Improved tactical sales forecasting for global supply chains: how to use leading indicators?
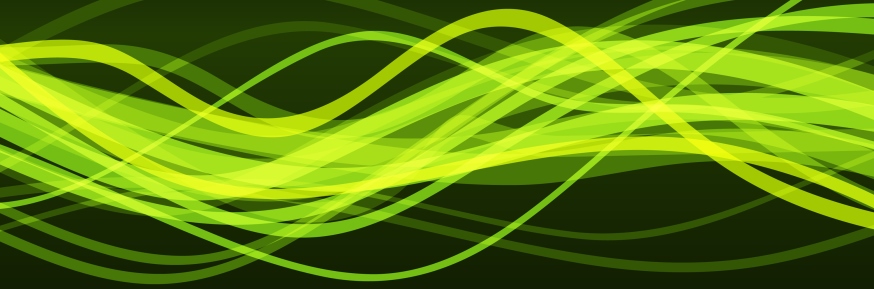
26 February 2016
26 February 2016
Long term tactical sales forecasts are vital for effective steering of global supply chains. Events and changing markets can cause radical changes from business as usual. How can manufacturers update their forecasts?
Conventional extrapolative forecasting methods, such as the commonly used exponential smoothing, cannot capture market dynamics and changes in the economic environment (Weller & Crone 2012). For such problems causal models, like regression, are valuable. However the problem with causal models lies with the identification of the appropriate drivers of sales. Which macro-economic variables provides the best early signal of a shift in the market and therefore allow us to revise our forecasts correctly? In fact this question has two aspects: one is to identify the correct indicator and the other is to identify how early this indicator carries information about the incoming changes, also known as the lead order of the indicator.
Consider a global supply chain with a wide geographical spread and all the possible macro-economic and other indicators. The problem complexity can quickly become overwhelming. It is common in practice to rely on experts to identify the appropriate indicators, but when the problem is put in perspective it is easy to see that the task is almost impossible to be solved solely by using judgment. To make matters worse, conventional regression models are not able to provide meaningful answers, particularly given the limited sales history in comparison to the very large set of potentially useful indicators. This is because the chance for picking spurious connections or building overfitted models that do not forecast well is too high.
Research at the Lancaster Centre for Forecasting (LCF) has shown that we can formulate advanced causal model capable of identifying useful inputs from a pool of more than 60,000 macro-economic indicators. When considering potential lead orders and all the dynamics, the problem explodes to select from at least 800,000 variables. The size of the task obviously makes it impossible to resolve by market expertise alone or conventional regression based forecasting models. Using the Least Absolute Shrinkage Selection Operator (LASSO), with a setup that was developed by the group, we are able to identify meaningful leading indicators that improved forecasting accuracy. Research at LCF has already shown the strength of LASSO in other areas such as promotional modelling and this study demonstrates the usefulness of the method for business forecasting (Ma et al. 2015).
In our case study company, we observed substantial accuracy improvements over current tactical forecasts and statistical benchmarks for both the USA and Europe markets. Furthermore, in discussions with supply chain managers it was confirmed that the identified leading indicators were meaningful and helped them to improve their insights.
This is ongoing research with several open questions. Embedding such tactical forecasting models in organisations provides opportunities and challenges, particularly in terms of embedding inputs from expert knowledge and providing market intelligence and feedback to managers. We are currently engaged in further research that investigates different ways that experts could contribute to the specification of the statistical forecasting method. If you are interested to learn more about this research please contact us.