of service parameter. Once this had been generated, the true system was simulated using these staffing levels to see
whether or not the 98% discharge within 4 hours (set by the government) was fulfilled. If not, the value of $\beta$ was changed until it was
met. This approach allowed a spreading out of pressure and waiting times over the day whilst reducing the man hours of the Doctors and Nurses.
I hope this post has shown that even something that sounds absurd, like assuming infinitely many doctors in a model, can still be of use in the
health service. I hope that in the next post or two, I will be able to give another example of where this has been useful.
References
[1] The Physics of the $M_t/G/\infty$ Queue,
Eick S., Massey W. and Whitt W., Operations Research, Vol.41, 31-42
(1993)
[2] $M_t/G/\infty$ Queues With Sinusoidal Arrival Rates,
Eick S., Massey W. and Whitt W., Management Science, Vol.39(2), 241-252
(1993)
[3] Networks of infinite-server queues with nonstationary Poisson input,
Massey W. and Whitt W., Queueing Systems, Vol.13(1), 183-250
(1993)
[4] Setting staffing requirements for time dependent queueing networks: The case
of accident and emergency departments, N. Izady, D. Worthington, European Journal of Operational Research, Vol.219, 531–540 (2012)