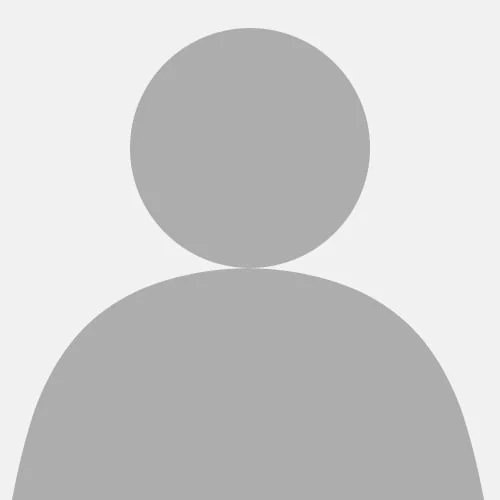
Rooney Adu-Gyamfi
Malaria is a serious mosquito-borne disease caused by the plasmodium parasite. It is transmitted when an infected mosquito bites a person. Early diagnosis of malaria is critical in the diagnosis of malaria before it multiplies and leads to death. The golden standard for diagnosis of malaria is the microscopic examination. The major drawback of this approach is that, it is time consuming and labour intensive. According to the WHO`s 2020 World malaria report, there was an estimated 241 malaria cases and 627,000 deaths worldwide in 2020 . About 47,000 of these cases were linked to improper diagnosis and disruptions. A study in Ghana found that there were only 1.72 microscopes per 100,000 population but only 0.85 trained technicians per 100,000 population . Due to this unavailability of microscopes and skill technicians, diagnosis are made on the basis of clinical signs and symptoms alone. The purpose of this study is to determine the effectiveness of convolutional neural networks in categorizing microscopic blood smear images as parasitized or healthy. This research aims to explore how the recent advancements in Artificial intelligence and Deep learning can be used to automate the diagnosis of malaria parasite. Two deep learning models VGG-19 and ResNet-50 will be largely examined and compared to determine the bet performing model on a malaria dataset.
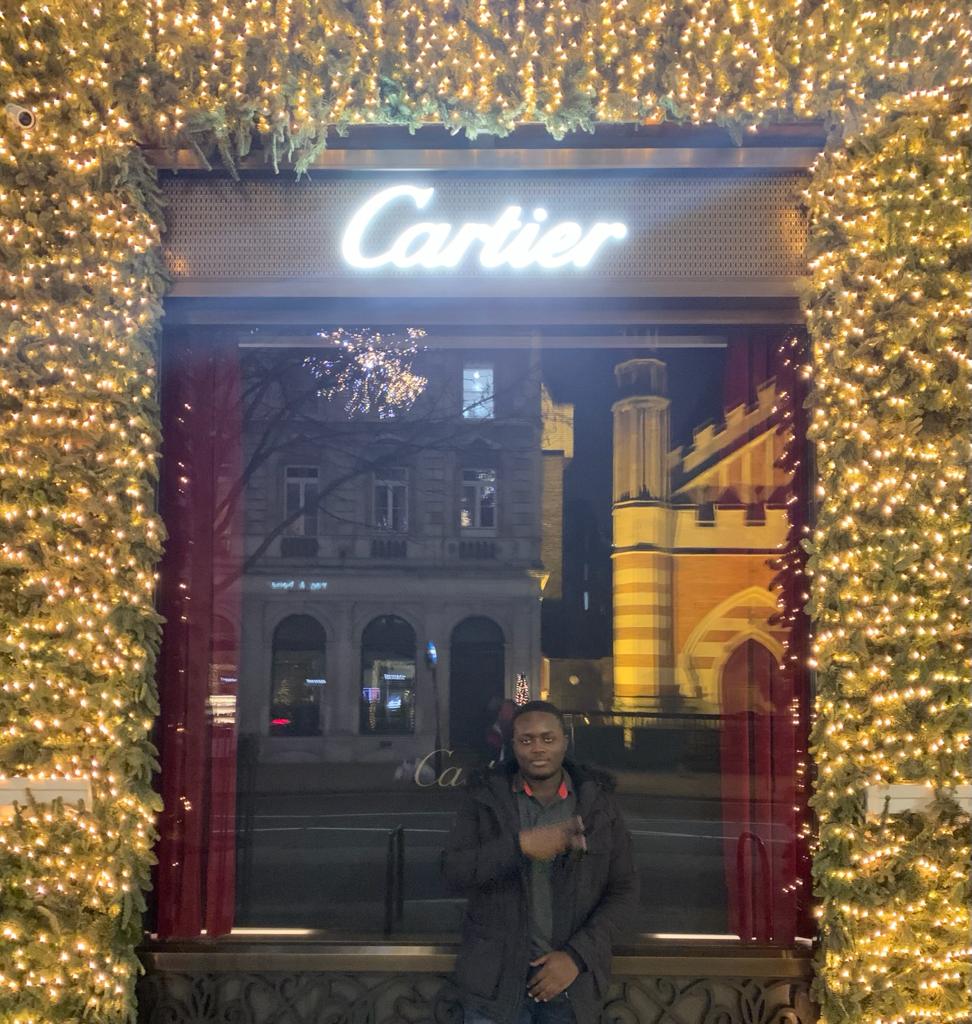
Rooney Adu-Gyamfi
Introduction
Malaria is an infectious mosquito borne disease the is caused by the plasmodium parasite. It is transmitted when an individual is bitten by an infected mosquito. According to the WHO`s 2020 World malaria report, there was an estimated 241 malaria cases and 627,000 deaths worldwide in 2020 (WHO, 2021). About 47,000 of these cases were linked to improper diagnosis and disruptions.
Malaria is more commonly found in underdeveloped areas where the diagnosis of medical conditions are not easily accessible. The plasmodium parasite works by infecting the liver and multiplying in large numbers then attacking the red blood cells. If this is not diagnosed properly, it could lead to untimely death to the human infected with it. An early diagnosis is essential for the treatment of malaria. The medically accepted form of diagnosis worldwide is the light microscopy technique. Microscopic diagnosis while currently properly is long and tedious and also requires skilled technicians which may take about 15-20 minutes to count all the cells. A study in Ghana found that there were only 1.72 microscopes per 100,000 population but only 0.85 trained technicians per 100,000 population (Bates, 2004). Due to this unavailability of microscopes and skill technicians, diagnosis are made on the basis of clinical signs and symptoms alone.
Research Aim
Light microscopy remains a good approach to diagnosing malaria however interpretation of the images is labour intensive intensive and limited to the skill level of the microscopist.The purpose of this study is to determine the effectiveness of convolutional neural networks in categorizing microscopic blood smear images as parasitized or healthy. A deep learning approach to malaria diagnosis will limit the need for a human expert and furthermore decrease the cost of equipment and minimize the use of electricity.
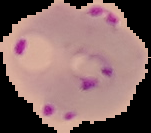
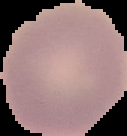
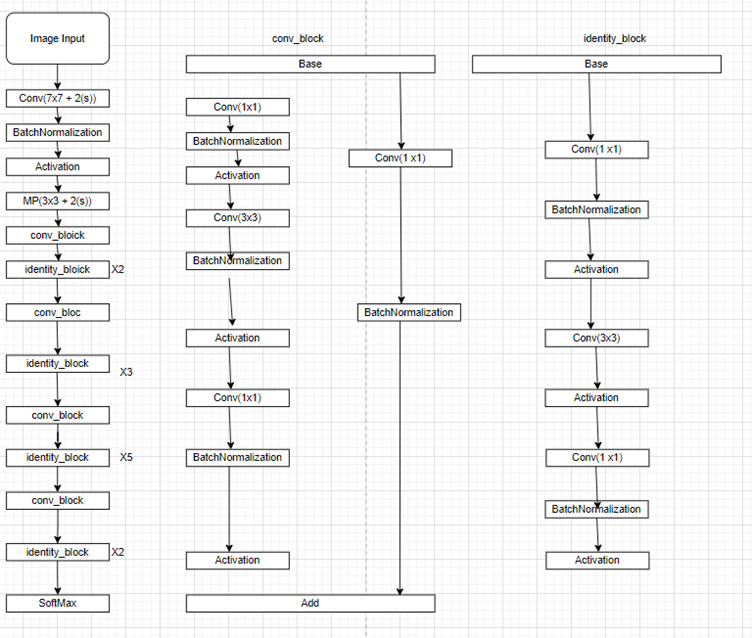
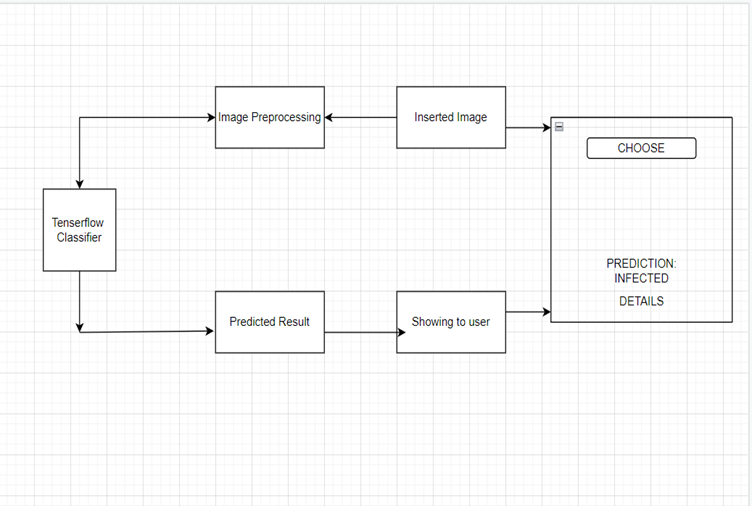
References
Bates, V. a.-A. (2004). Improving the accuracy of malaria-related laboratory tests. Brydegaard, M. M. (2011). Versatile multispectral microscope based on light emiting diodes. Review of Scientific Instruments, 82.- Ohrt, D. T. (1999). “Impact of microscopy error on protective efficacy estimates in. Clinical Pharmacology and Therapeutics, 134.
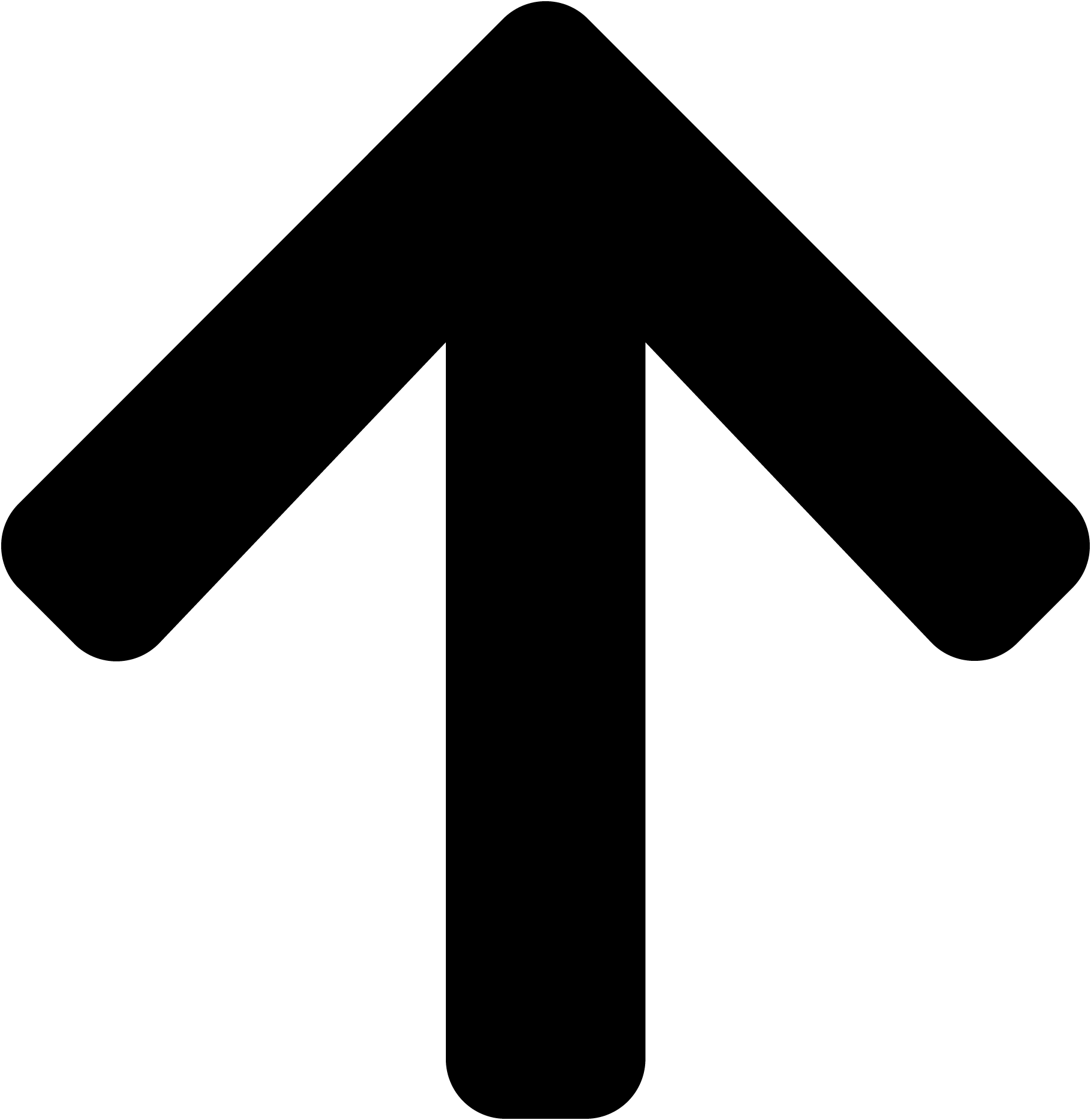
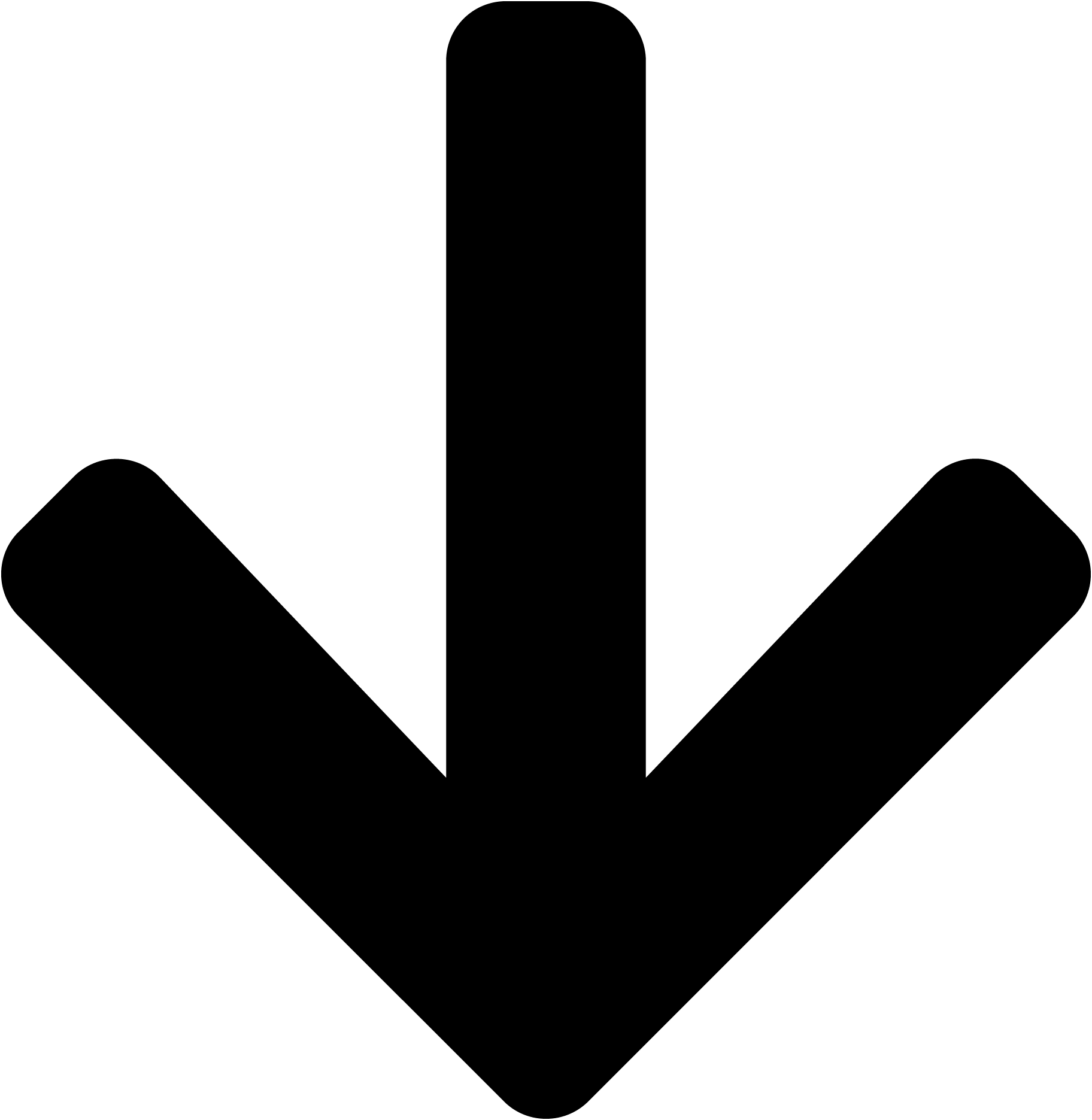
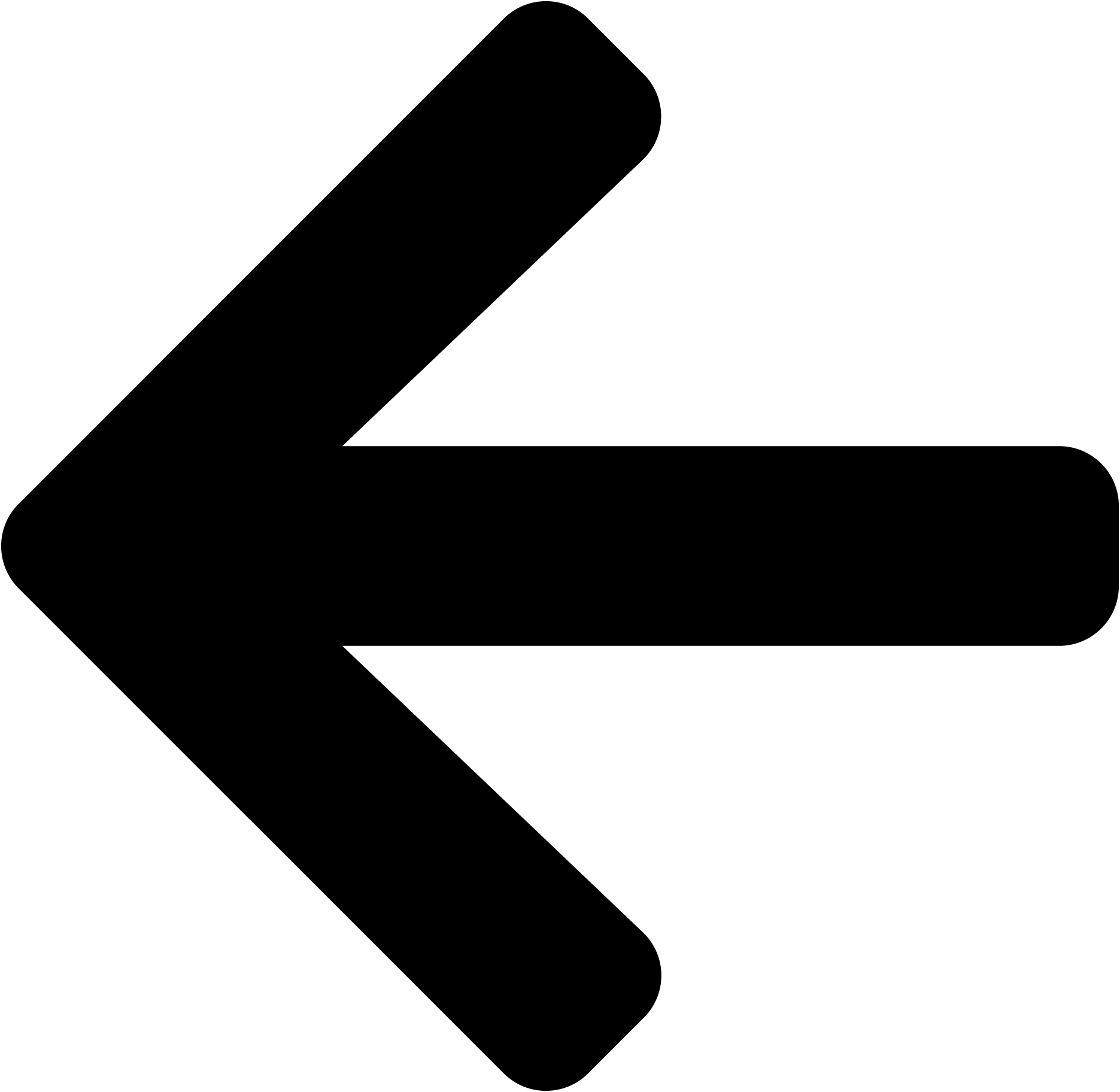
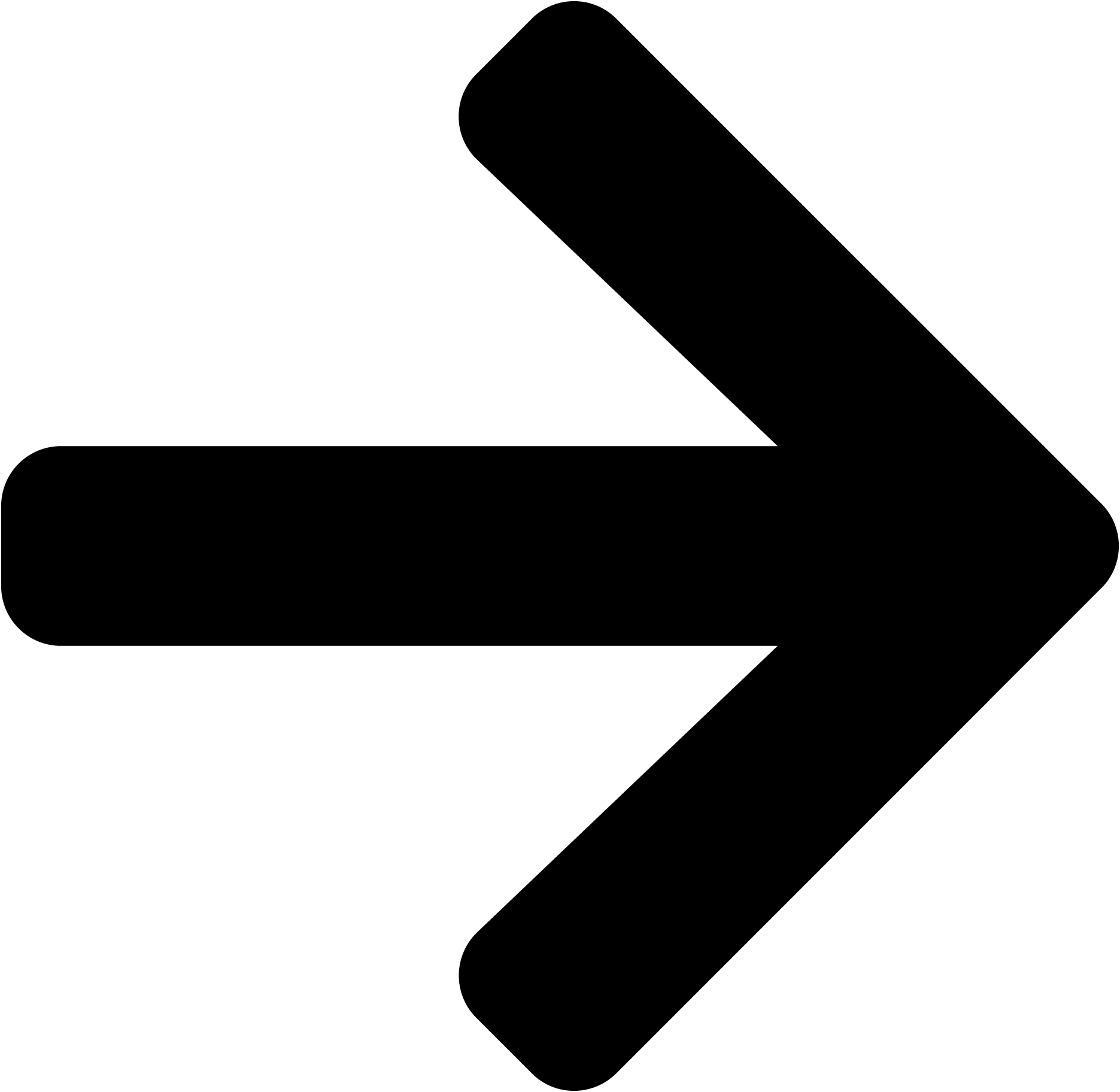
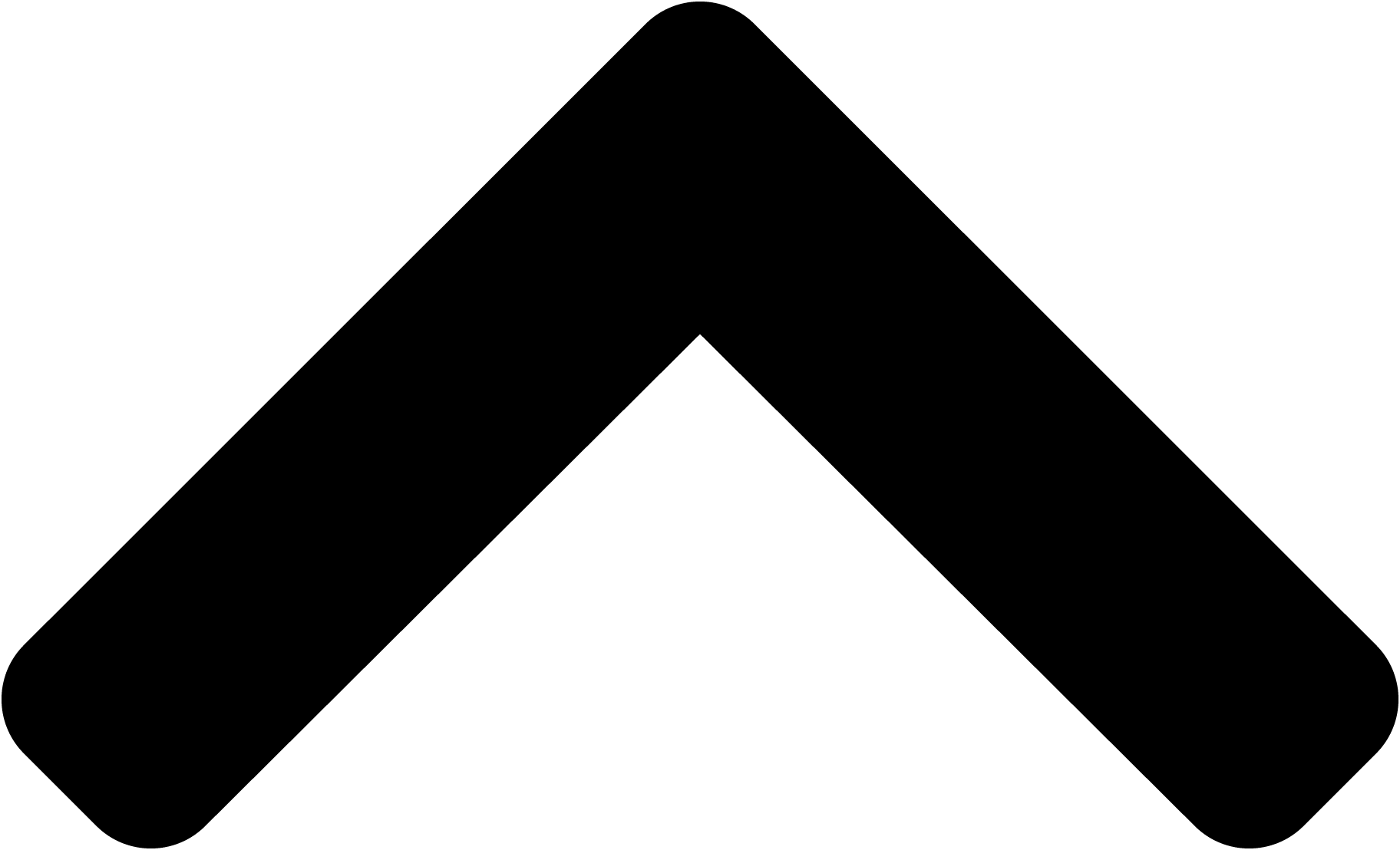
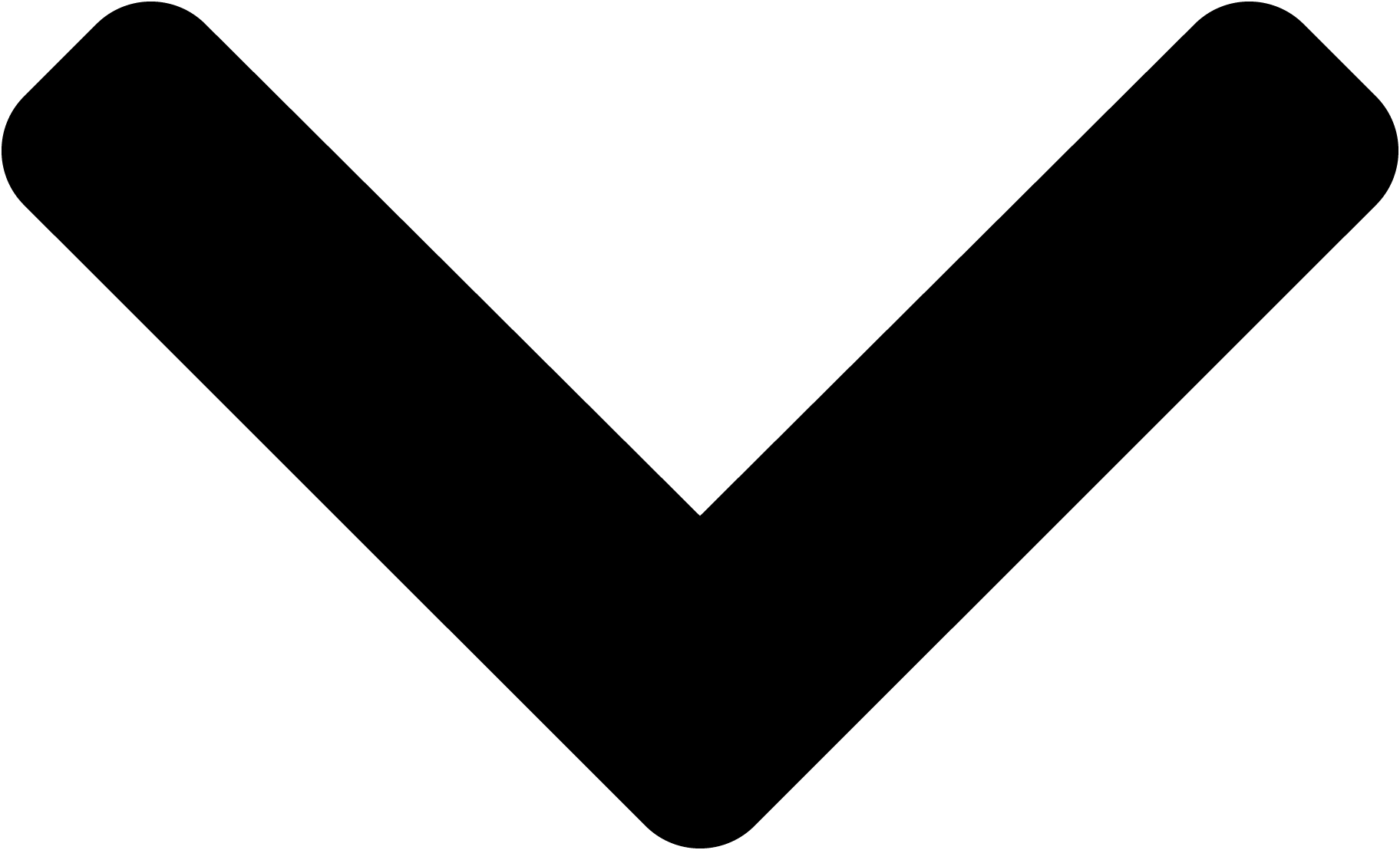
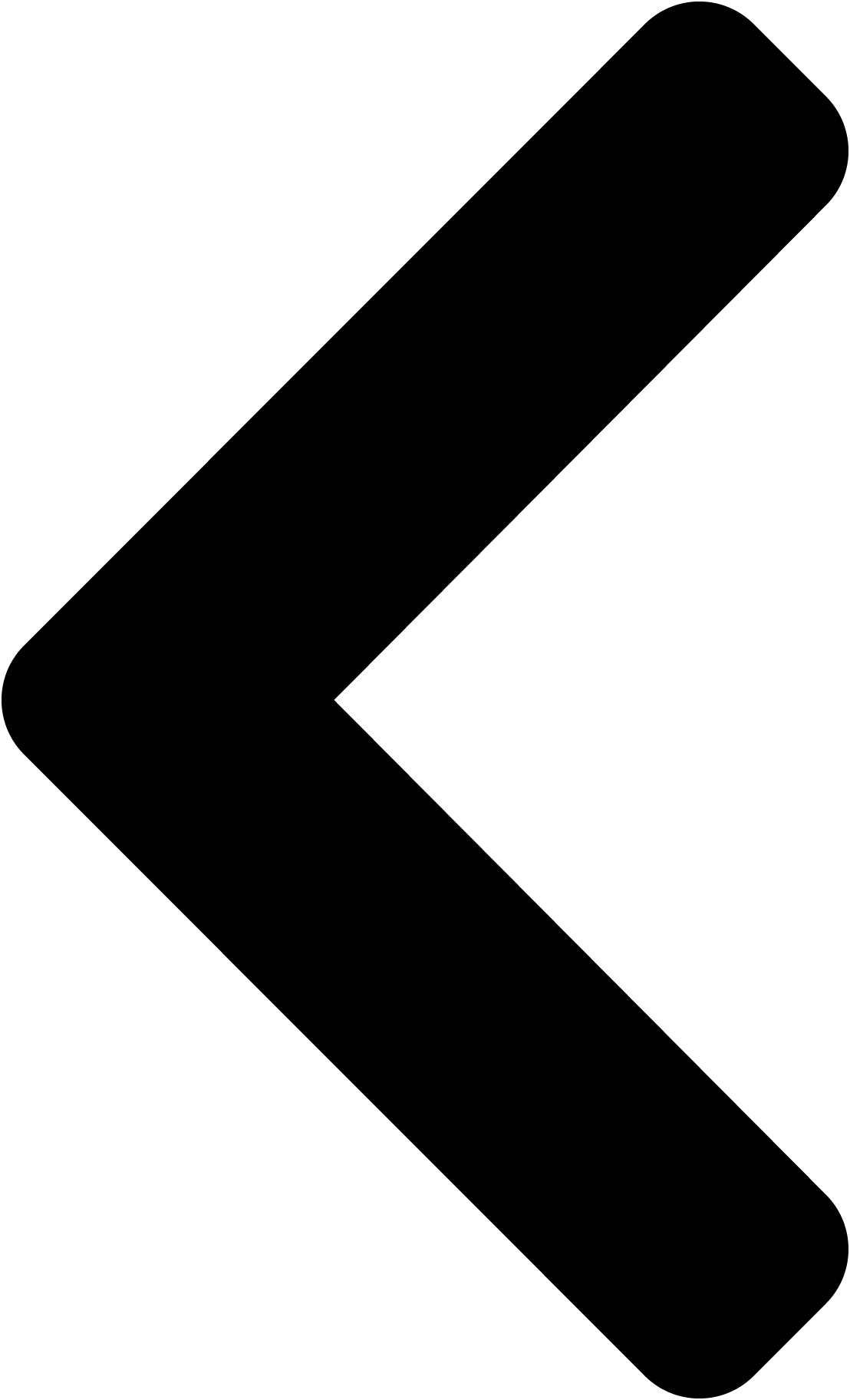
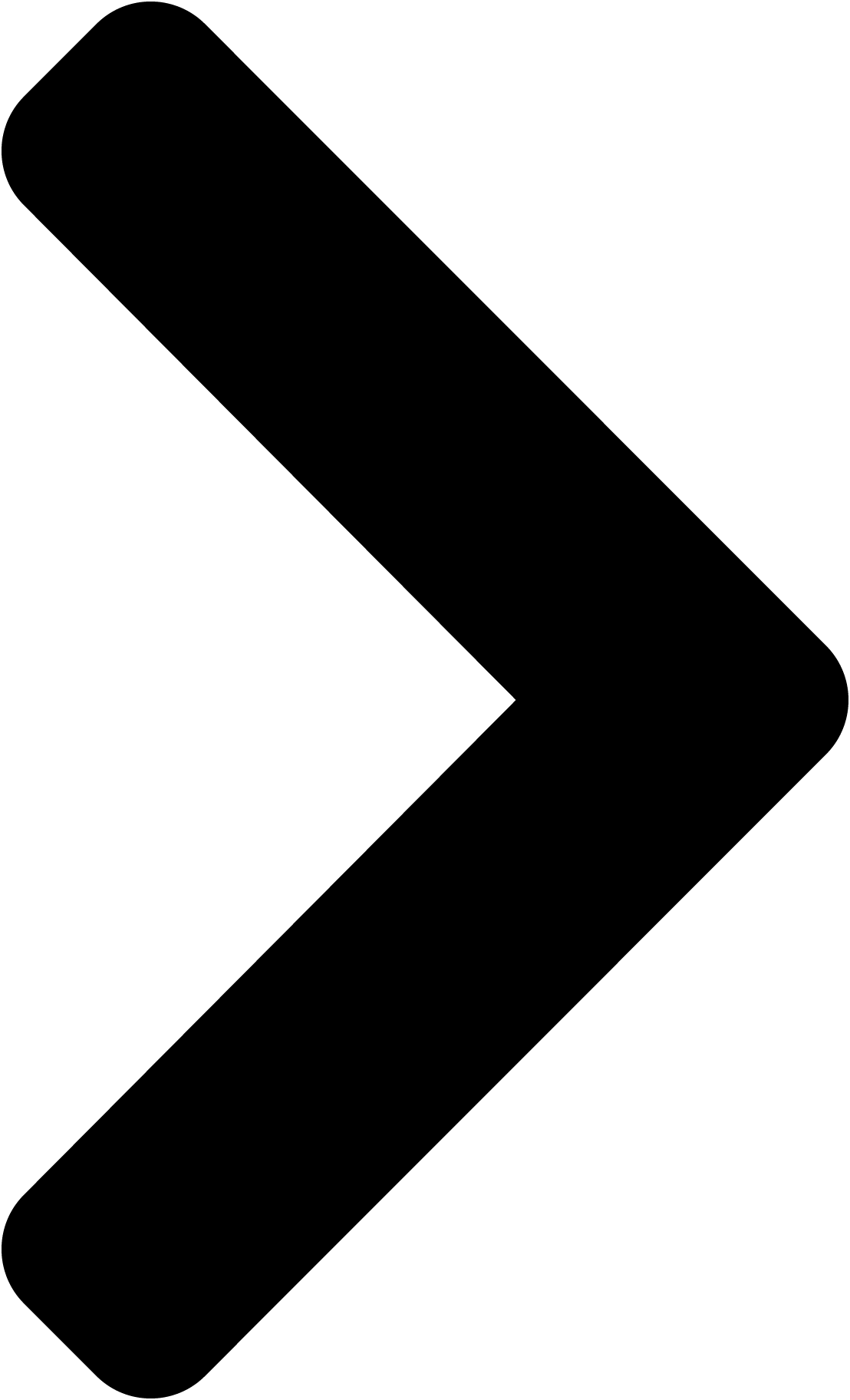
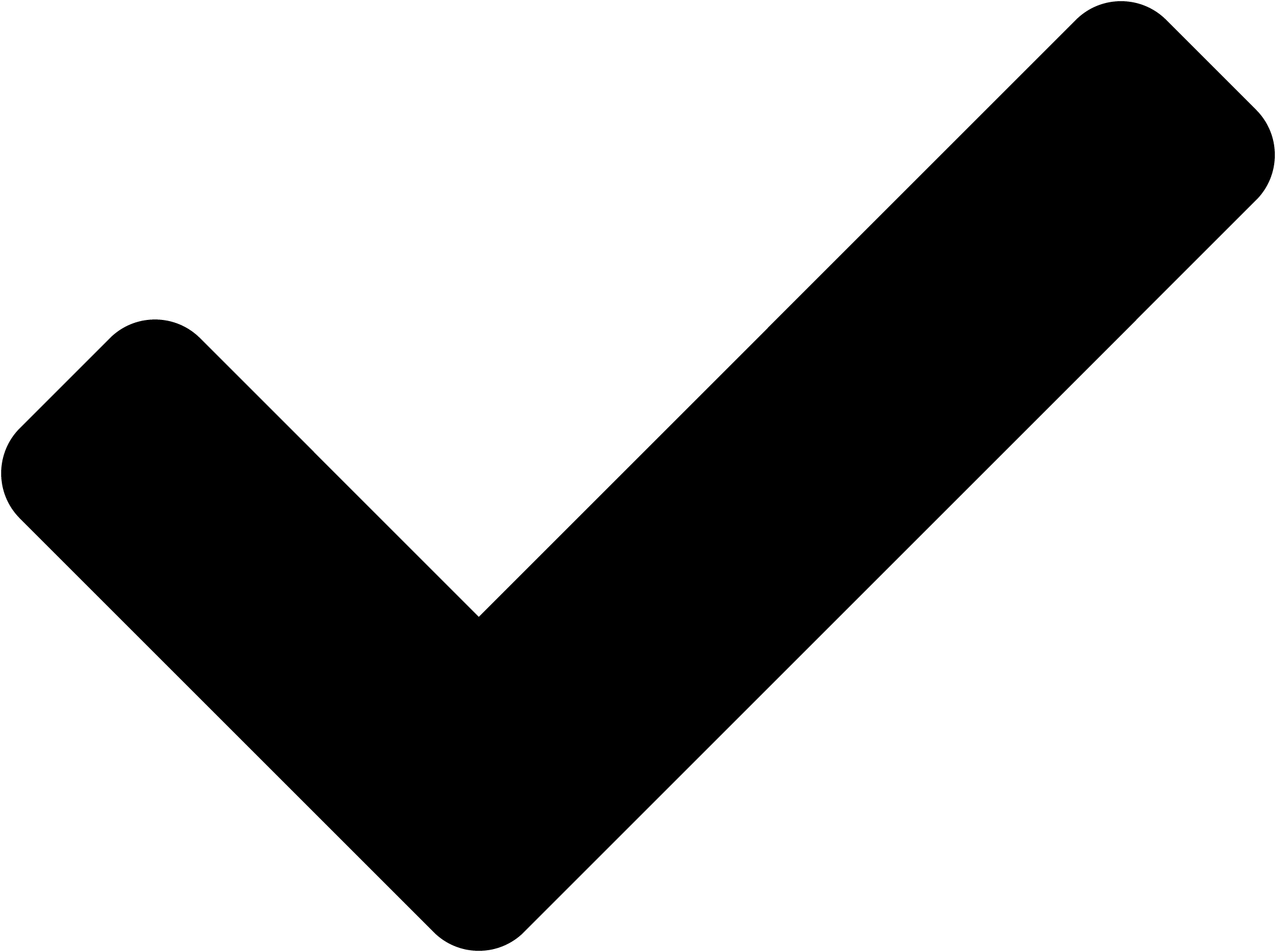
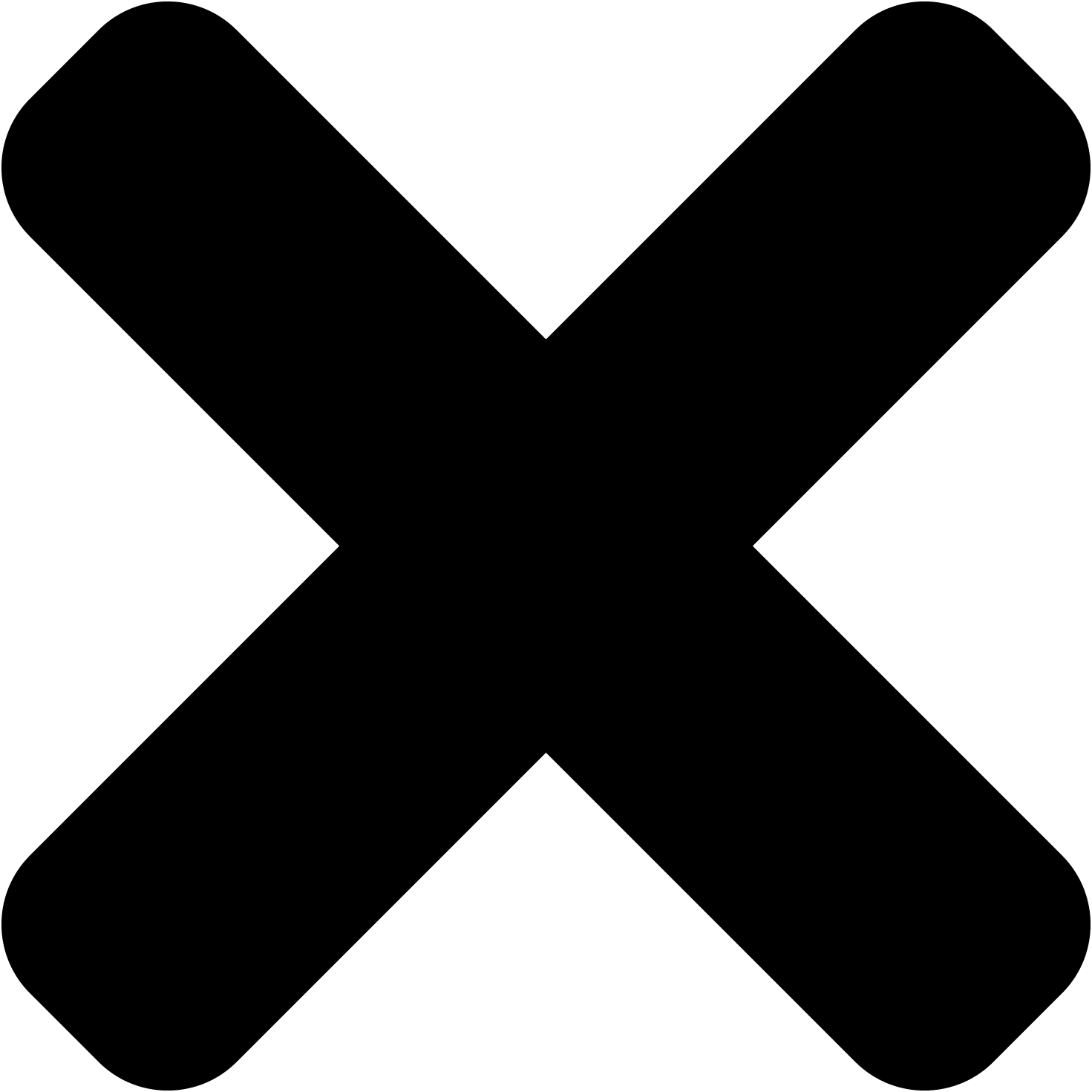
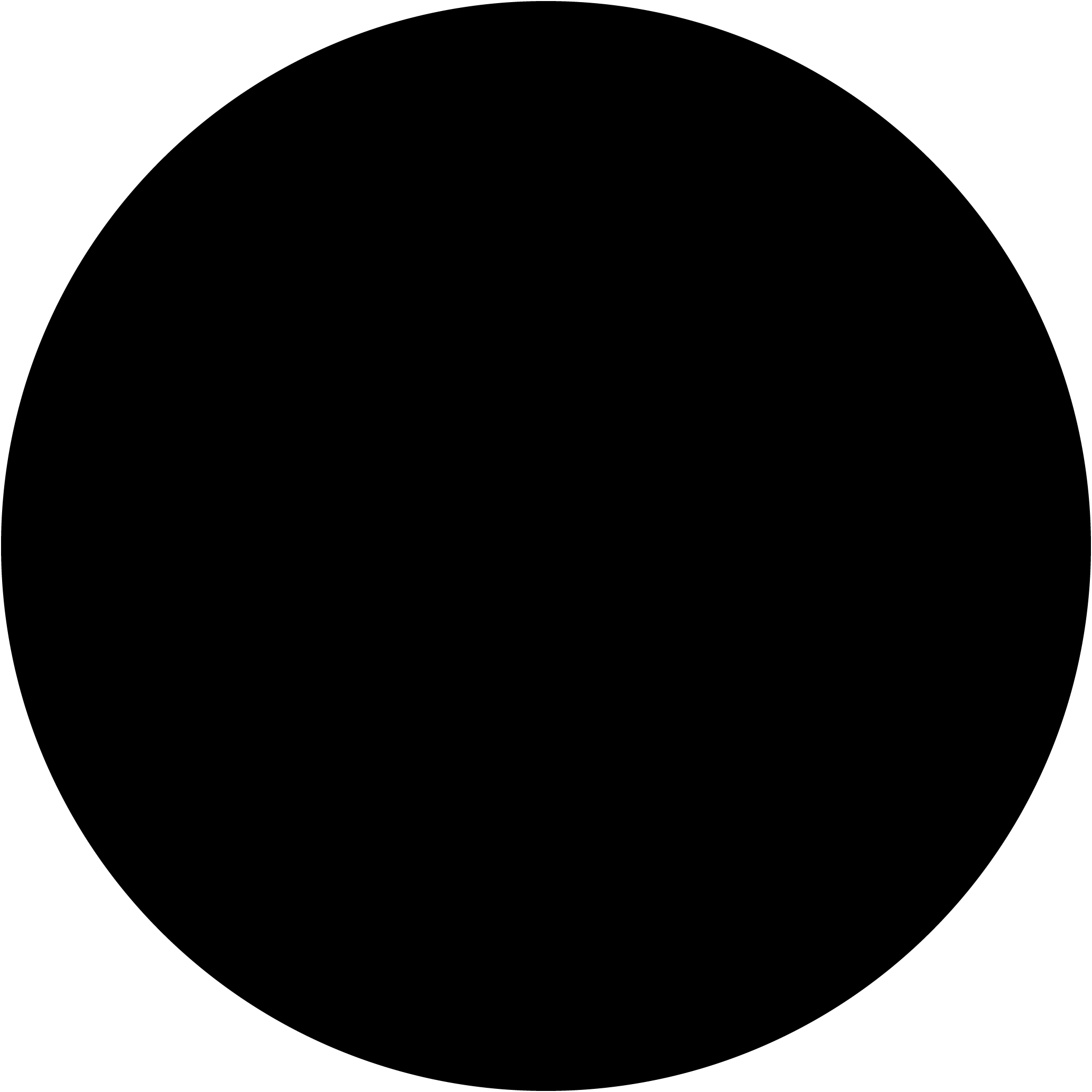
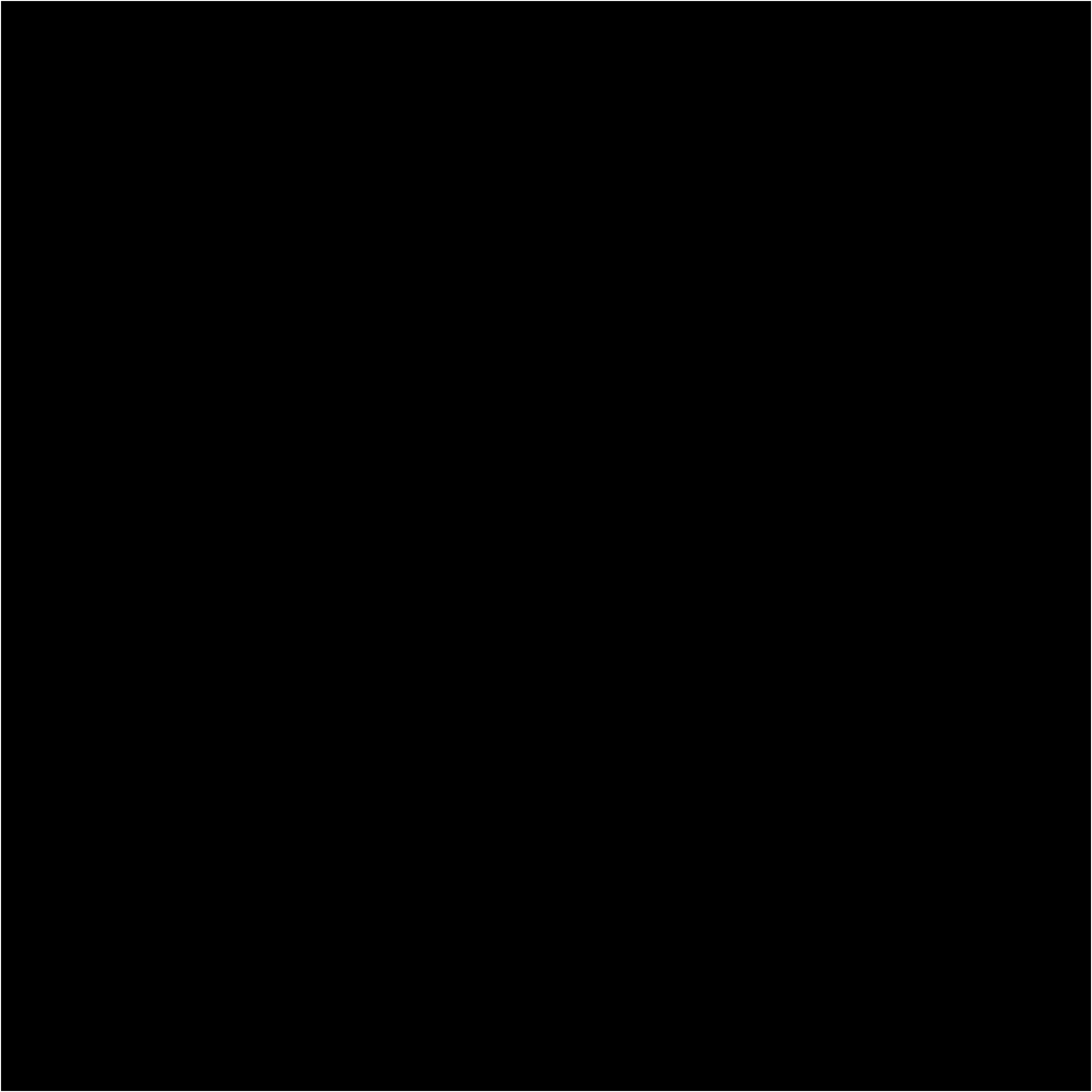
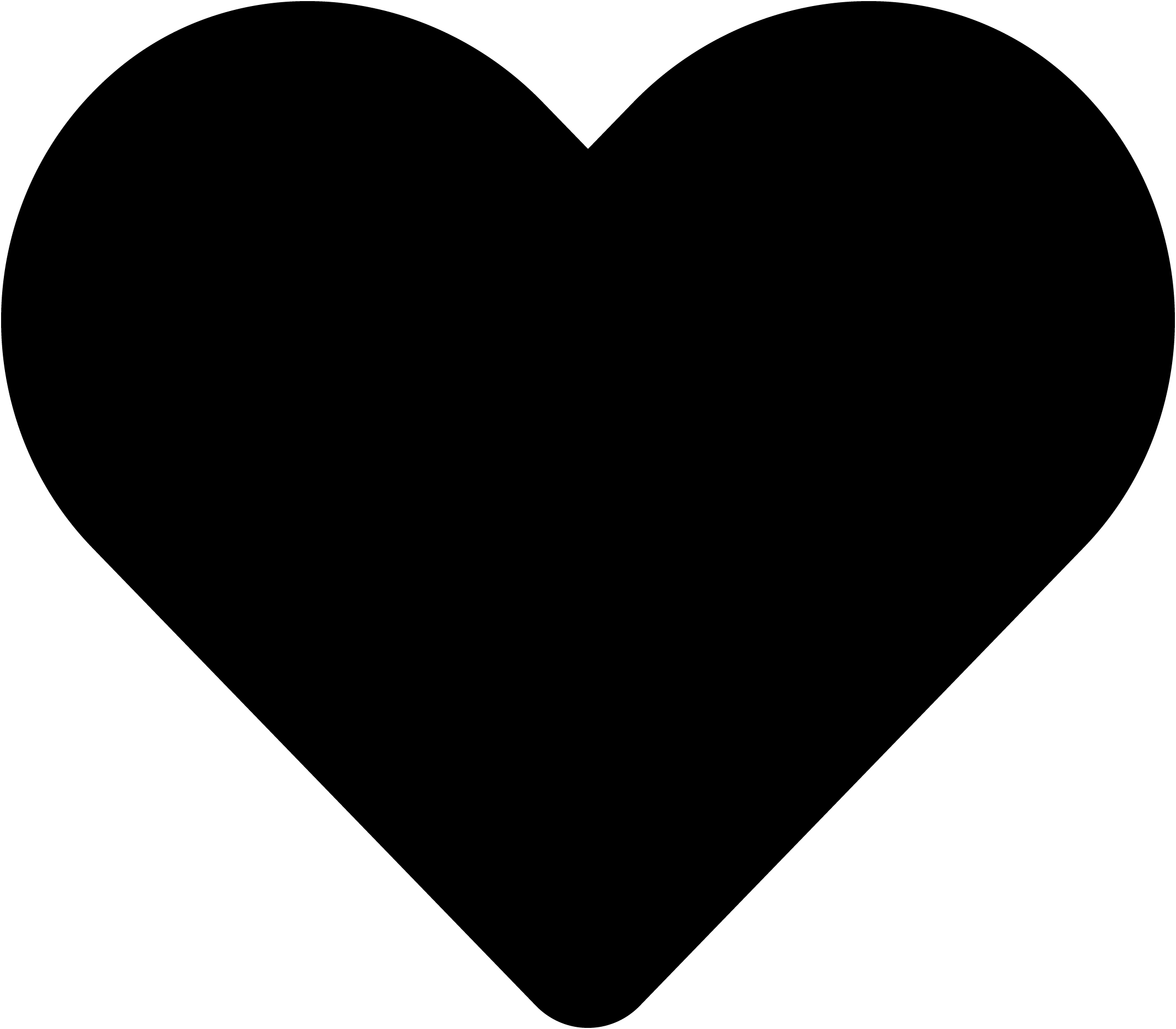
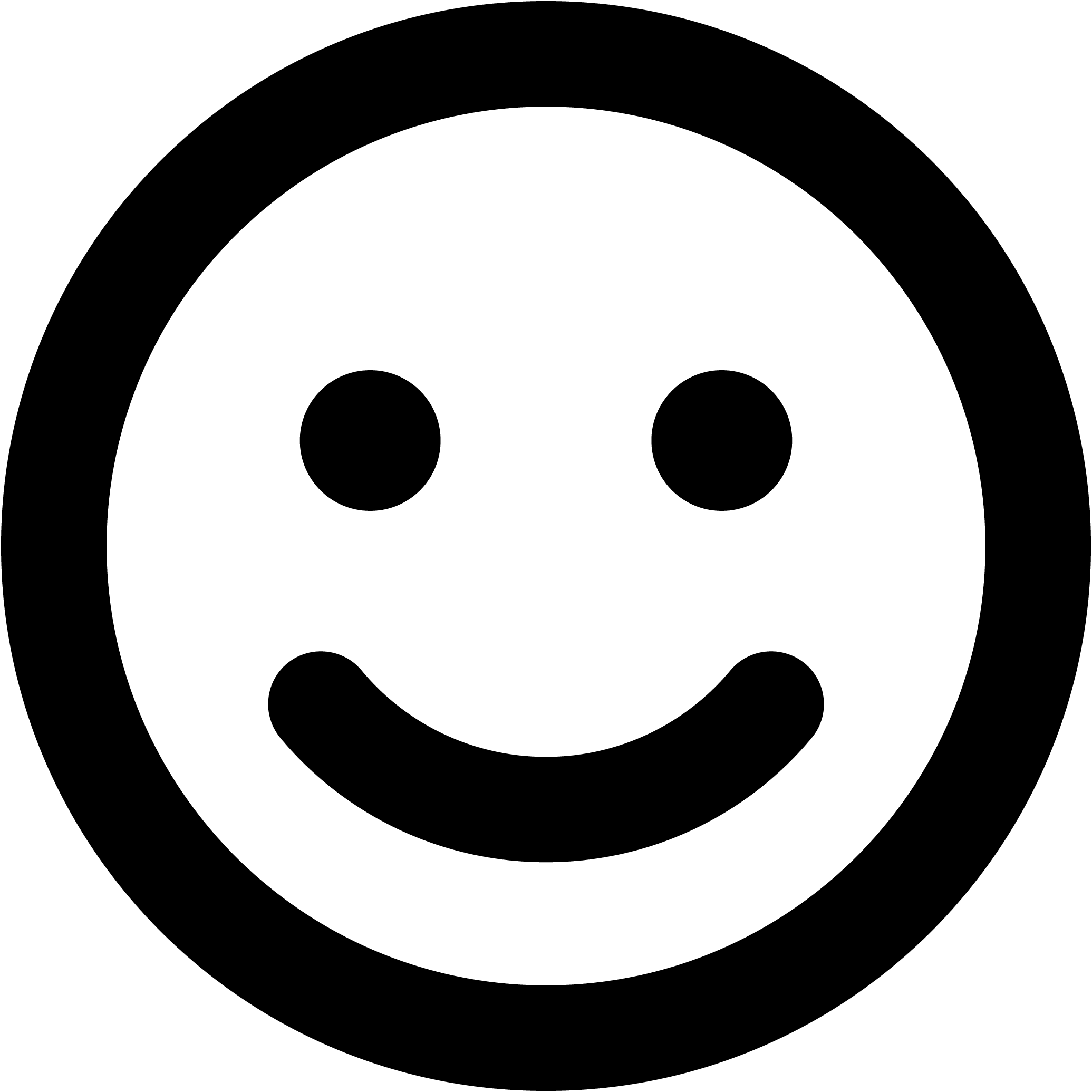
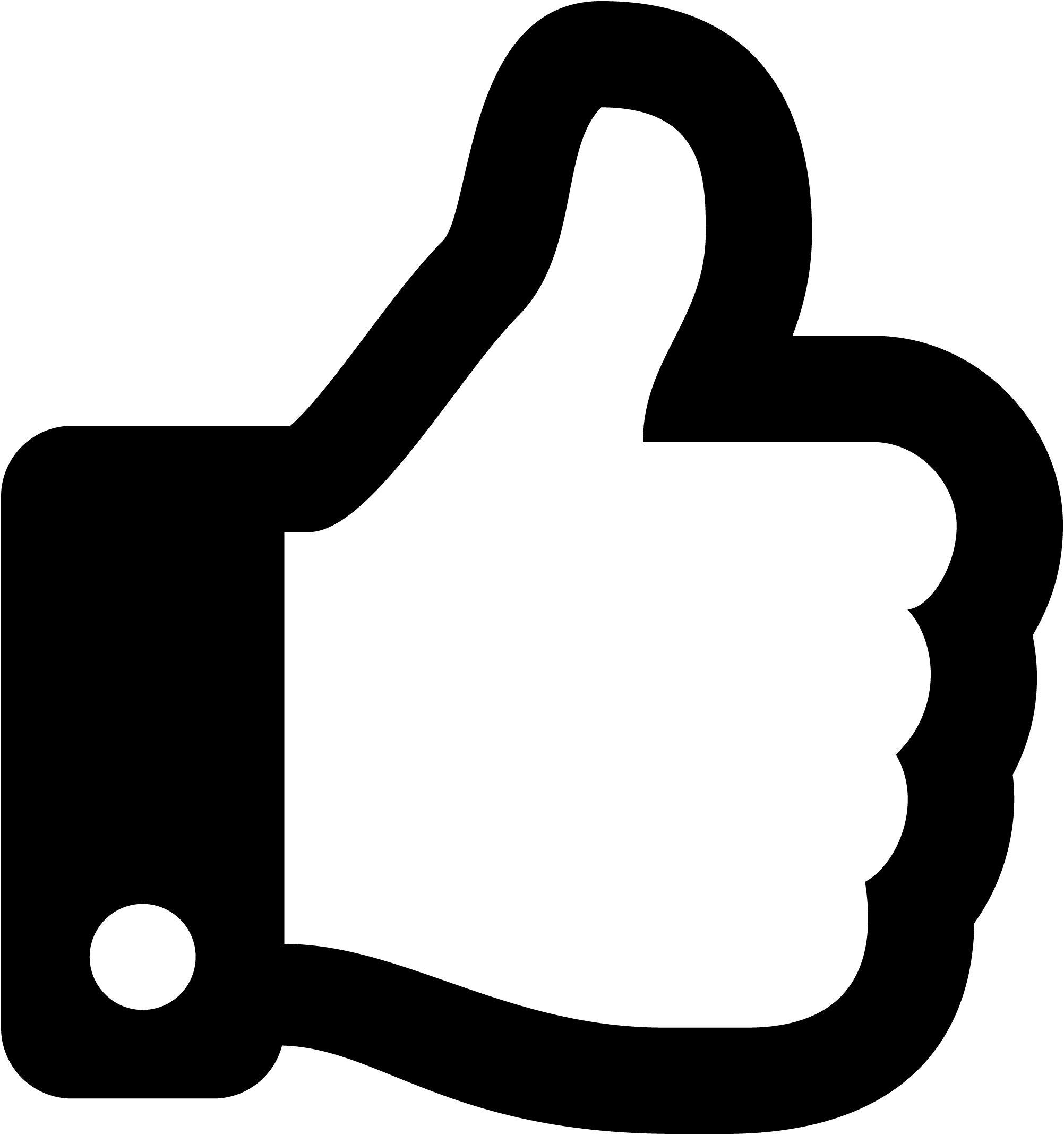
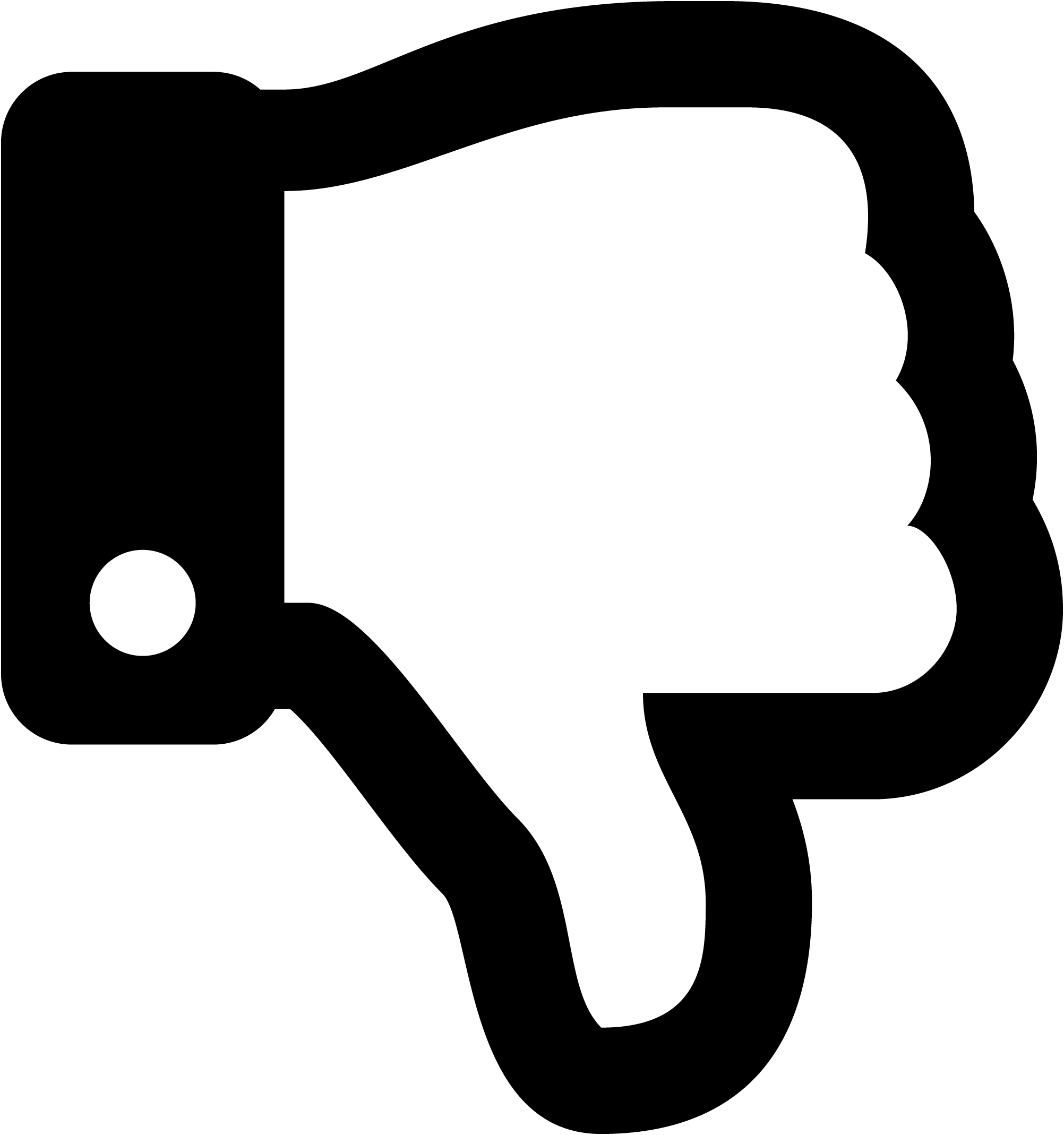
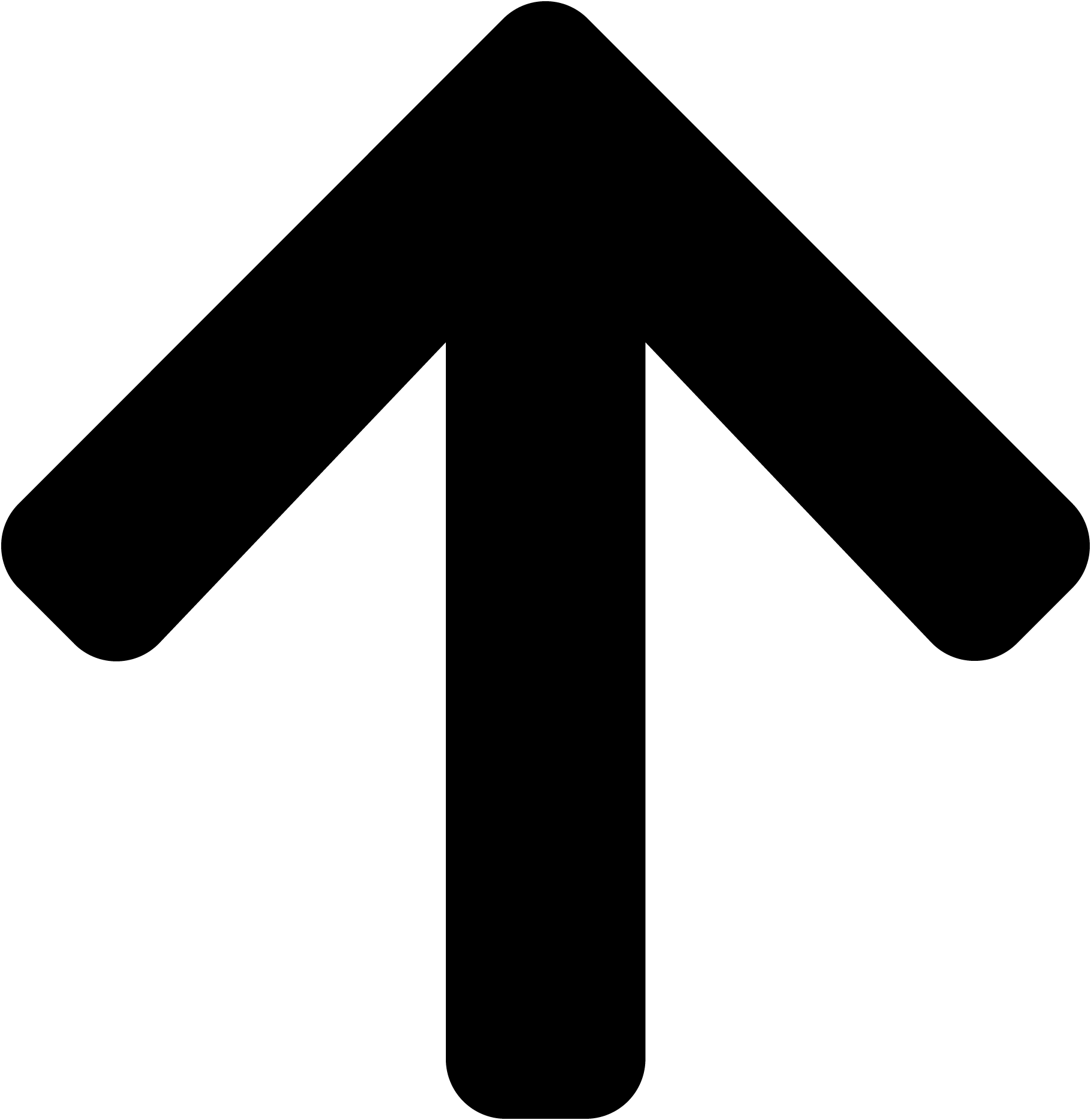
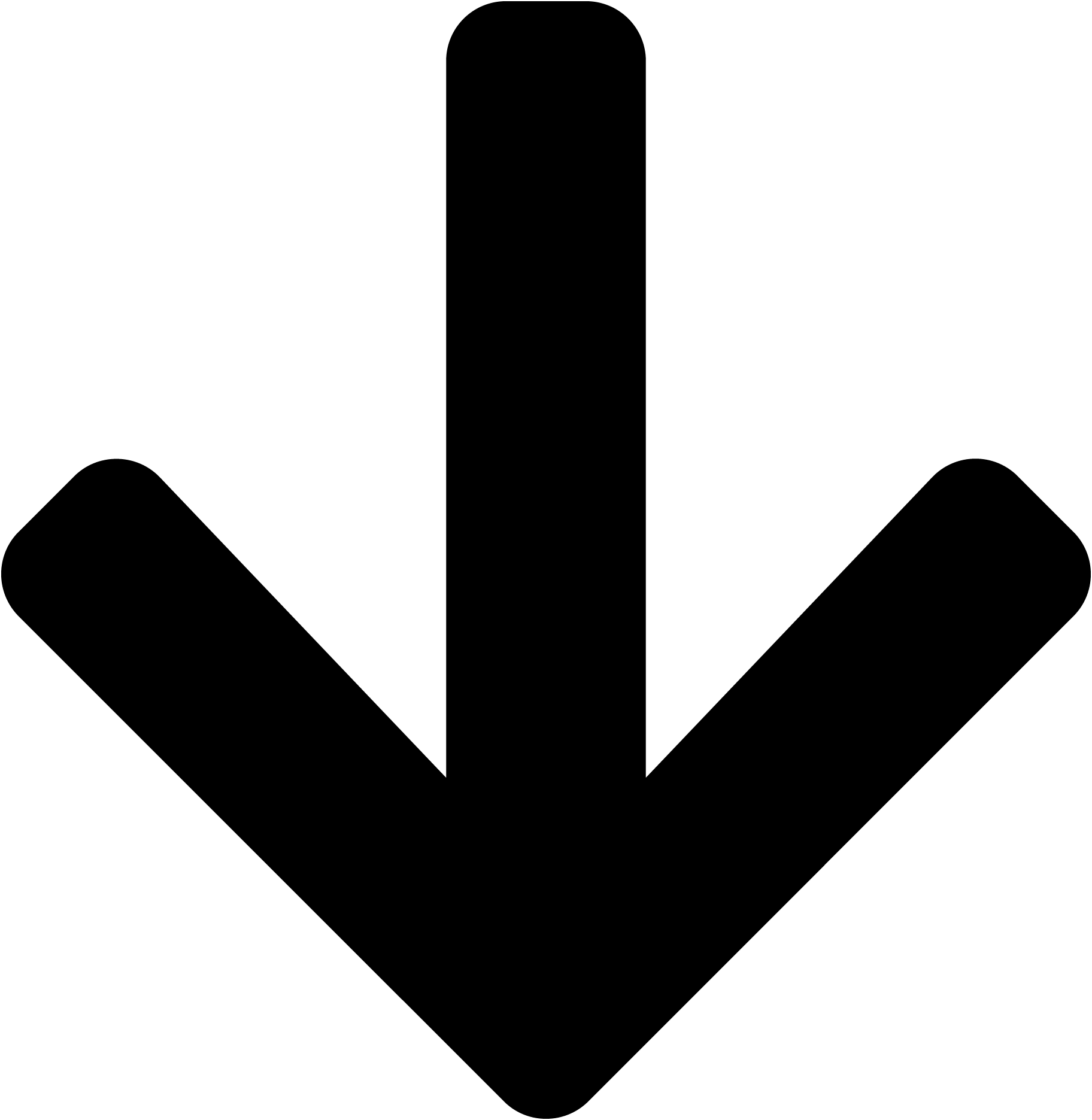
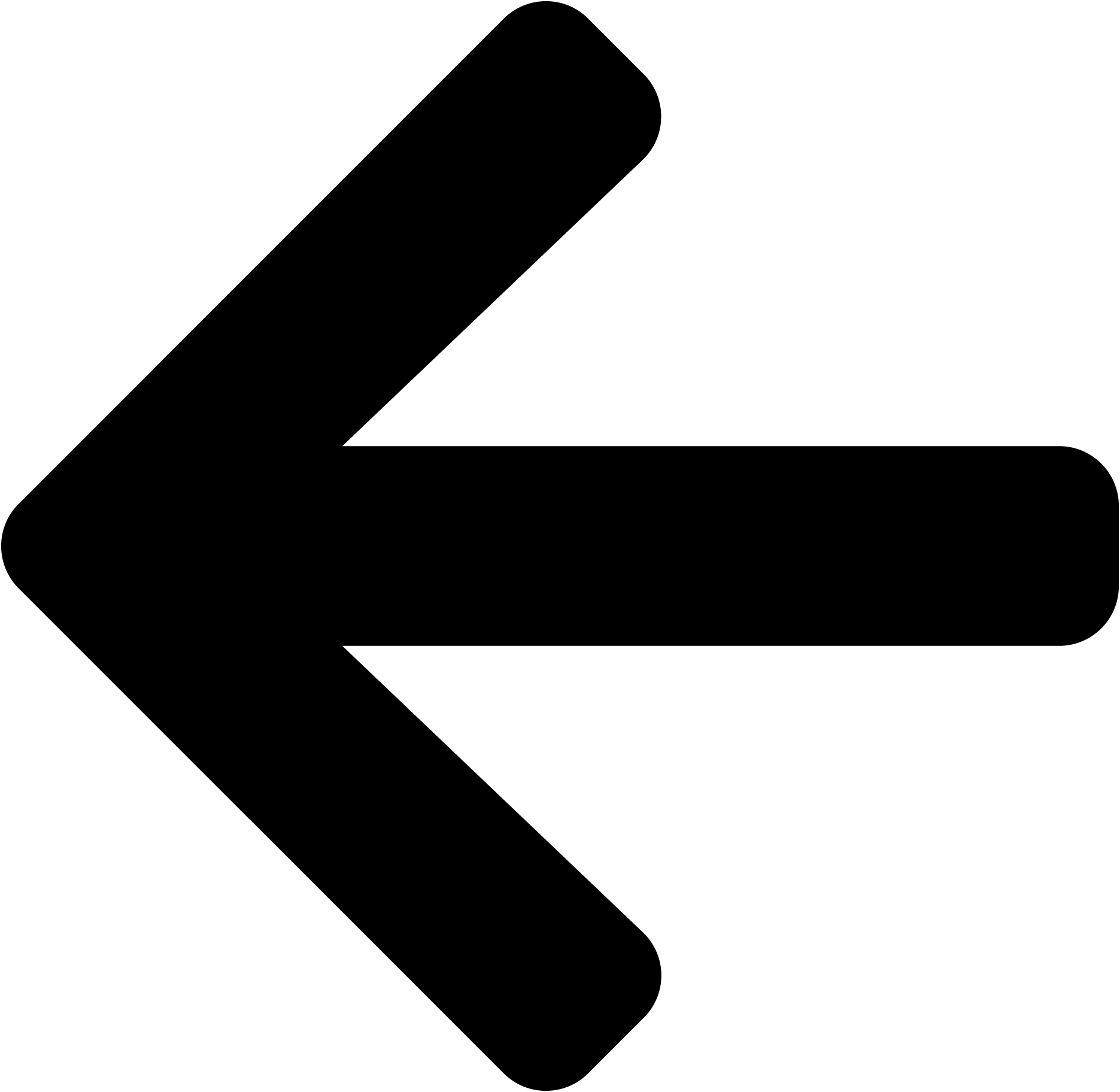
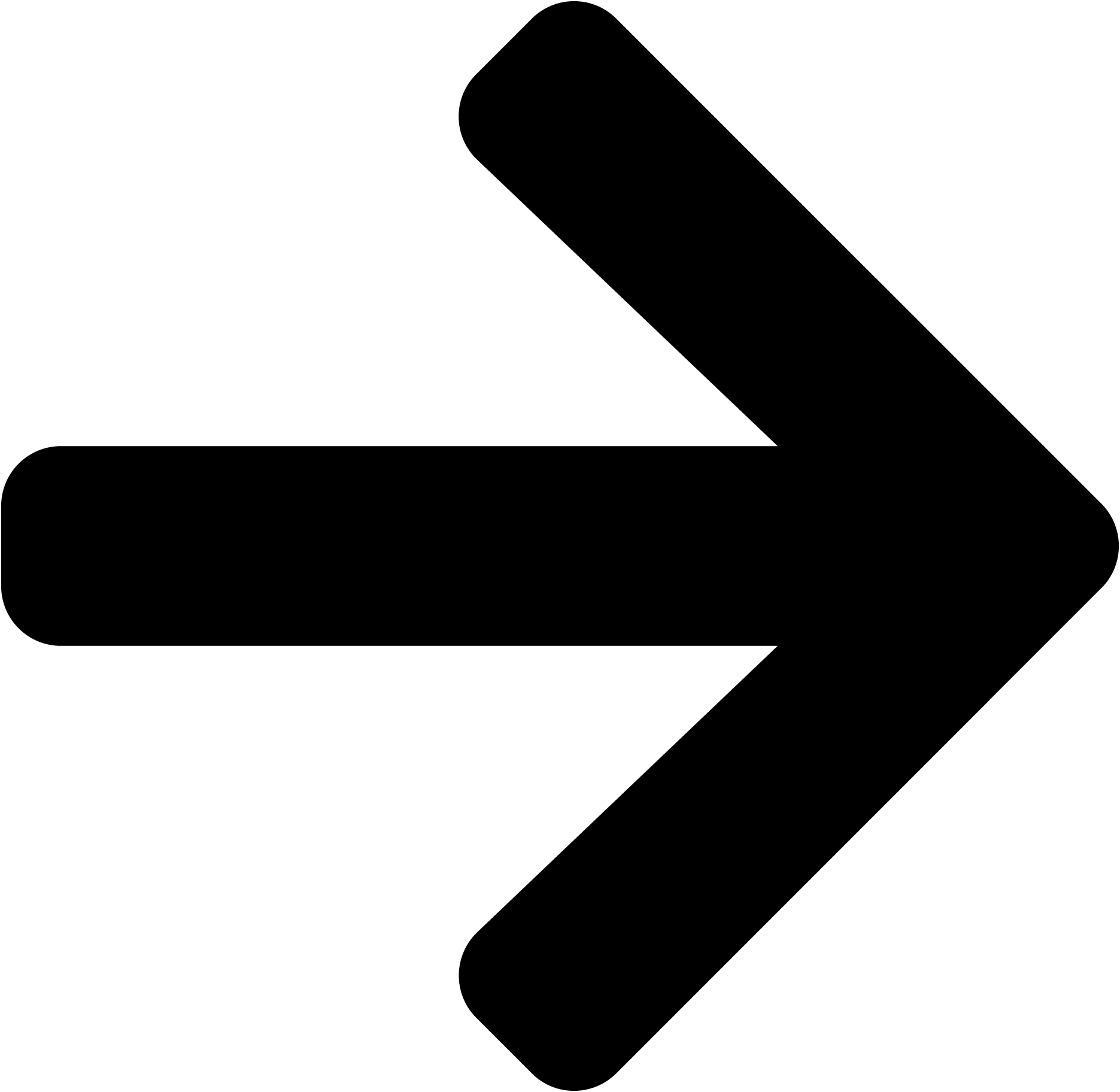
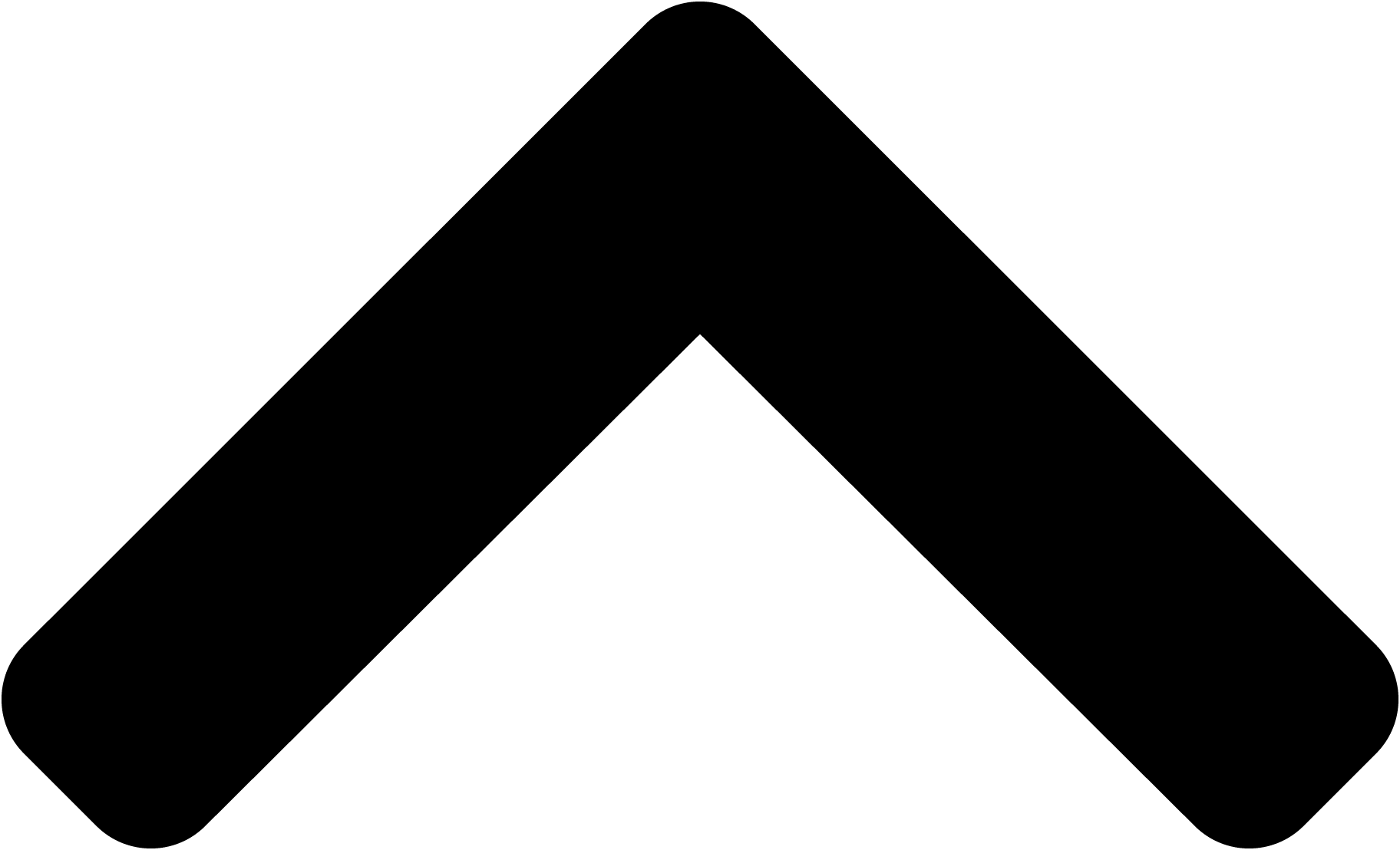
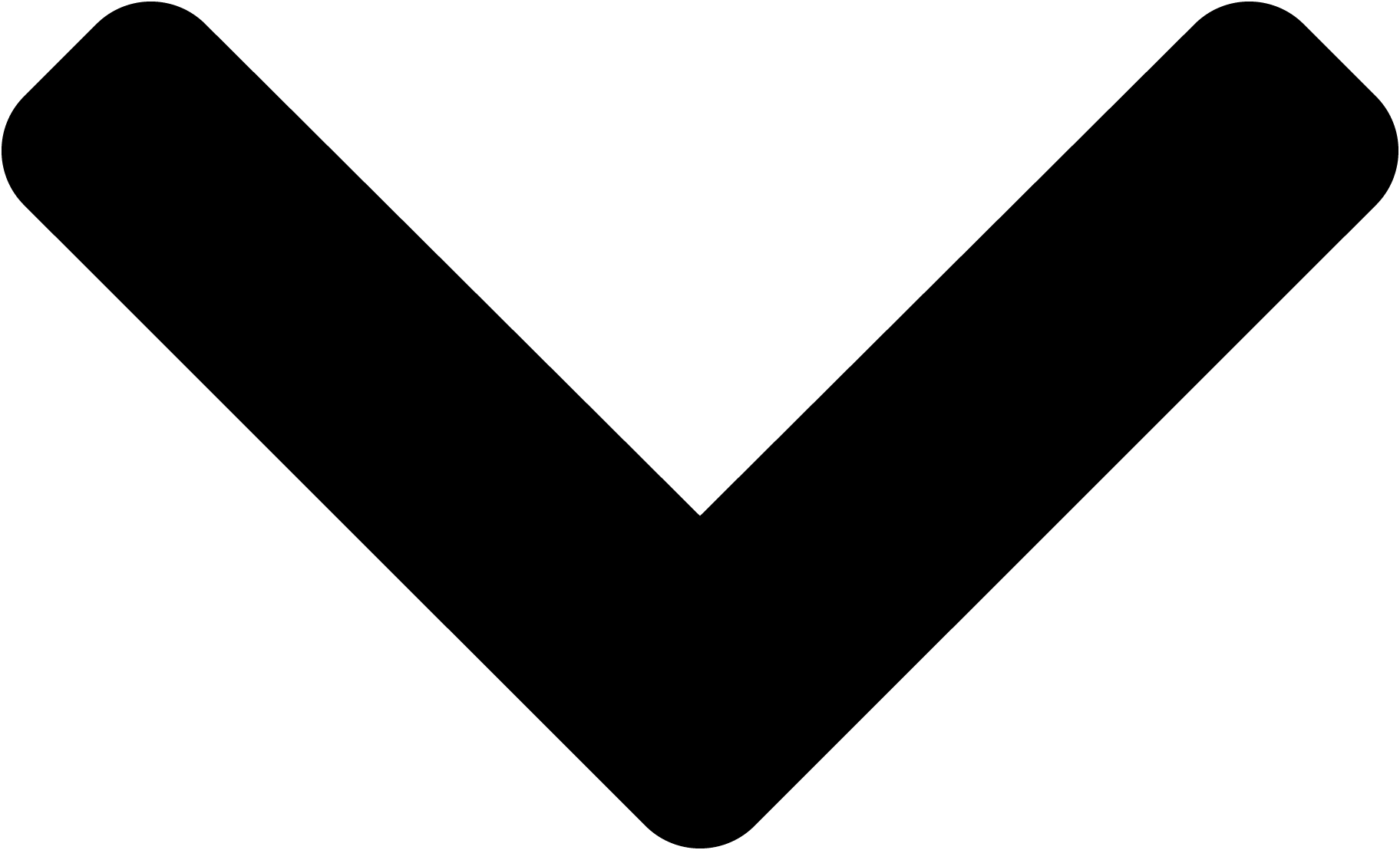
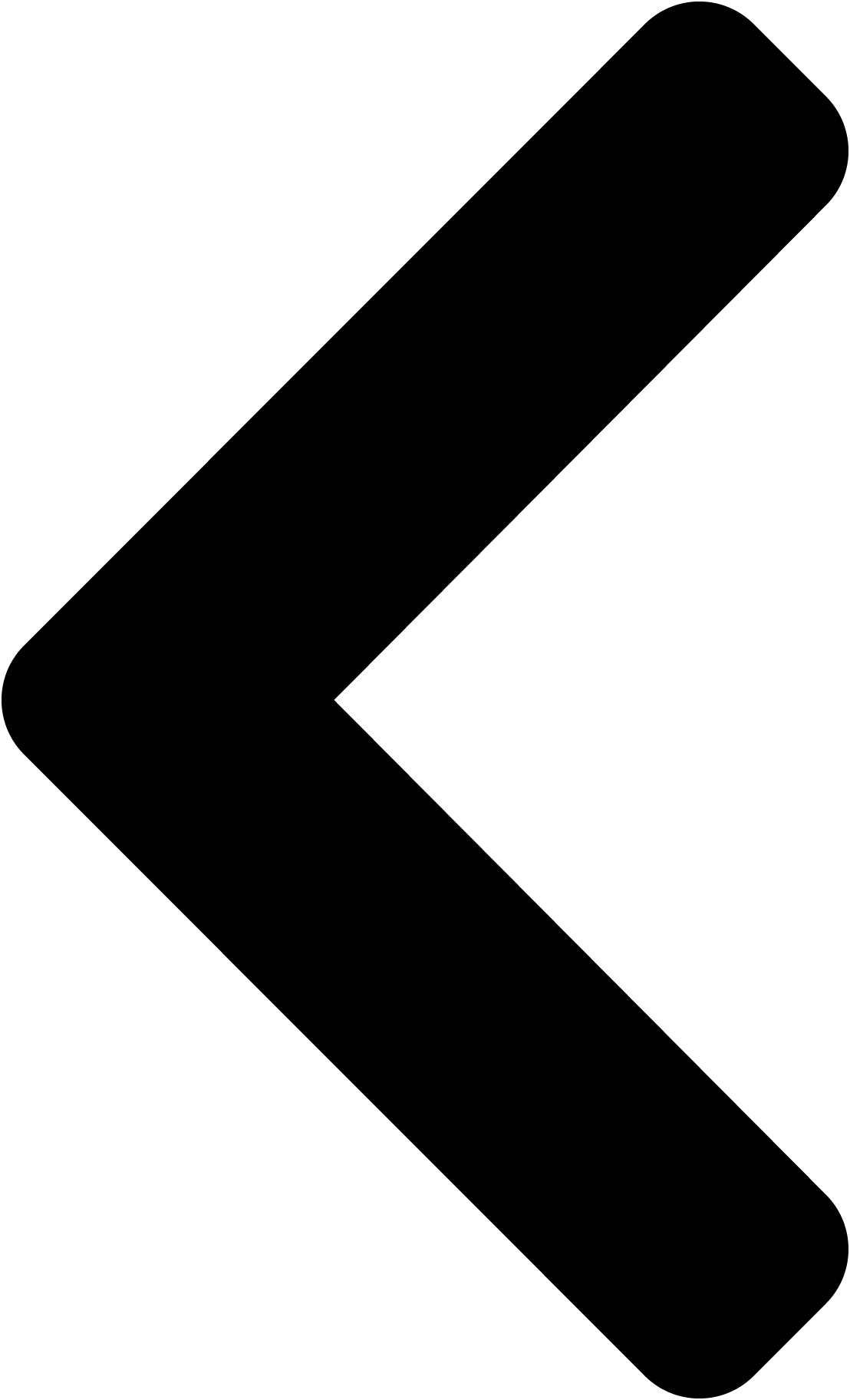
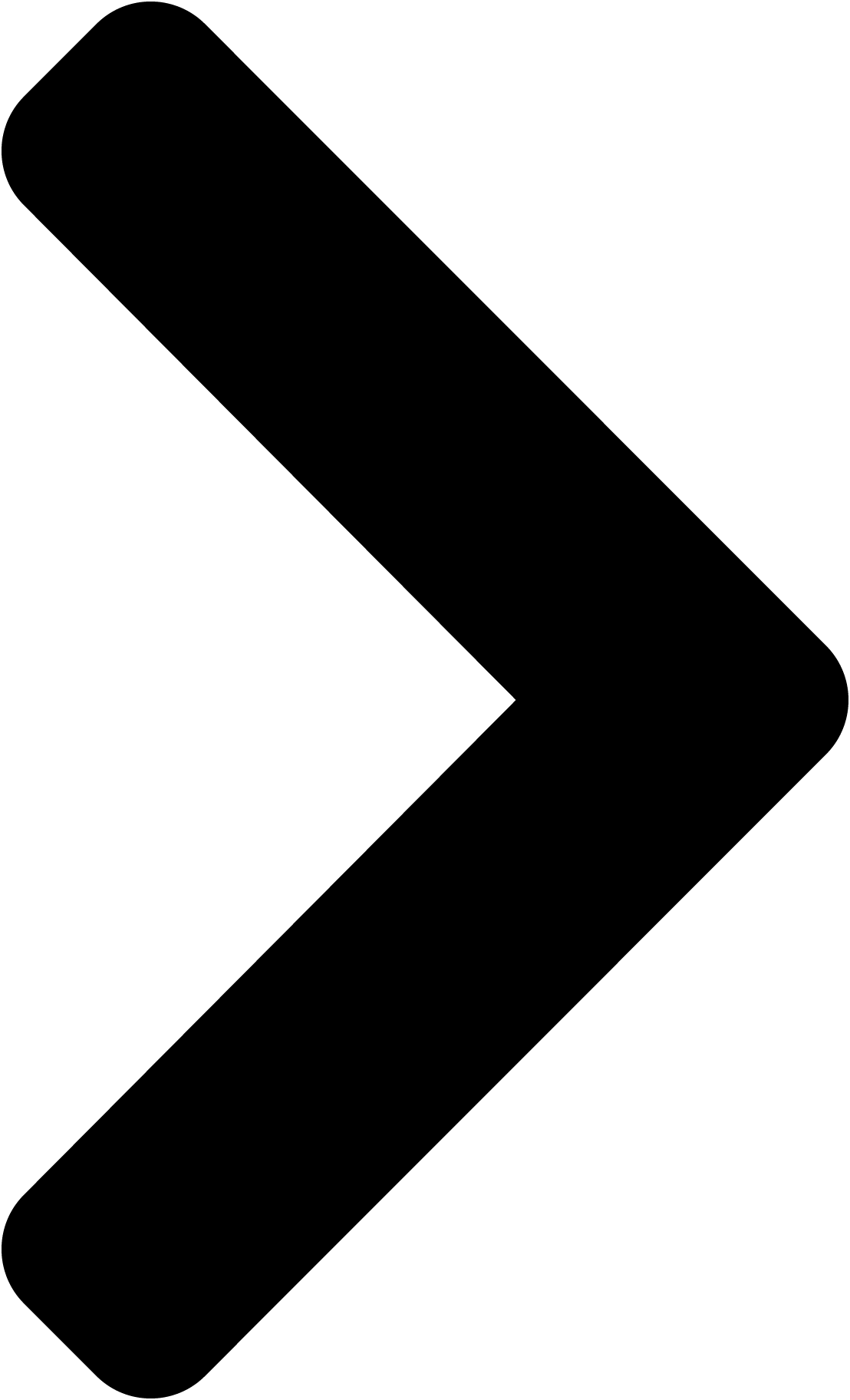
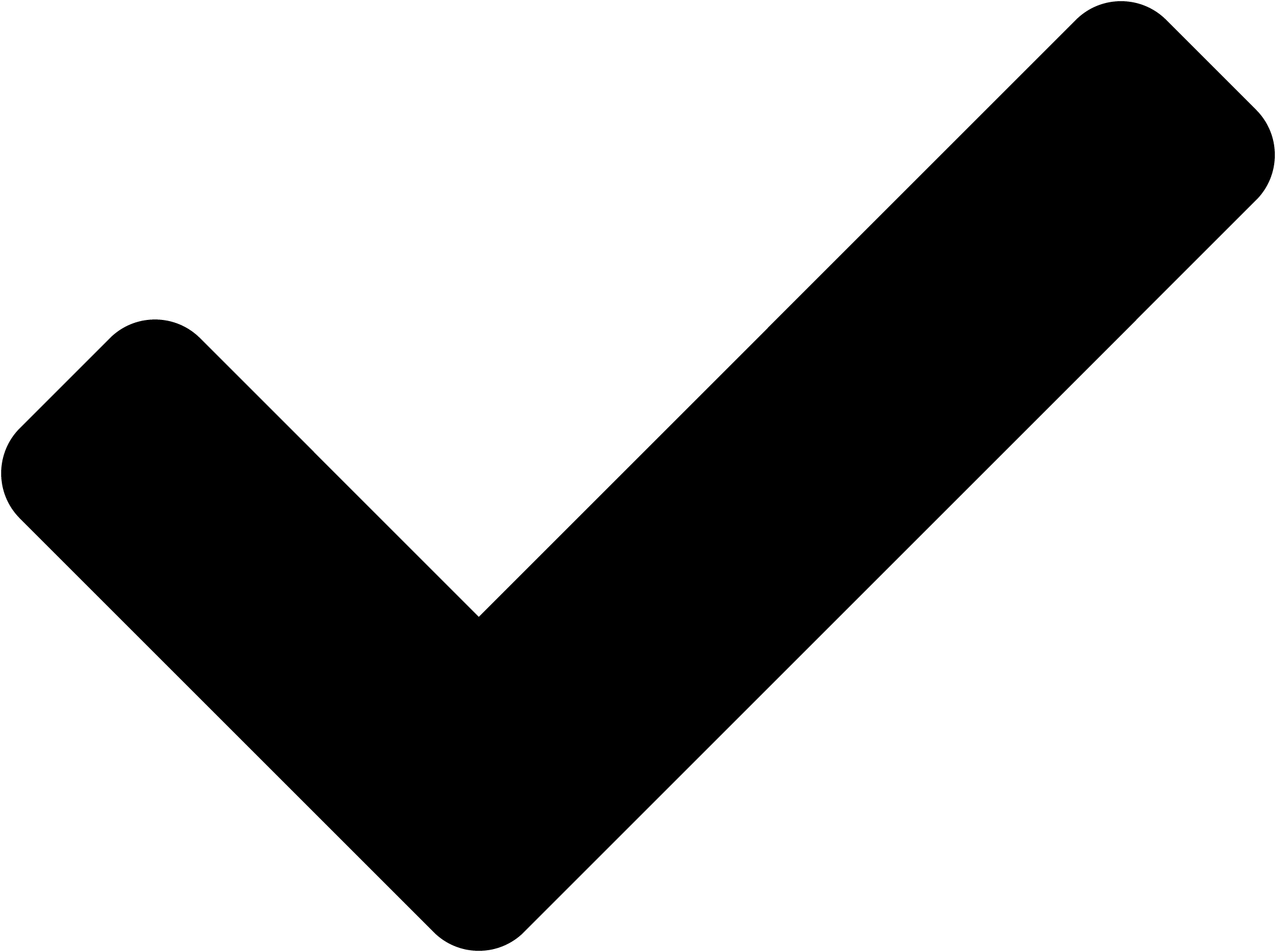
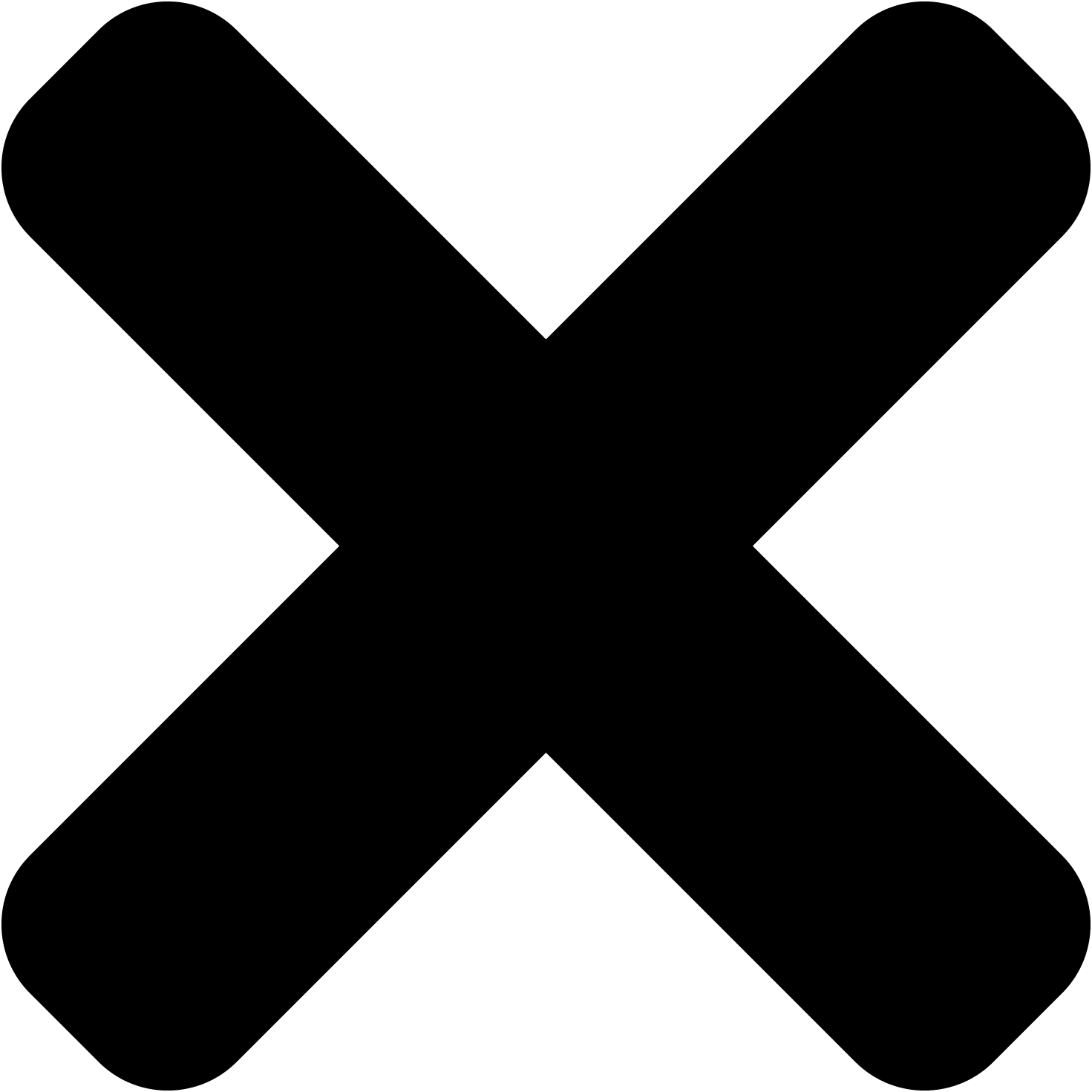
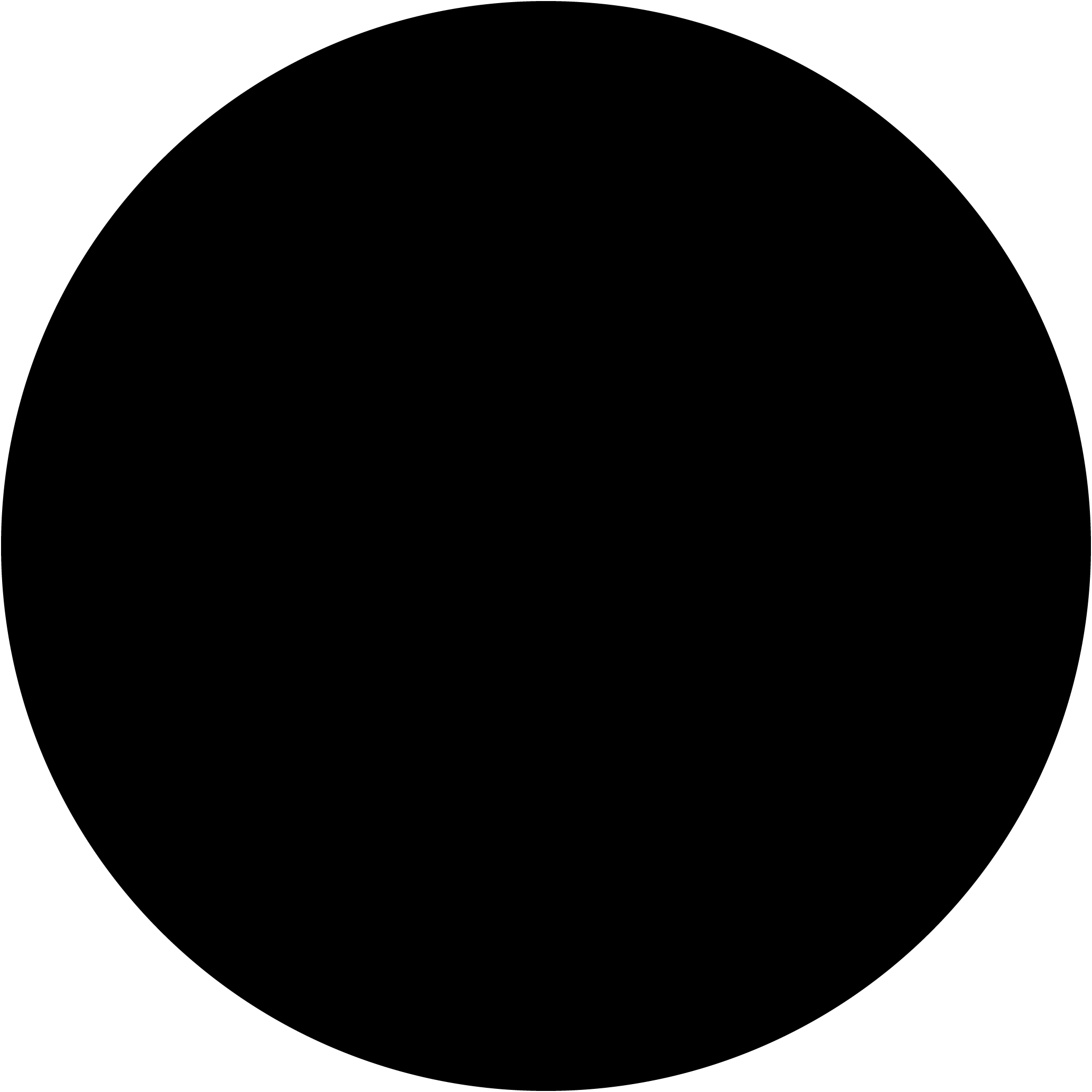
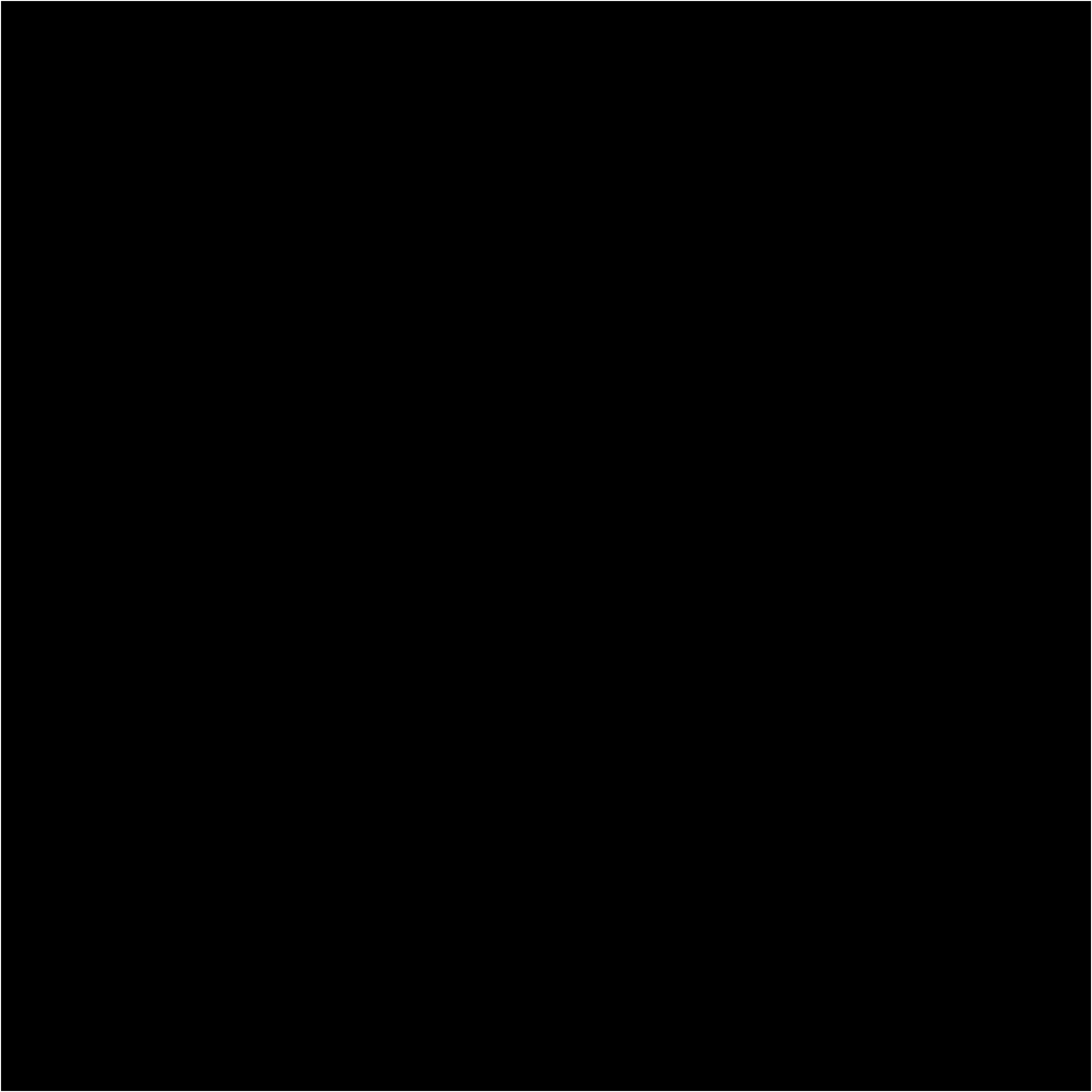
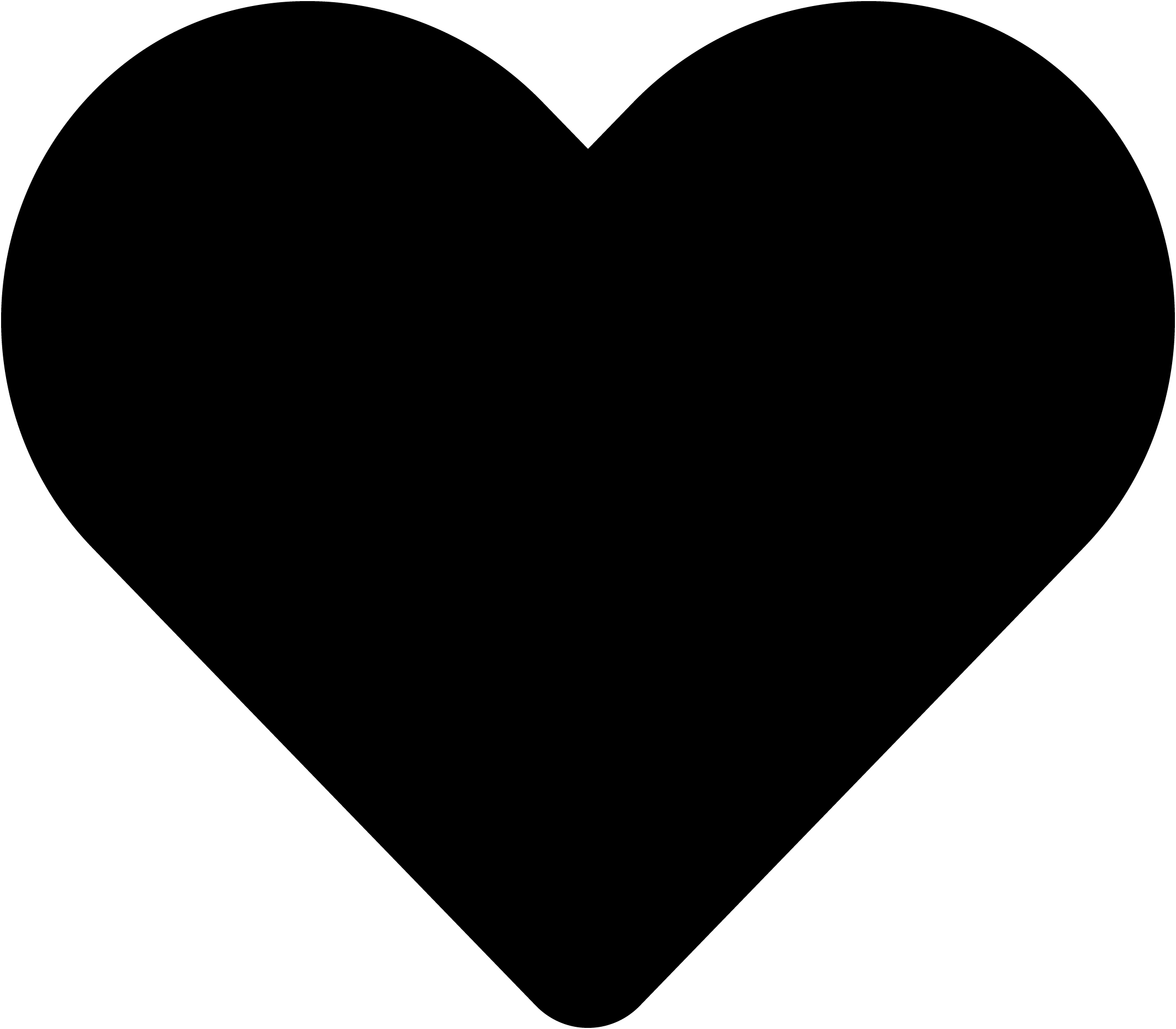
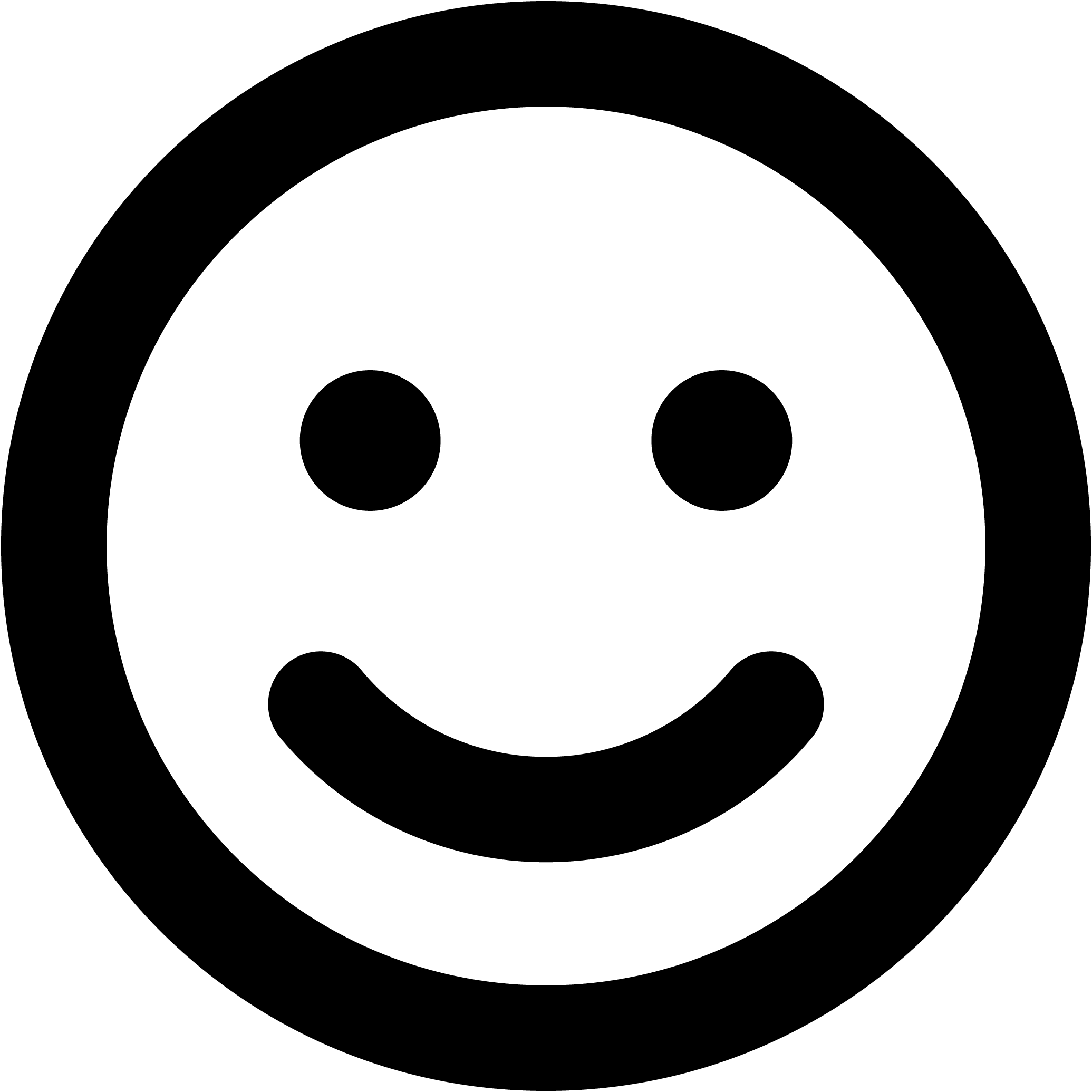
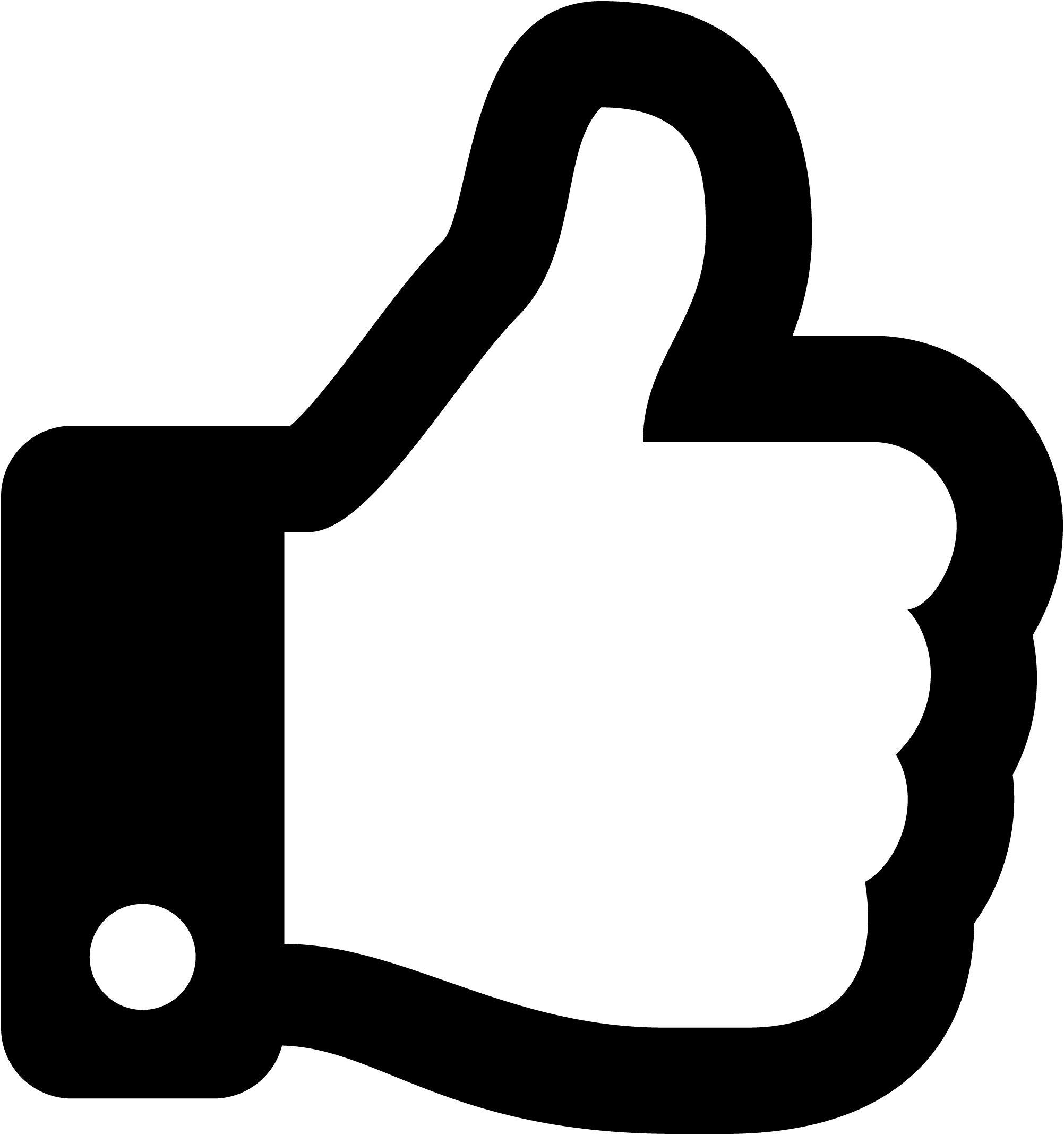
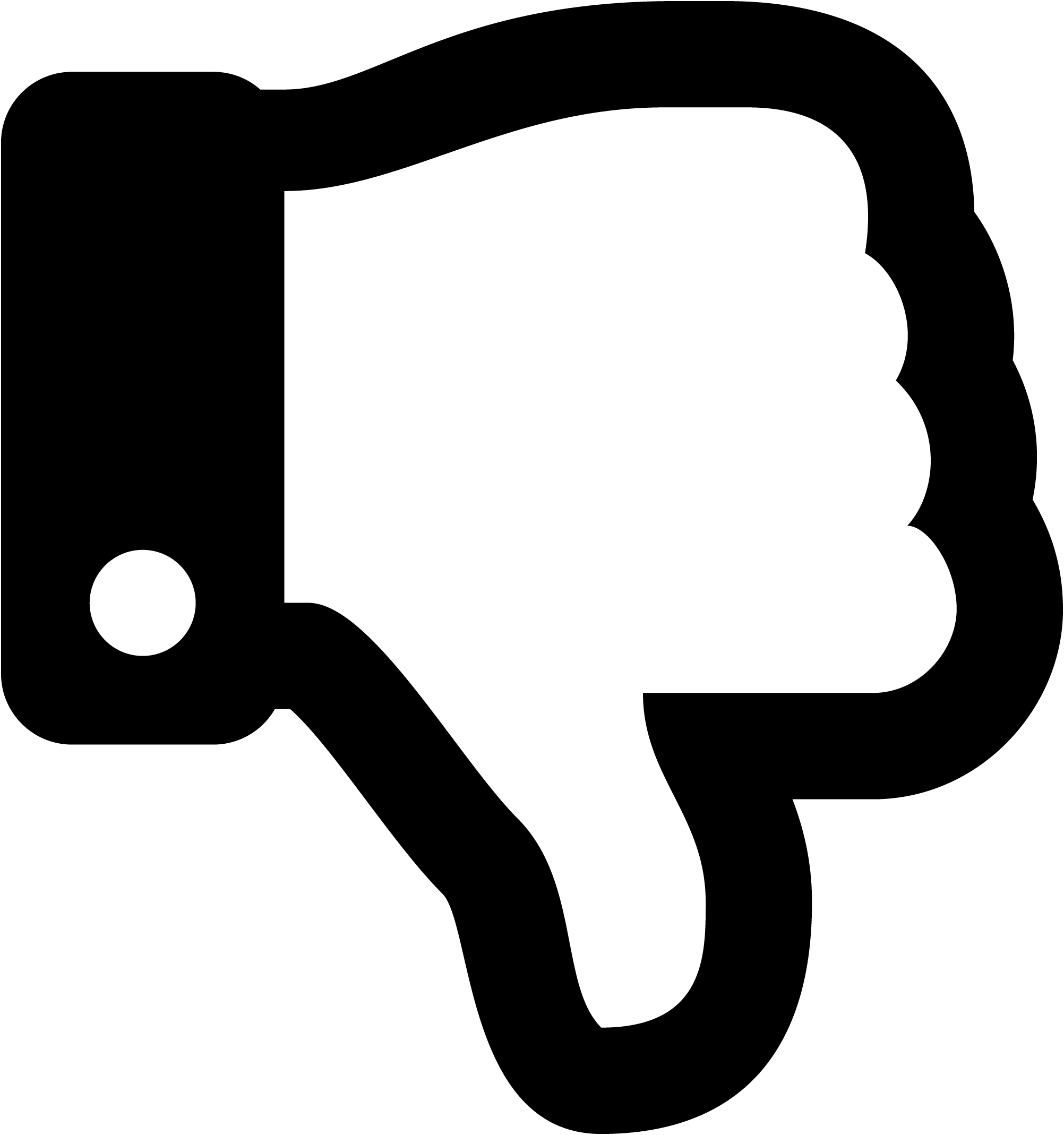
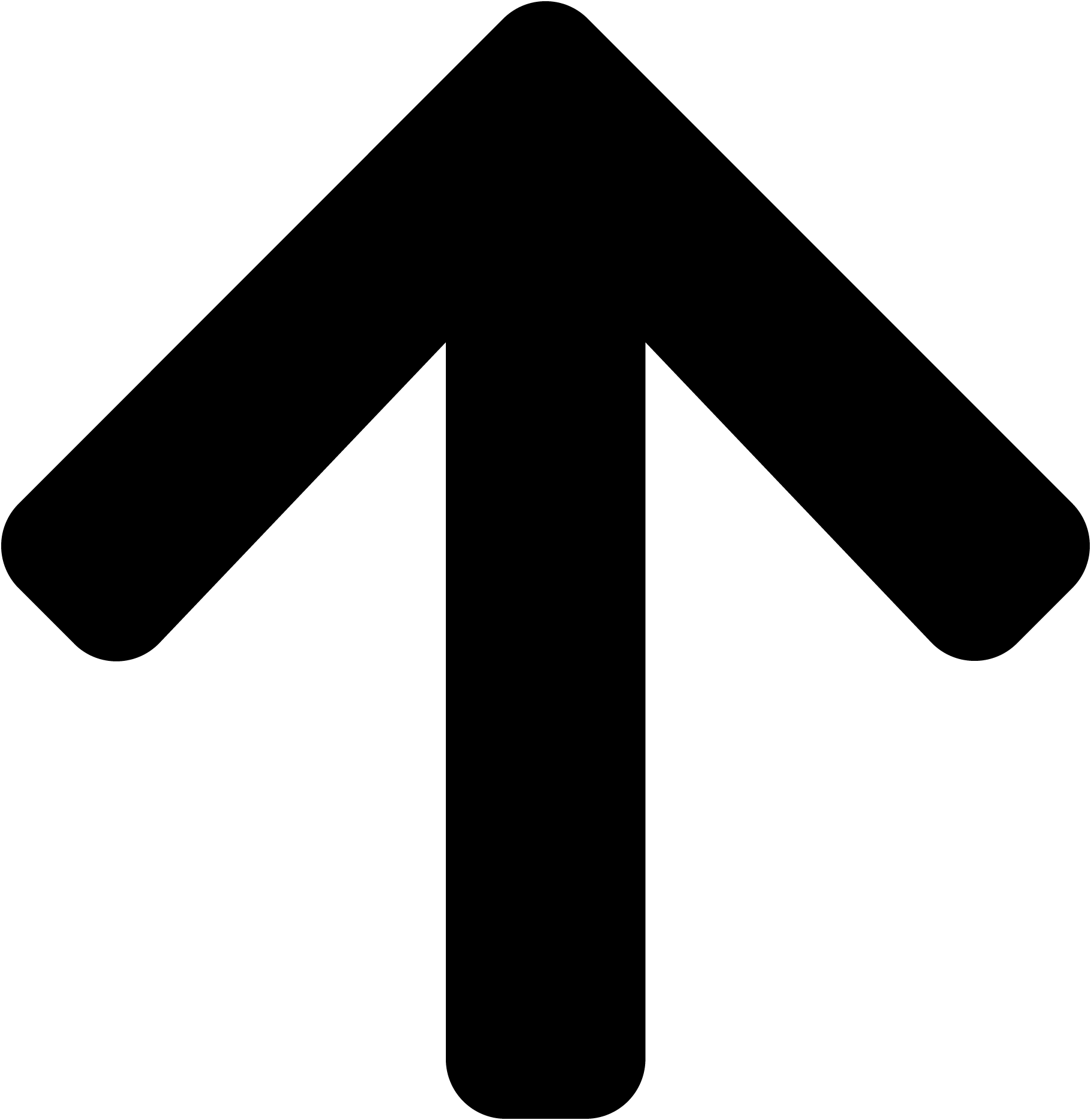
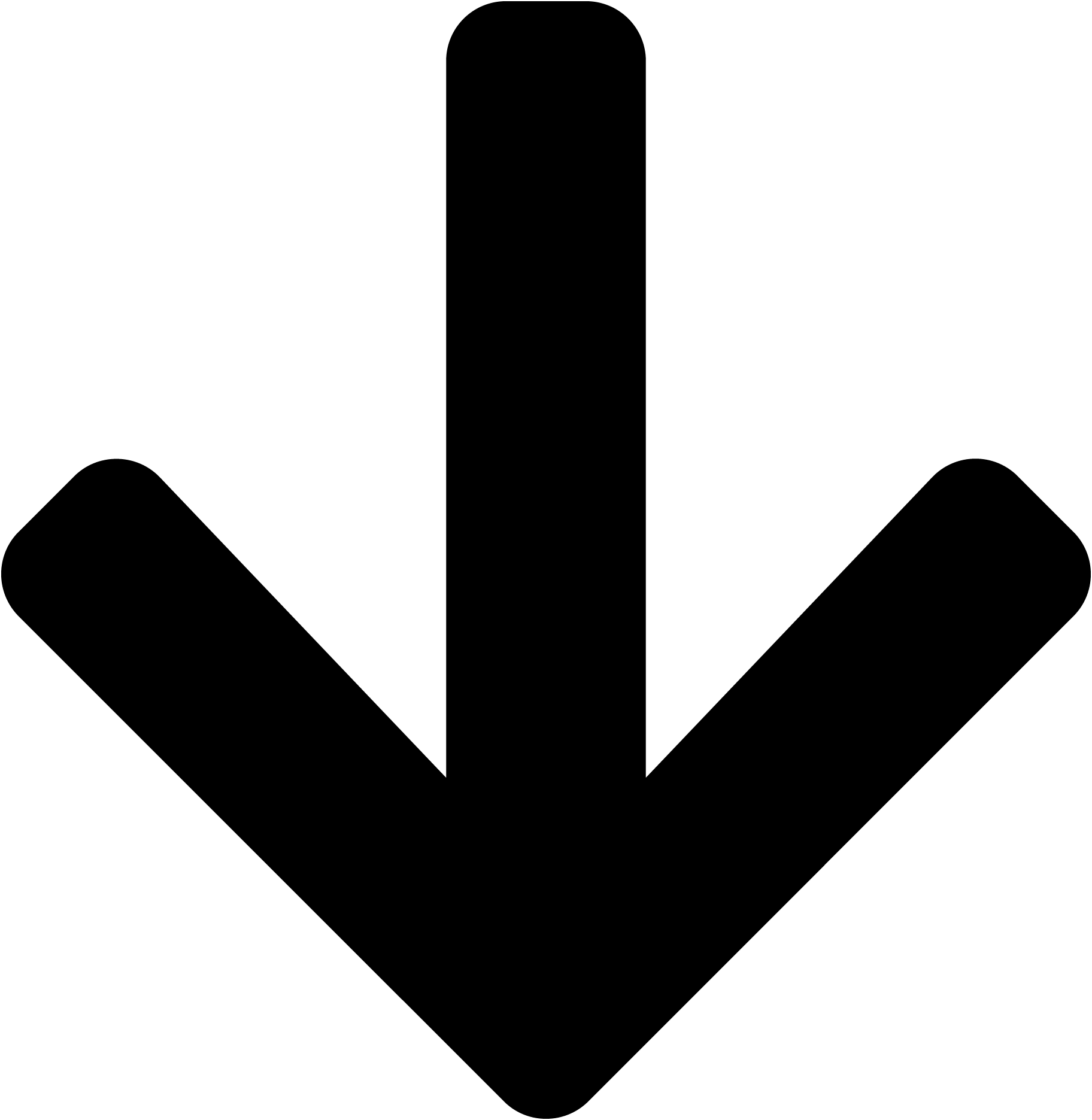
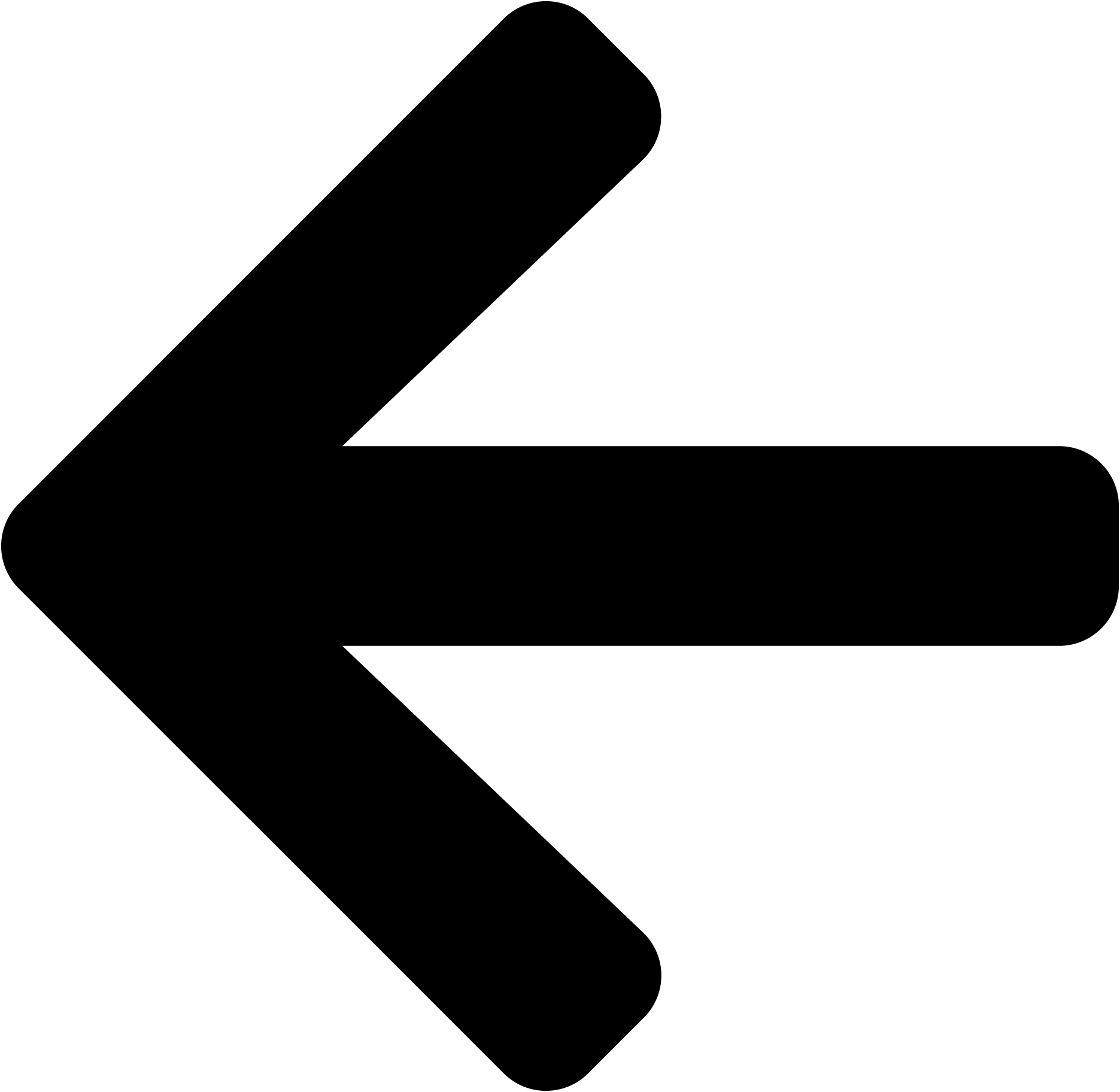
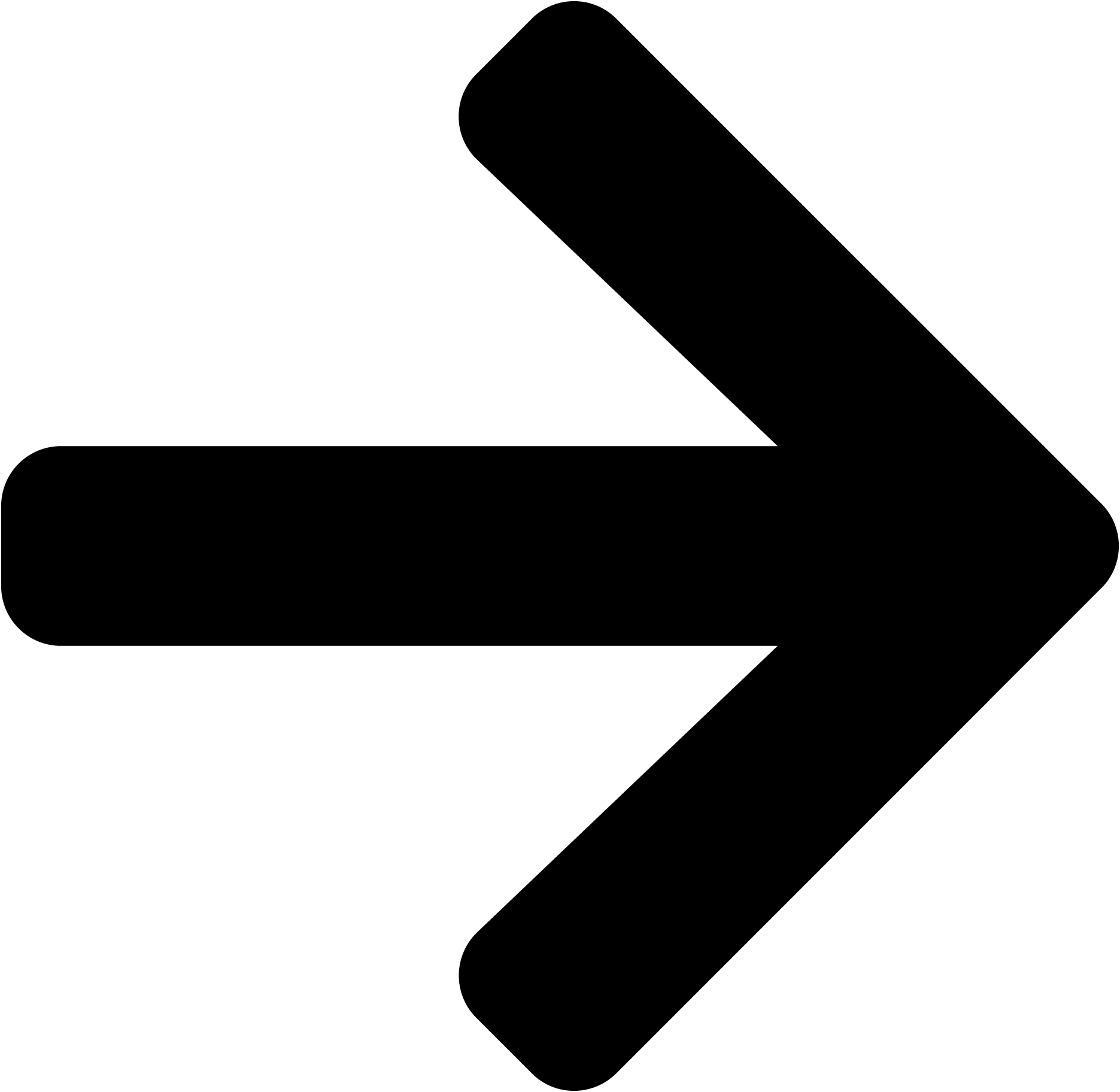
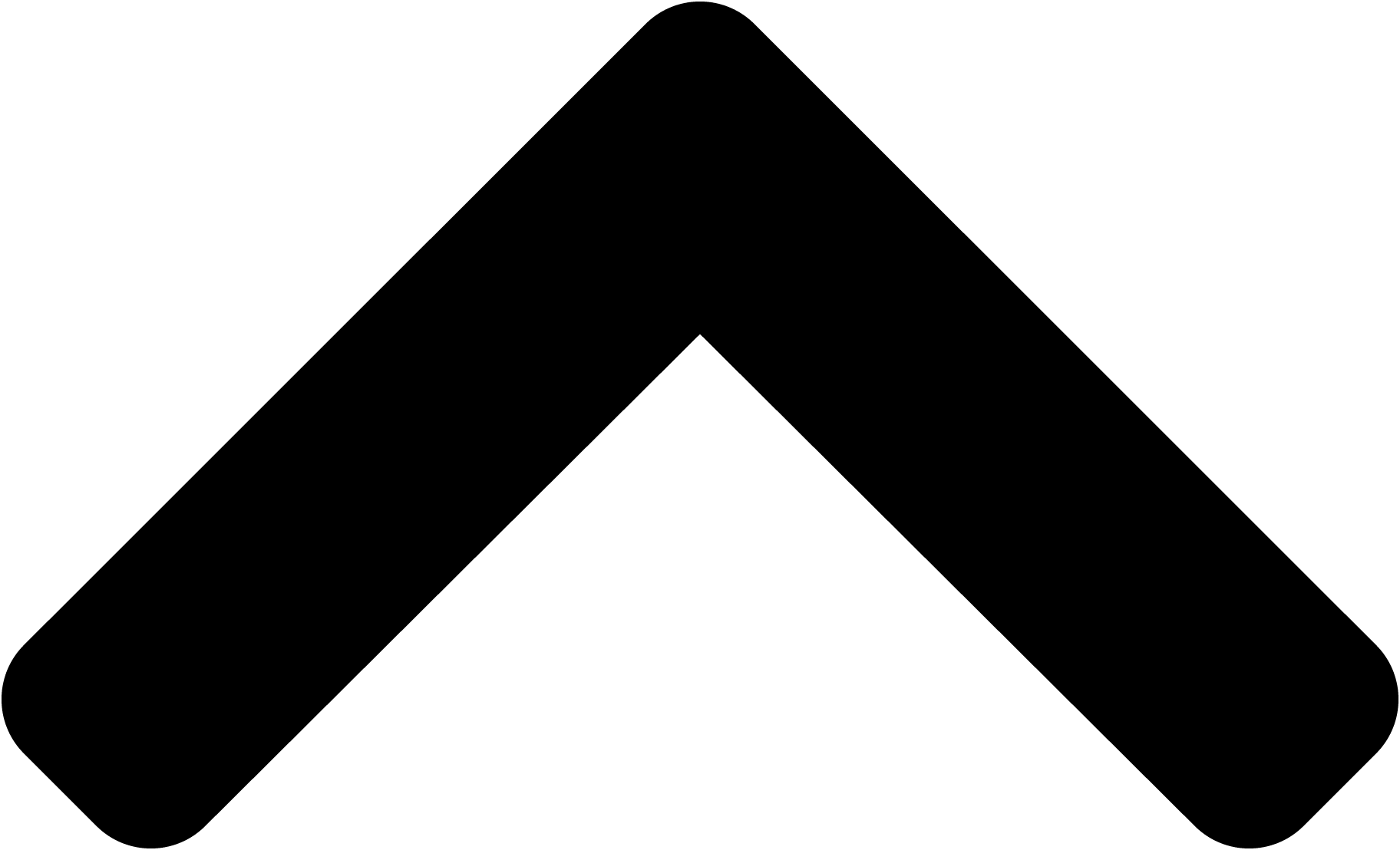
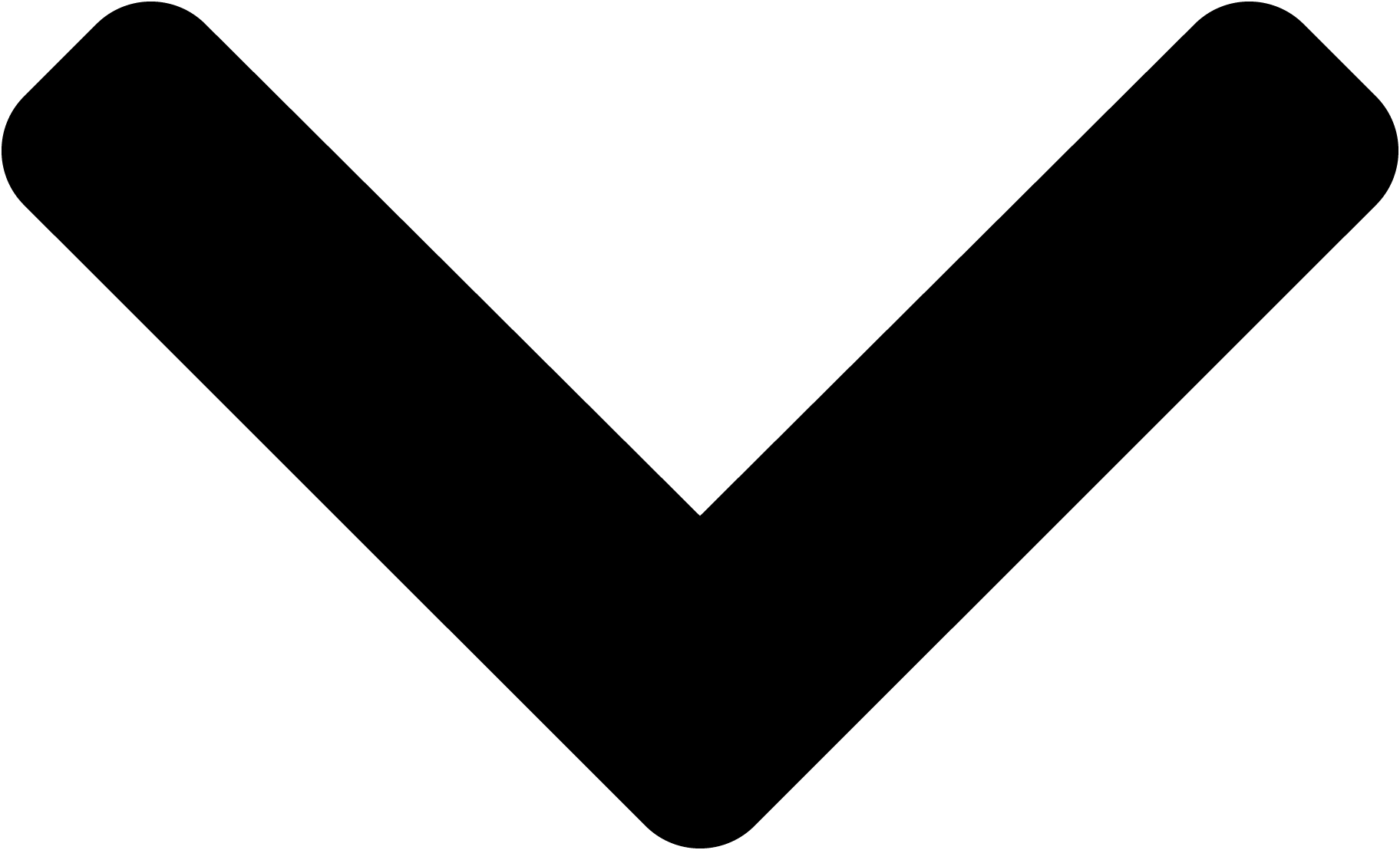
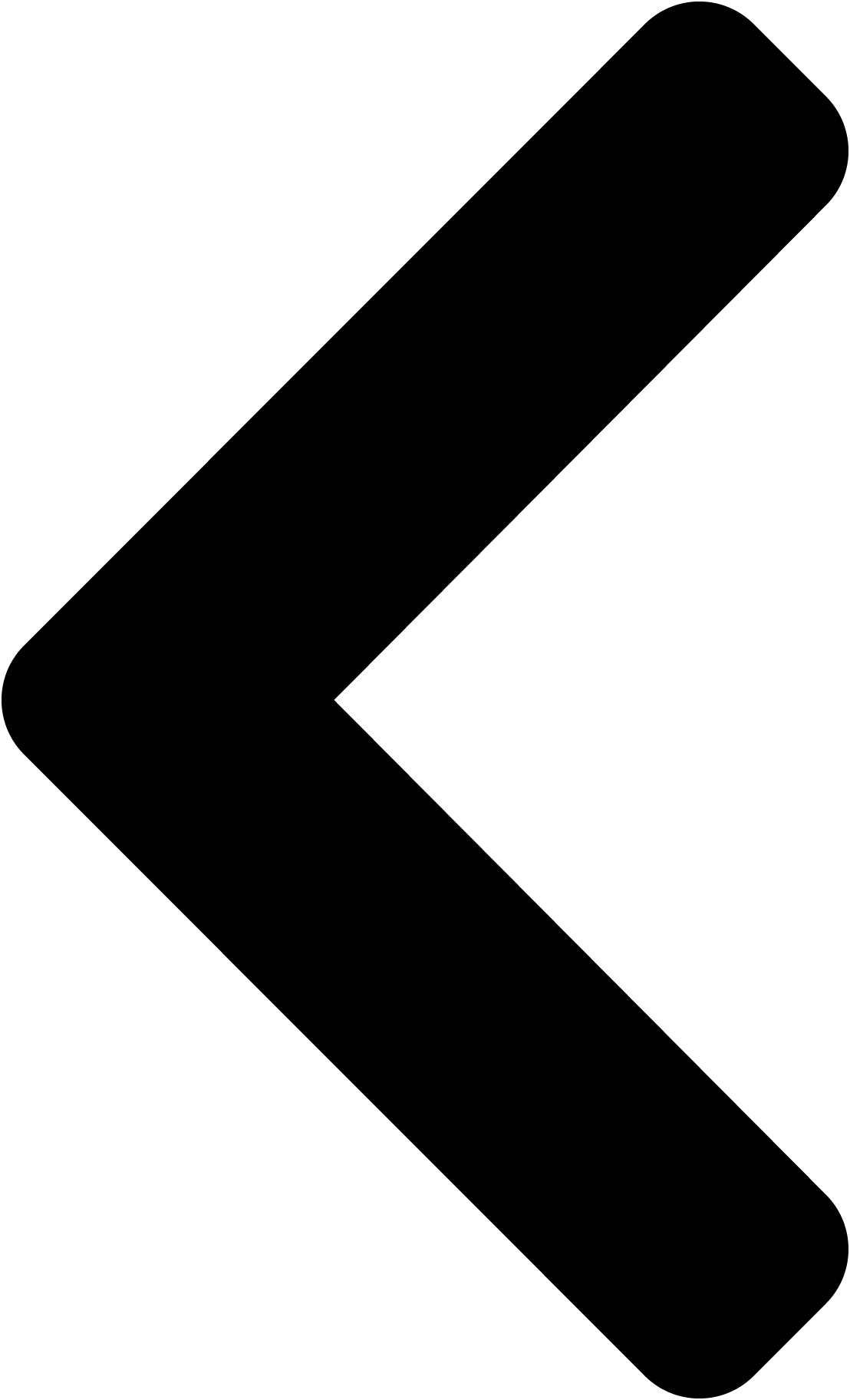
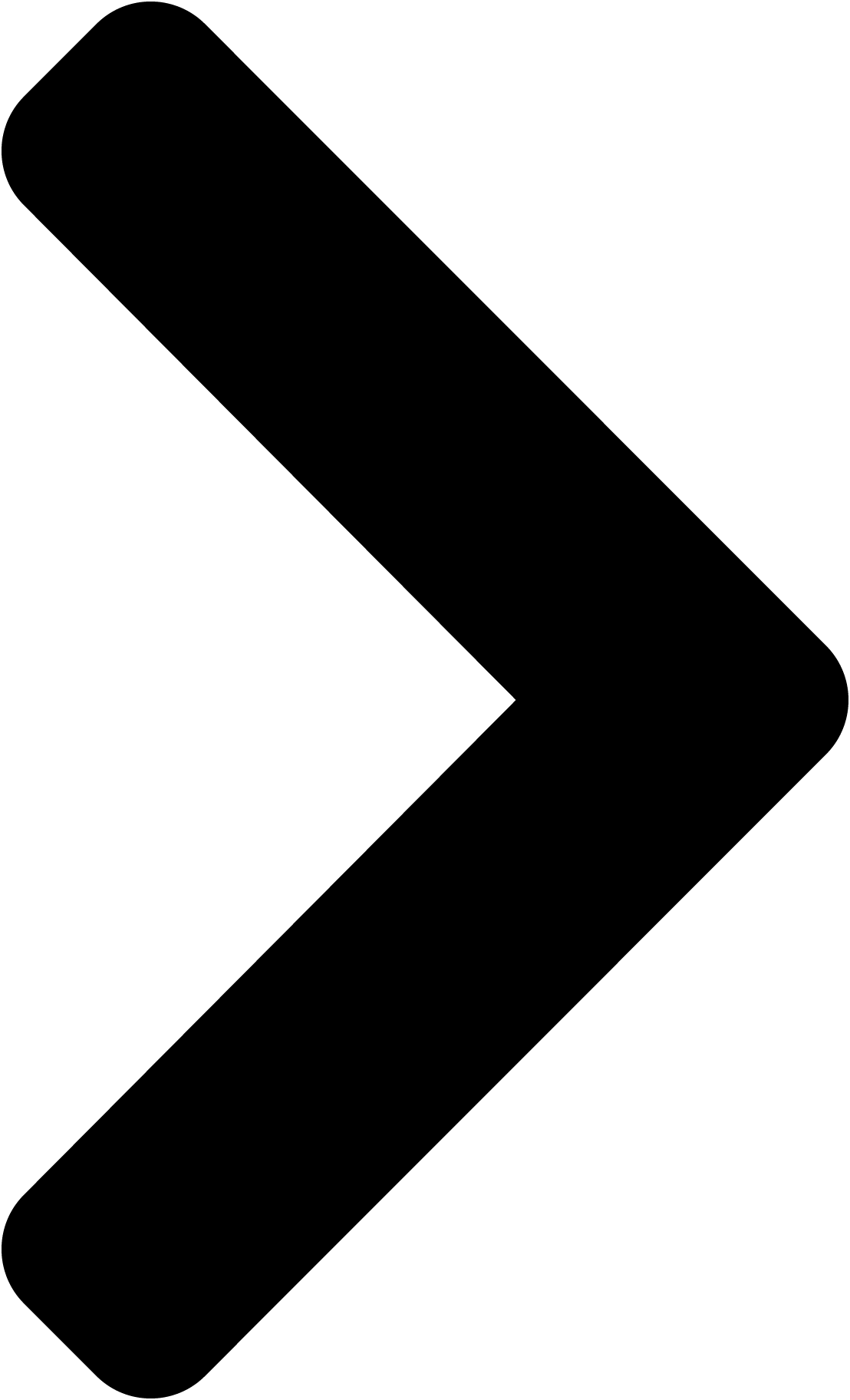
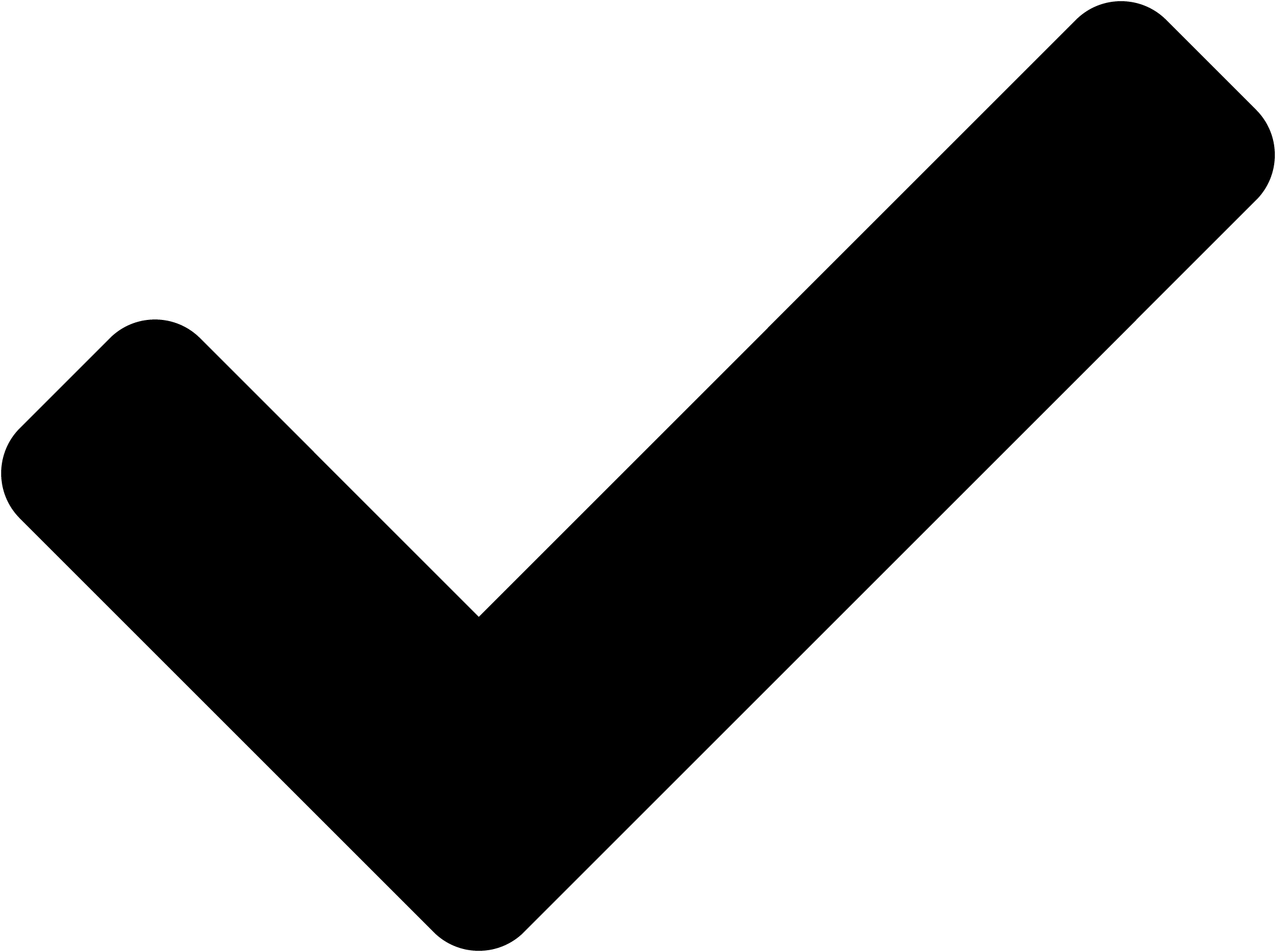
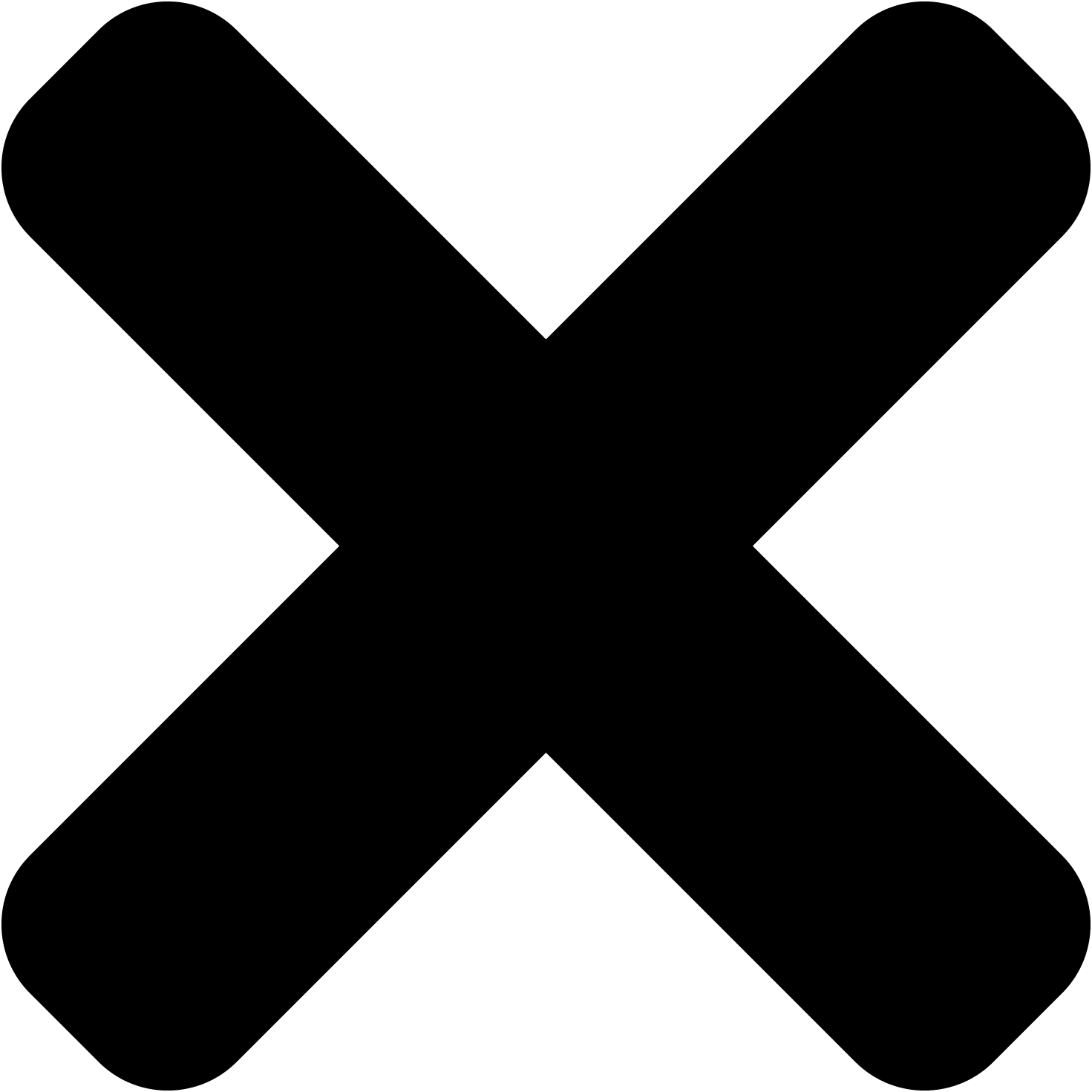
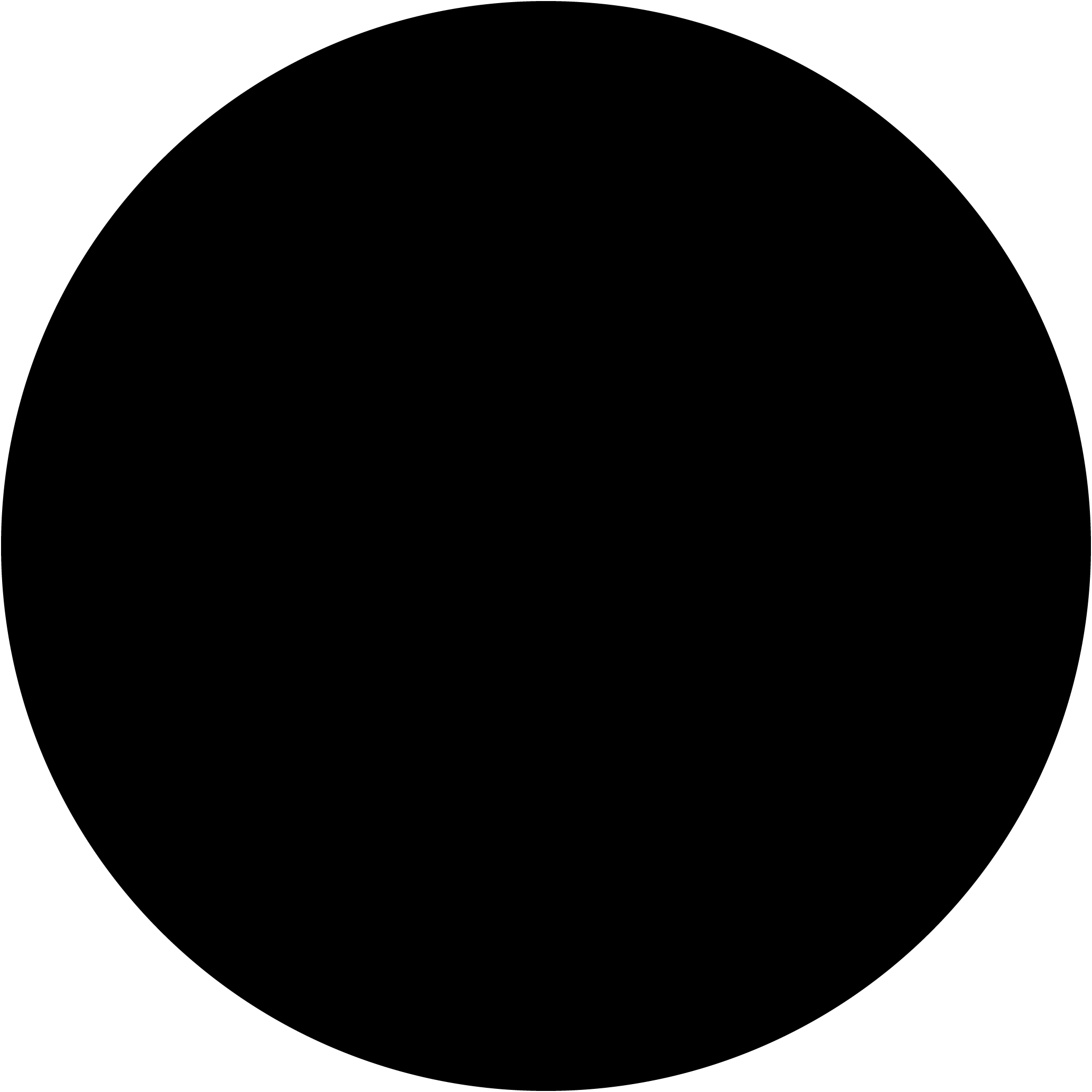
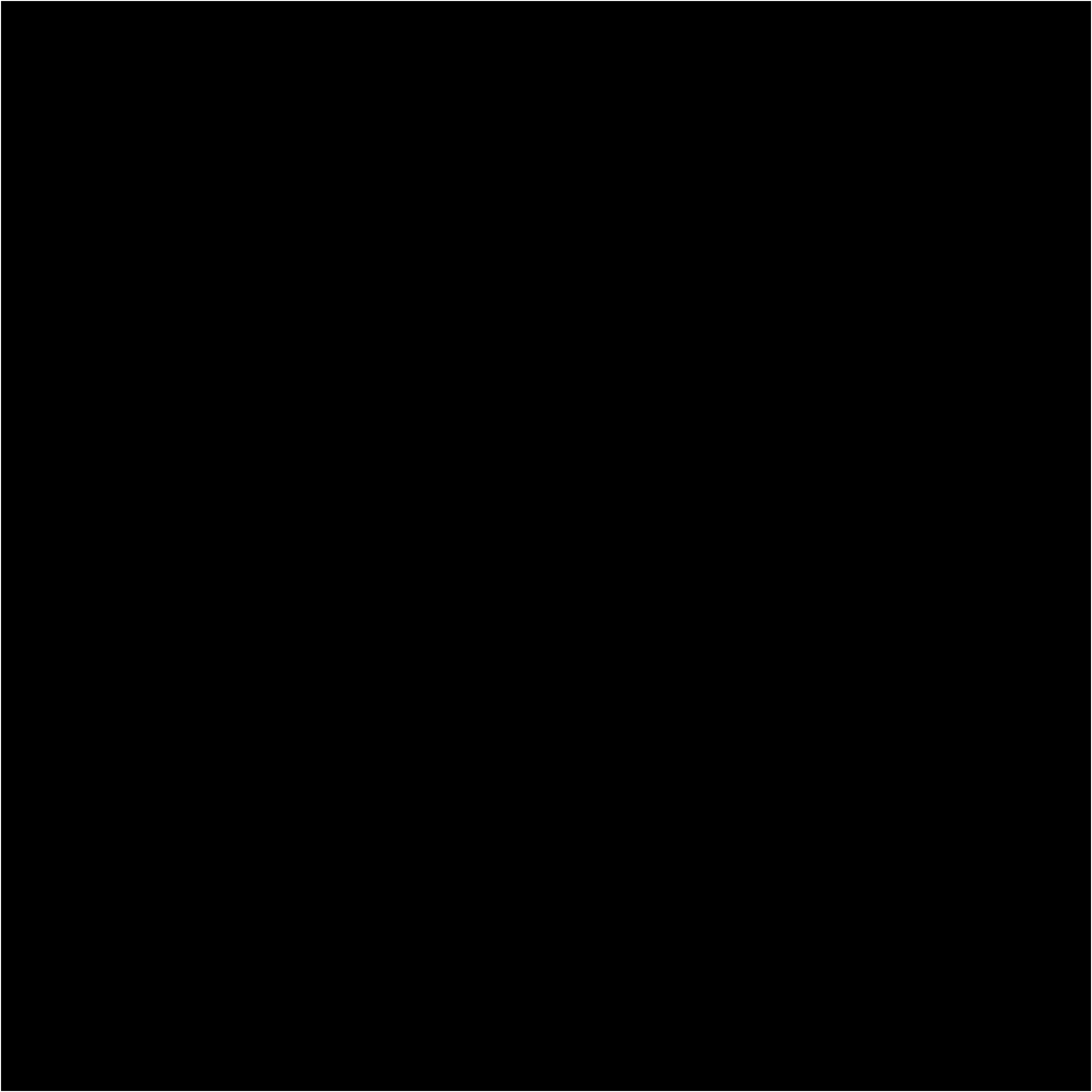
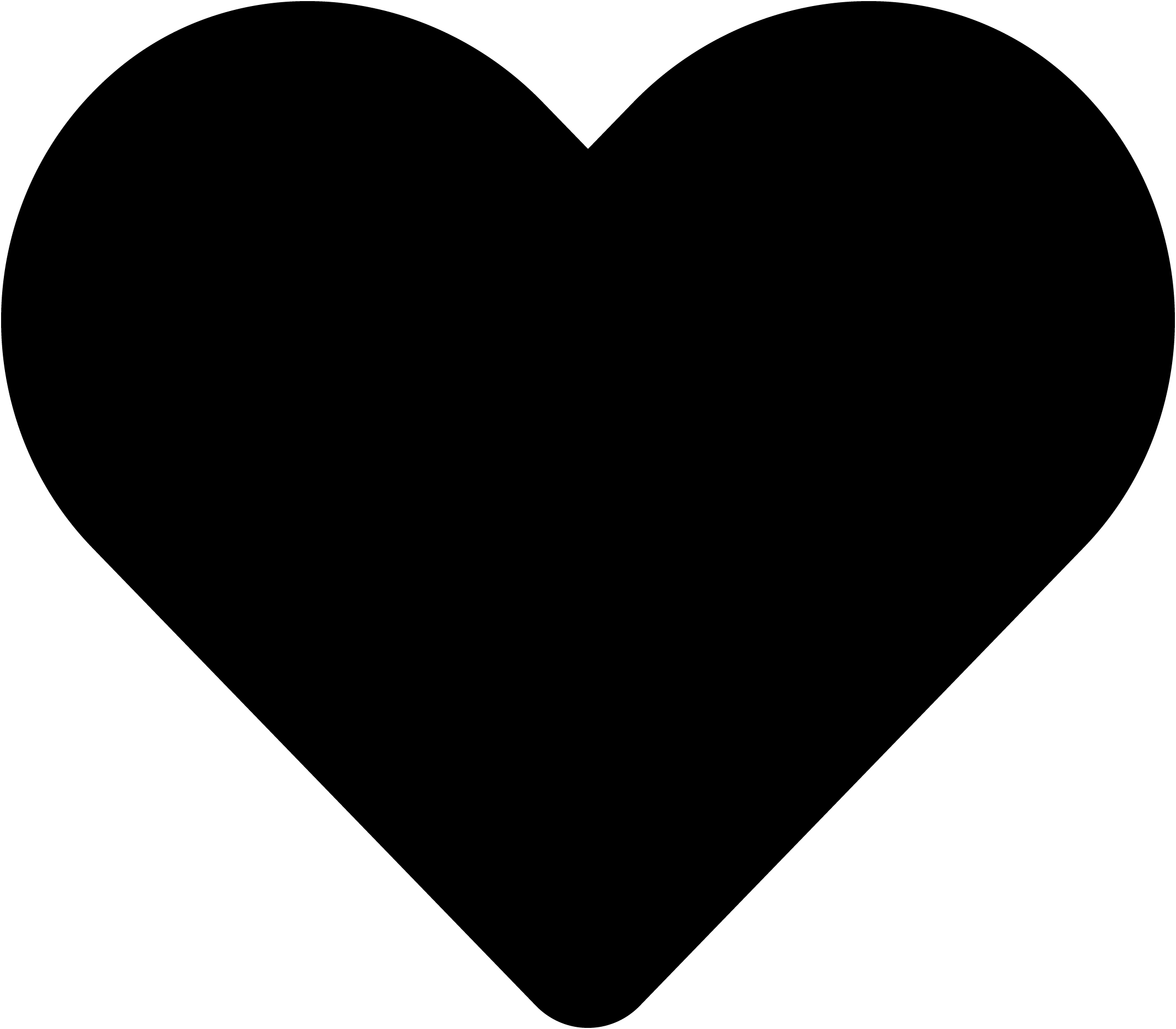
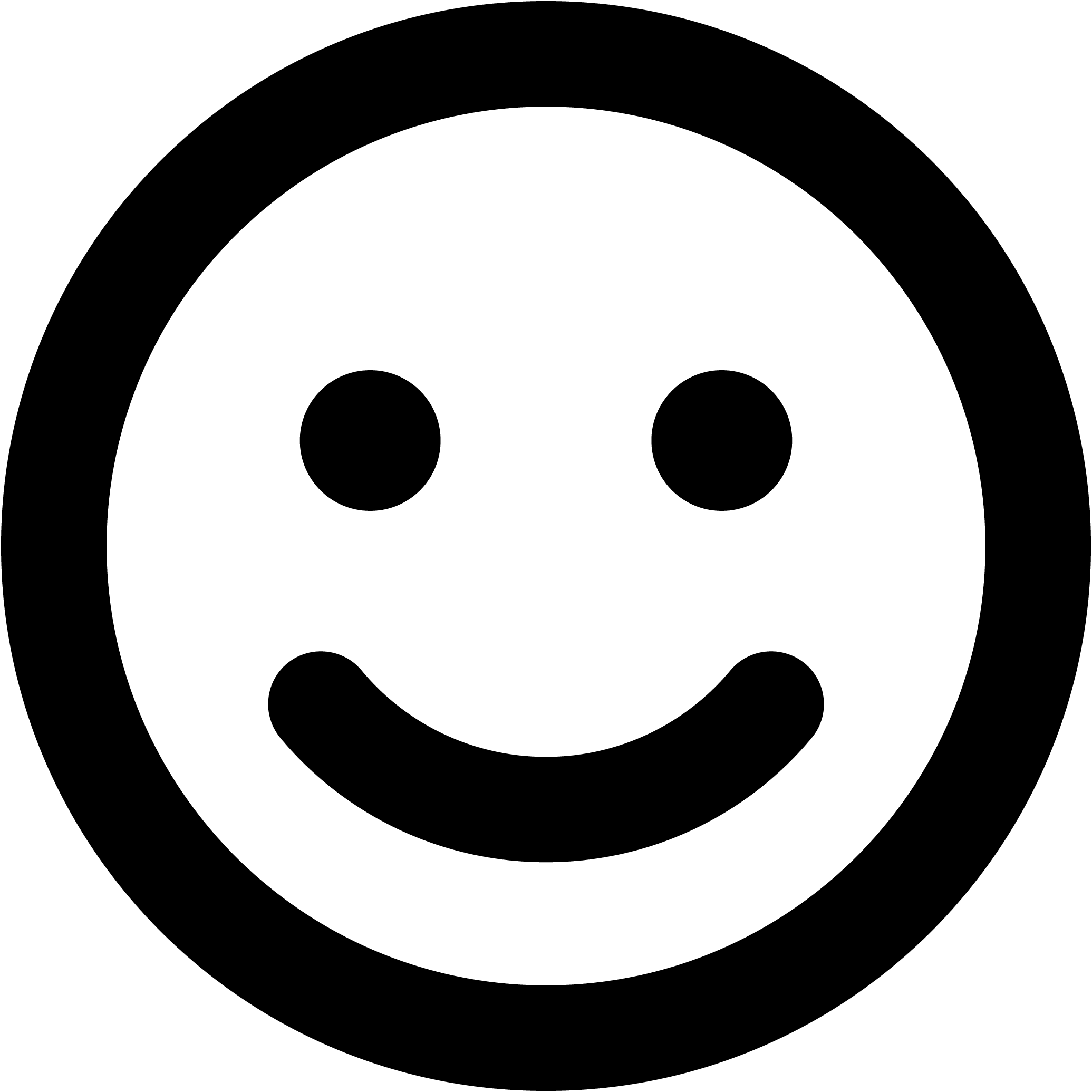
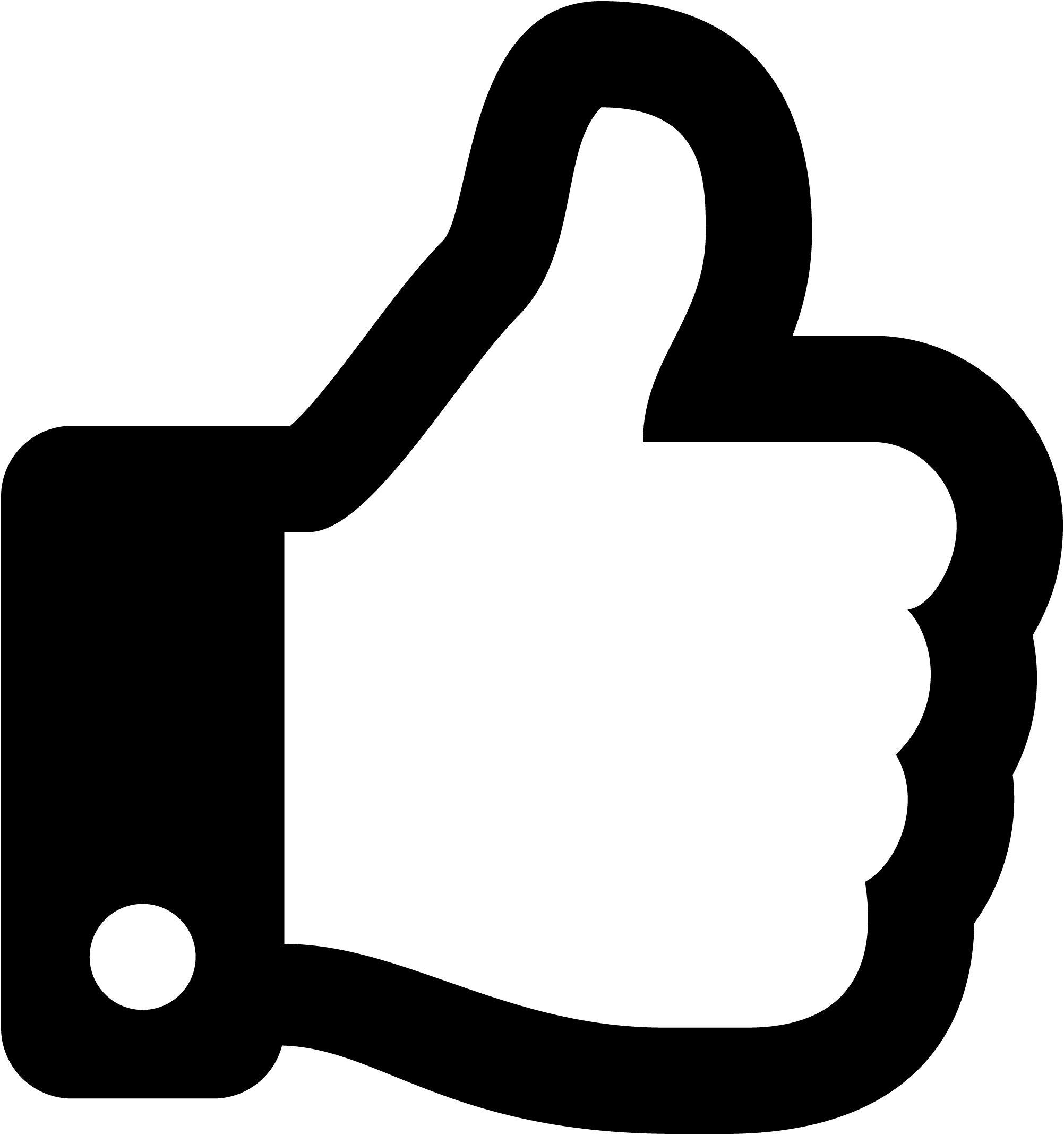
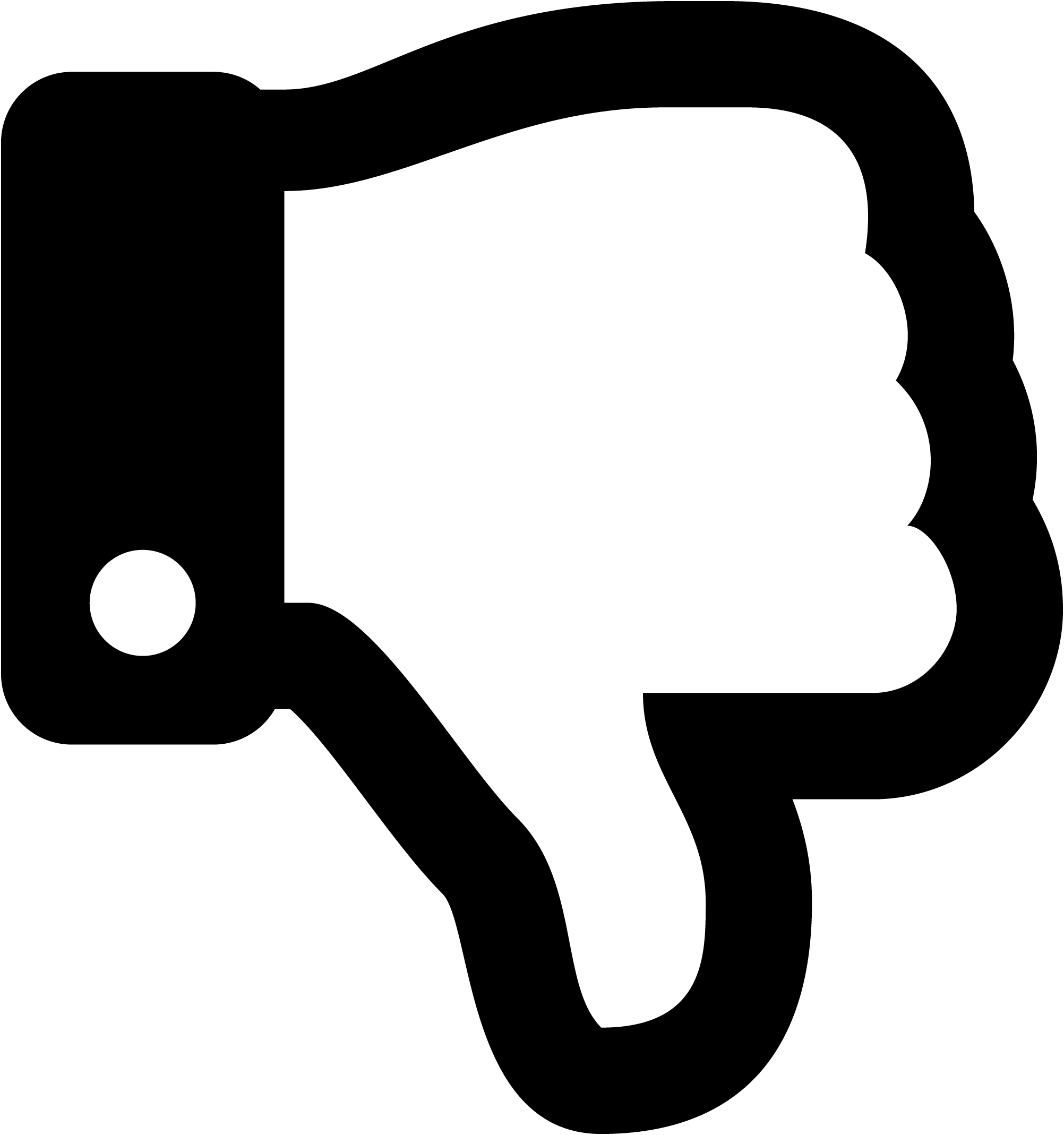
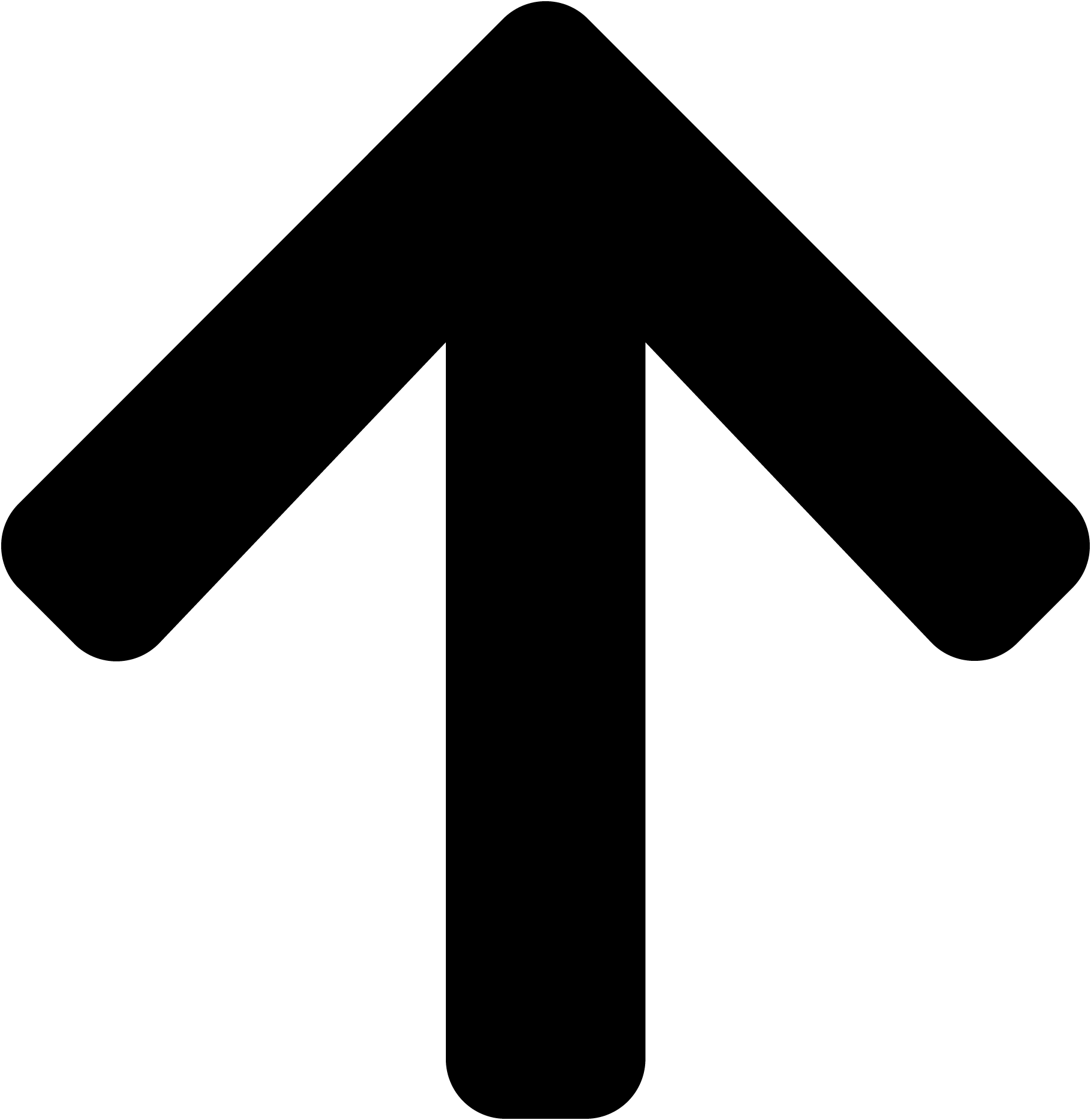
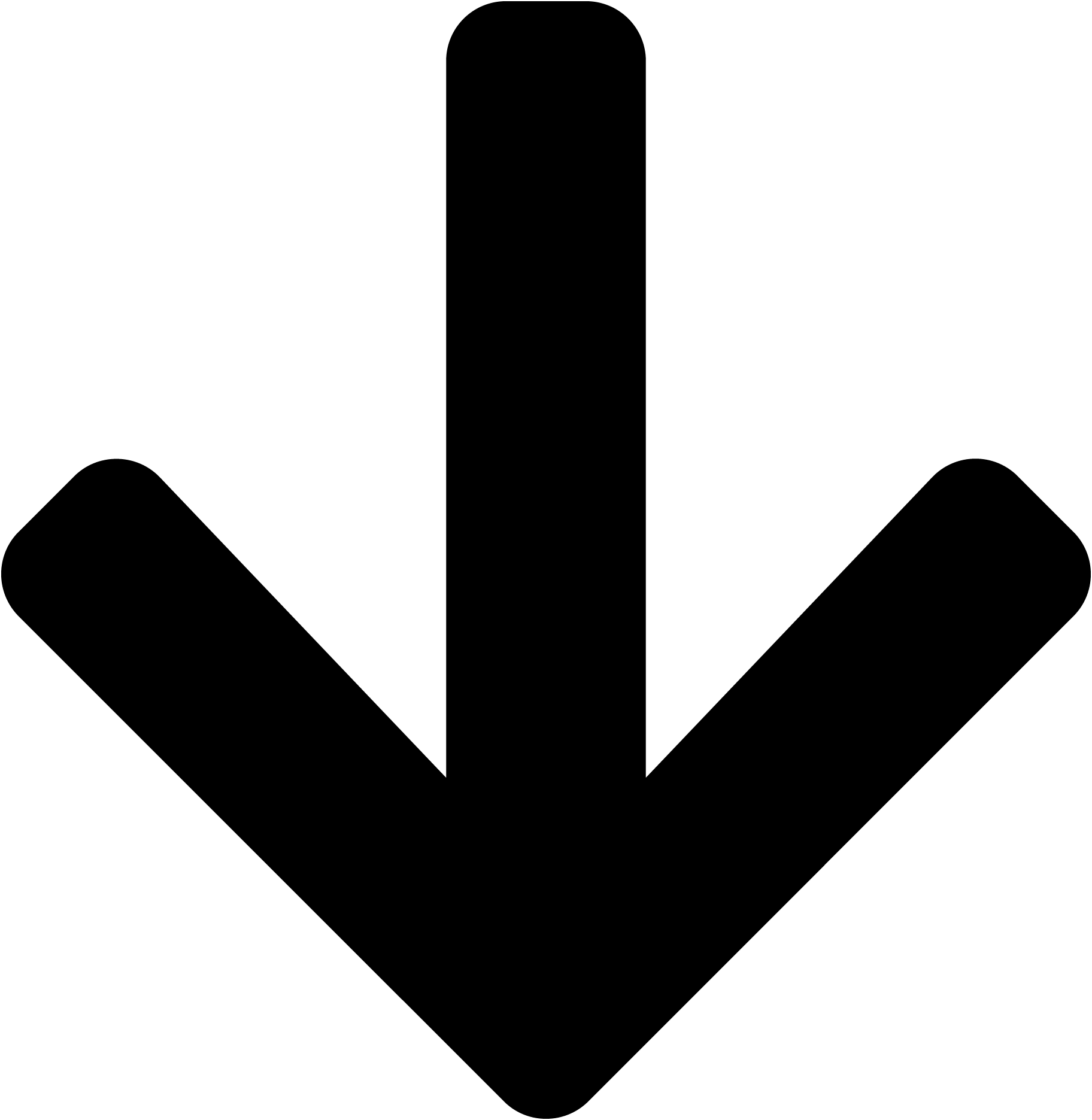
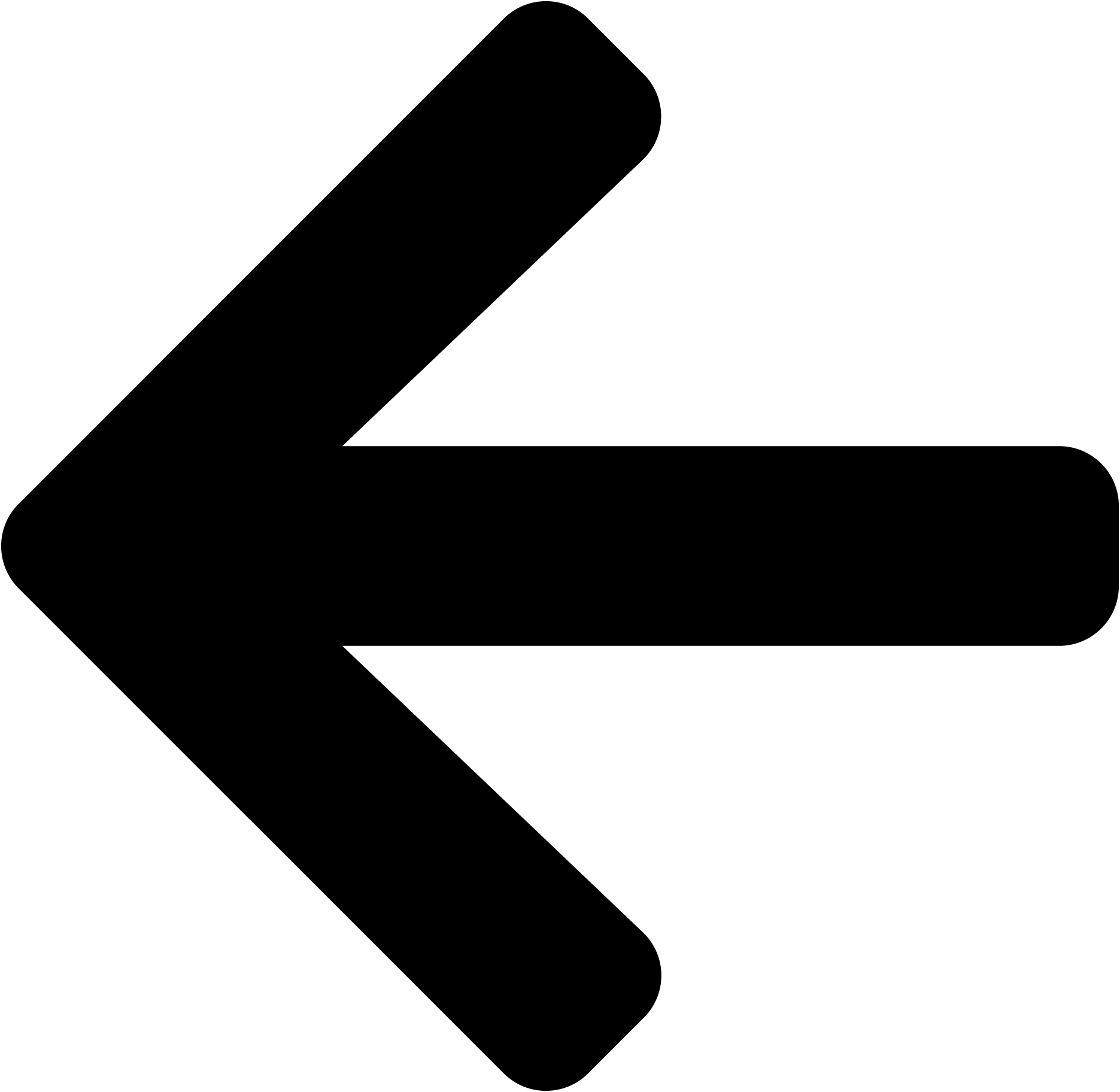
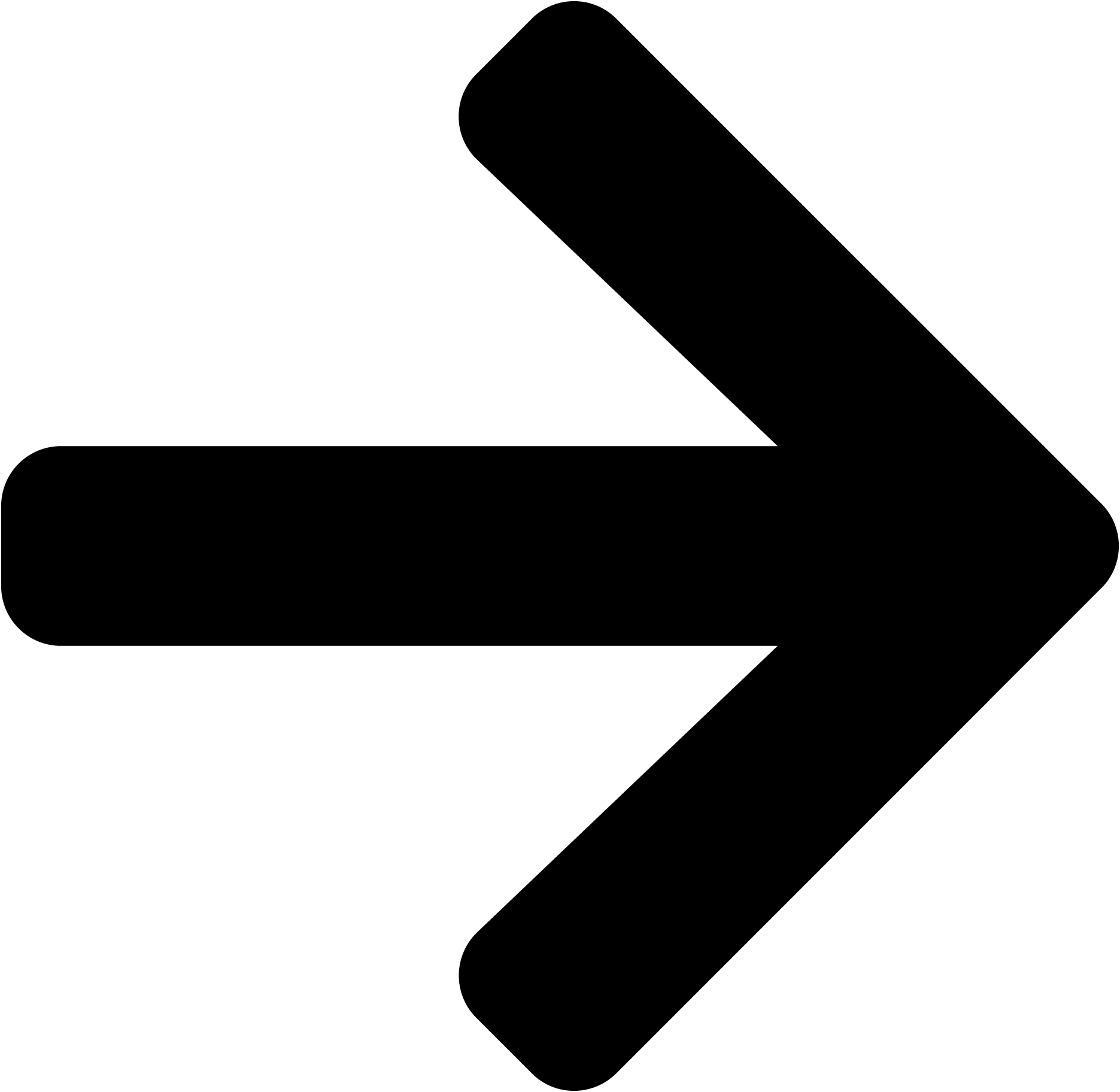
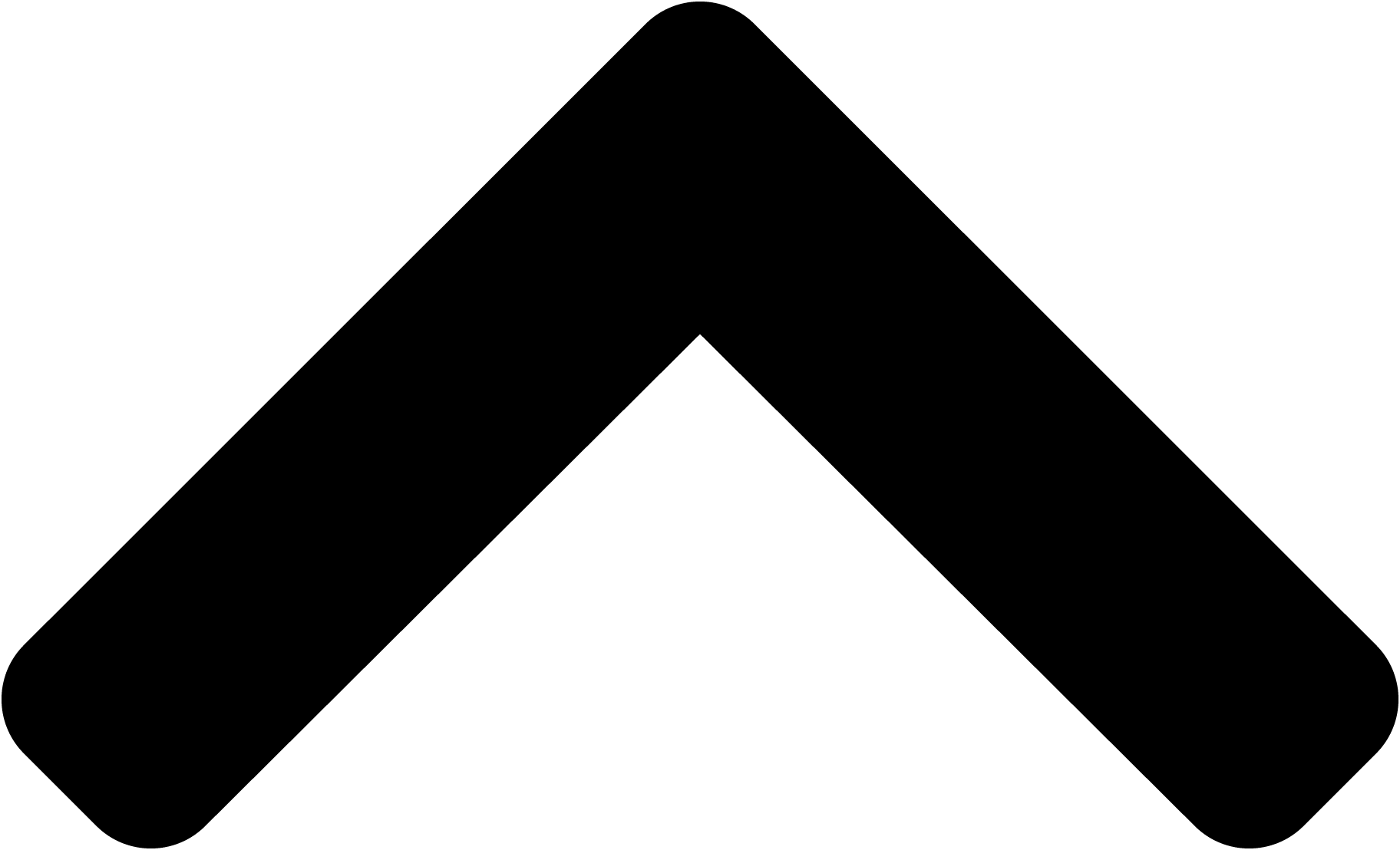
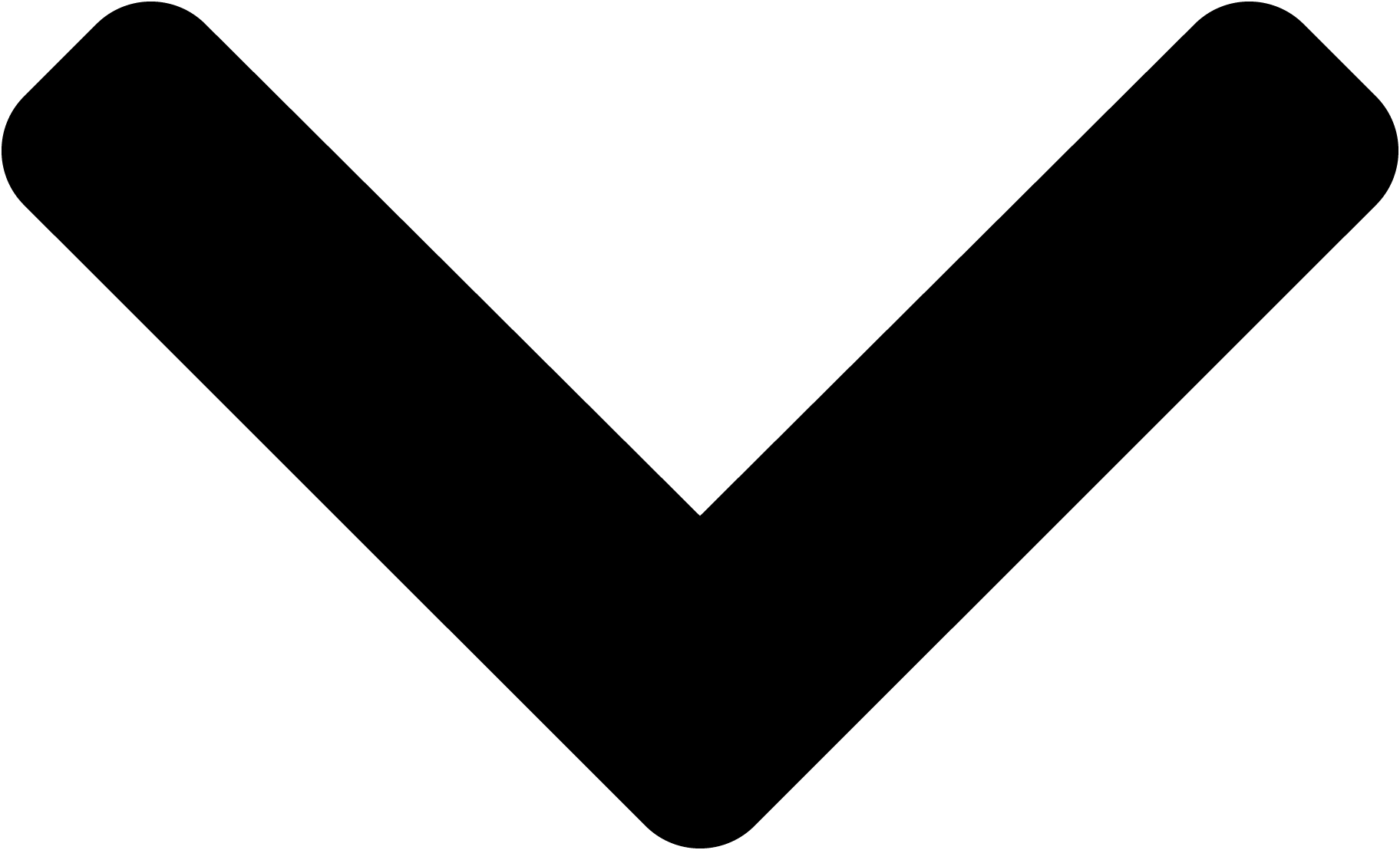
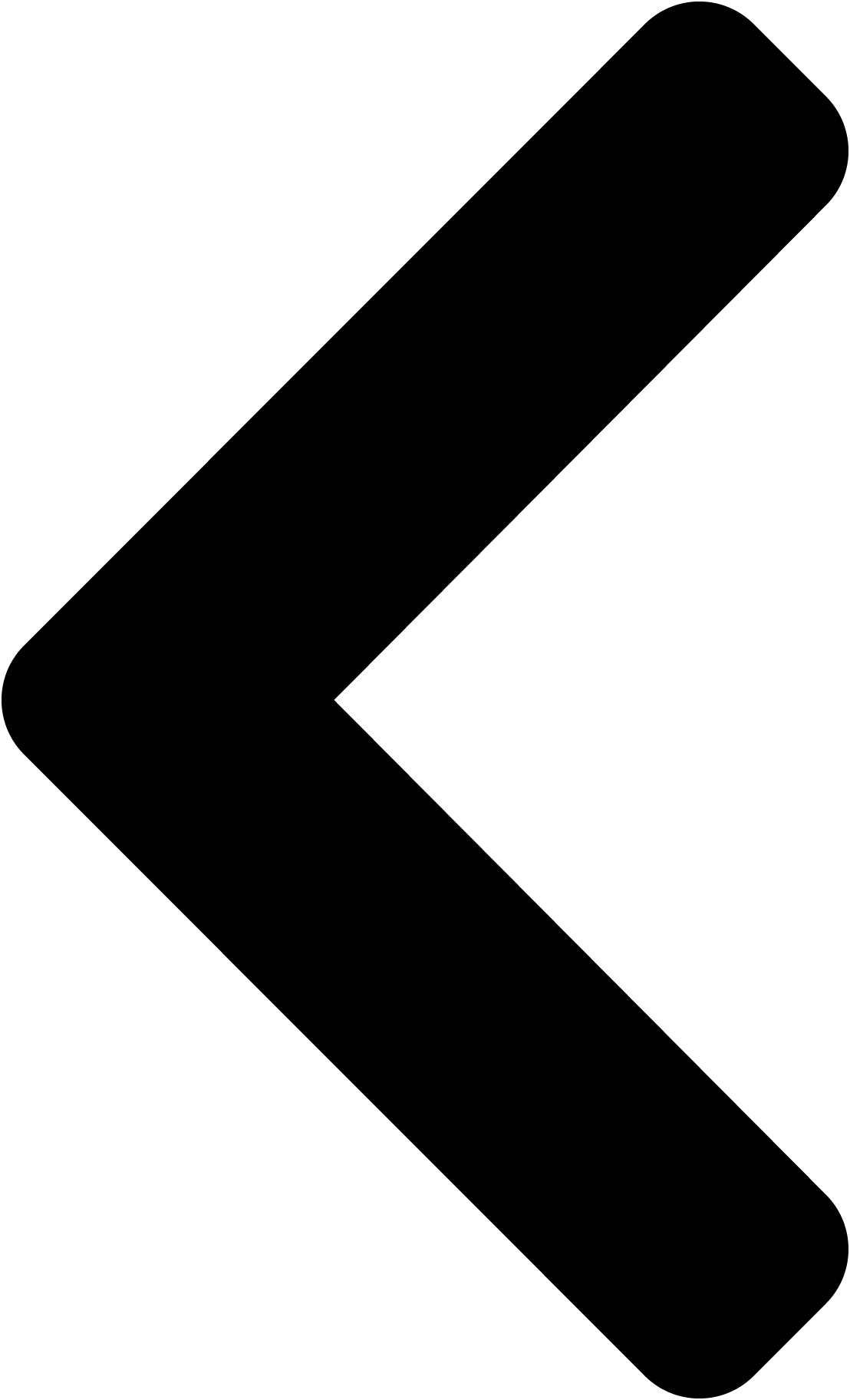
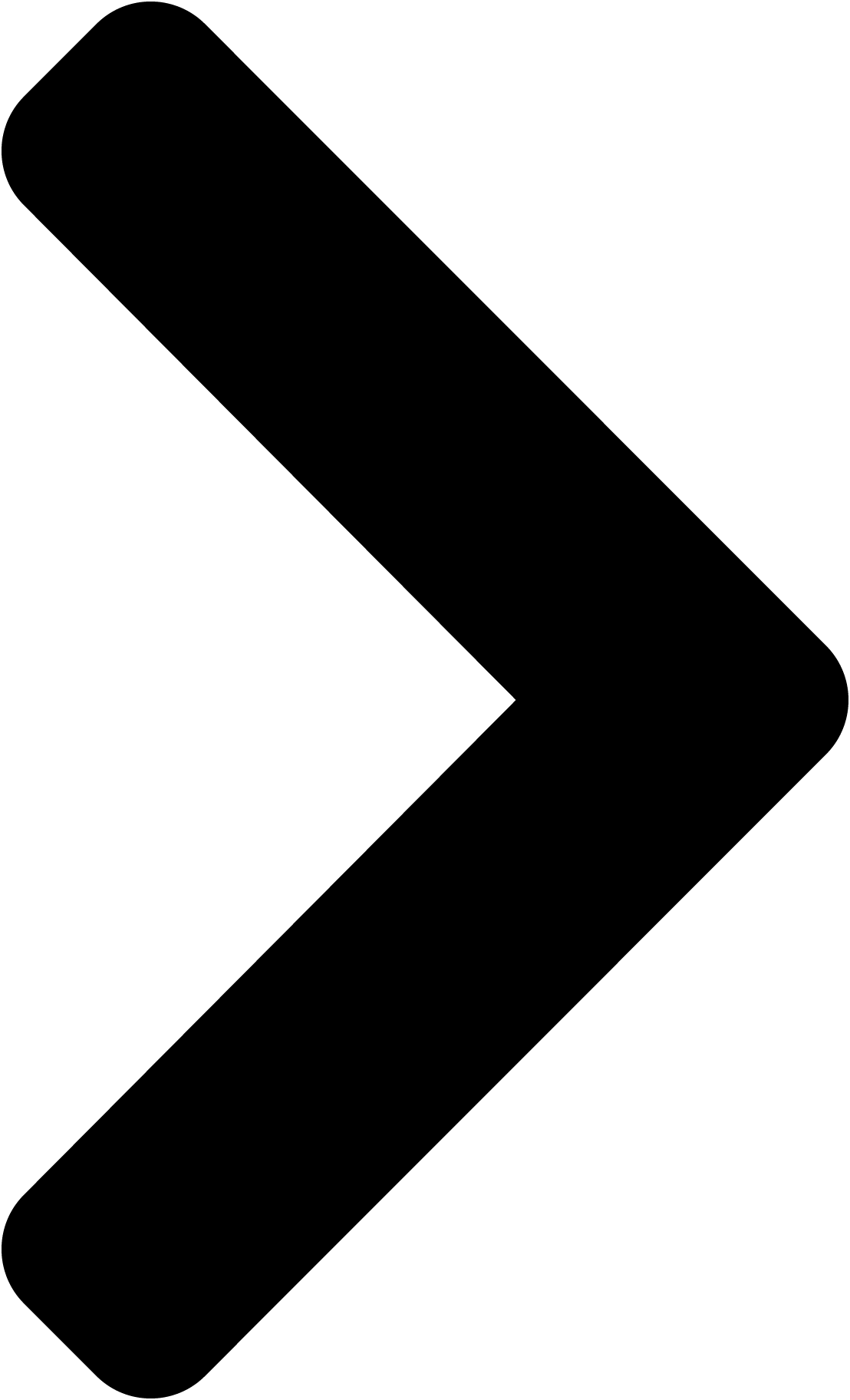
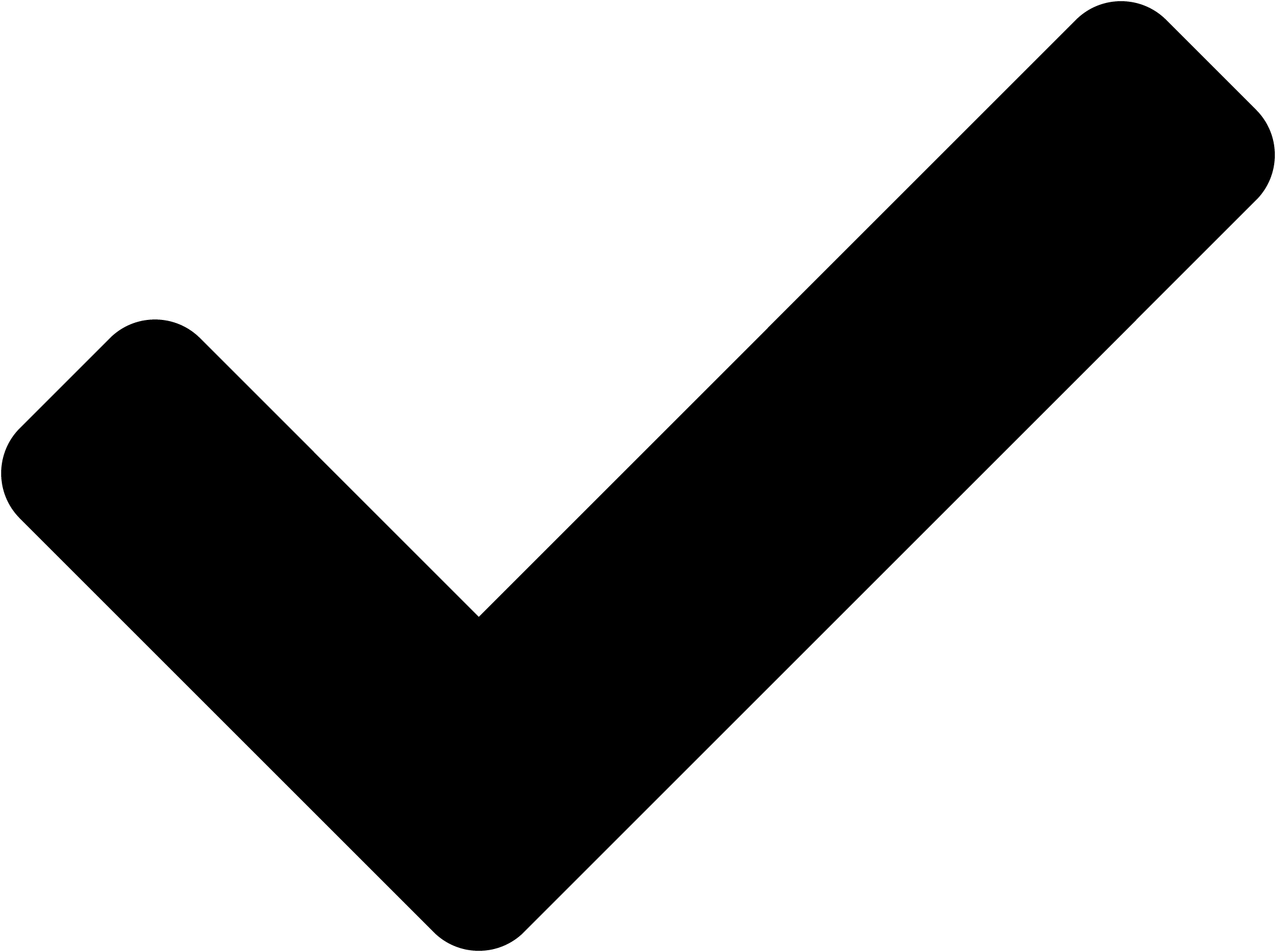
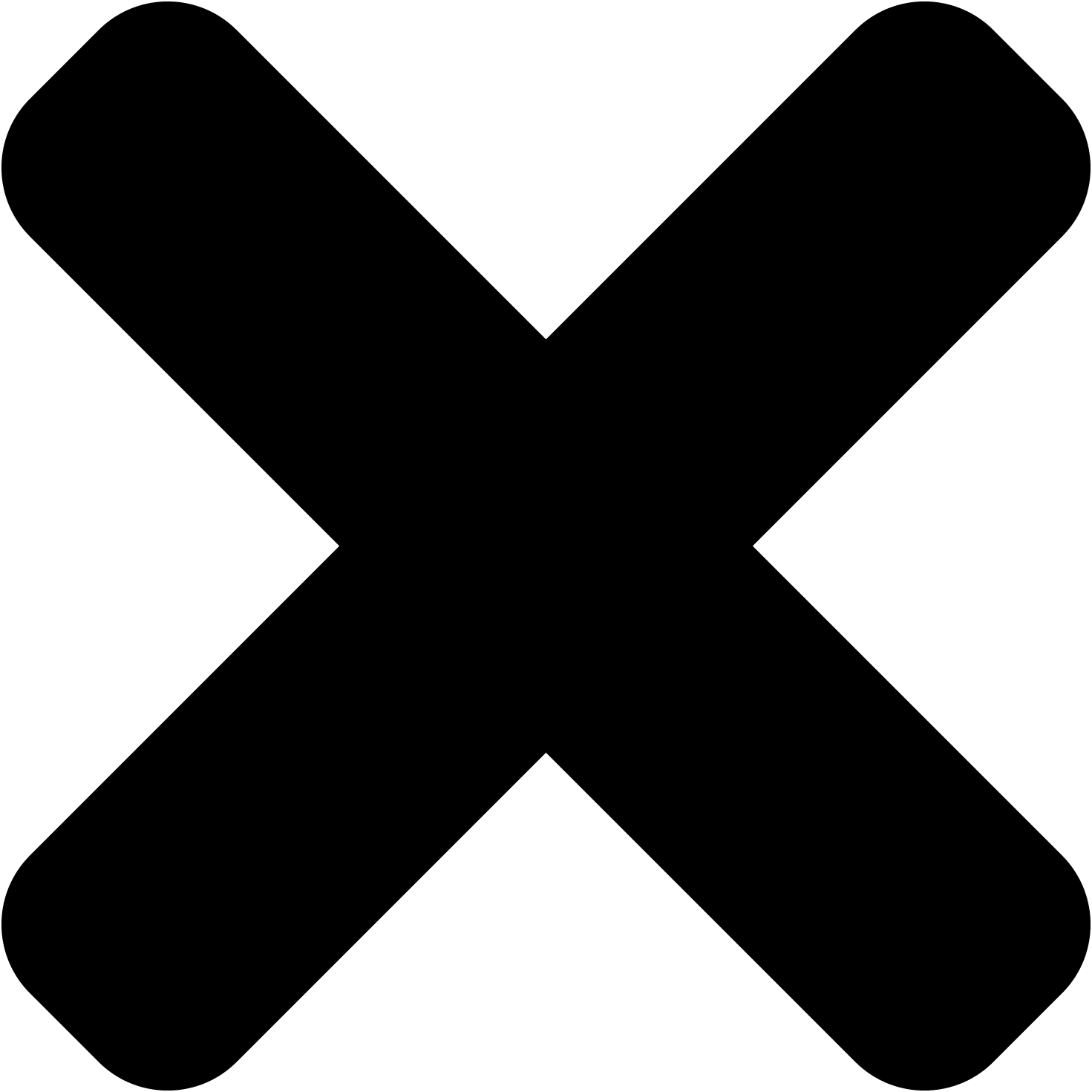
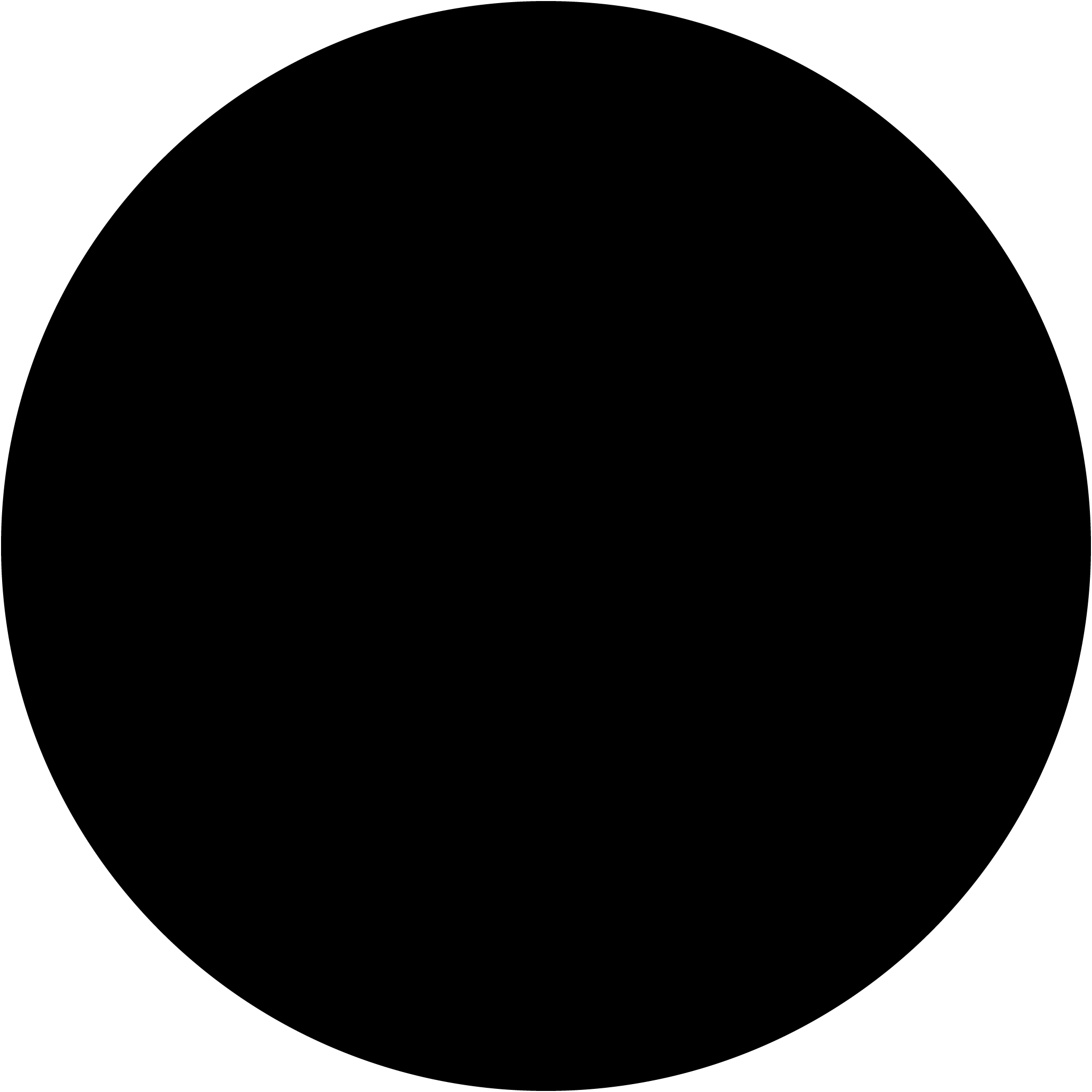
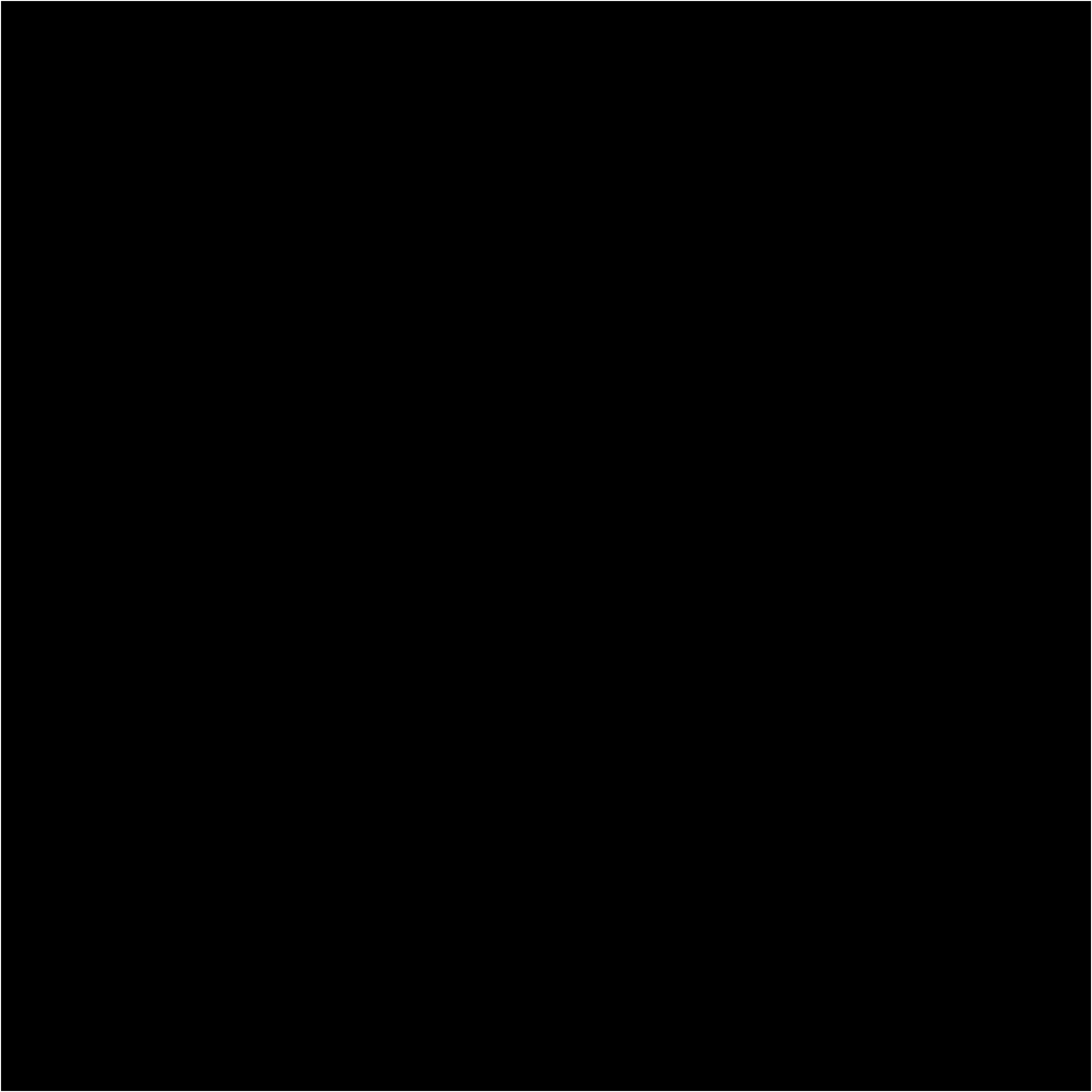
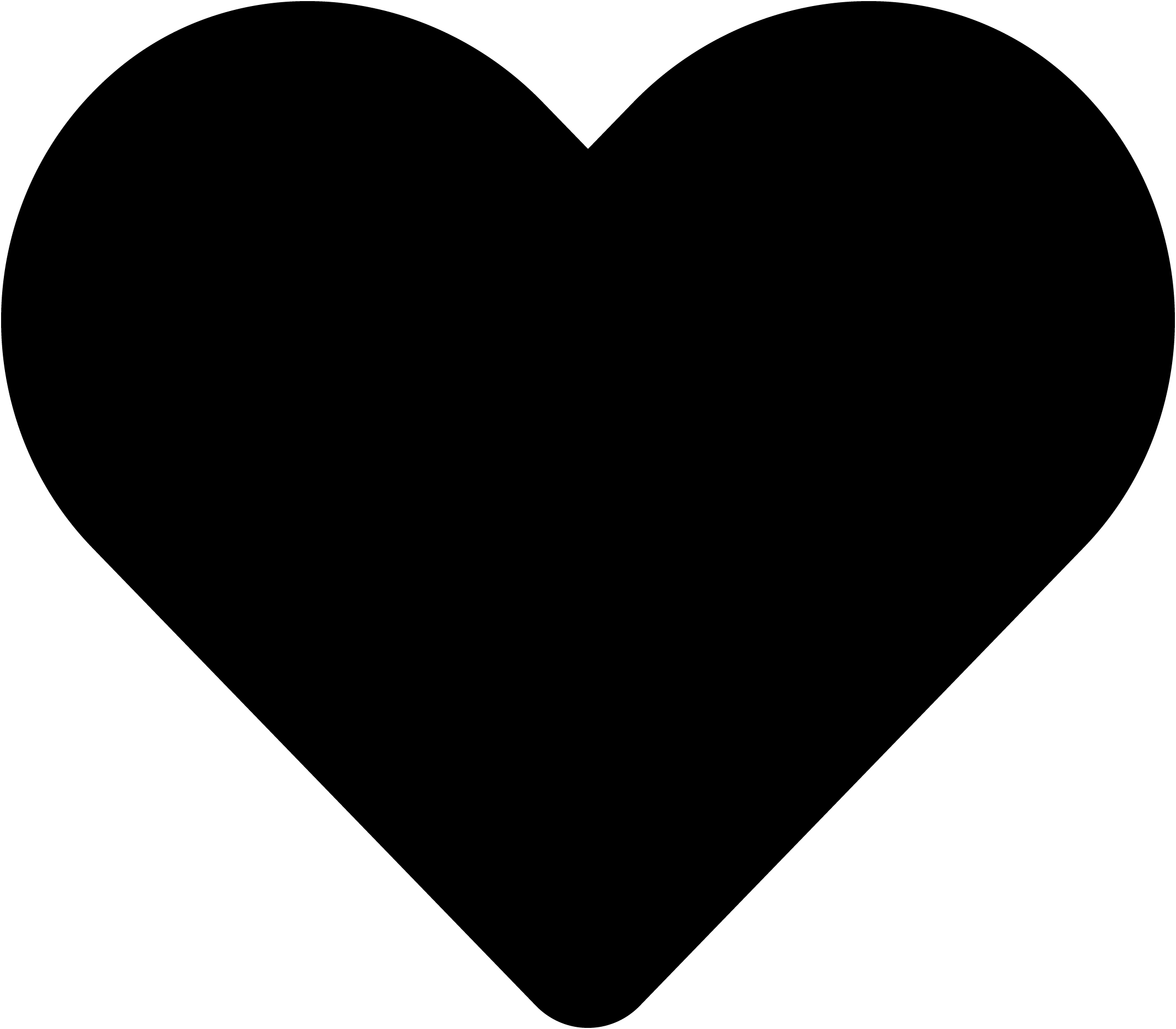
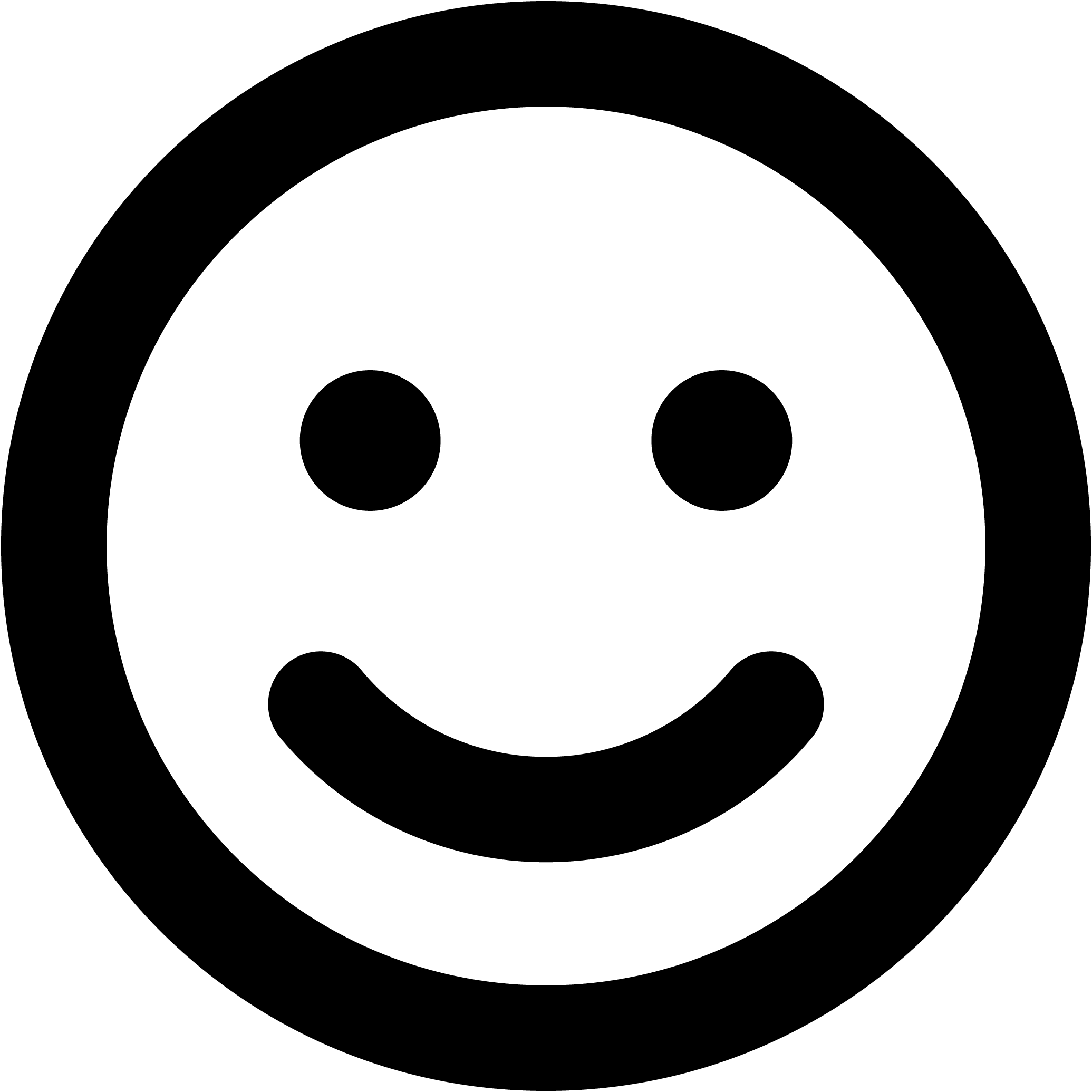
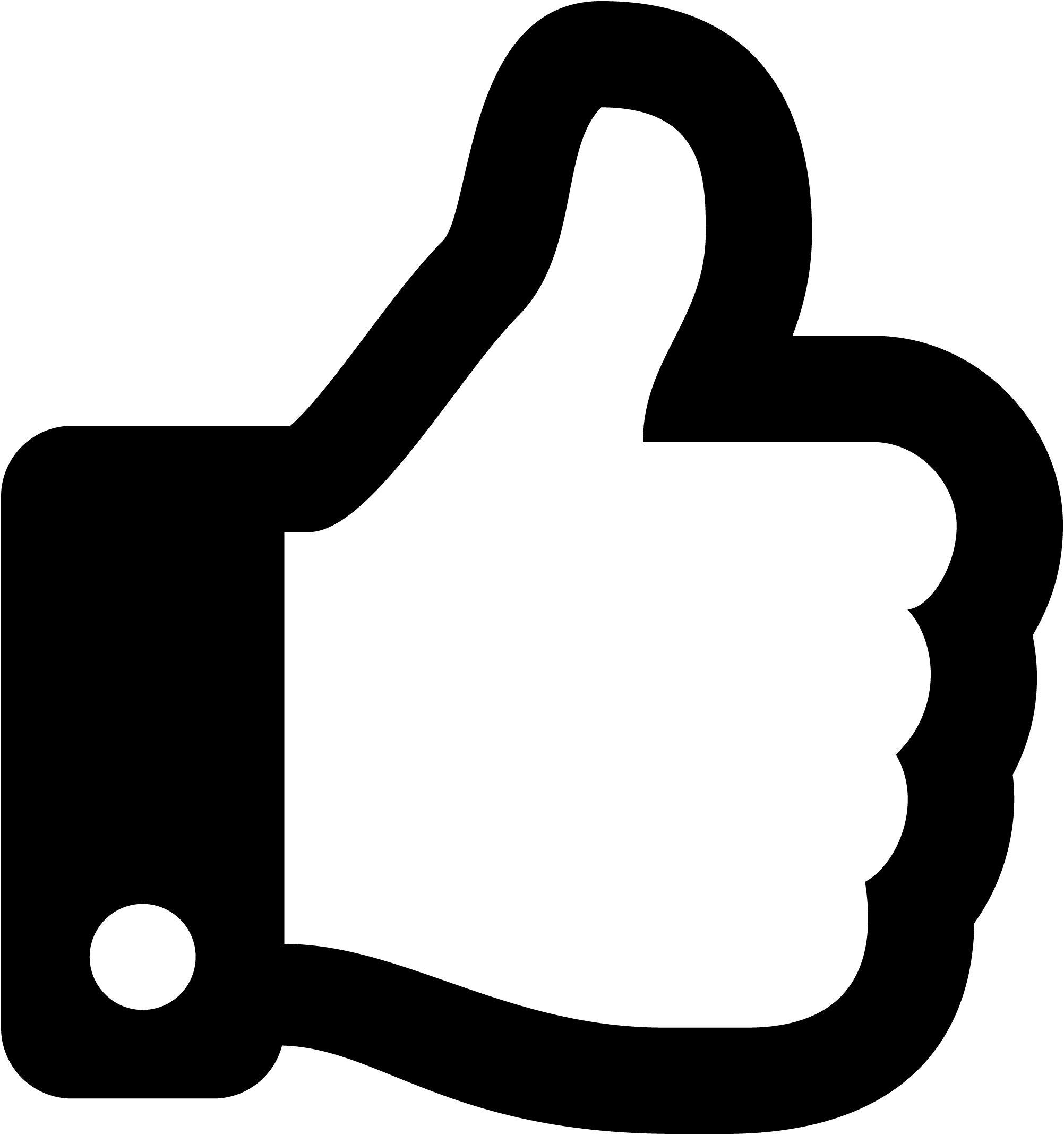
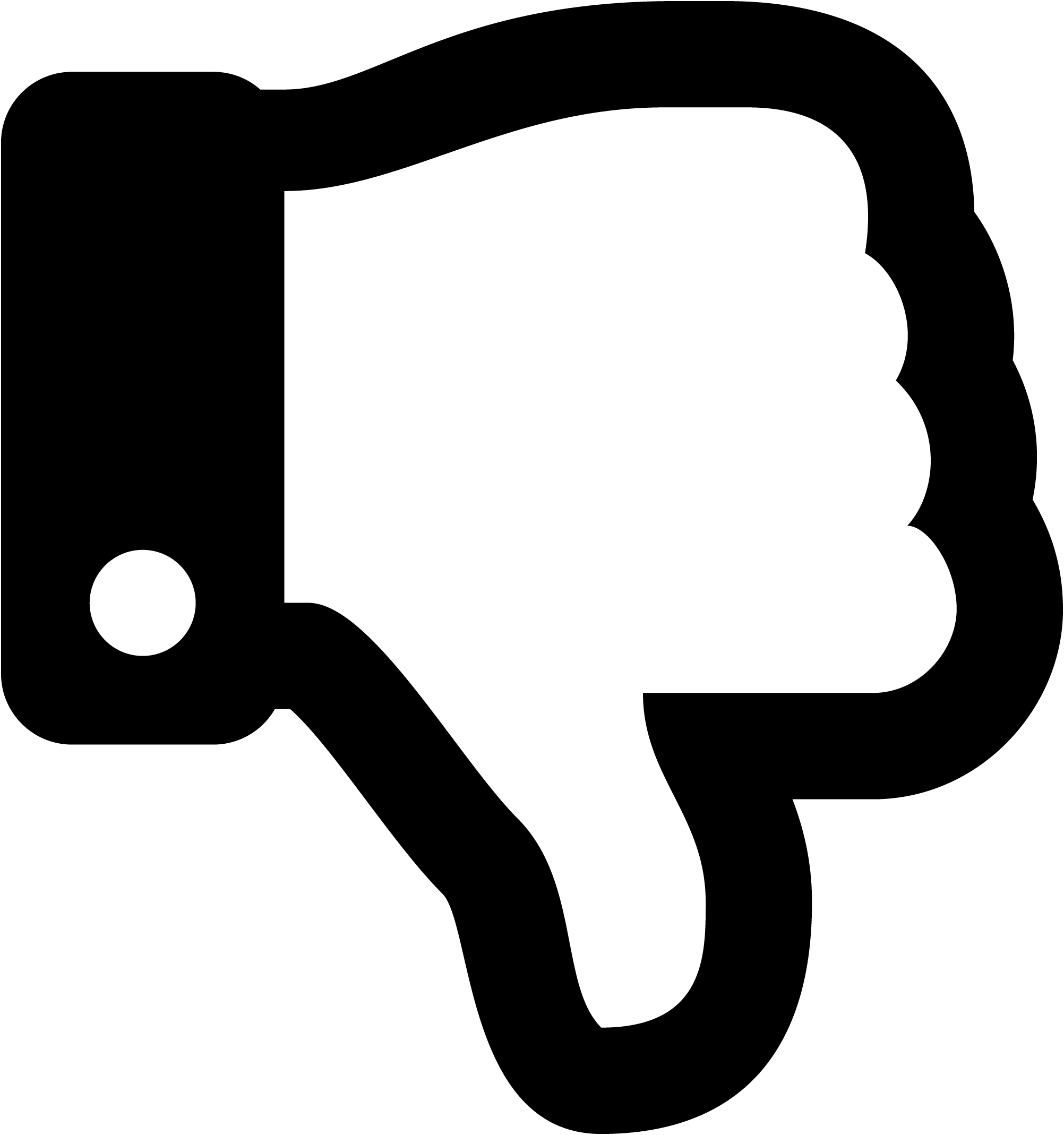
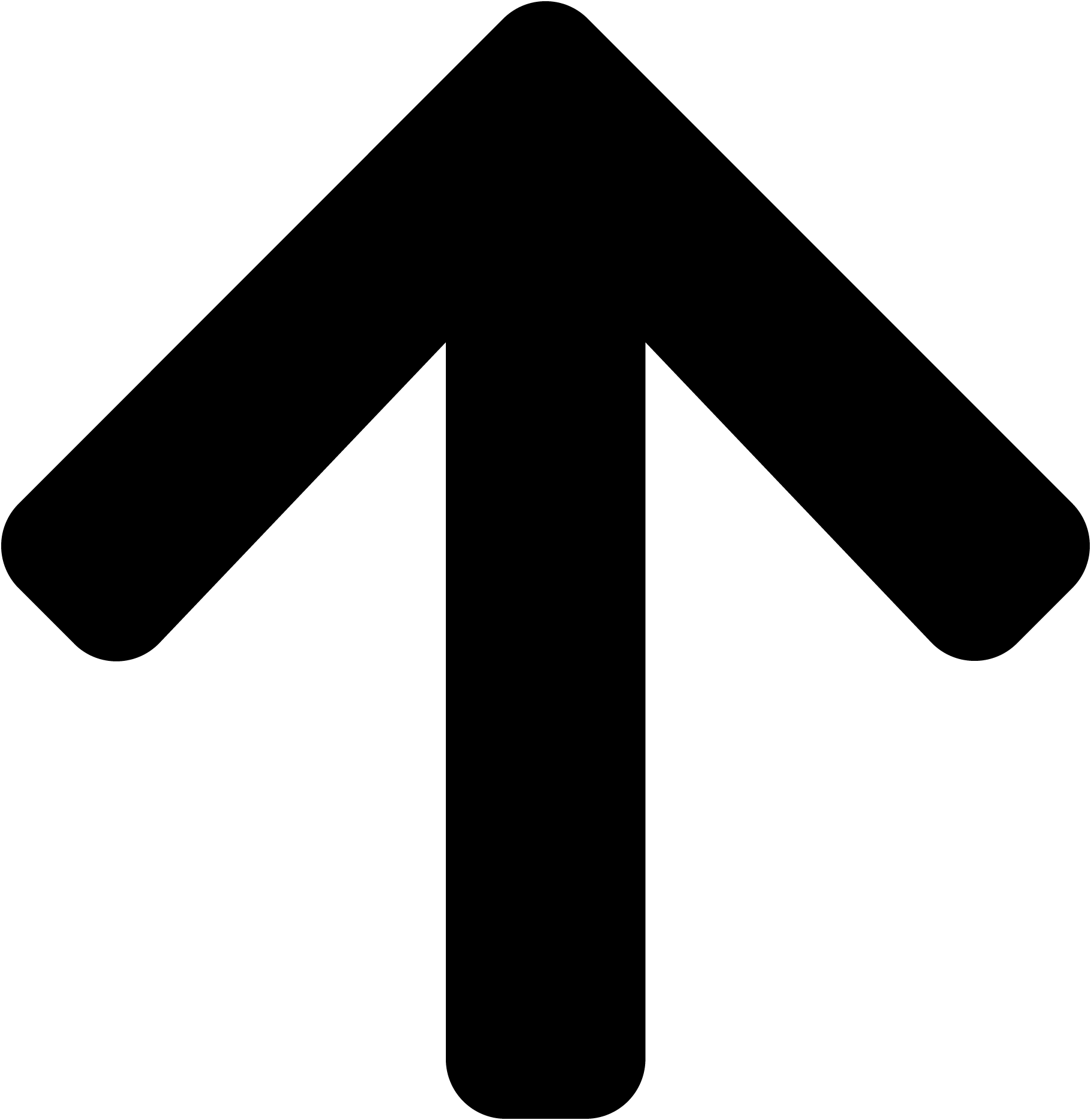
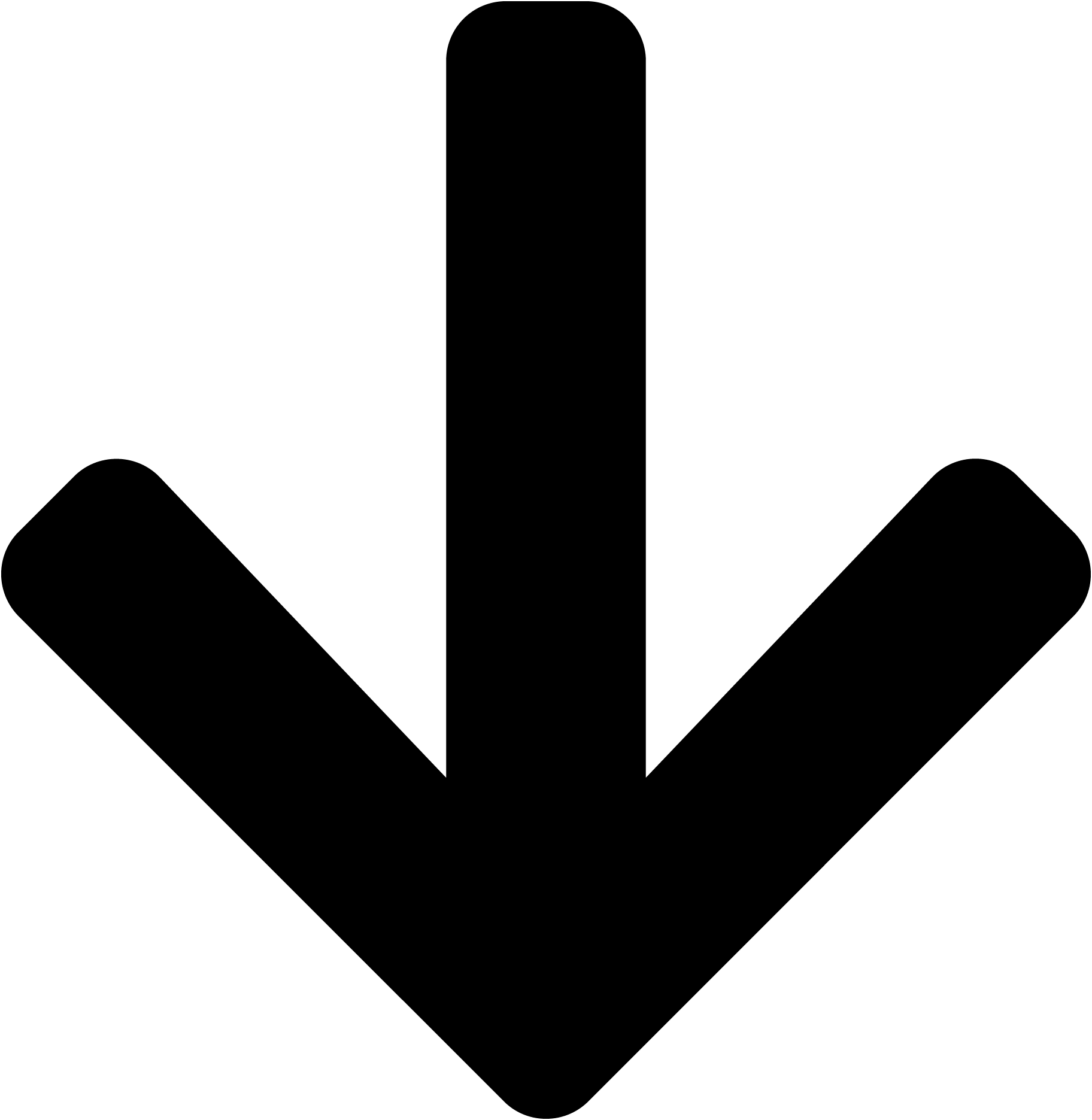
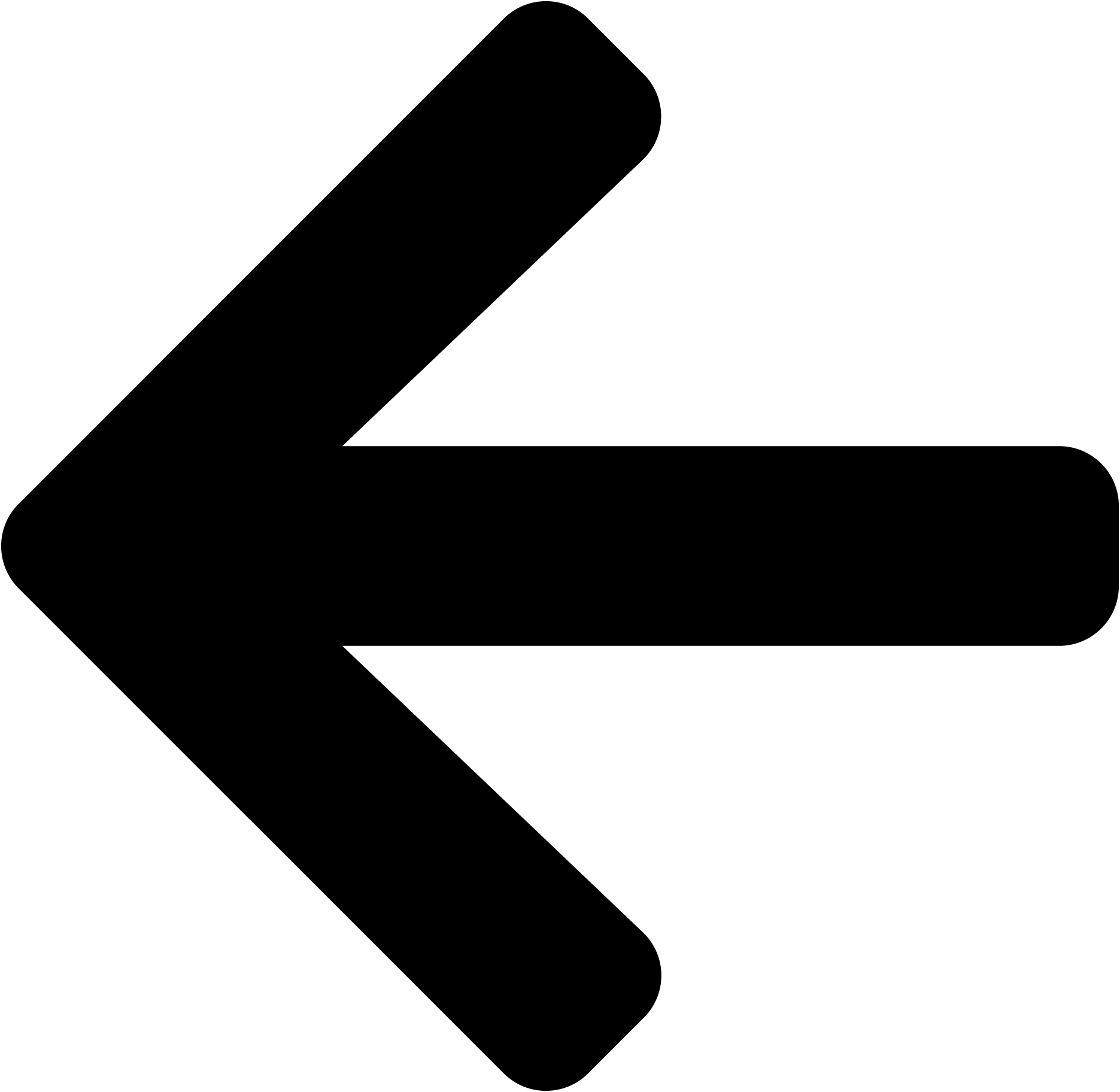
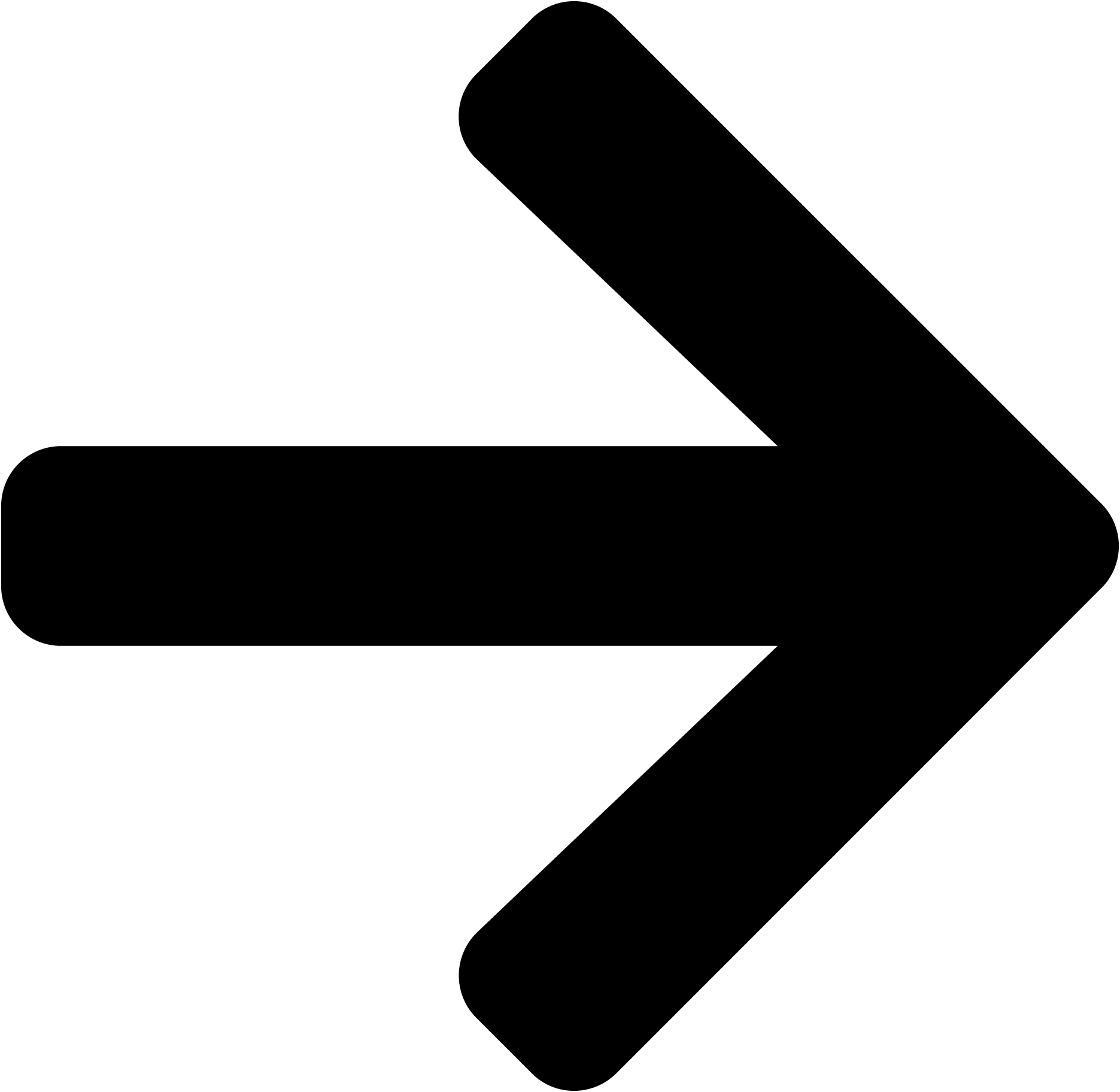
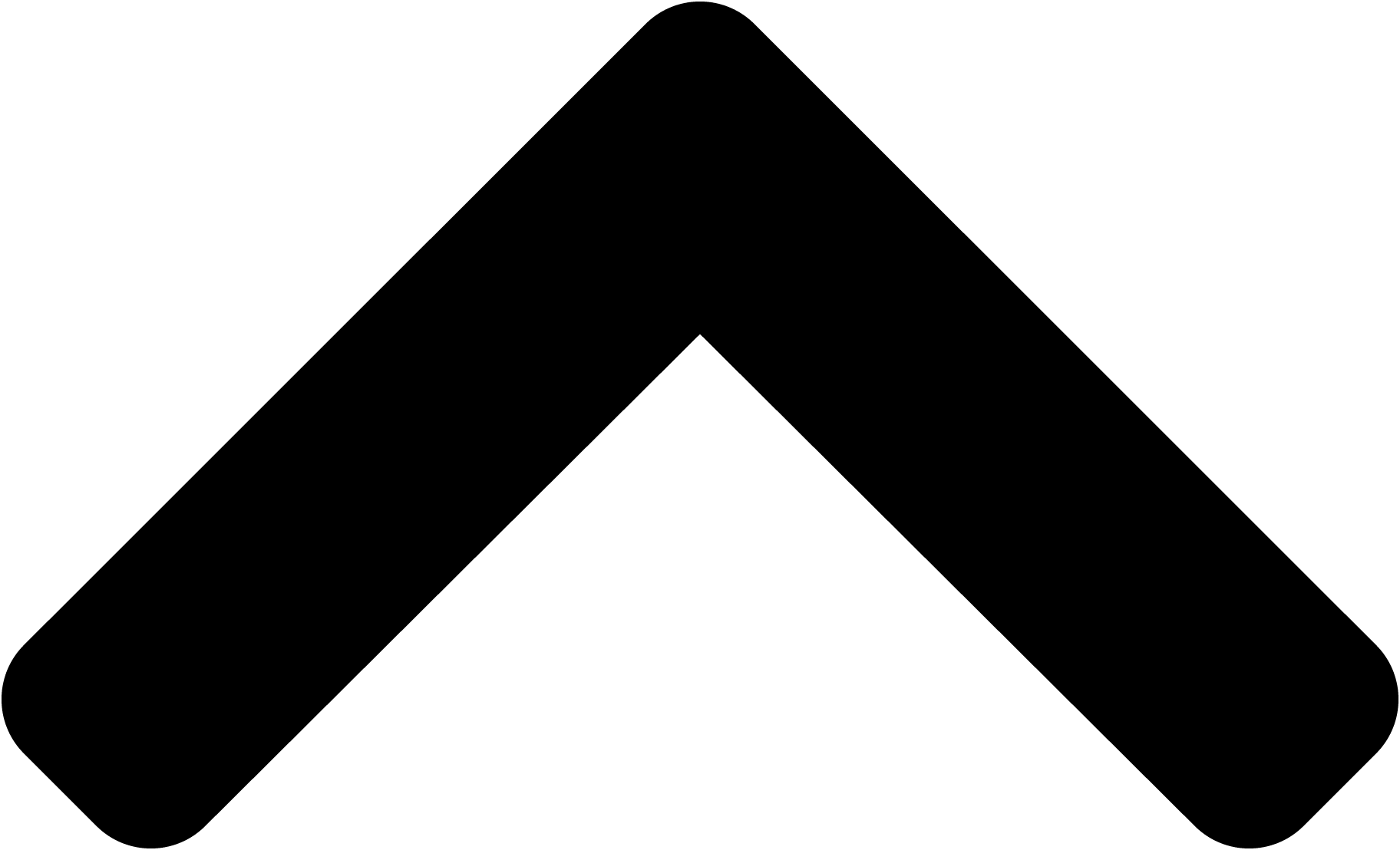
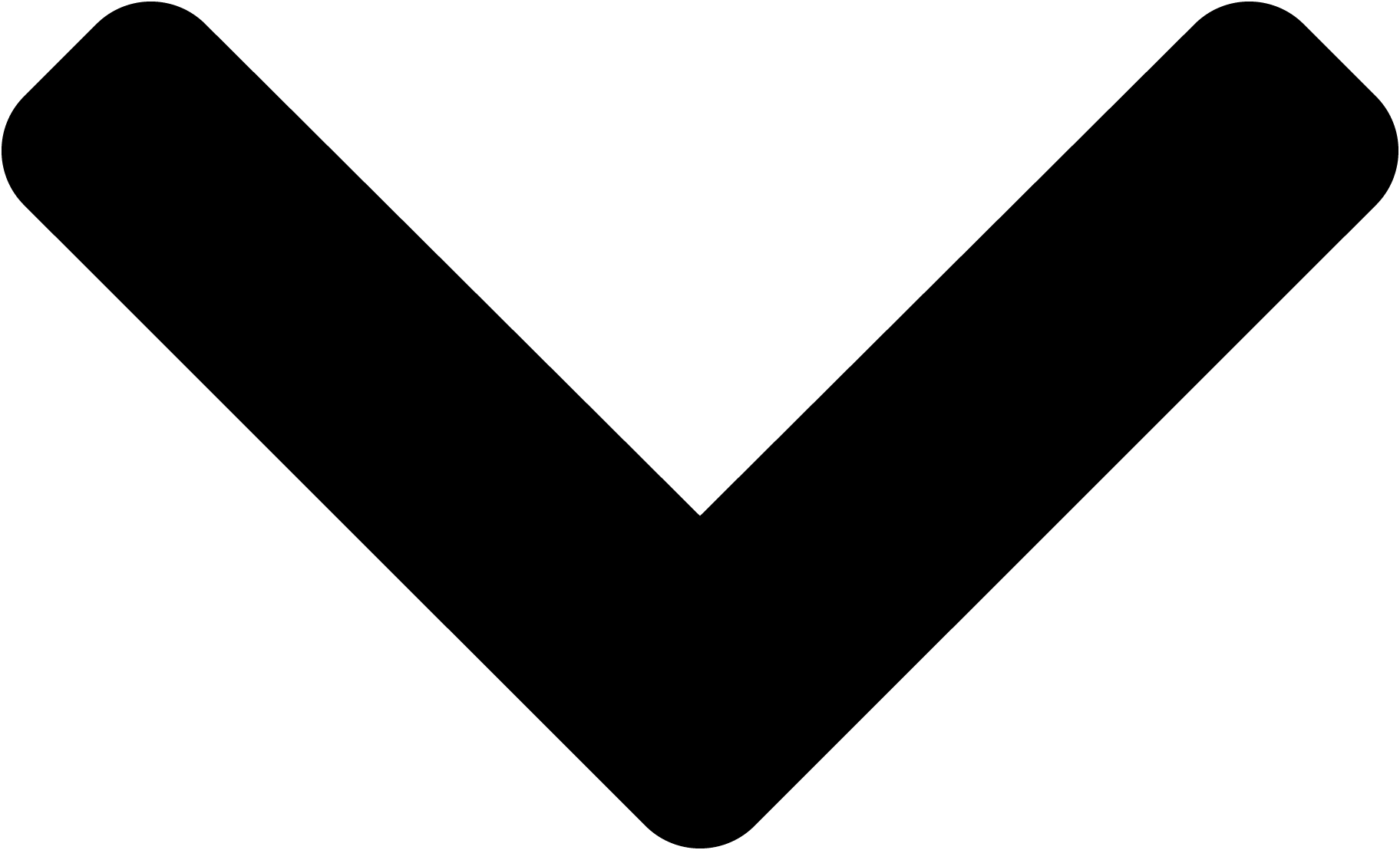
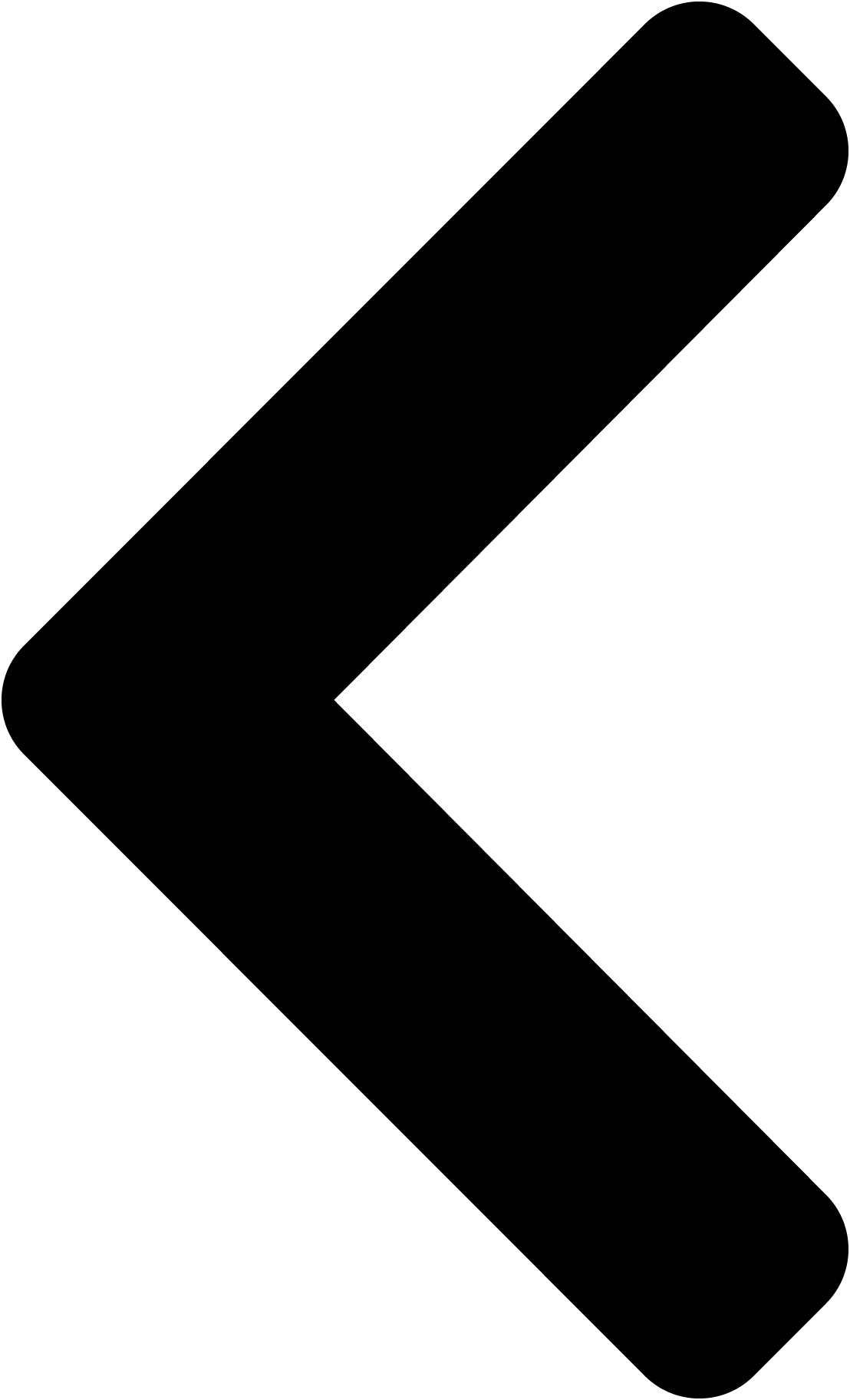
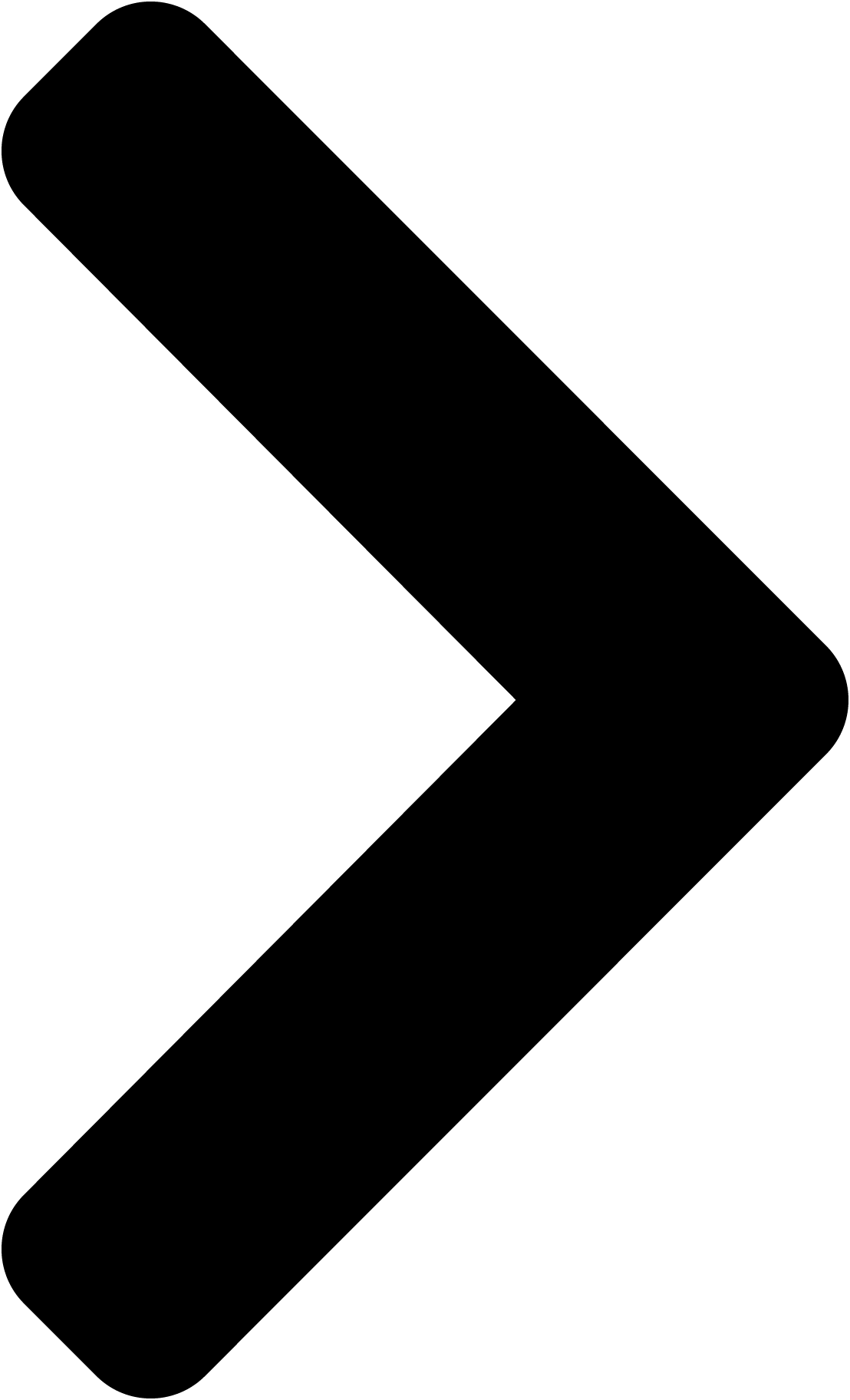
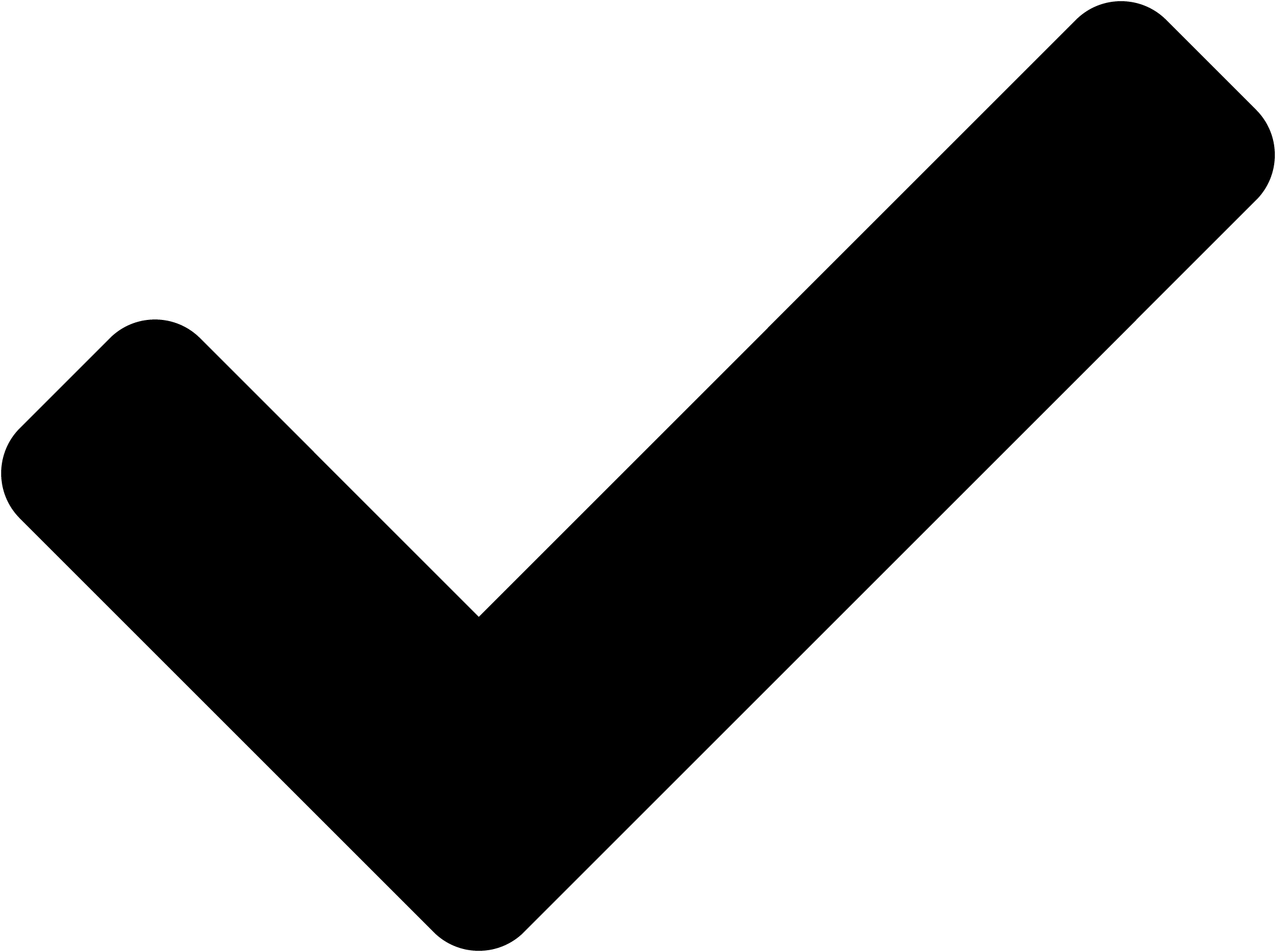
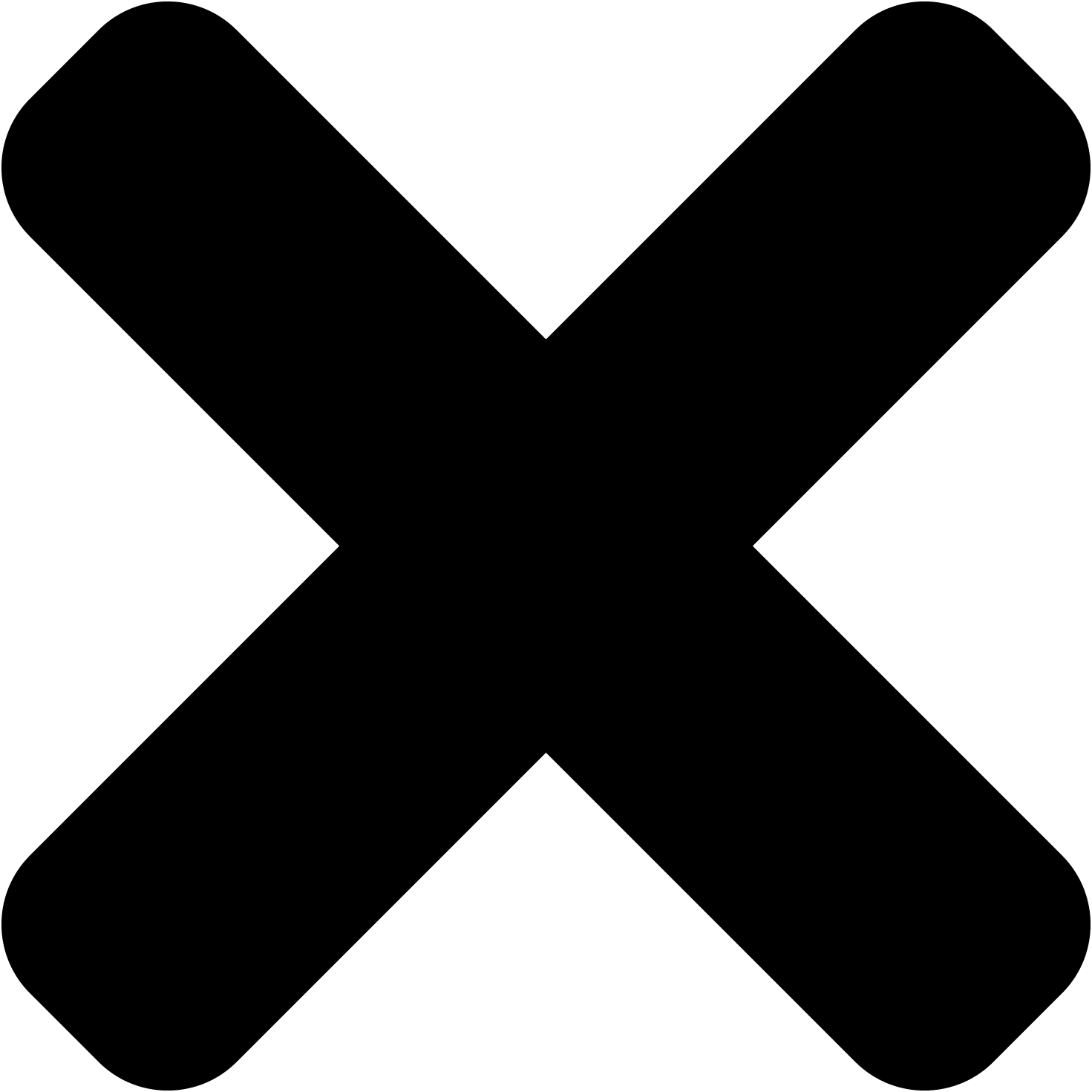
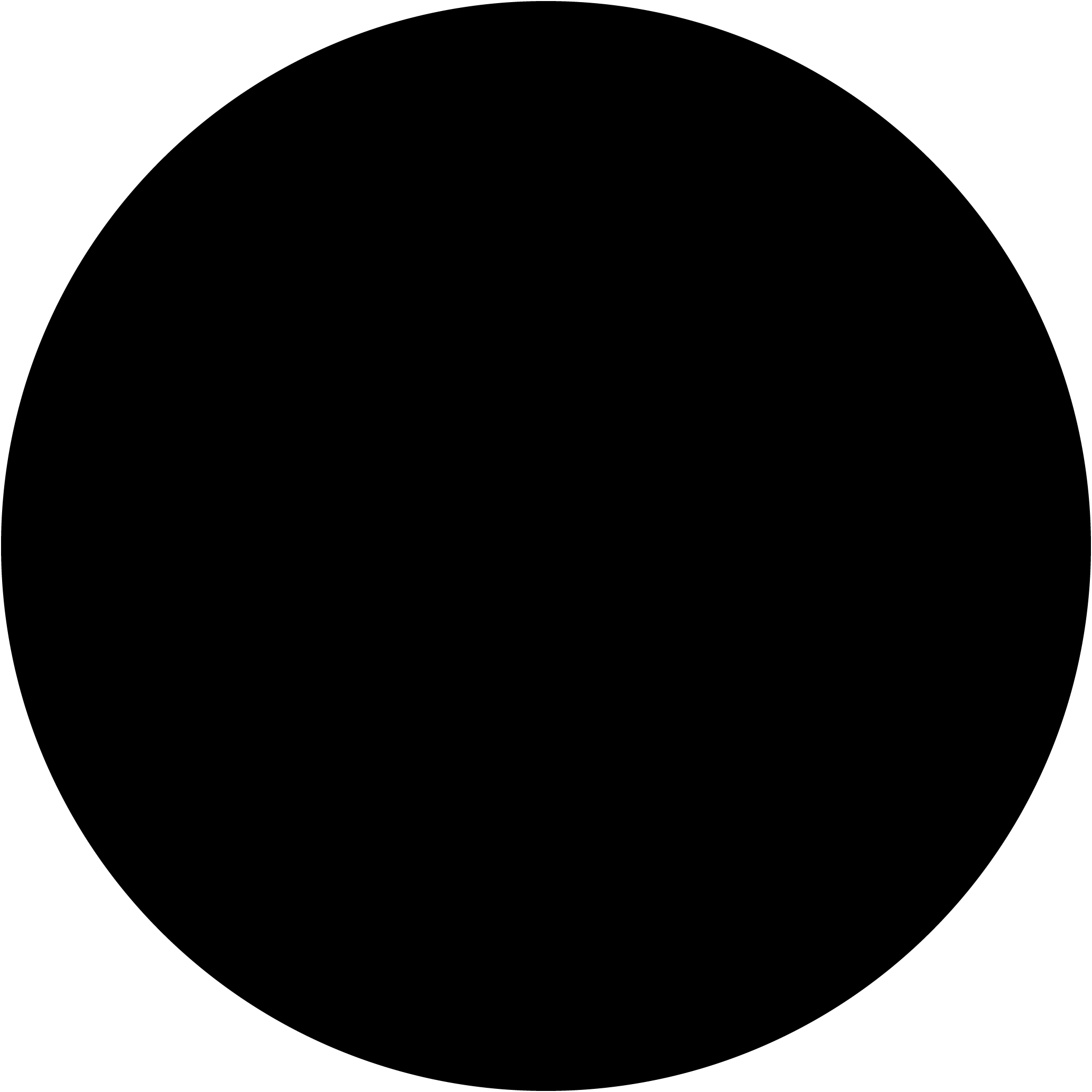
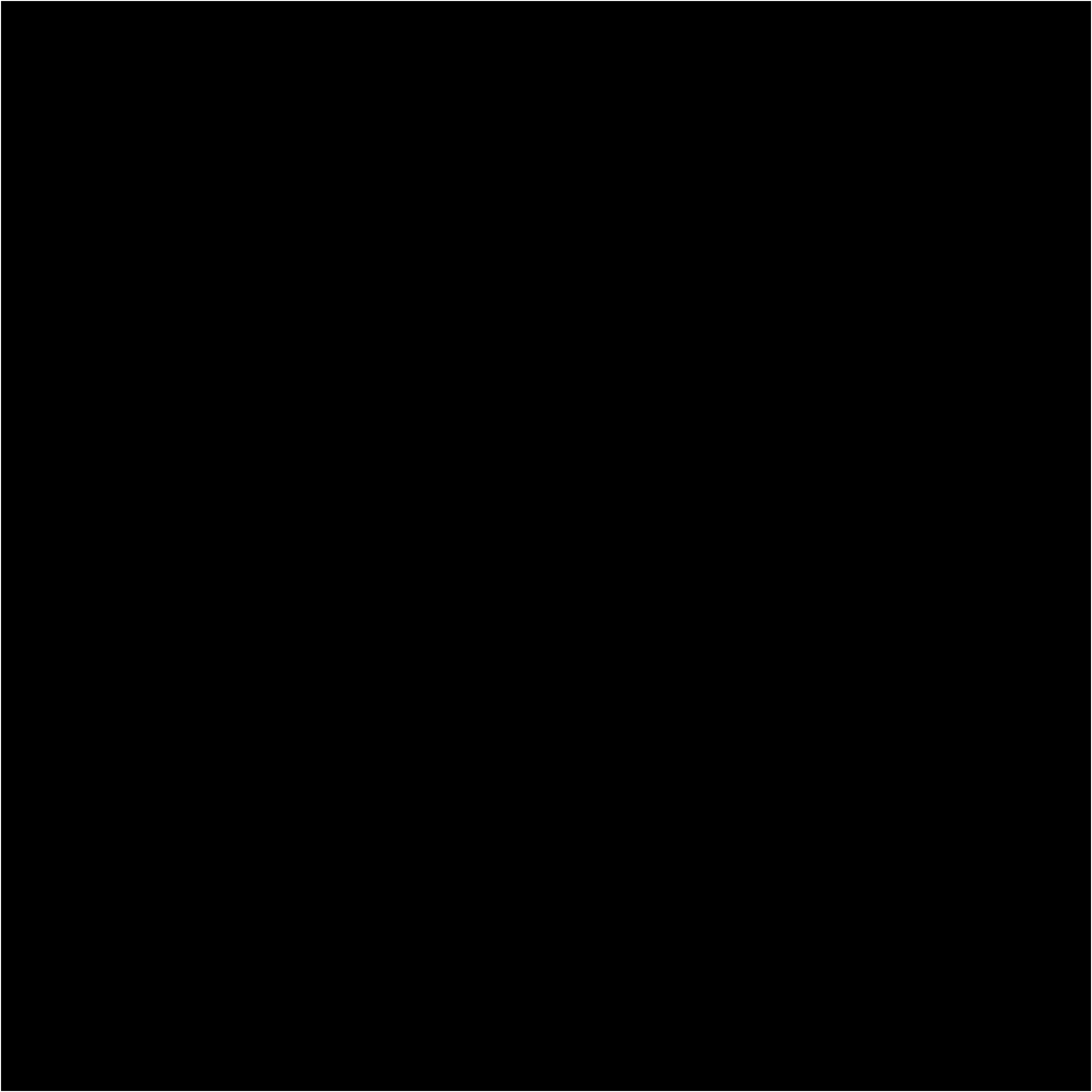
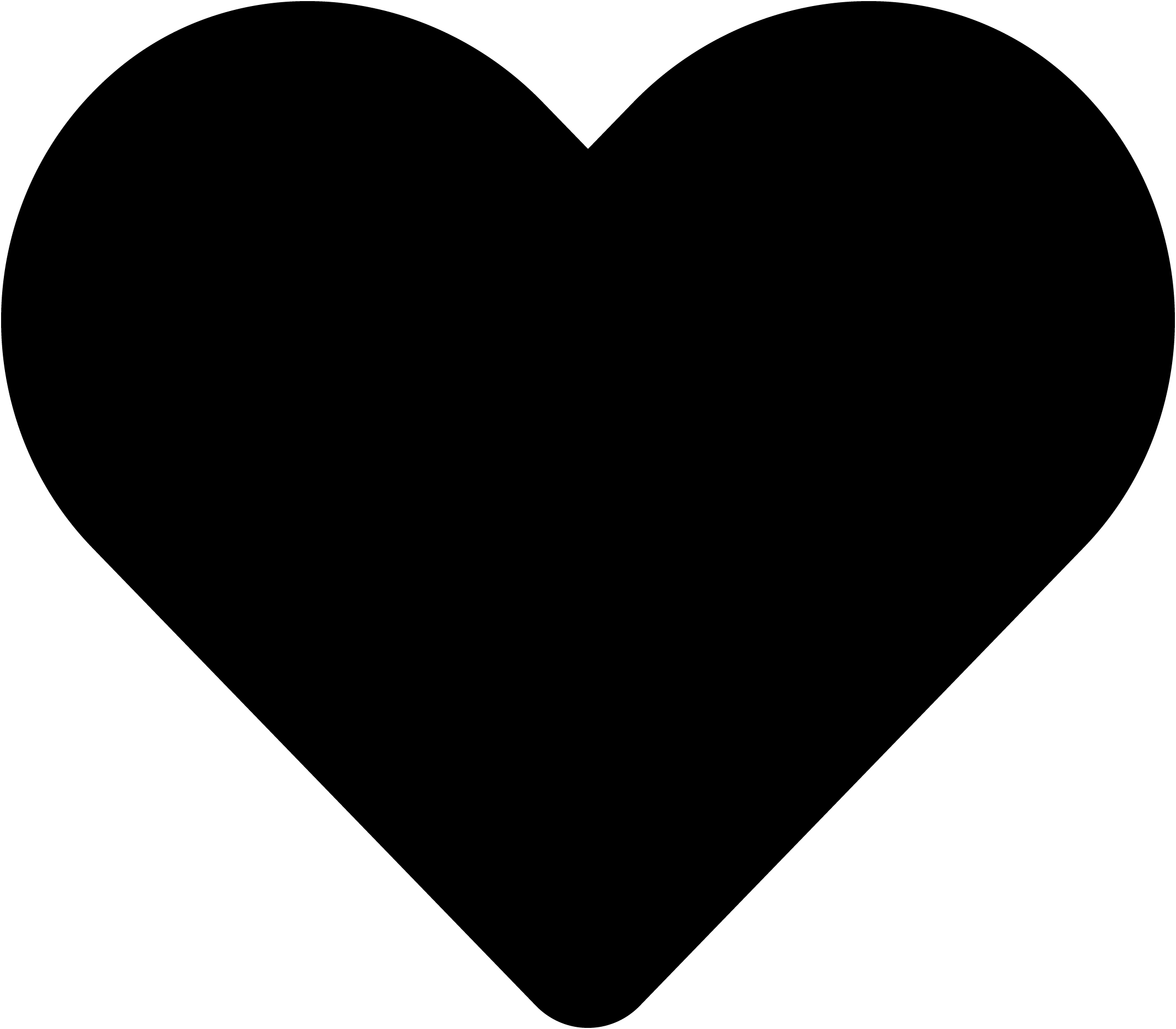
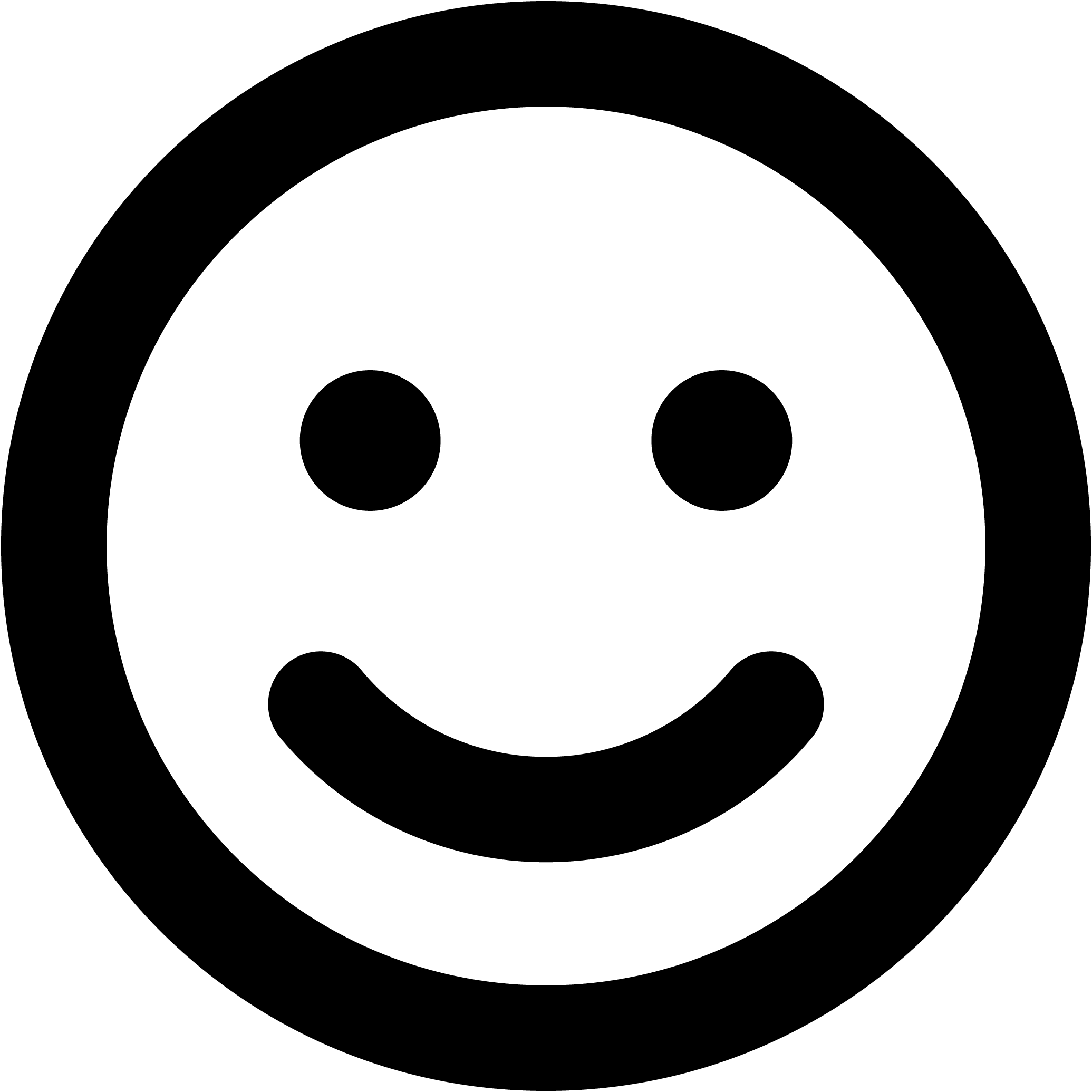
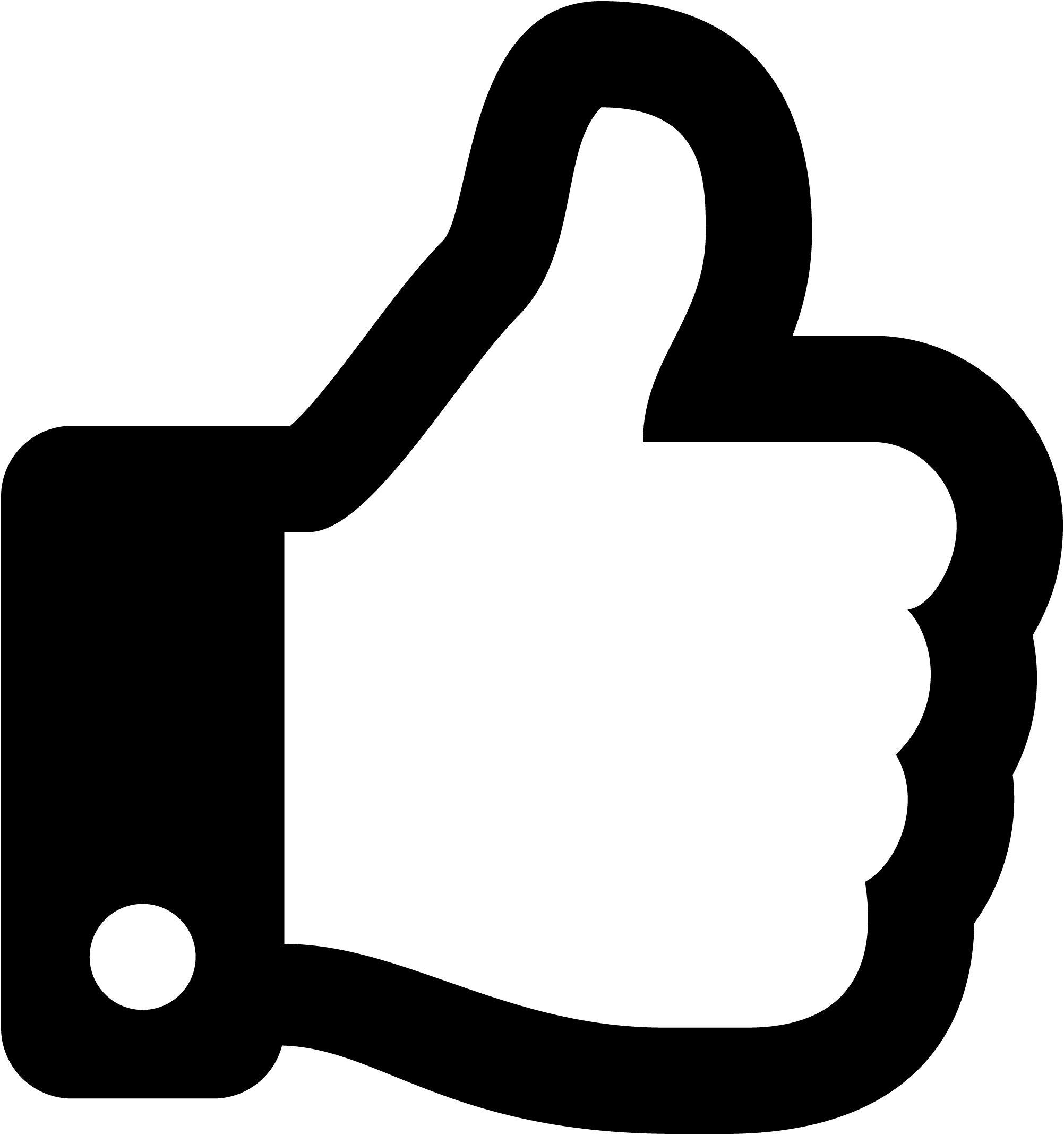
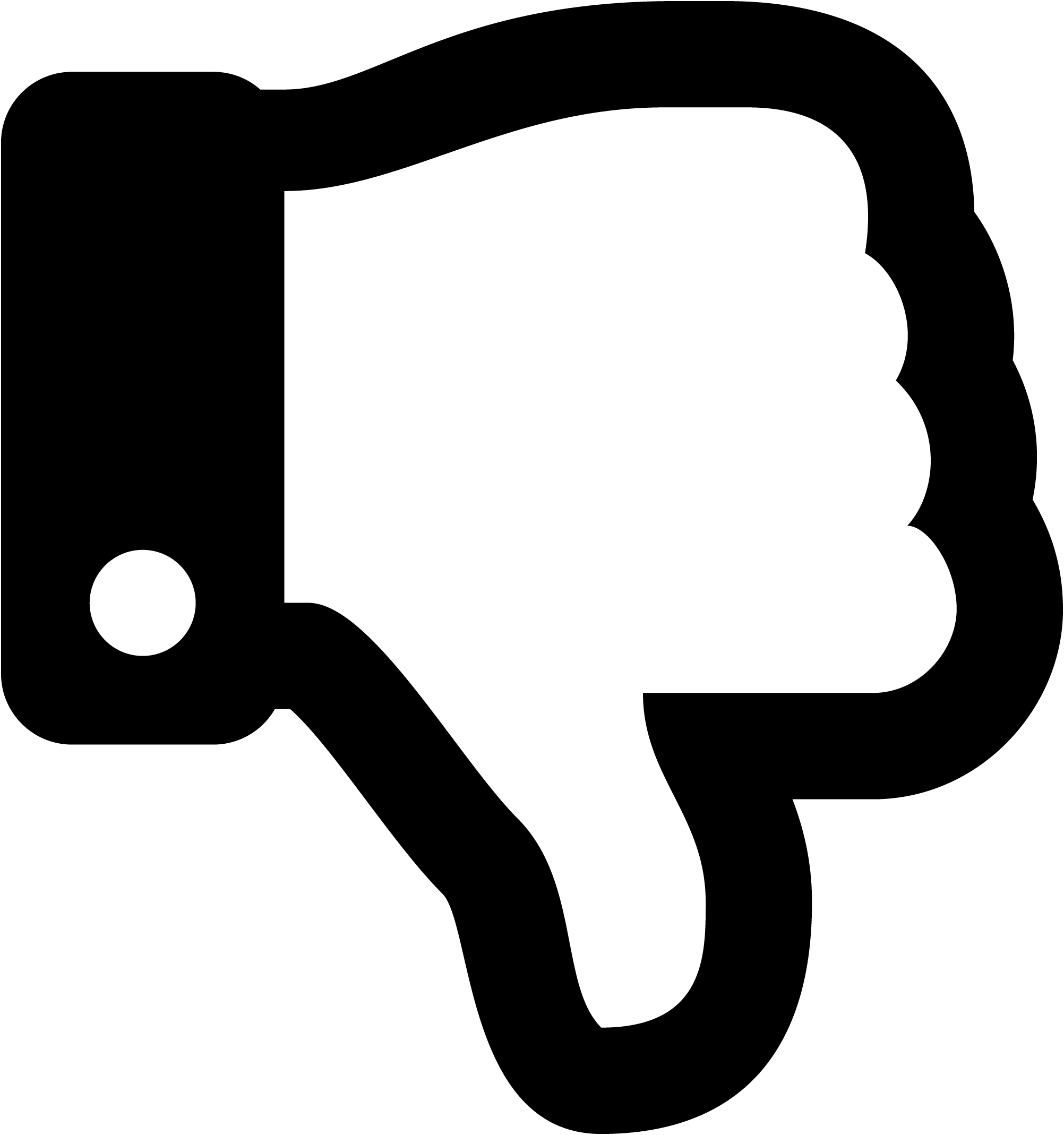
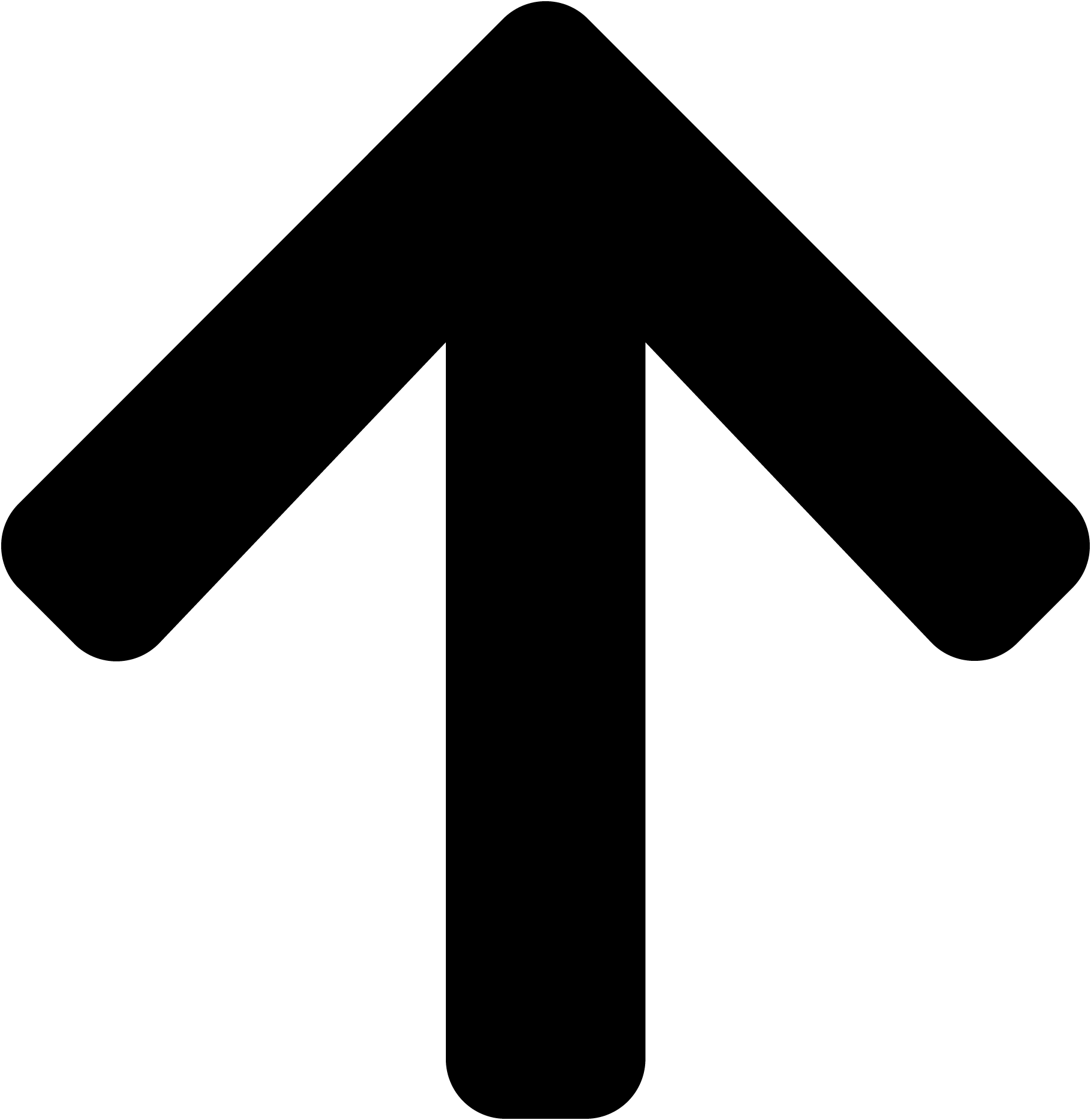
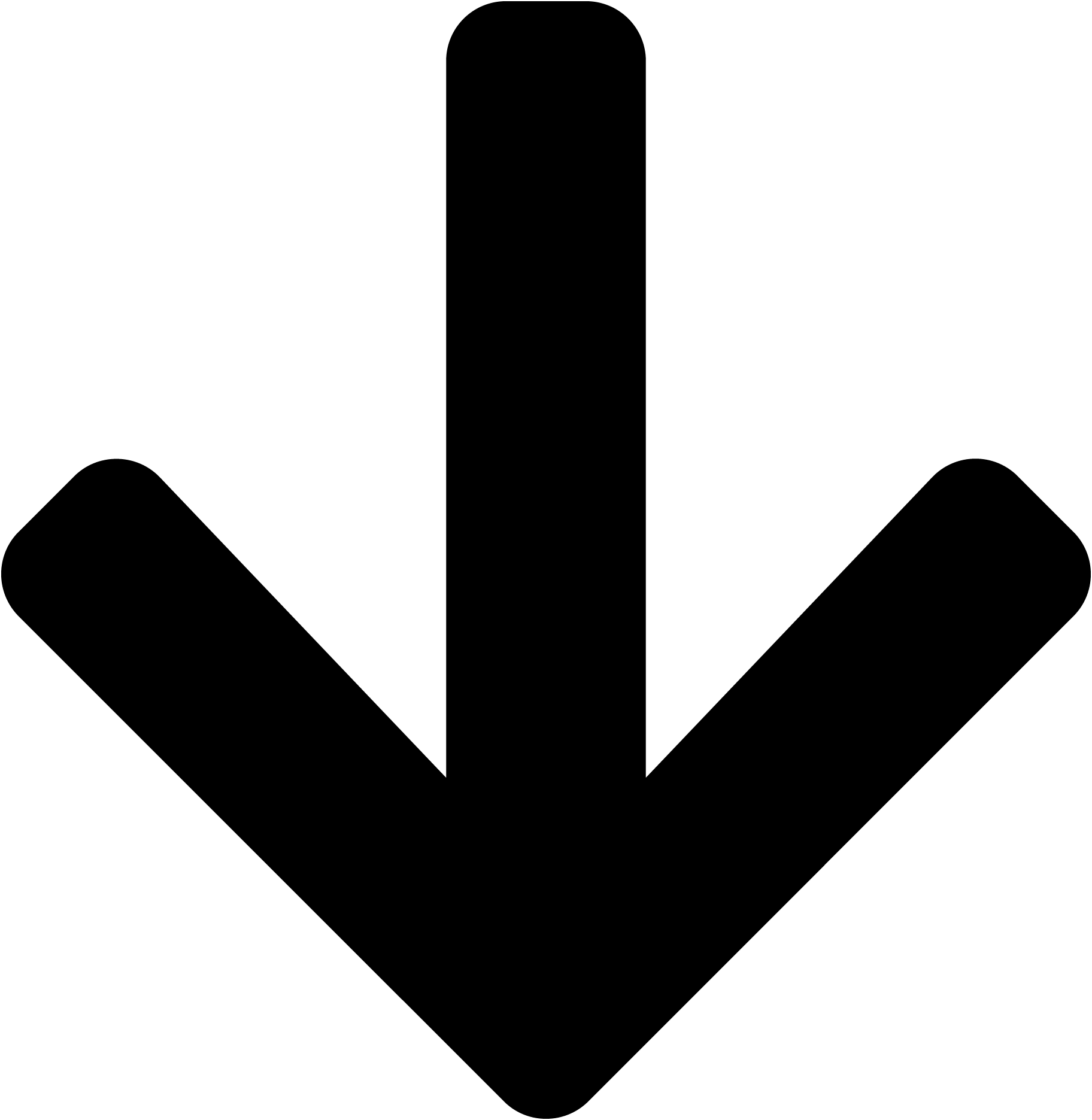
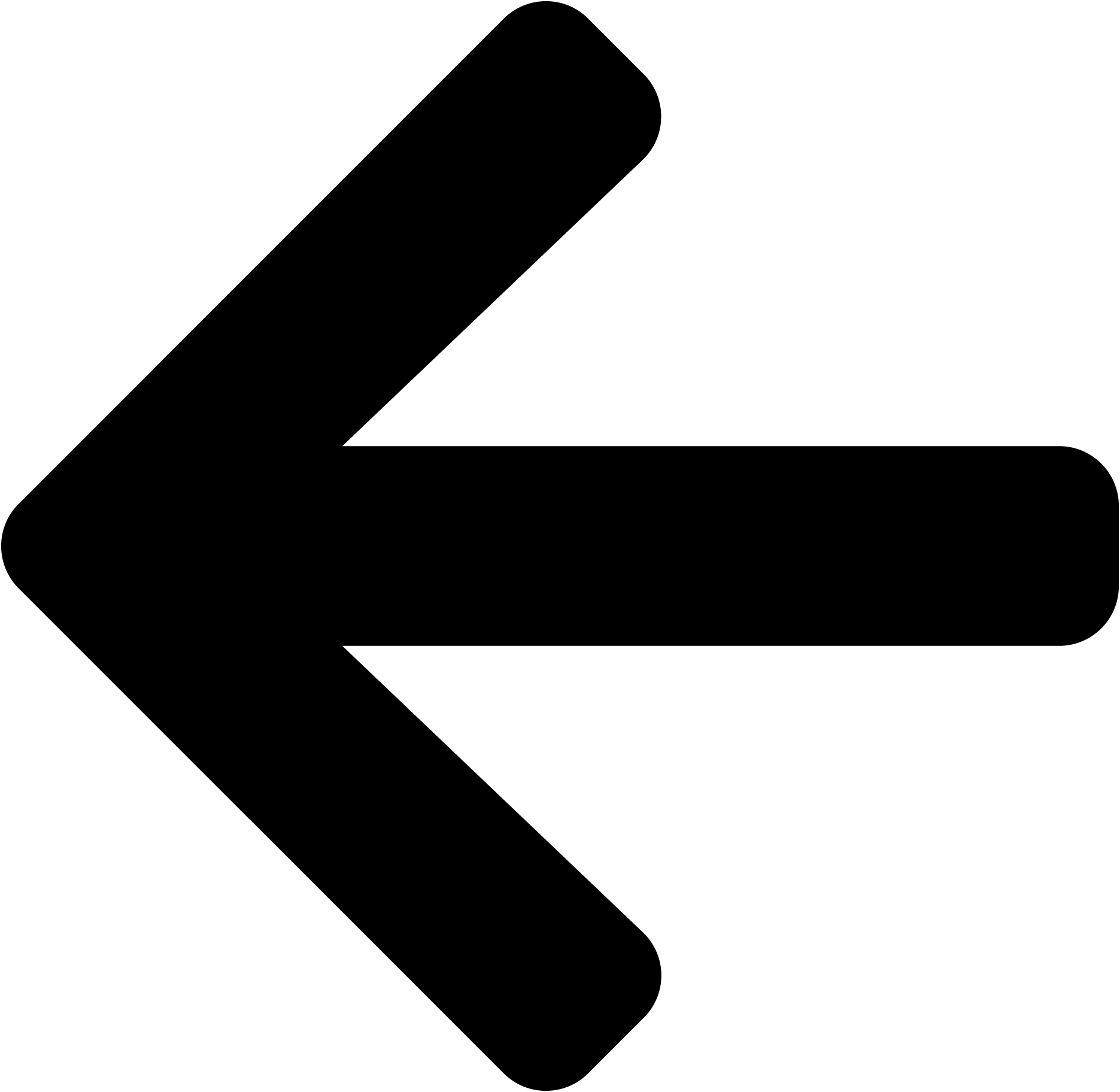
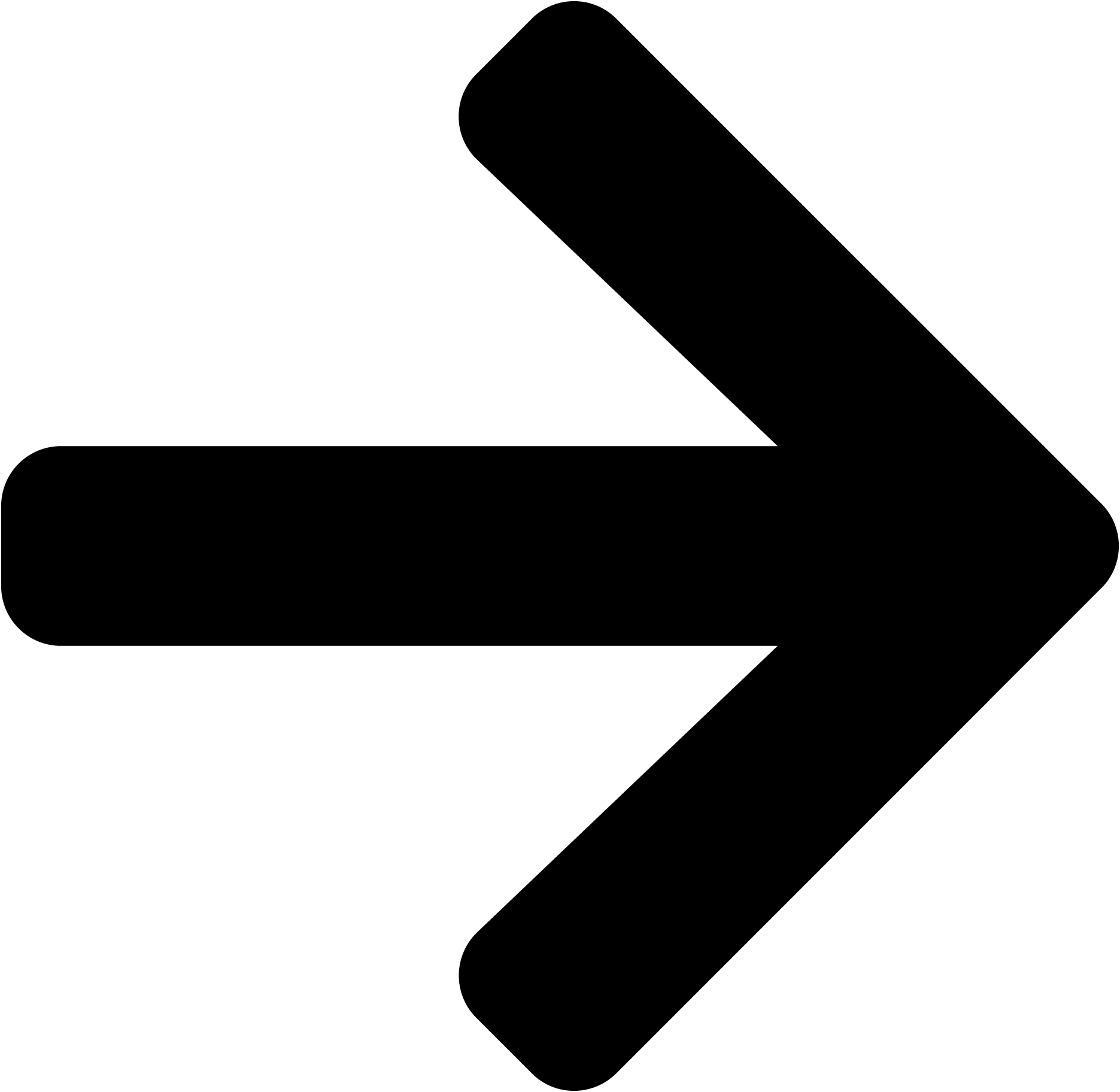
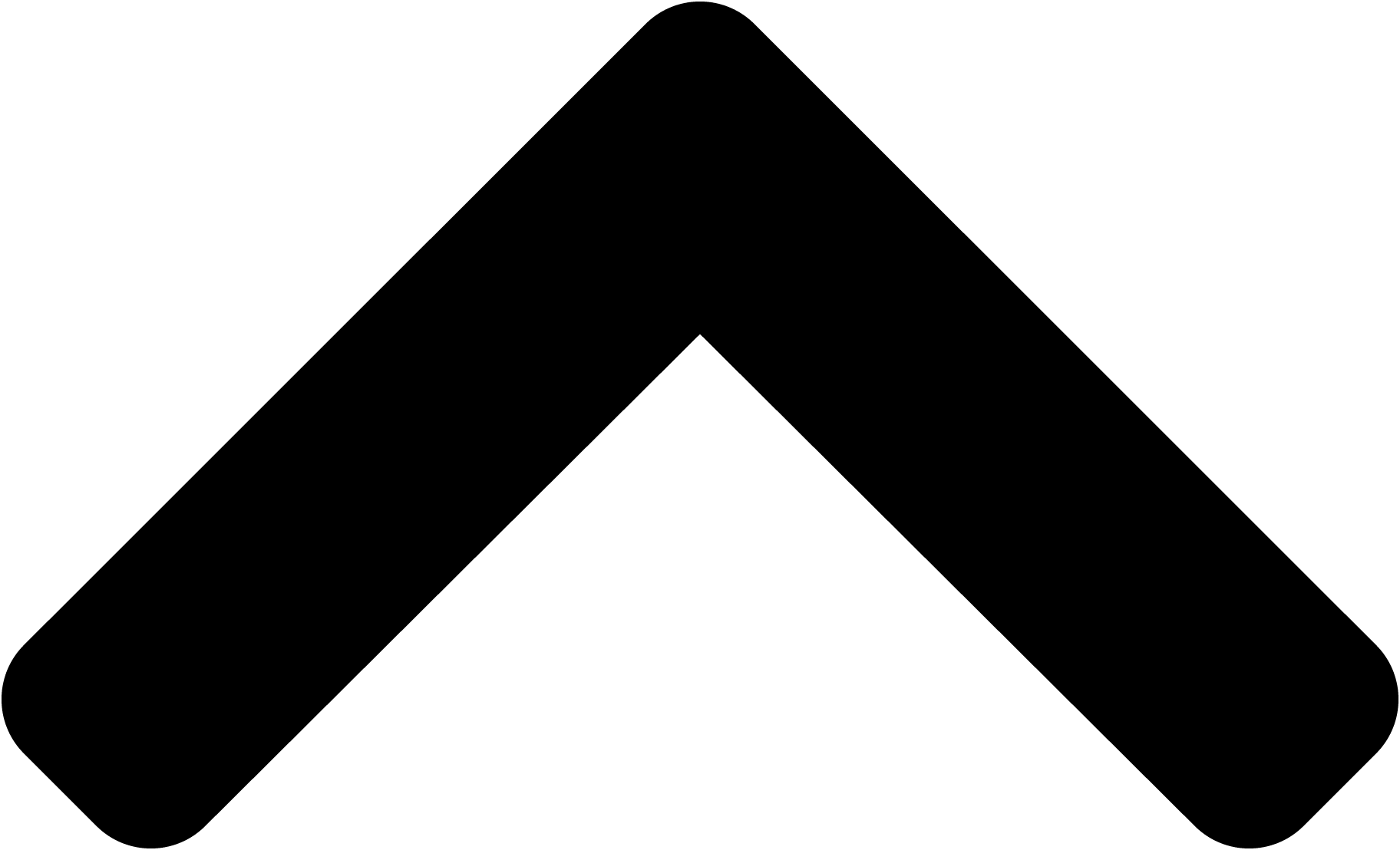
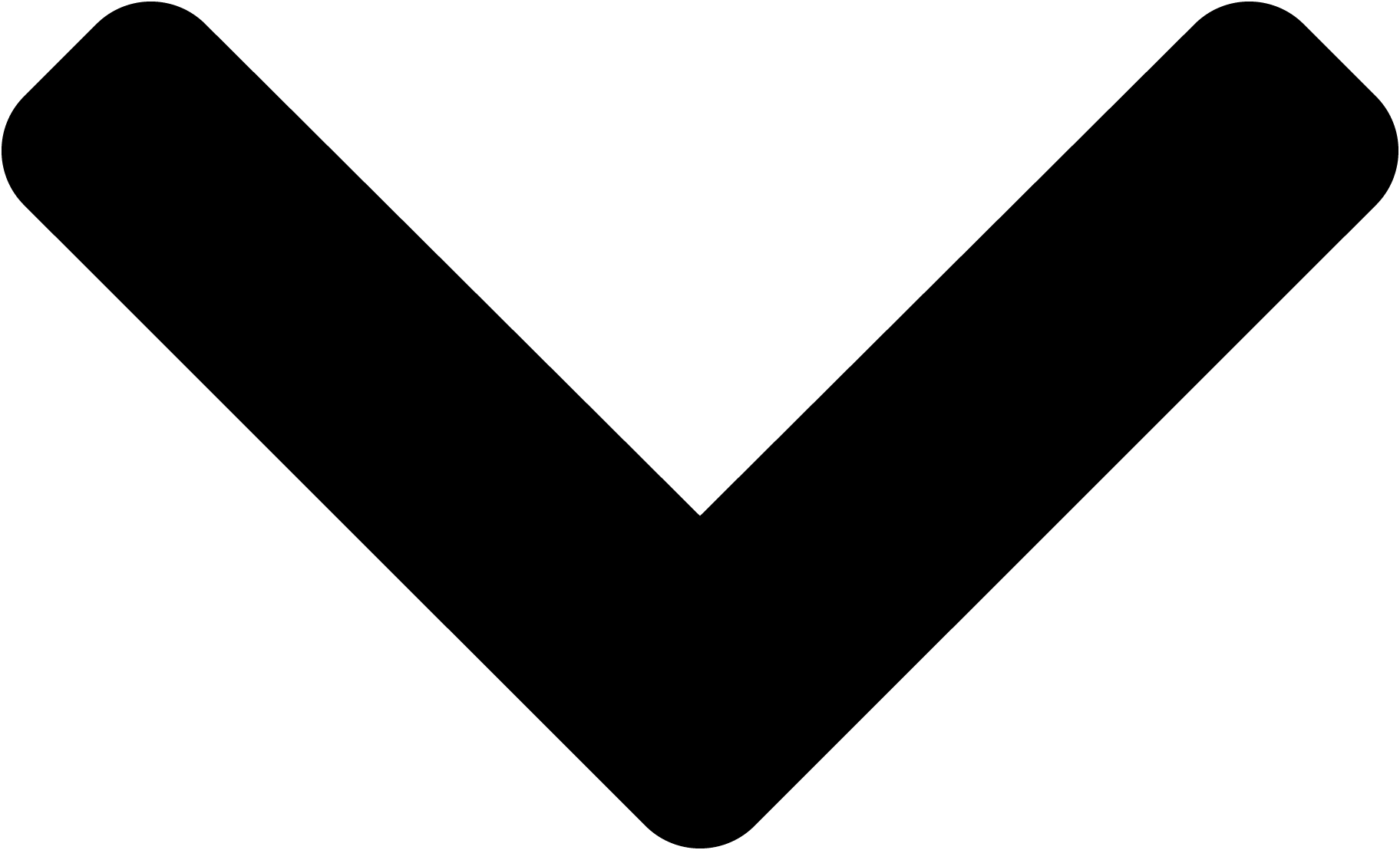
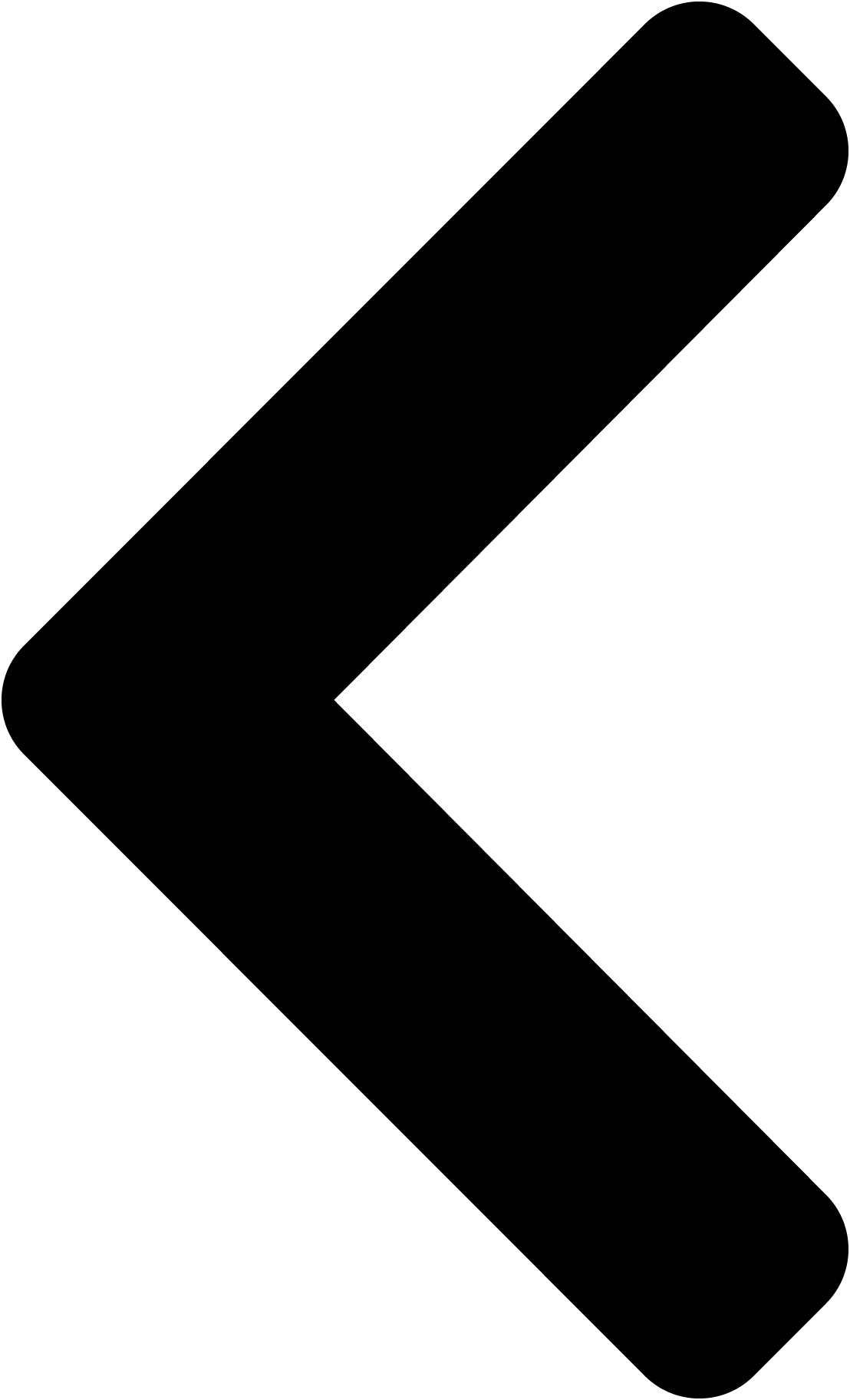
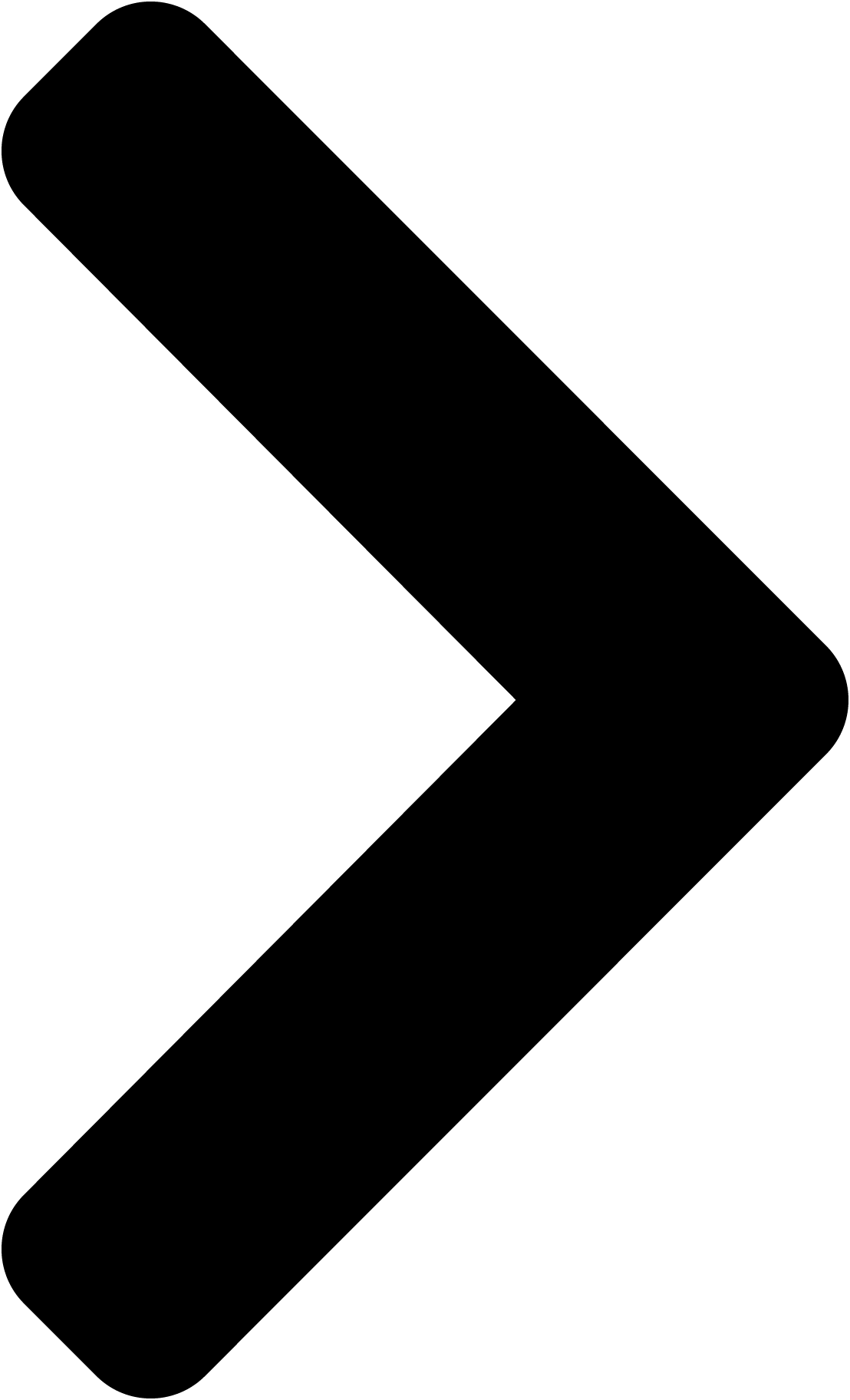
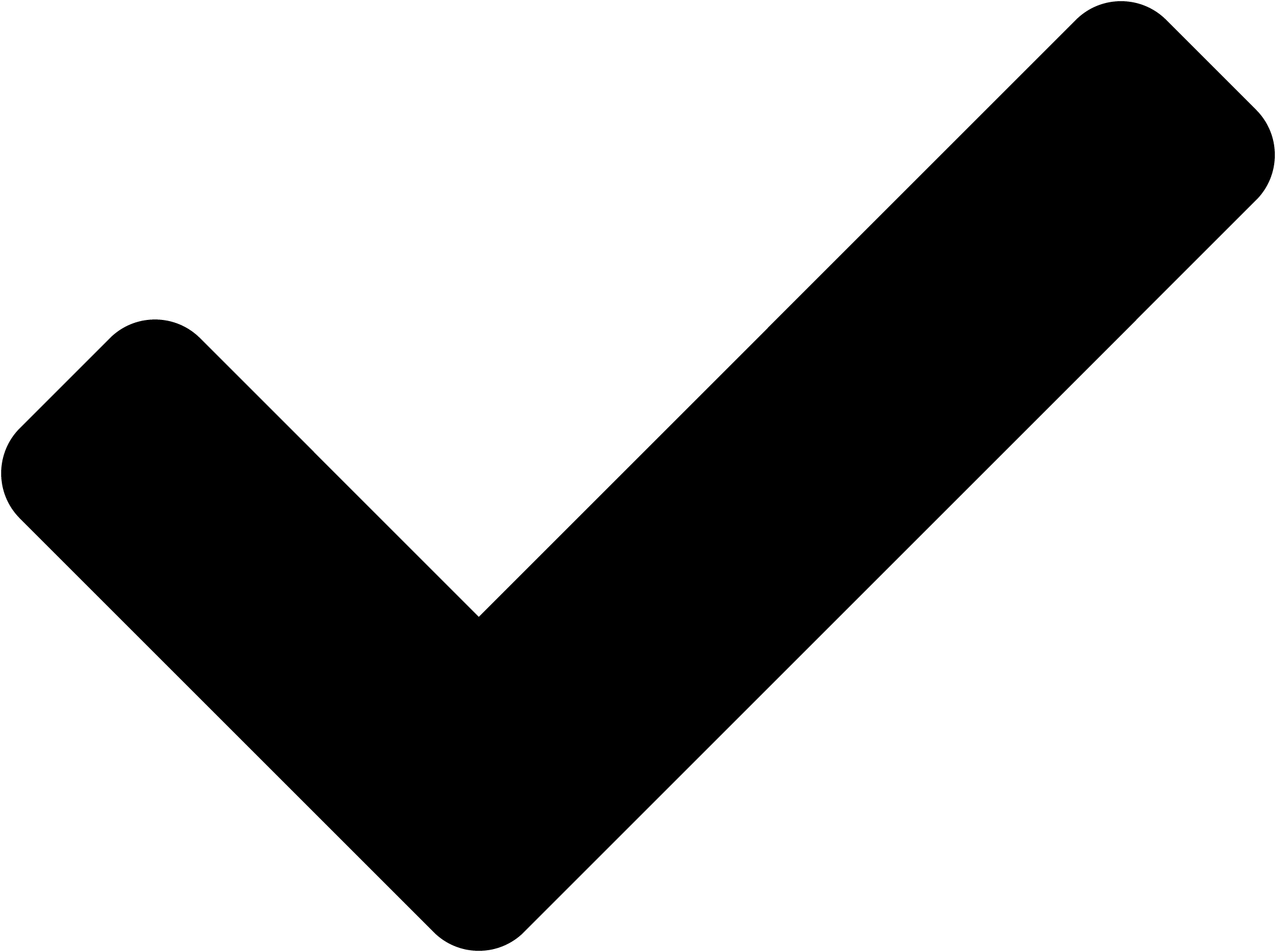
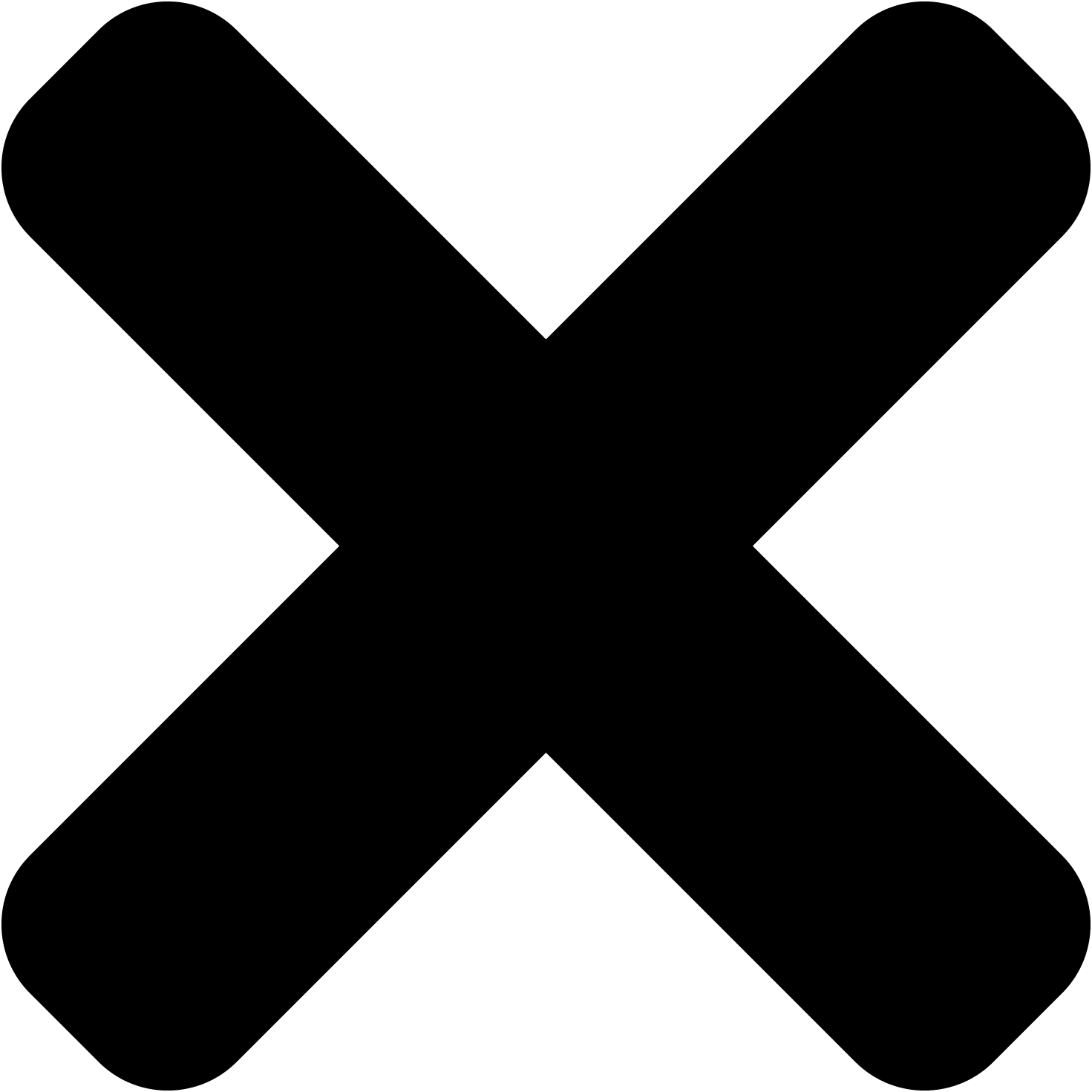
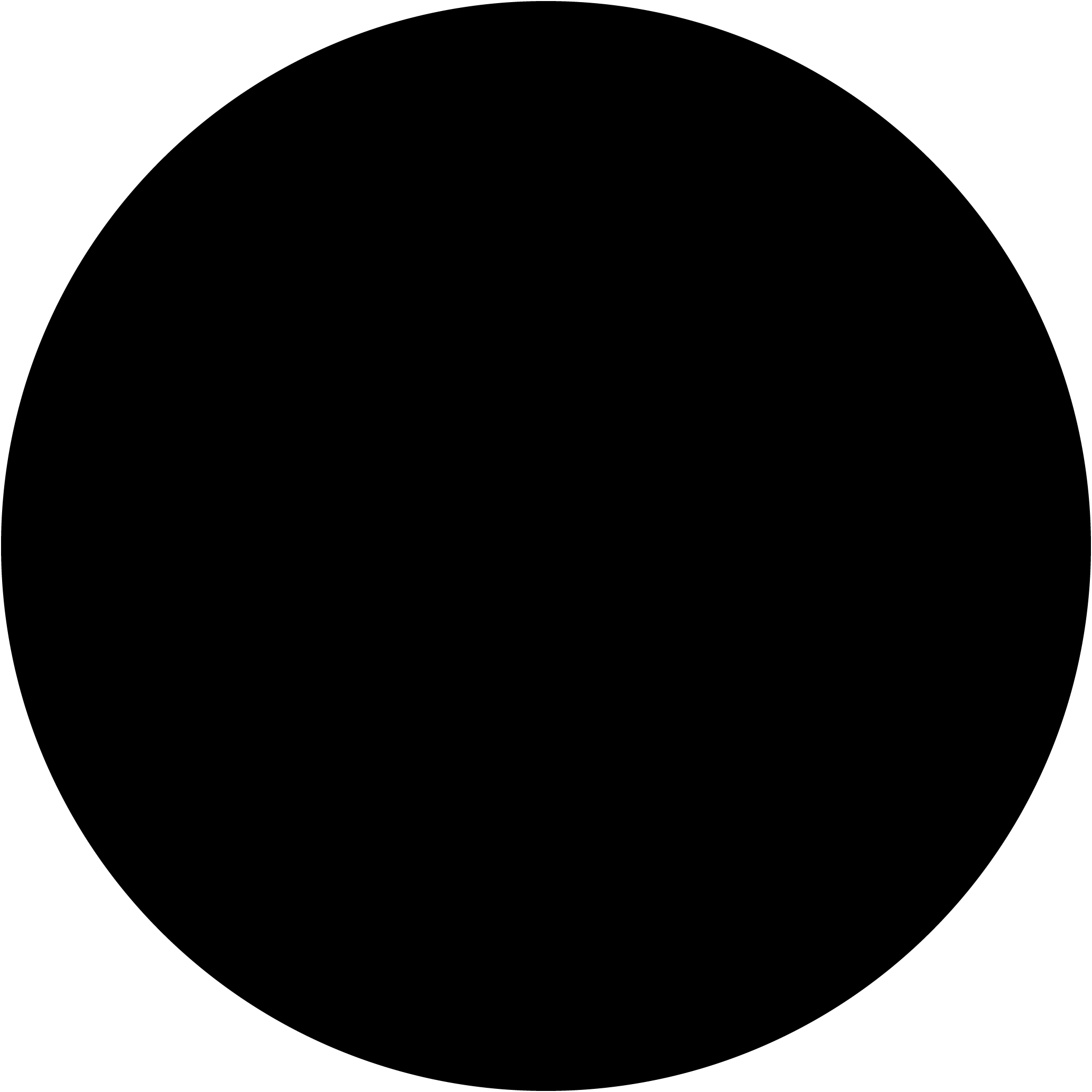
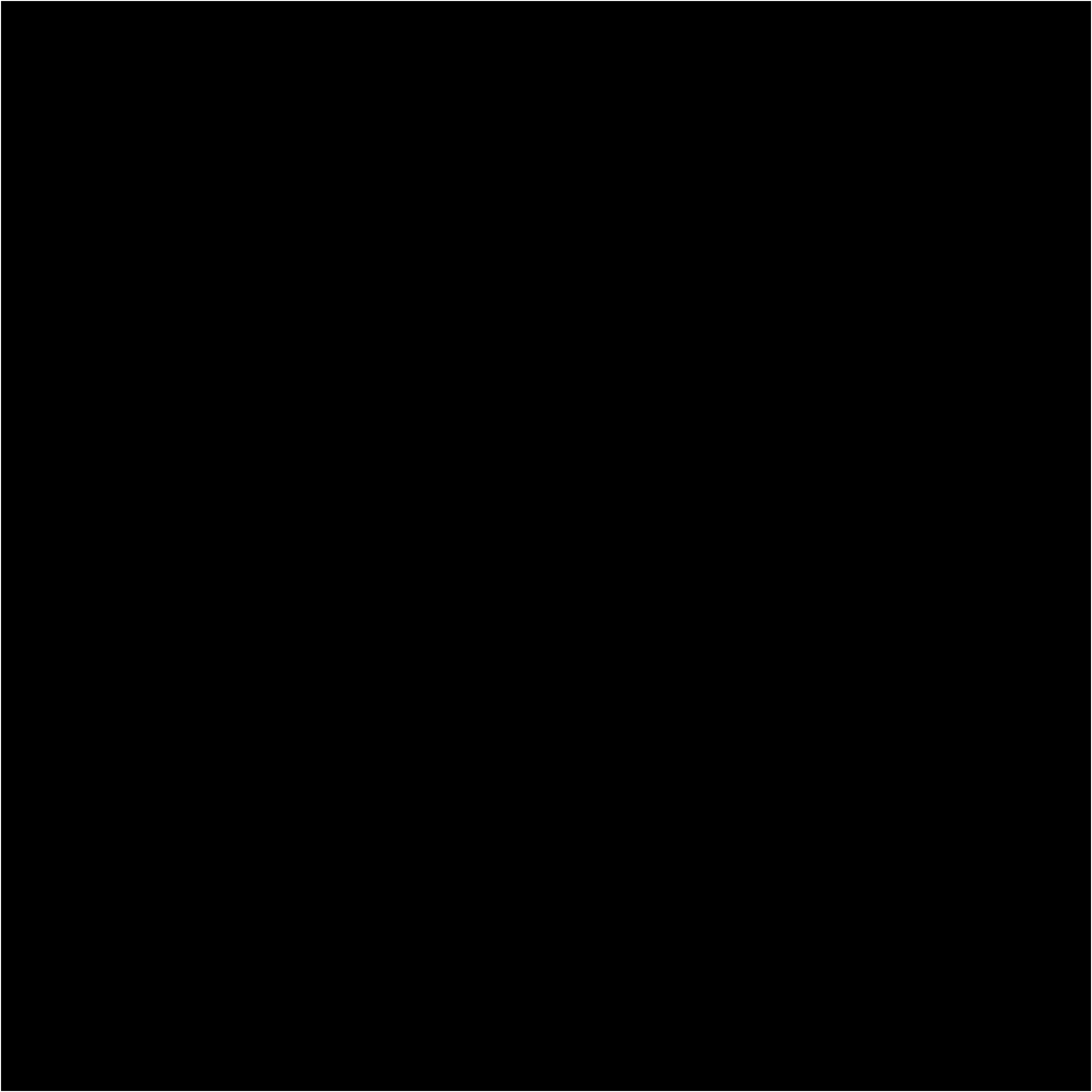
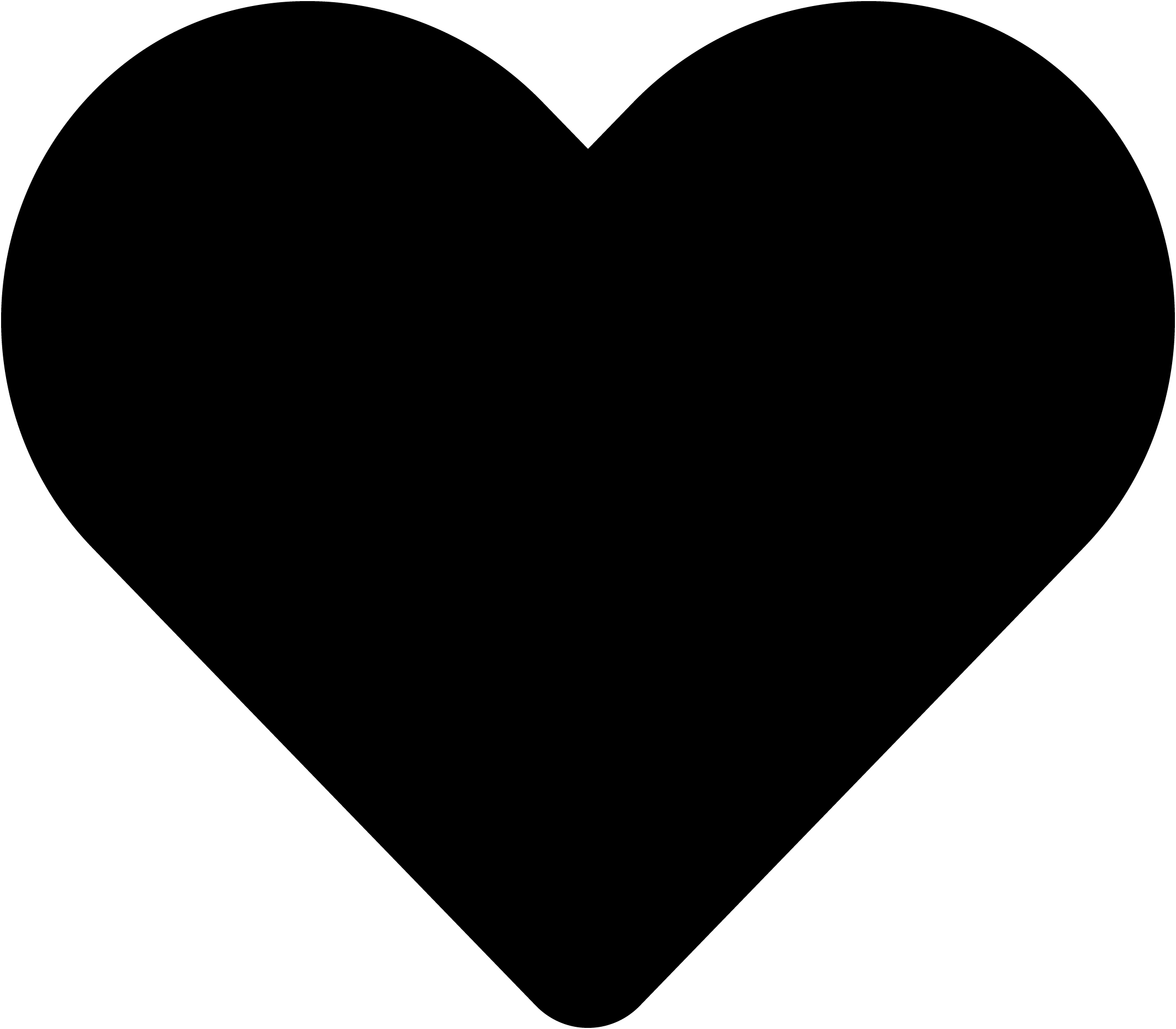
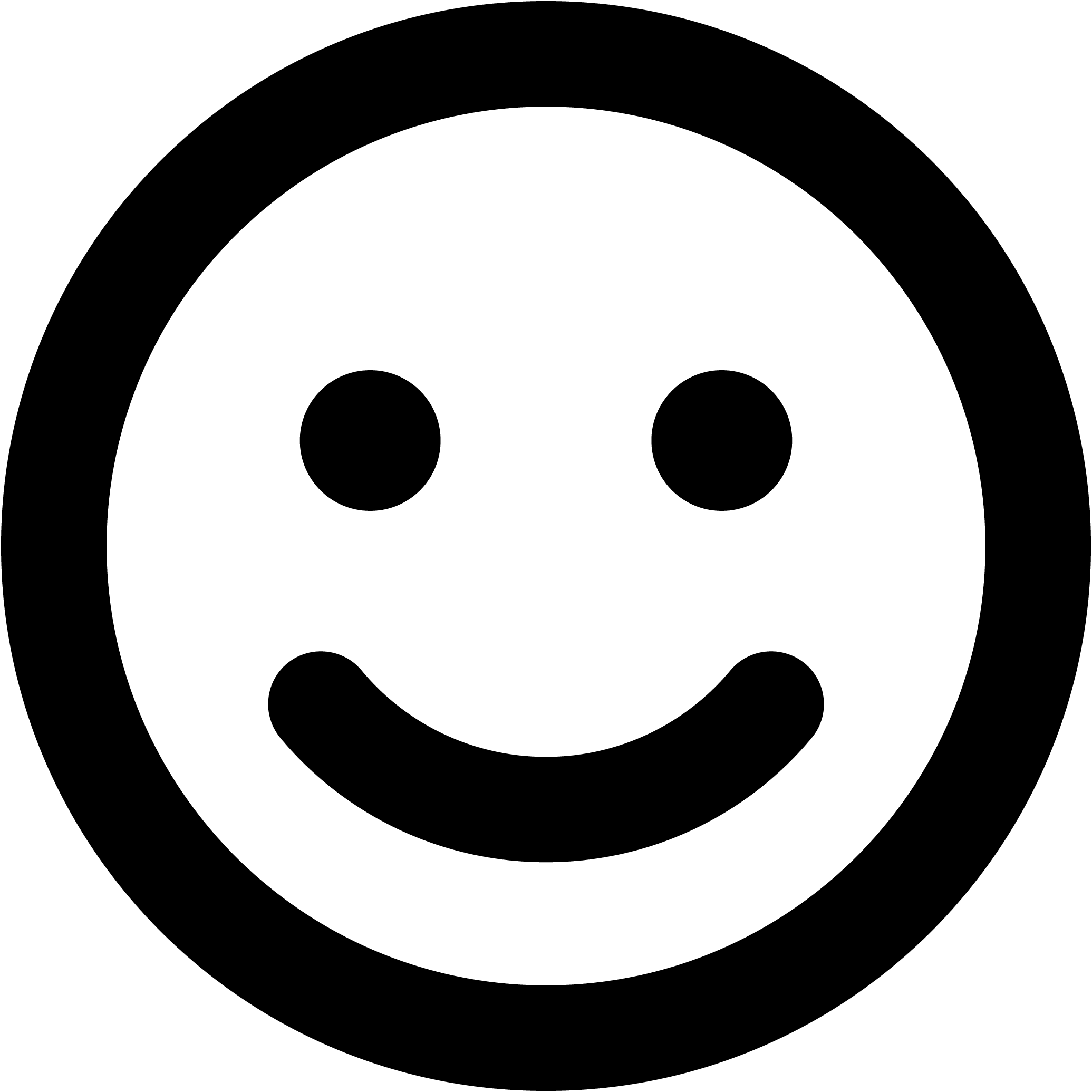
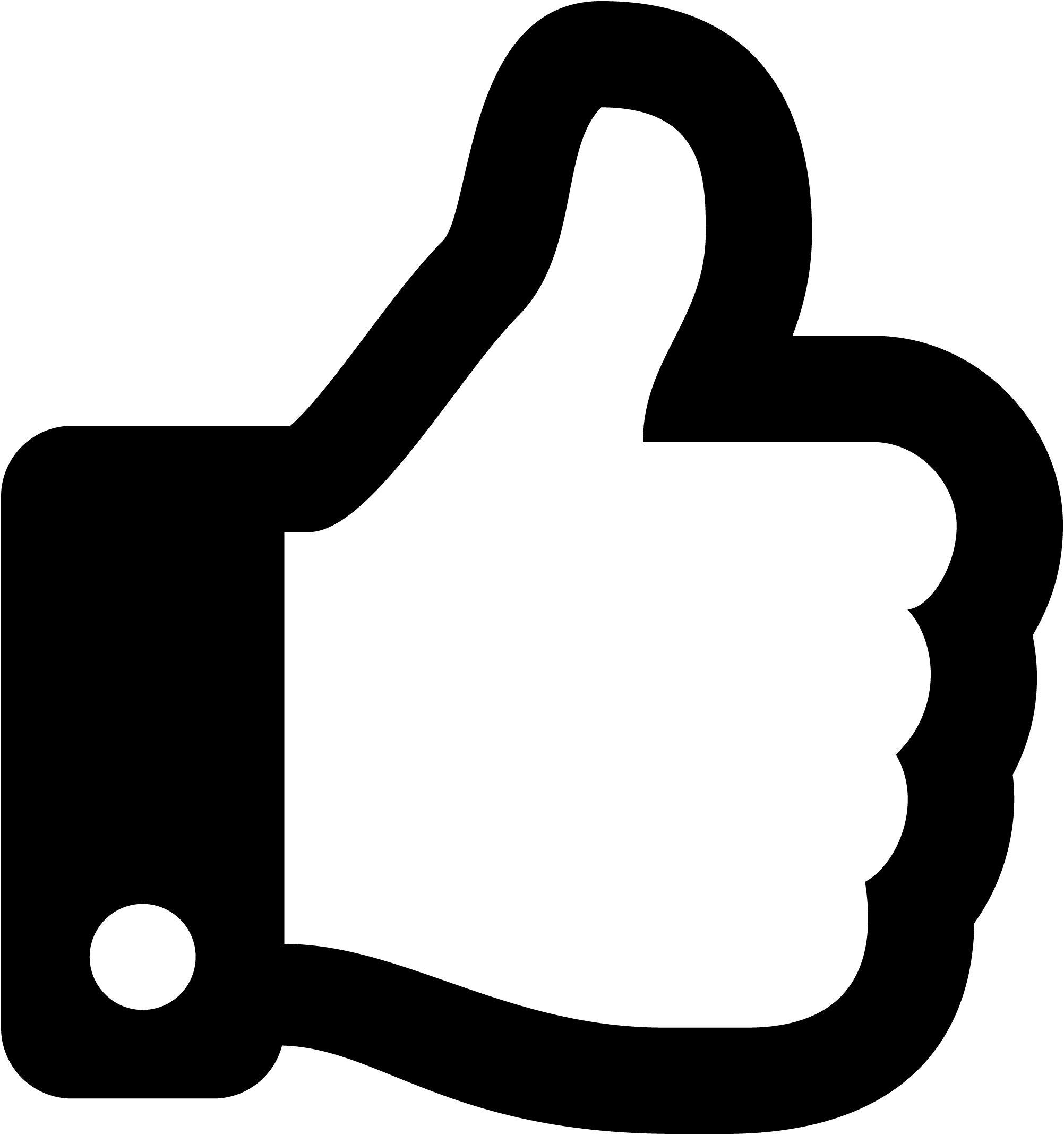
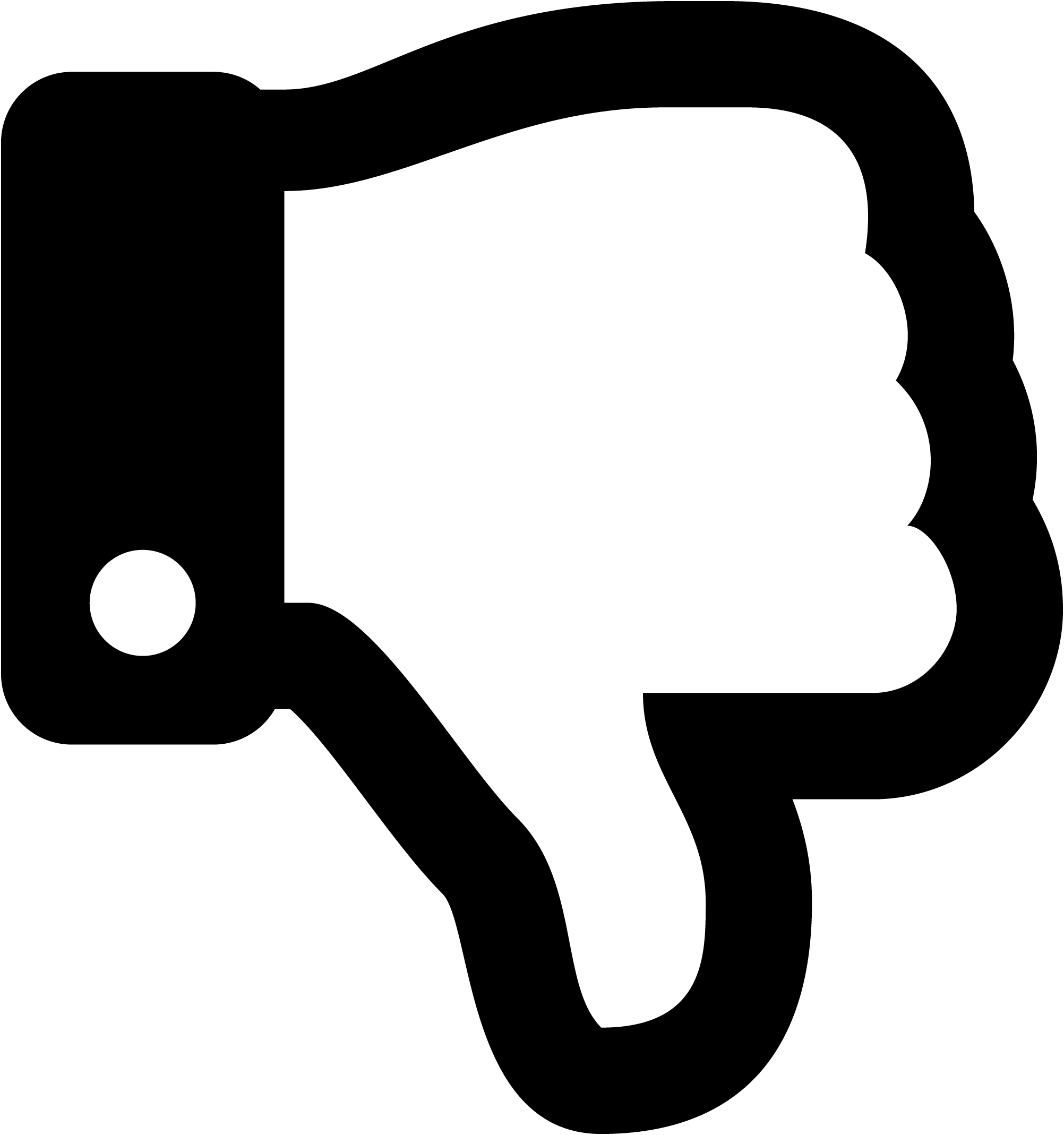
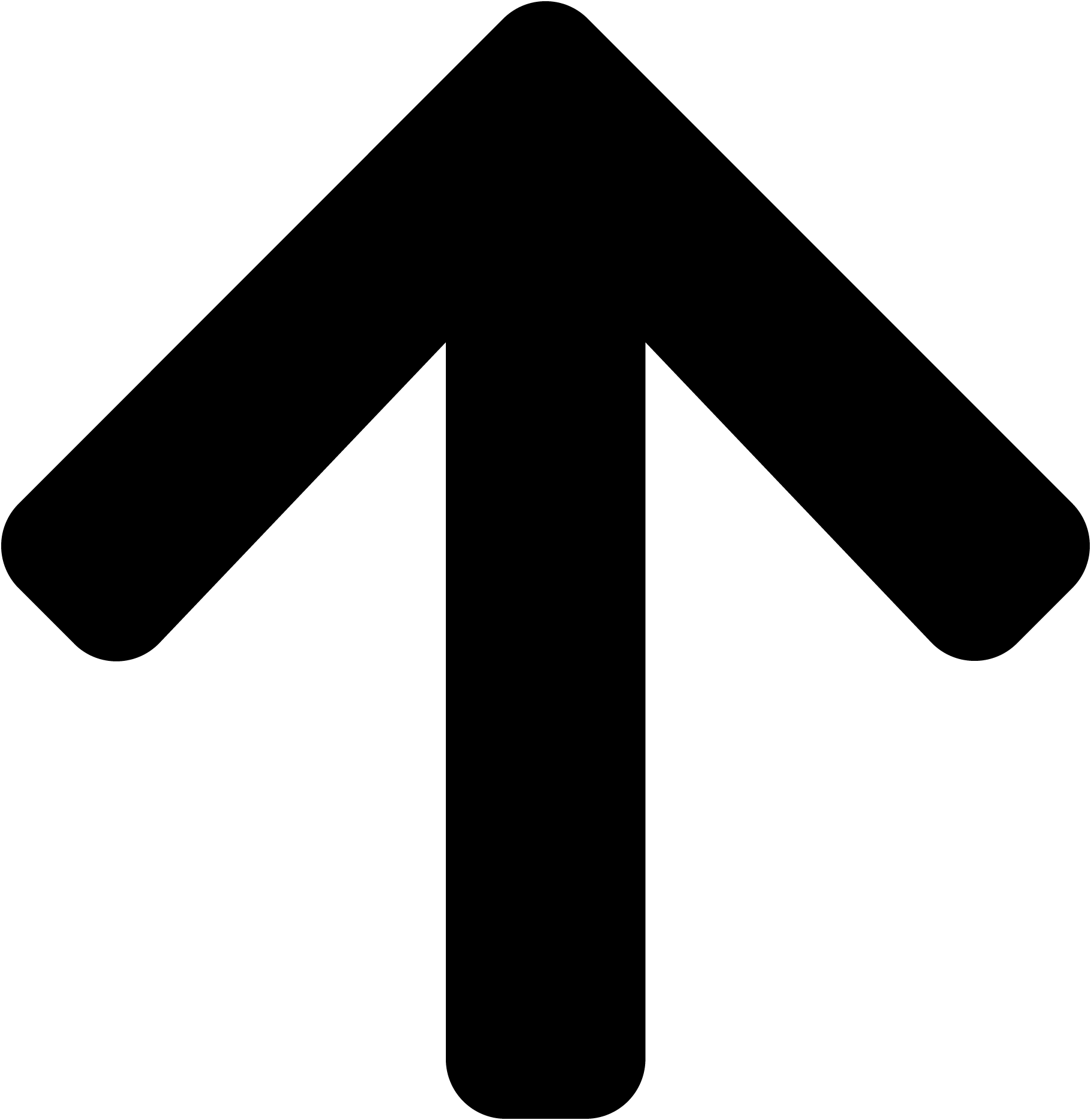
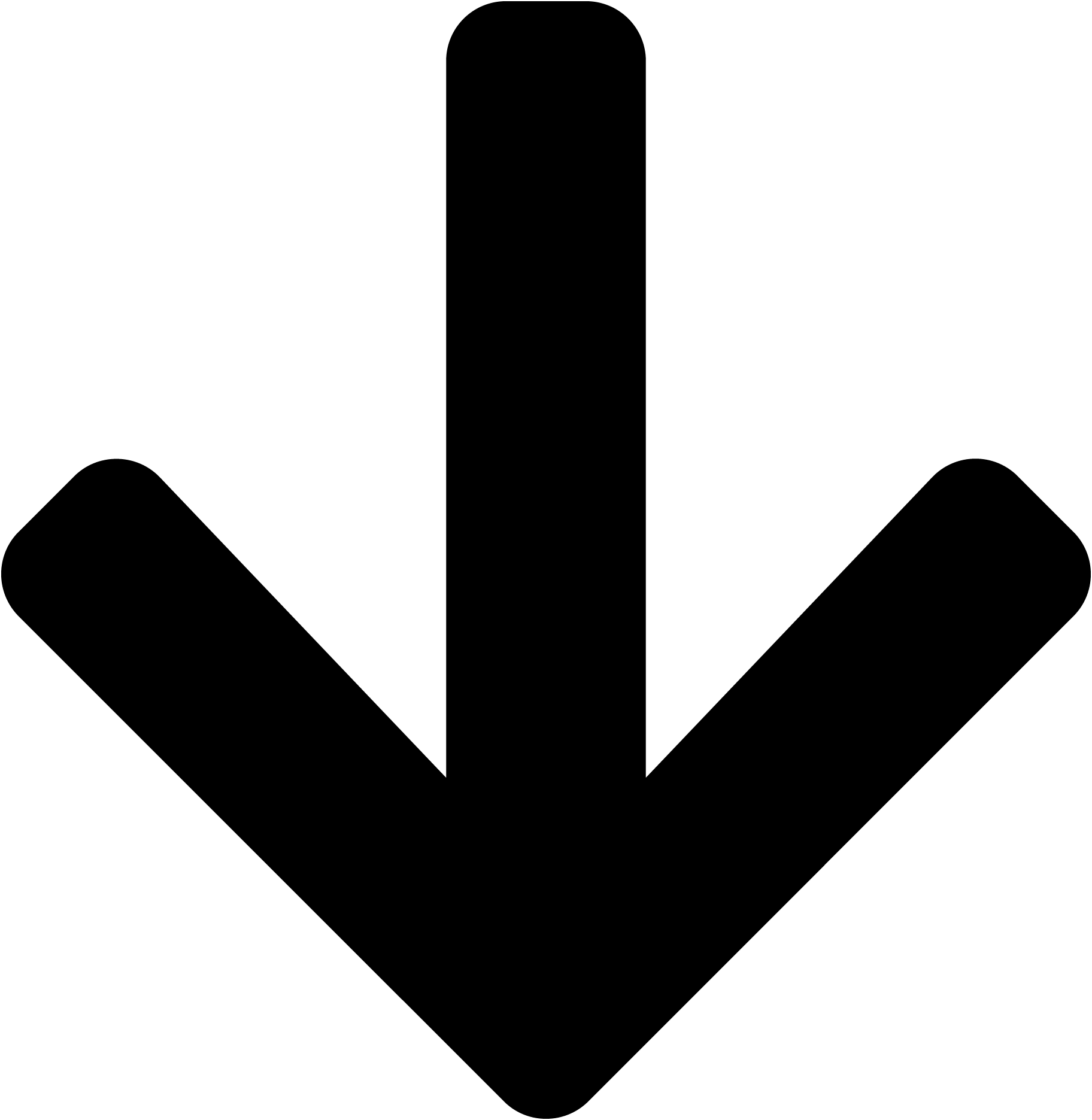
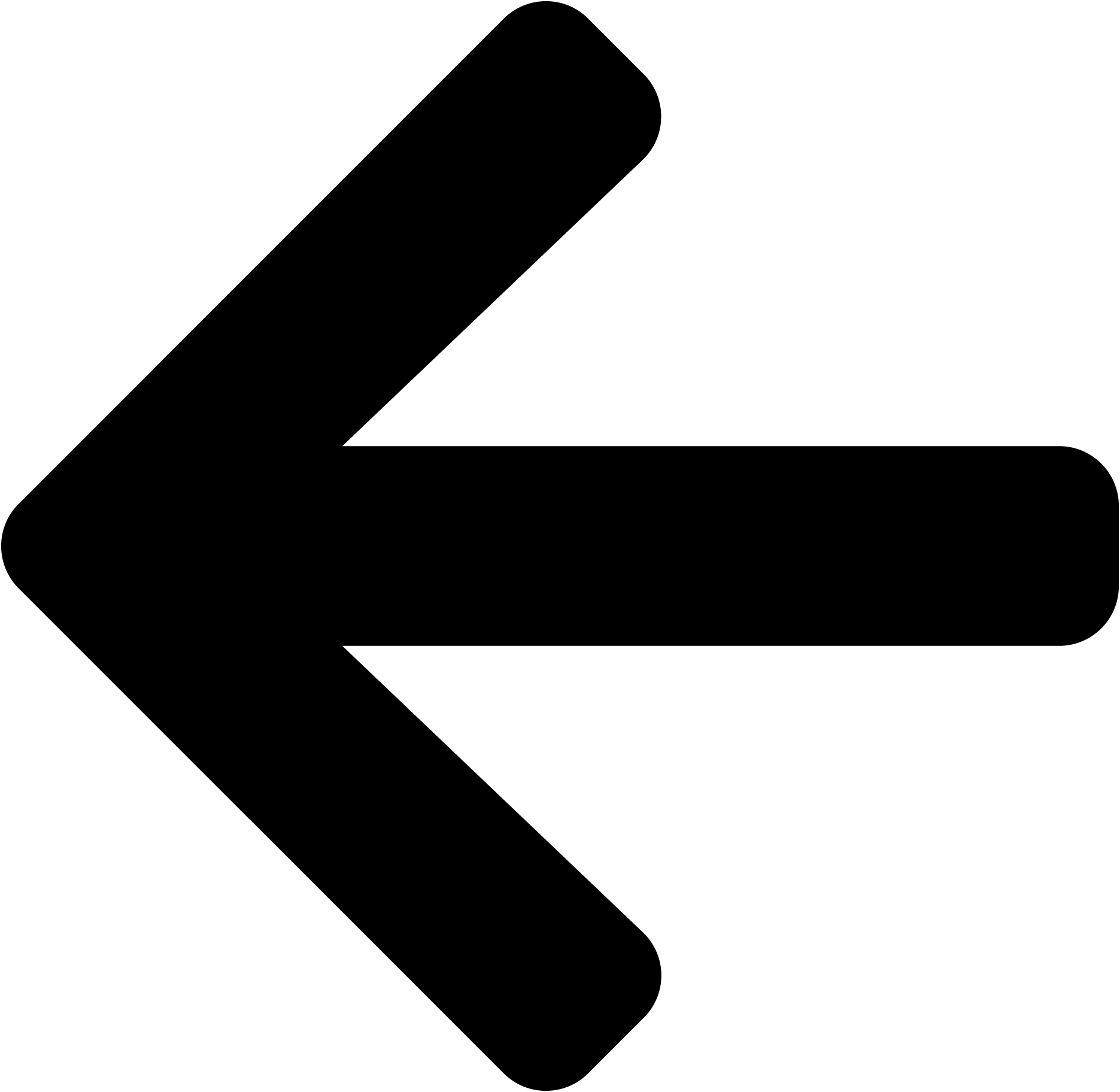
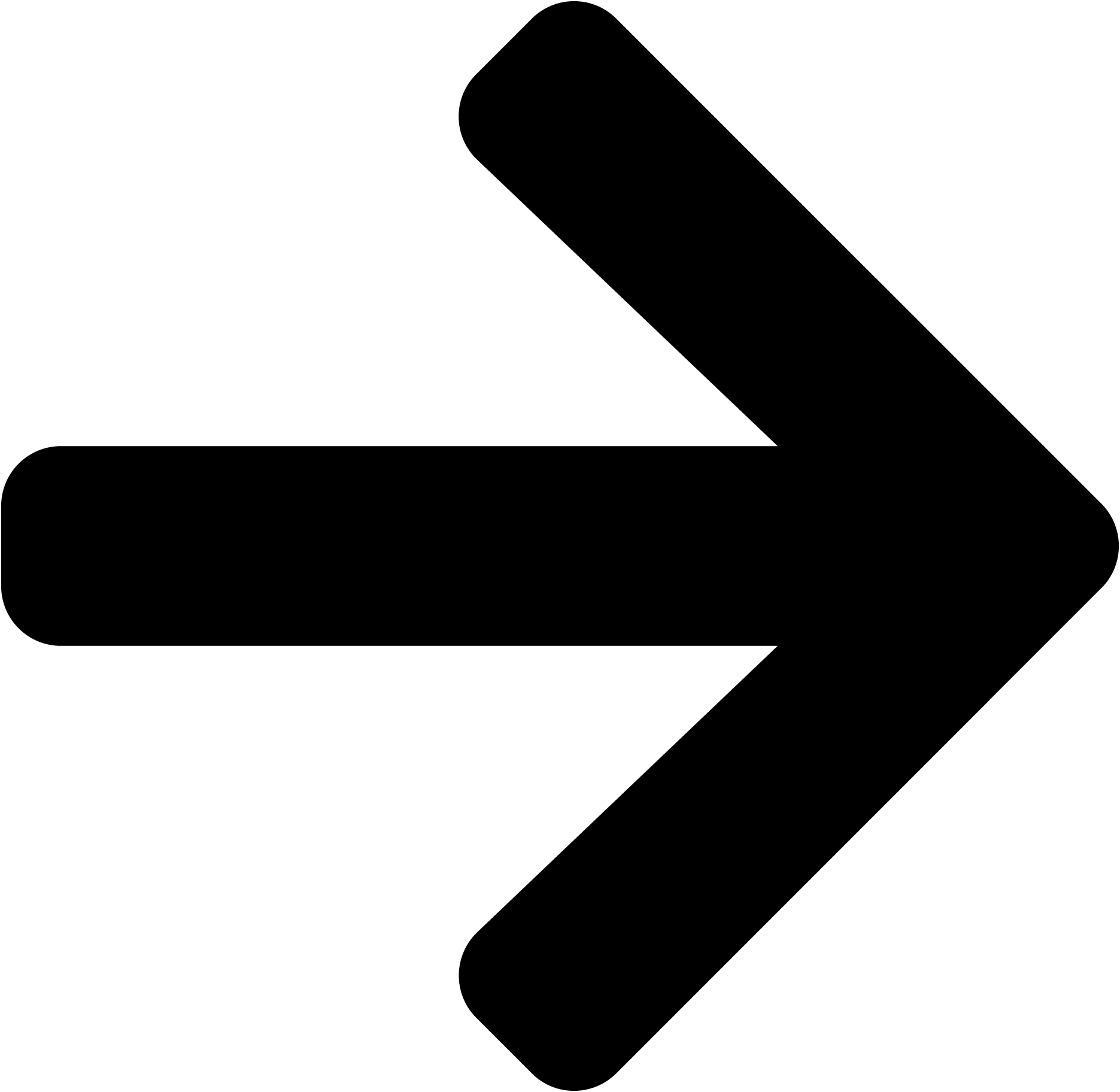
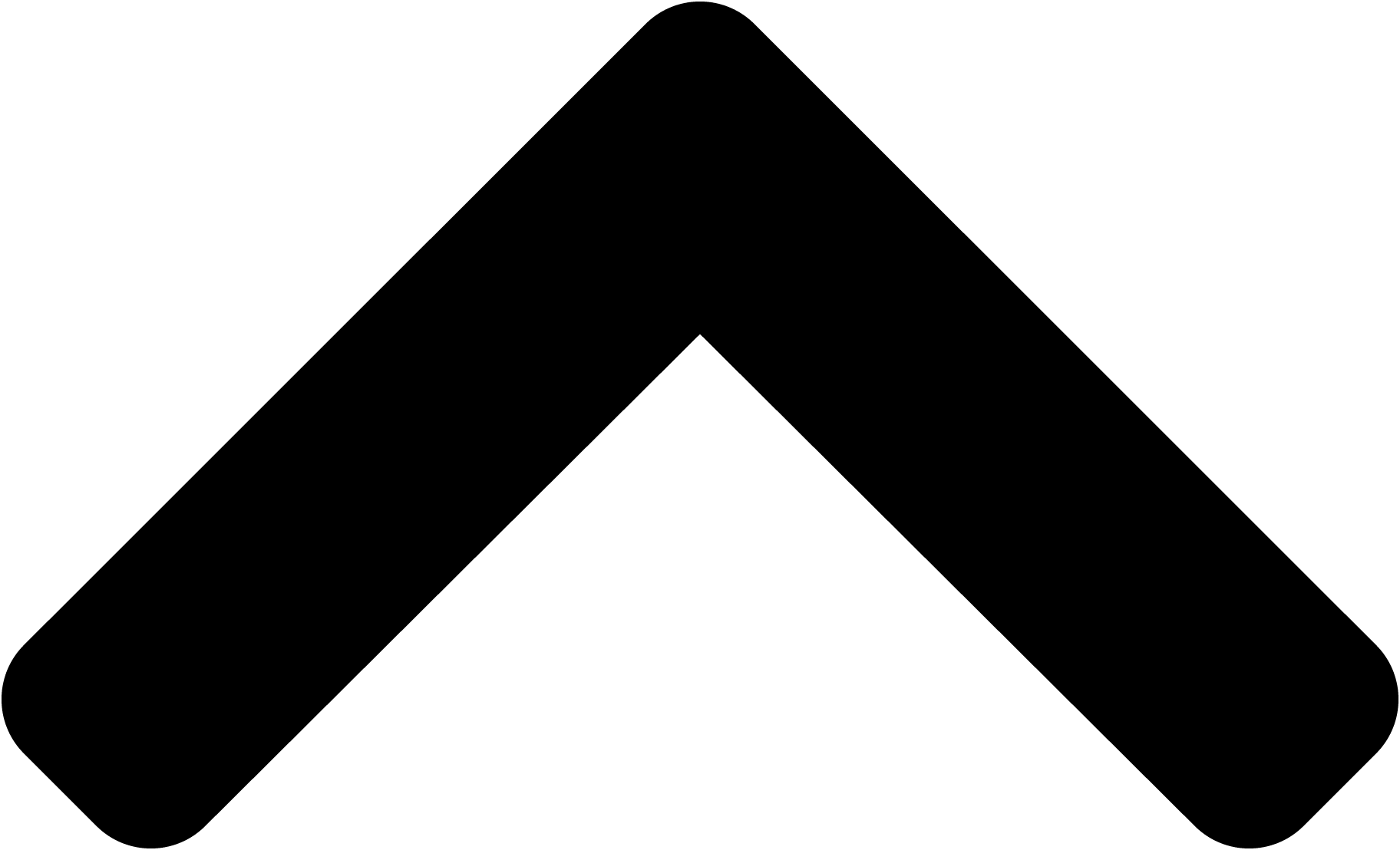
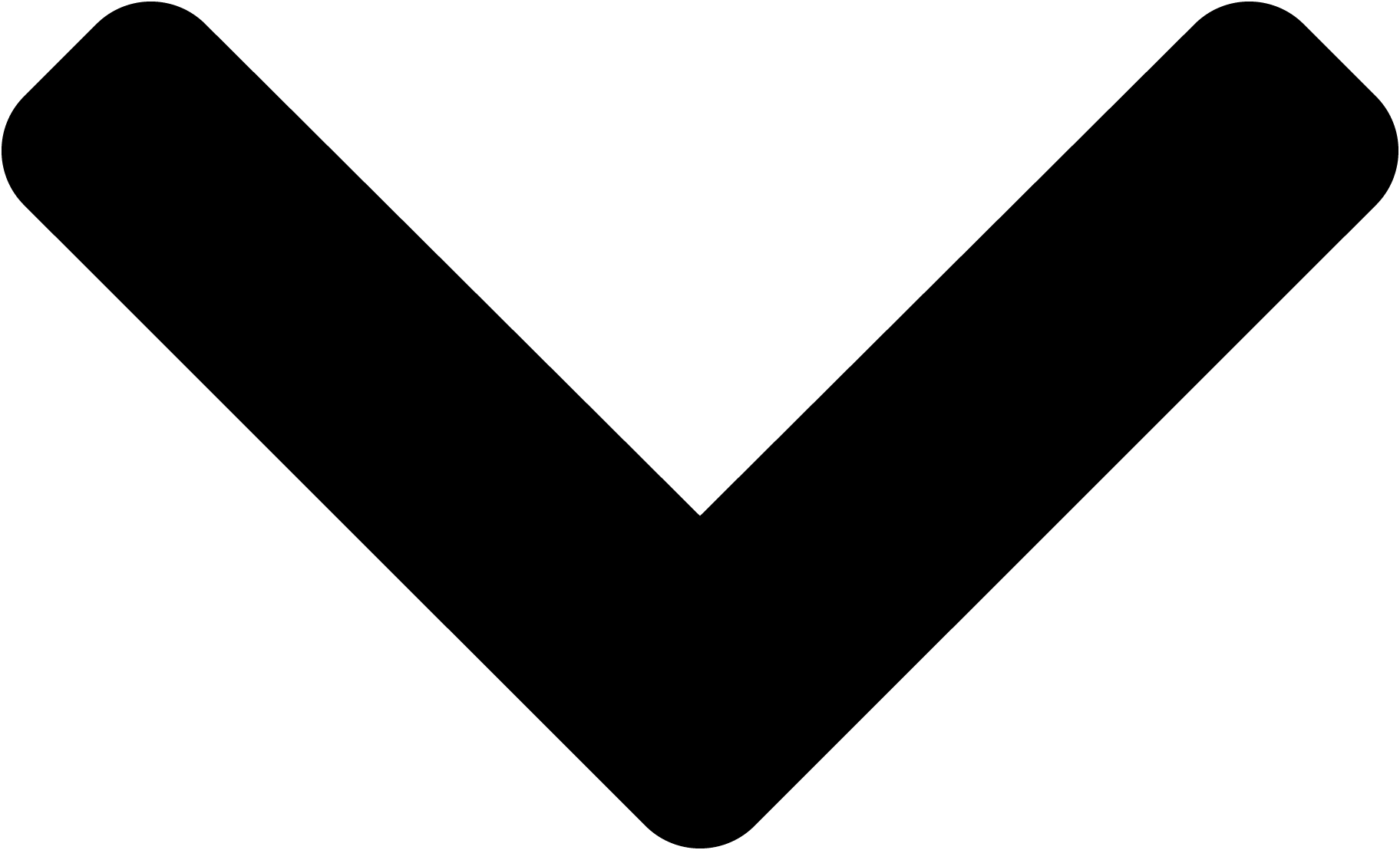
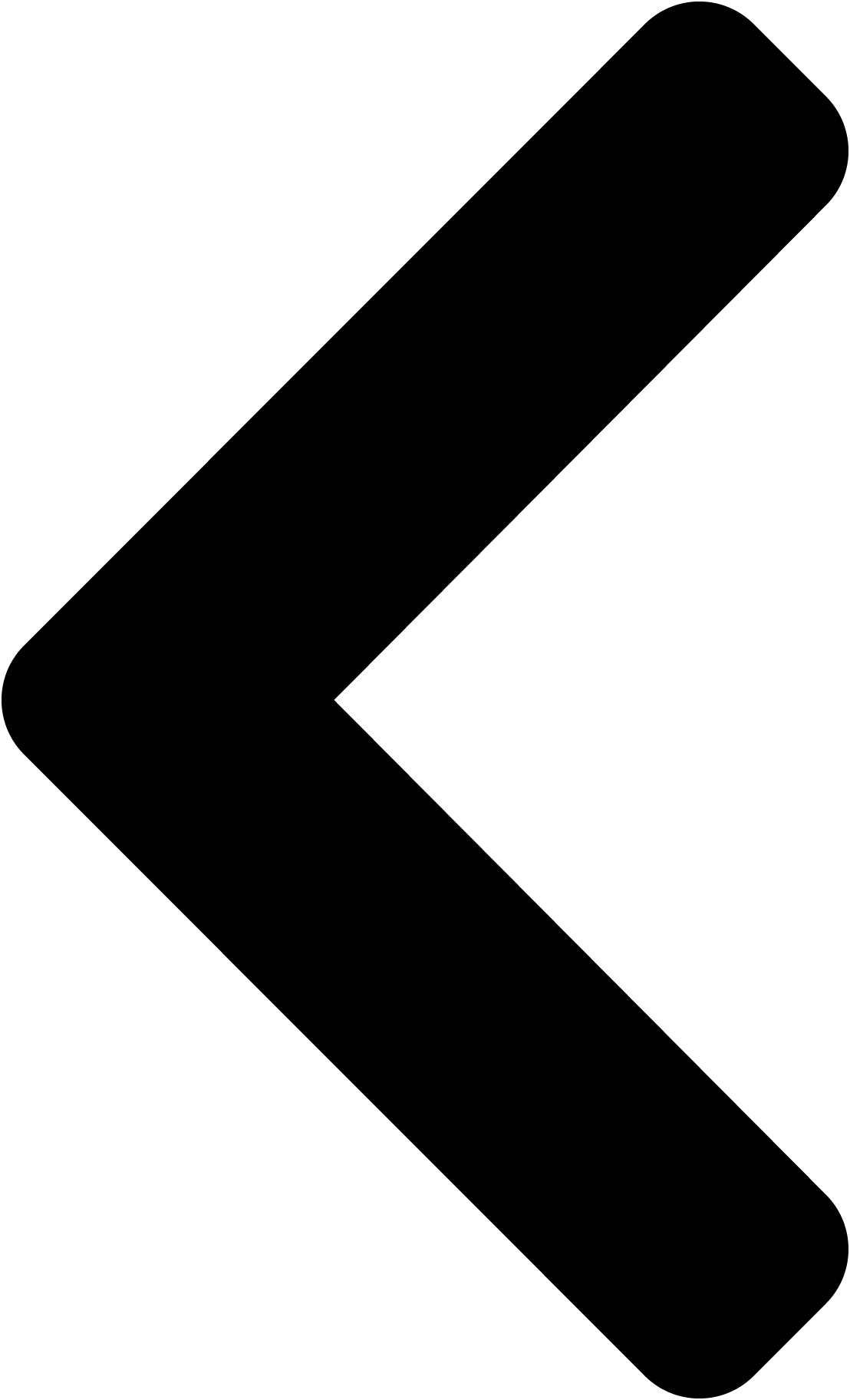
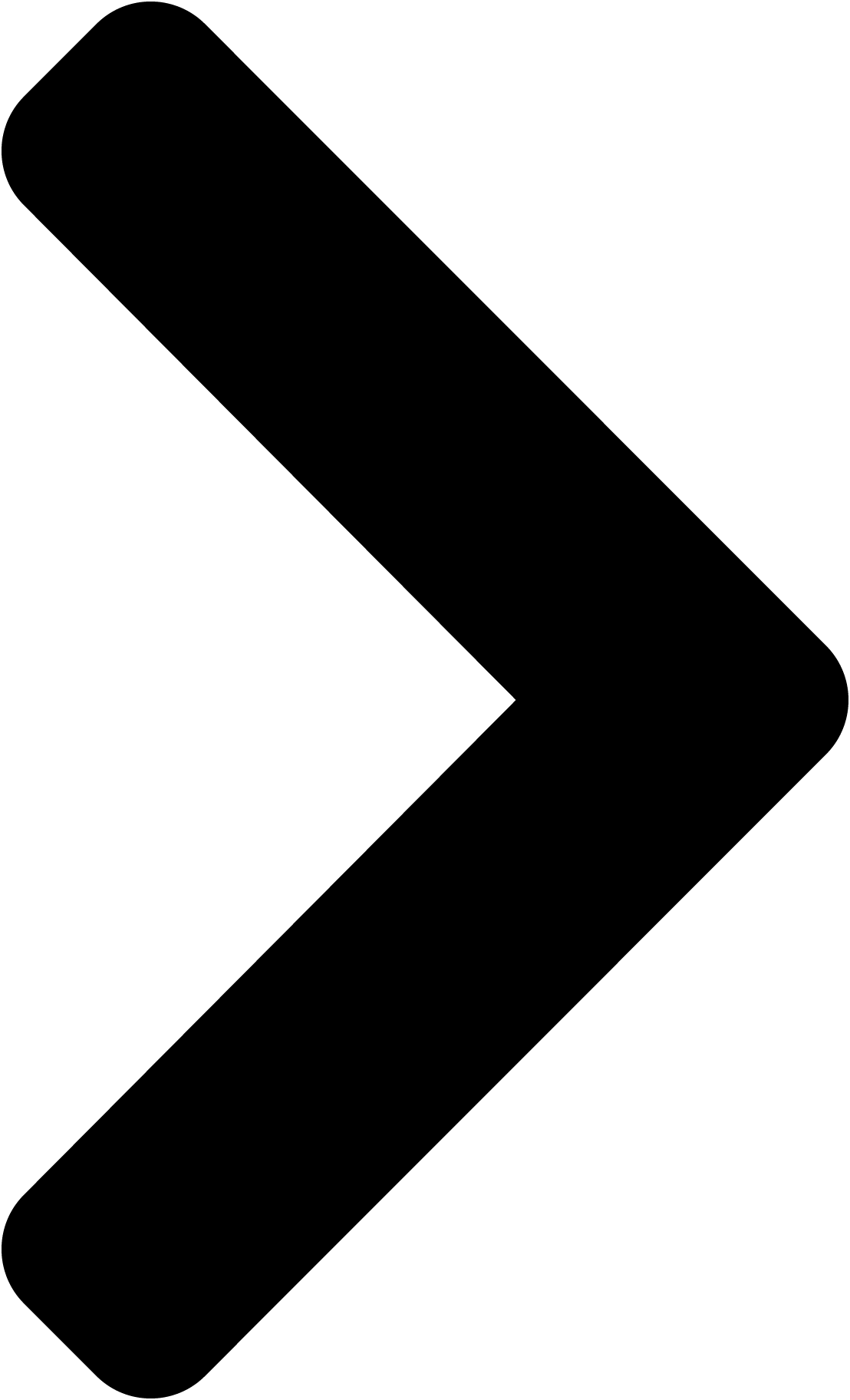
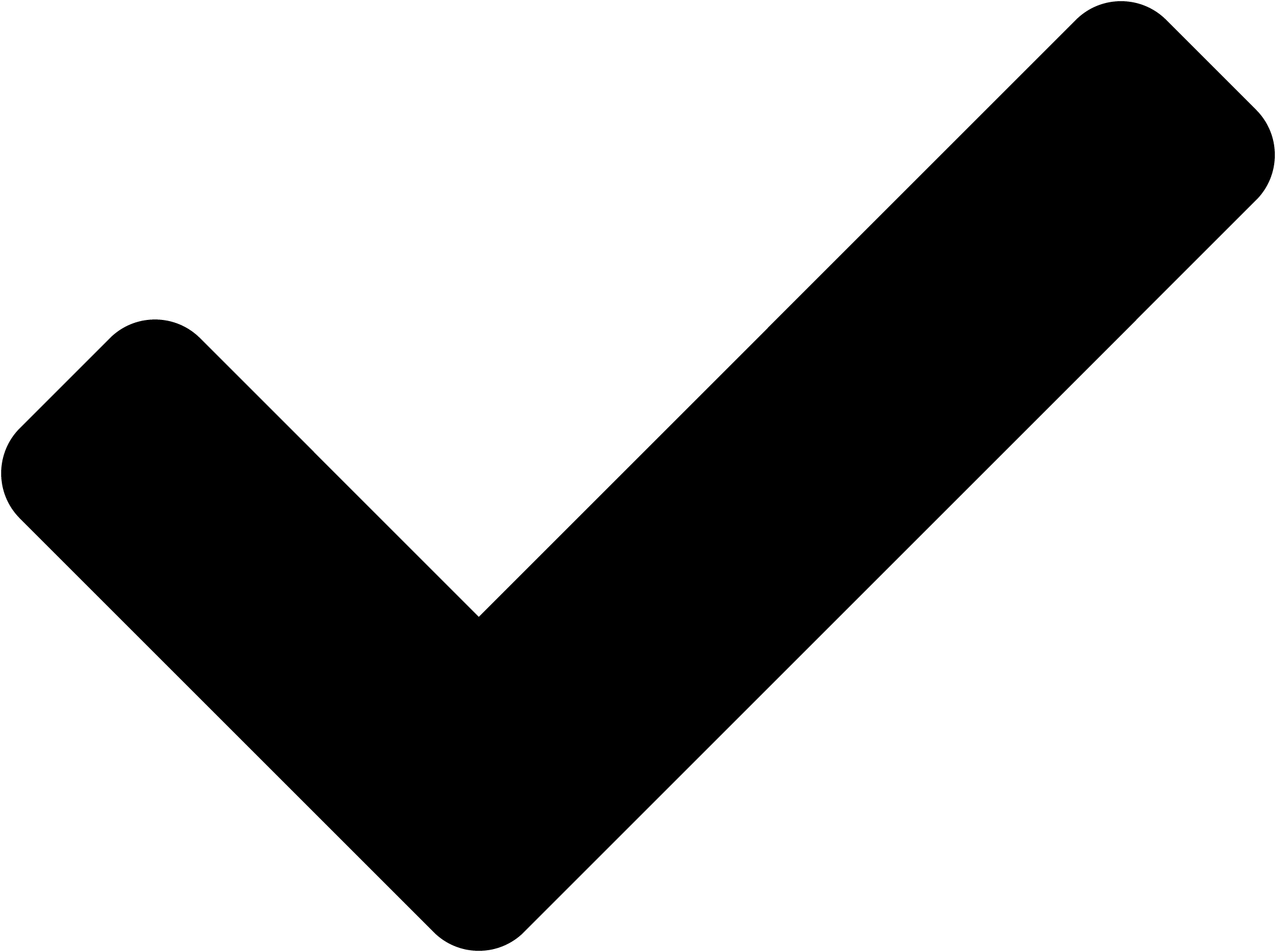
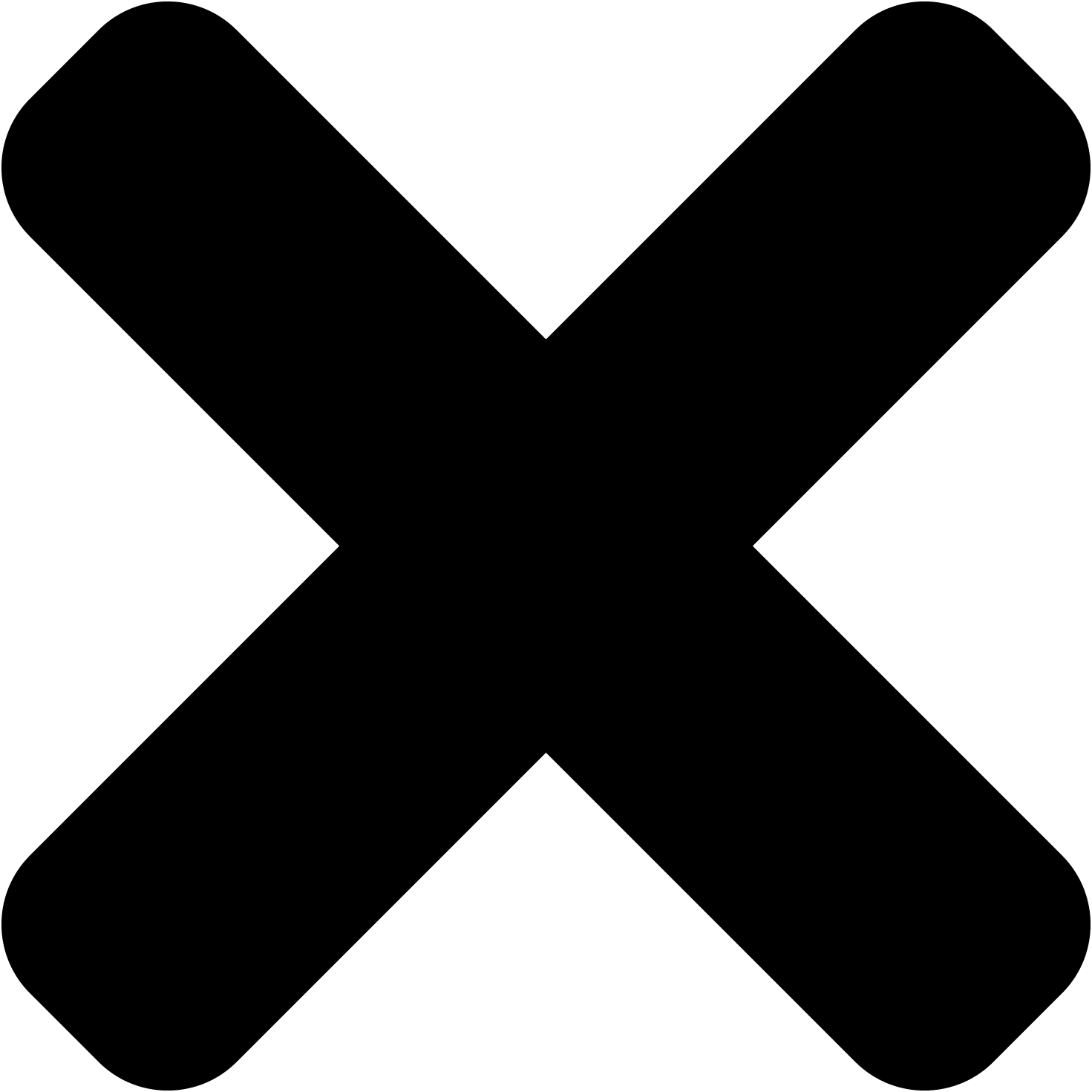
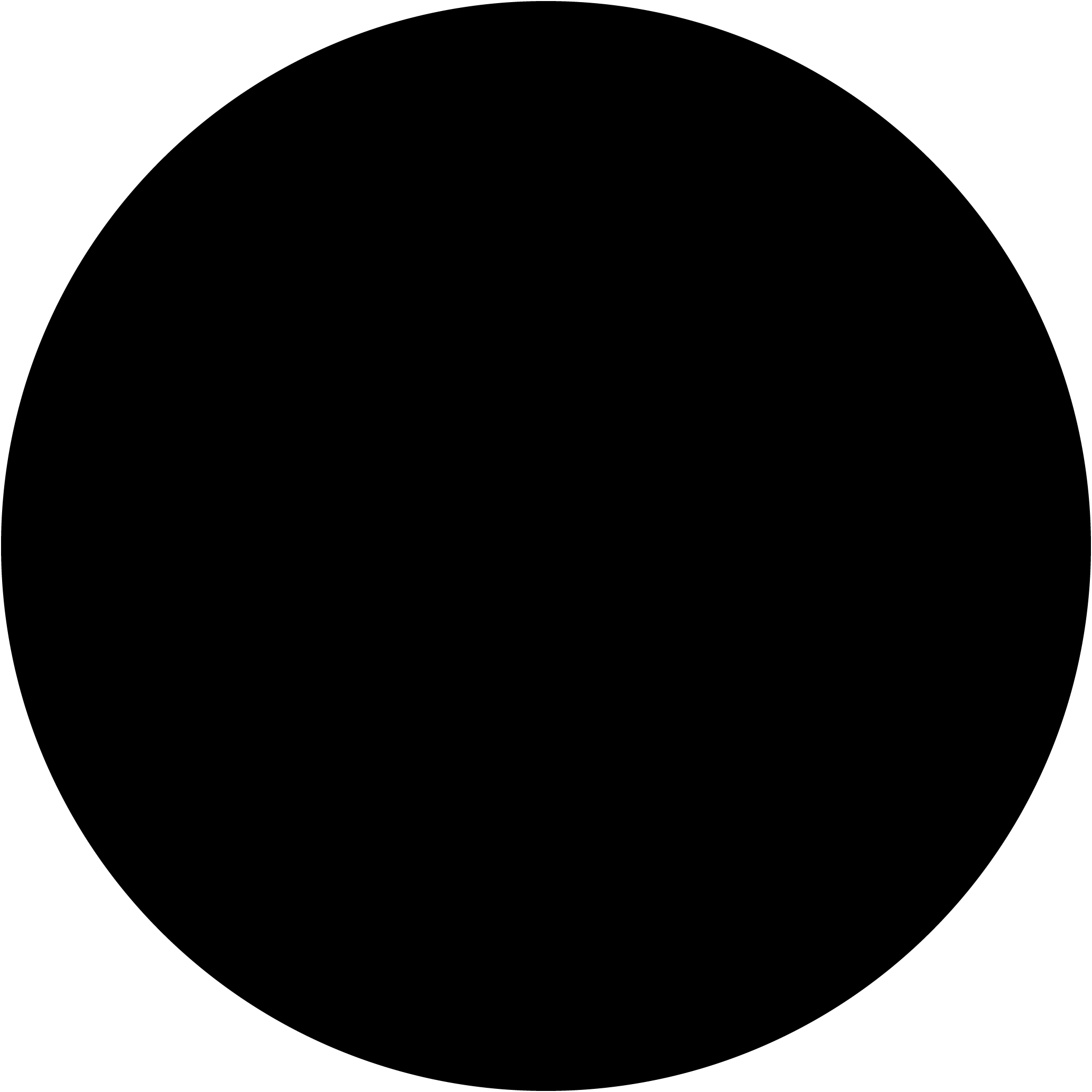
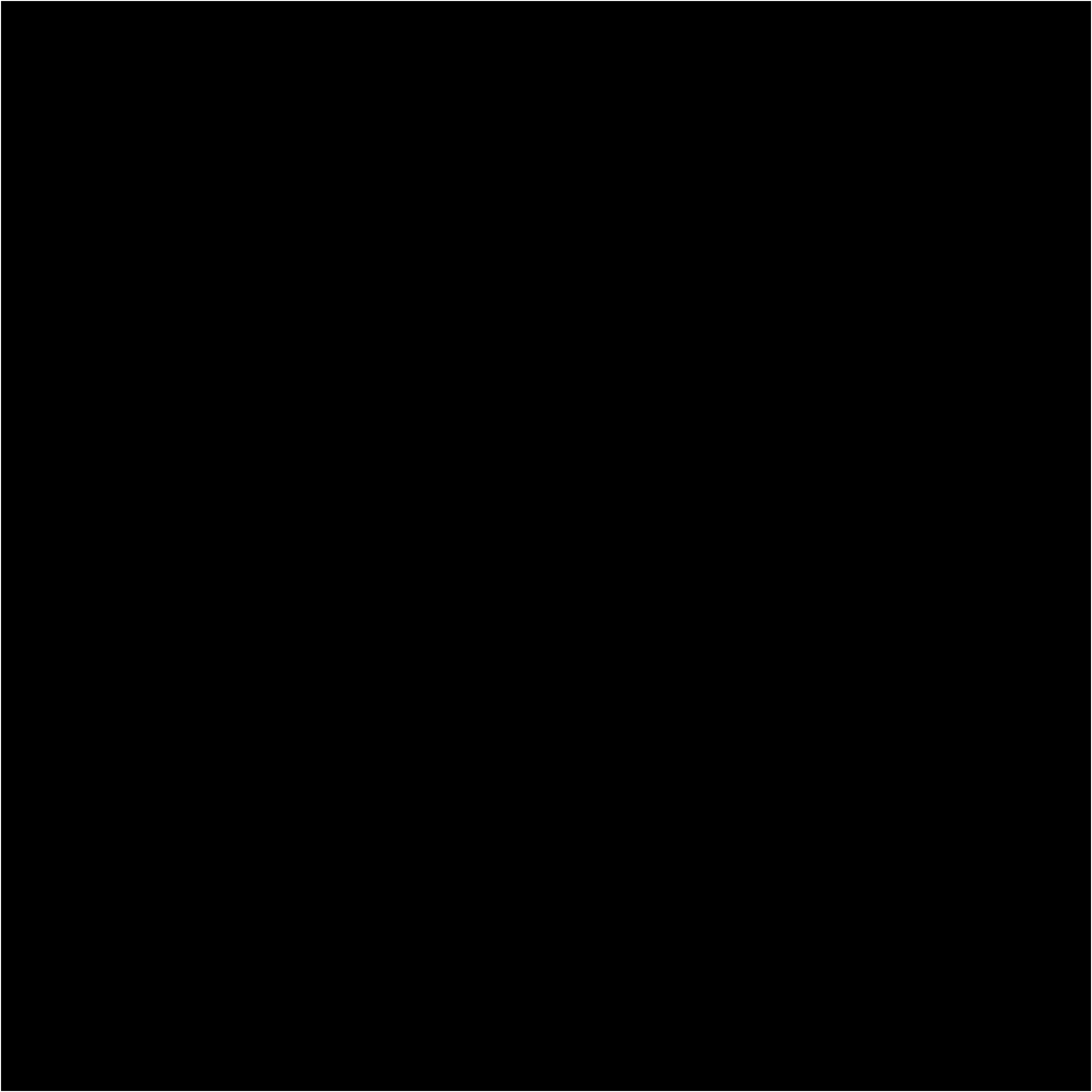
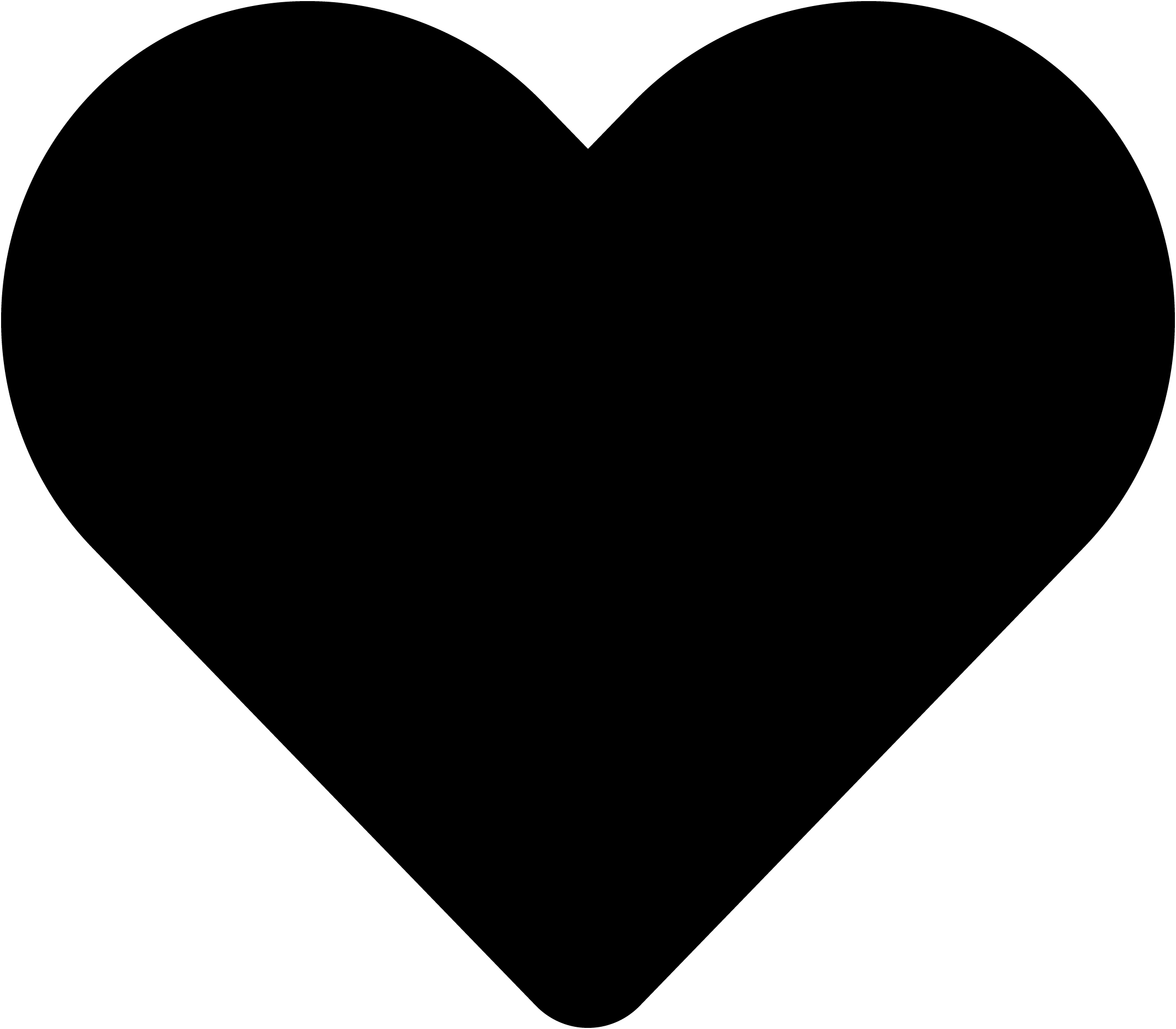
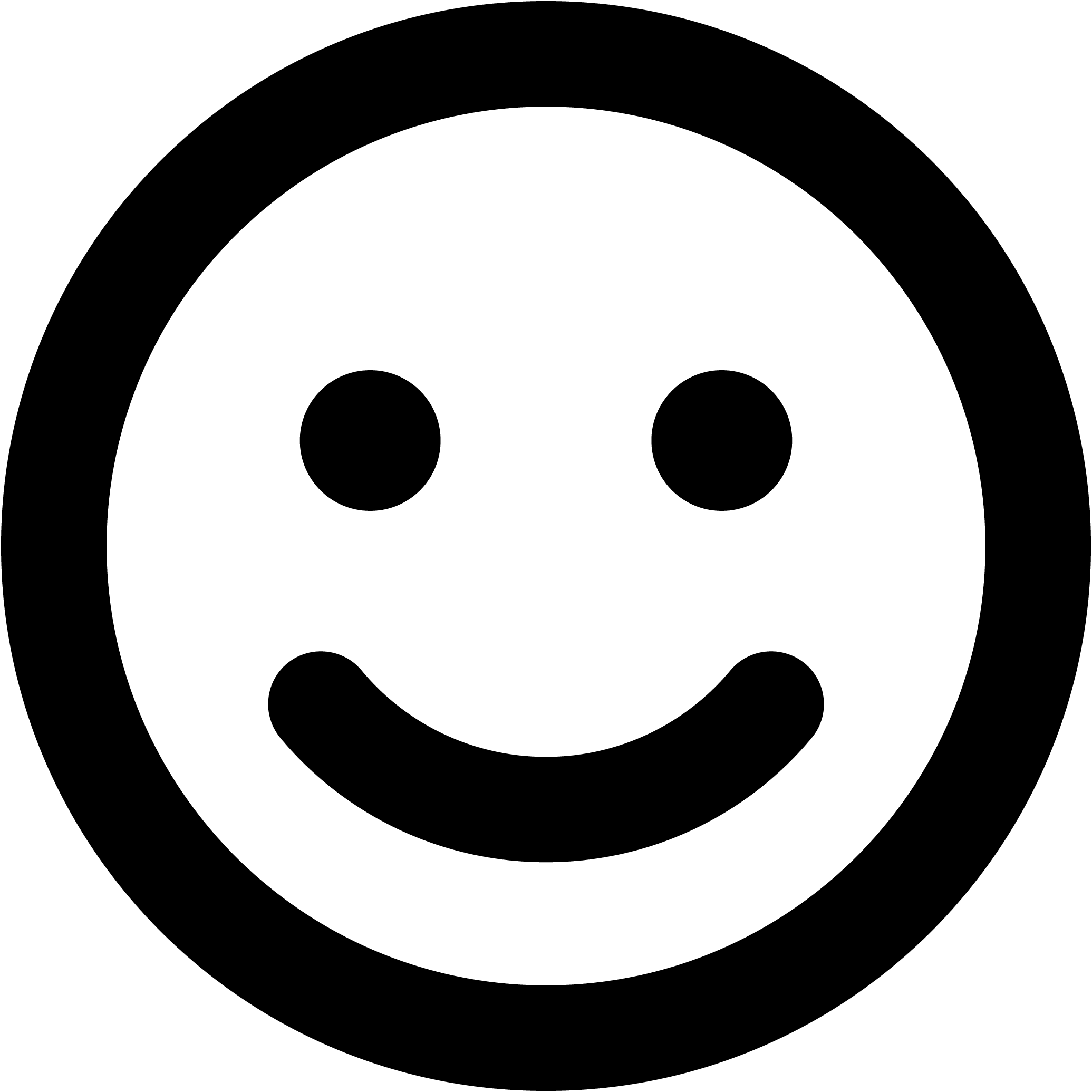
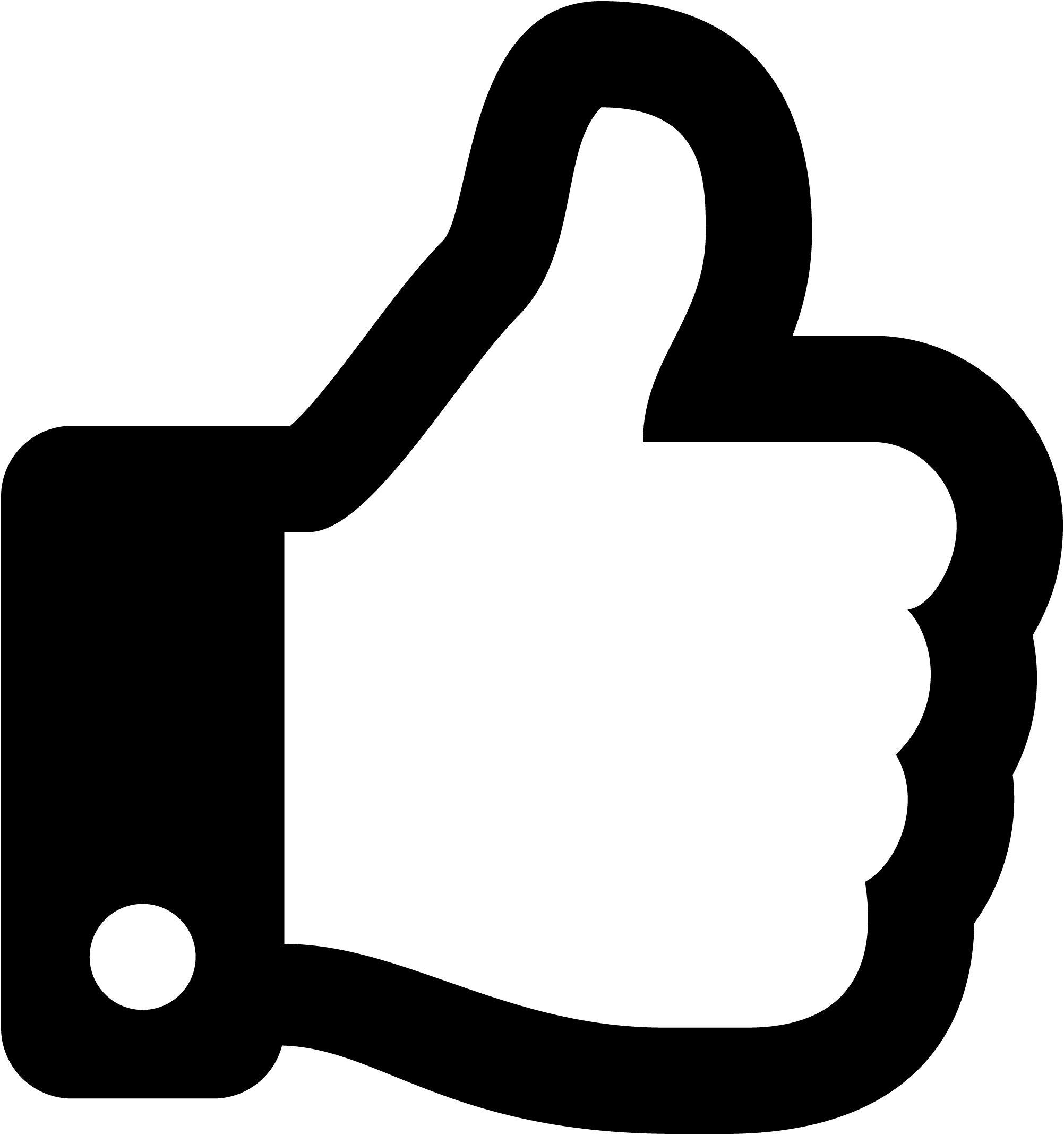
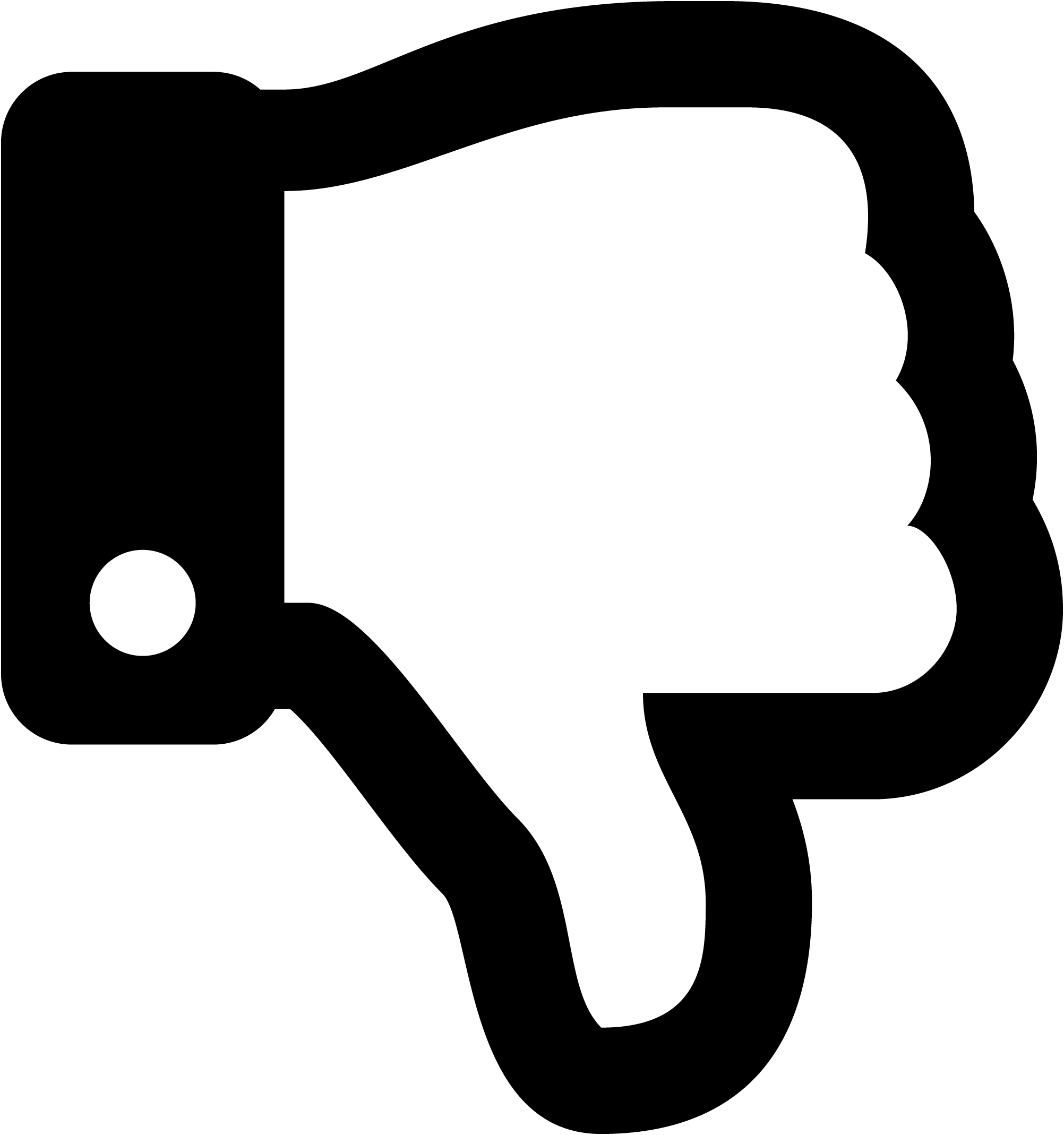
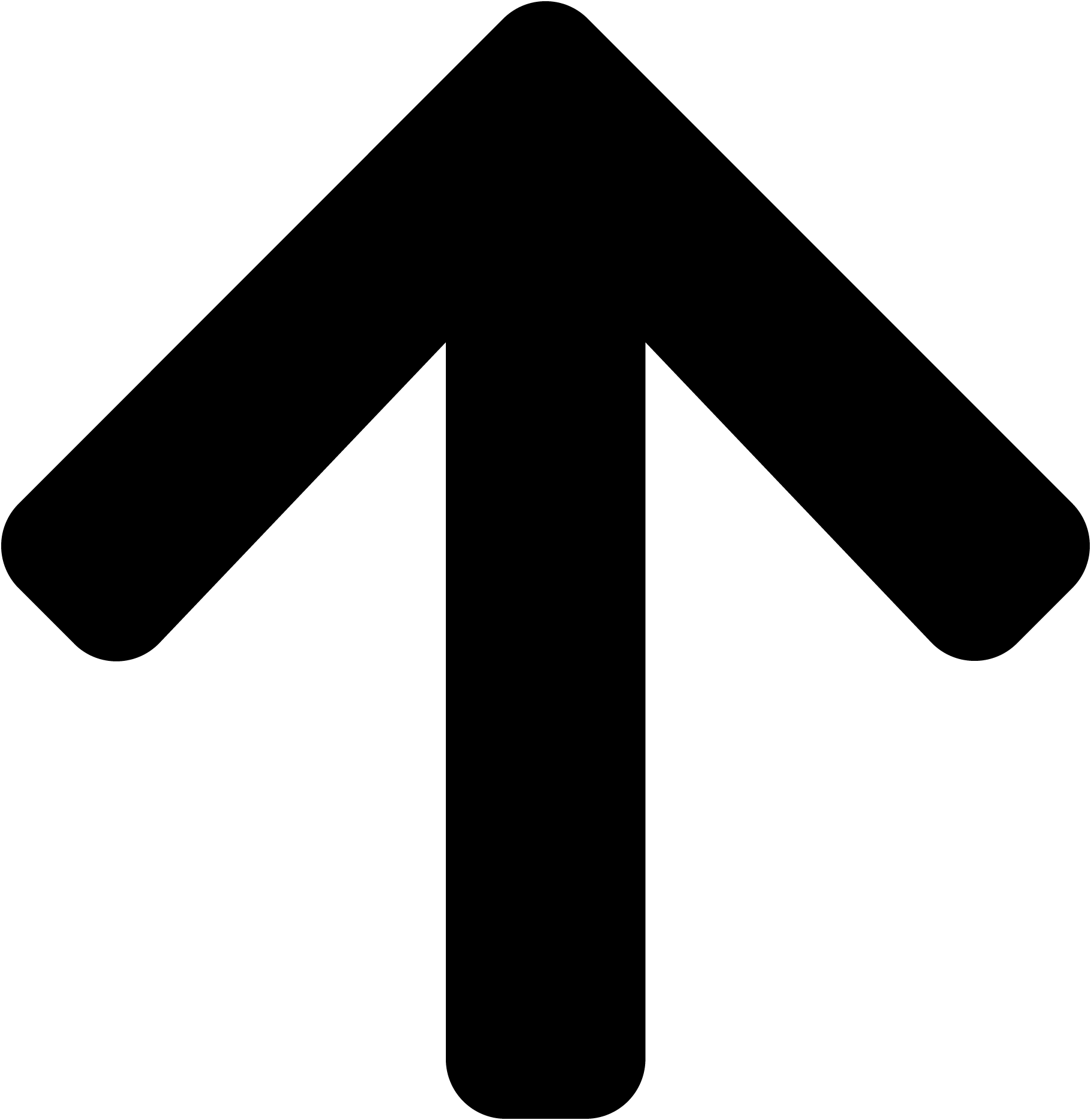
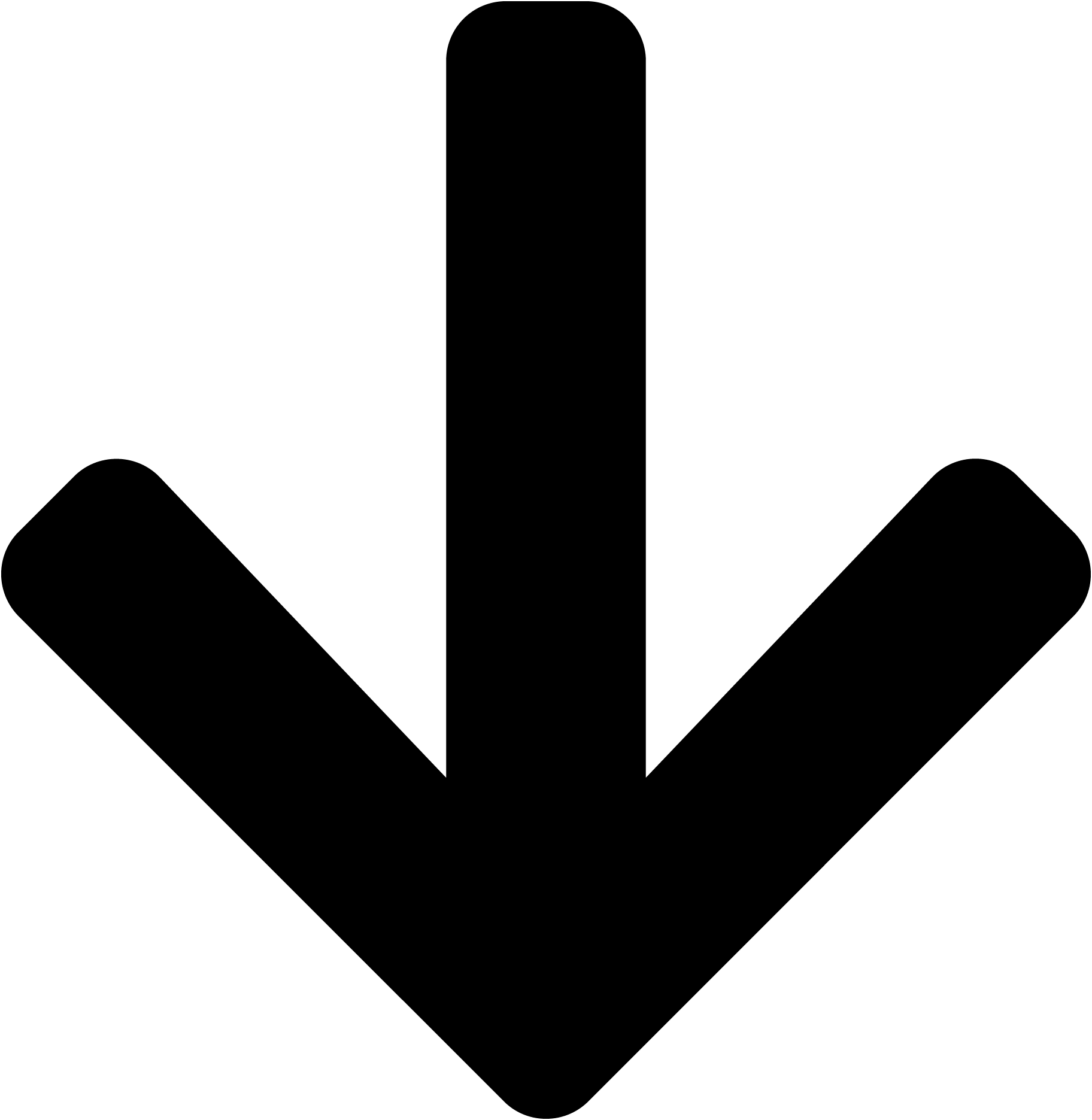
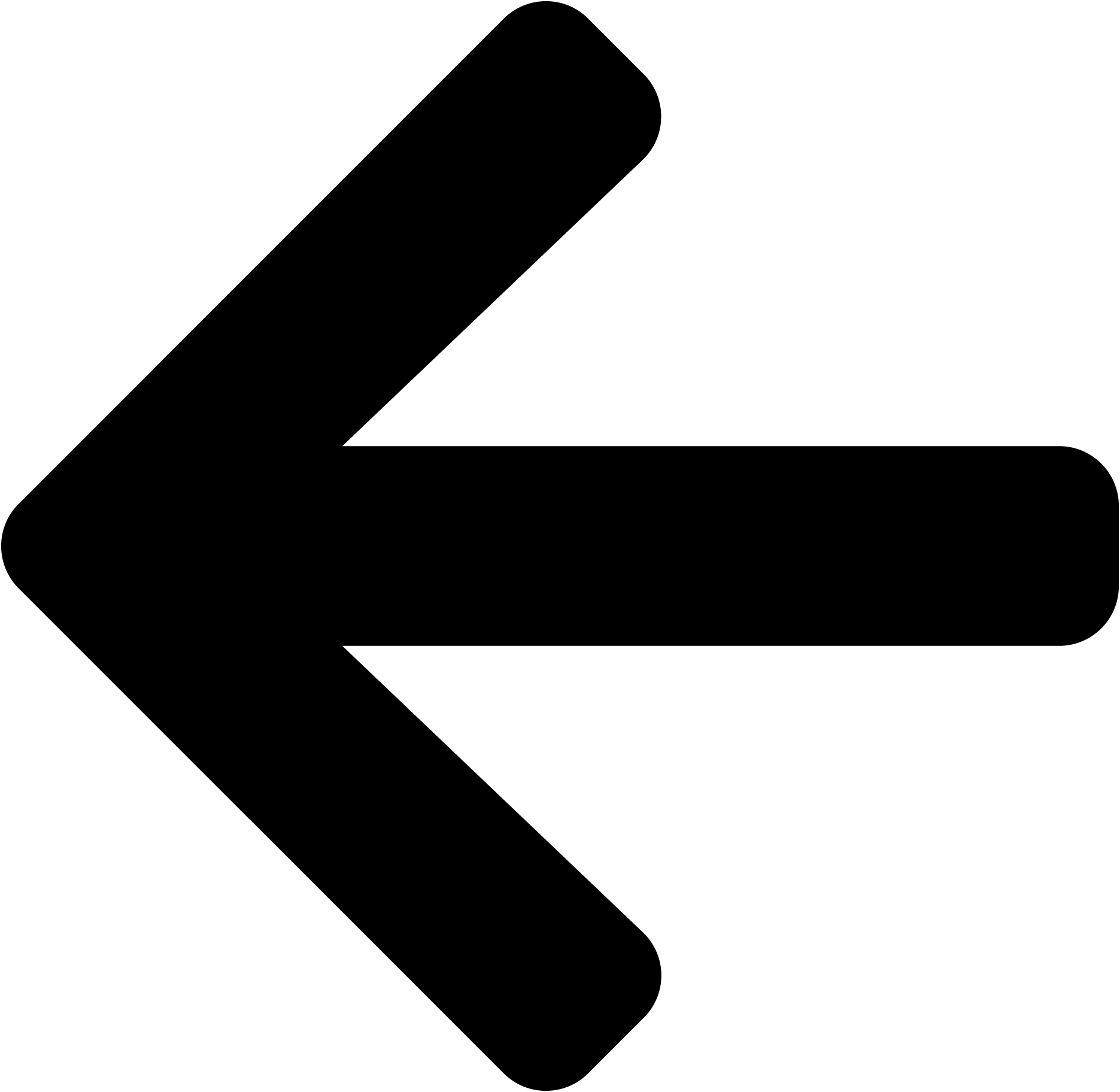
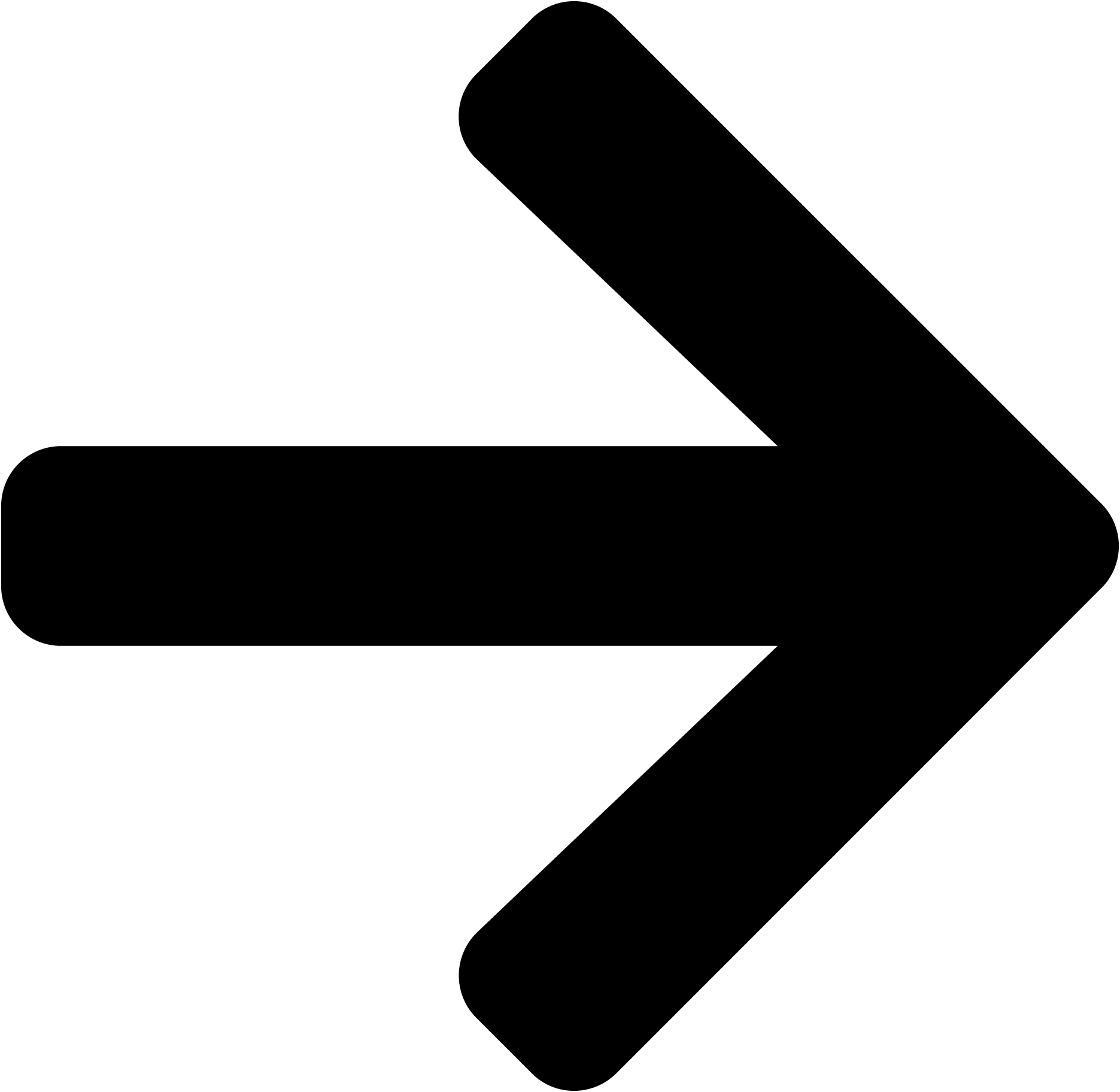
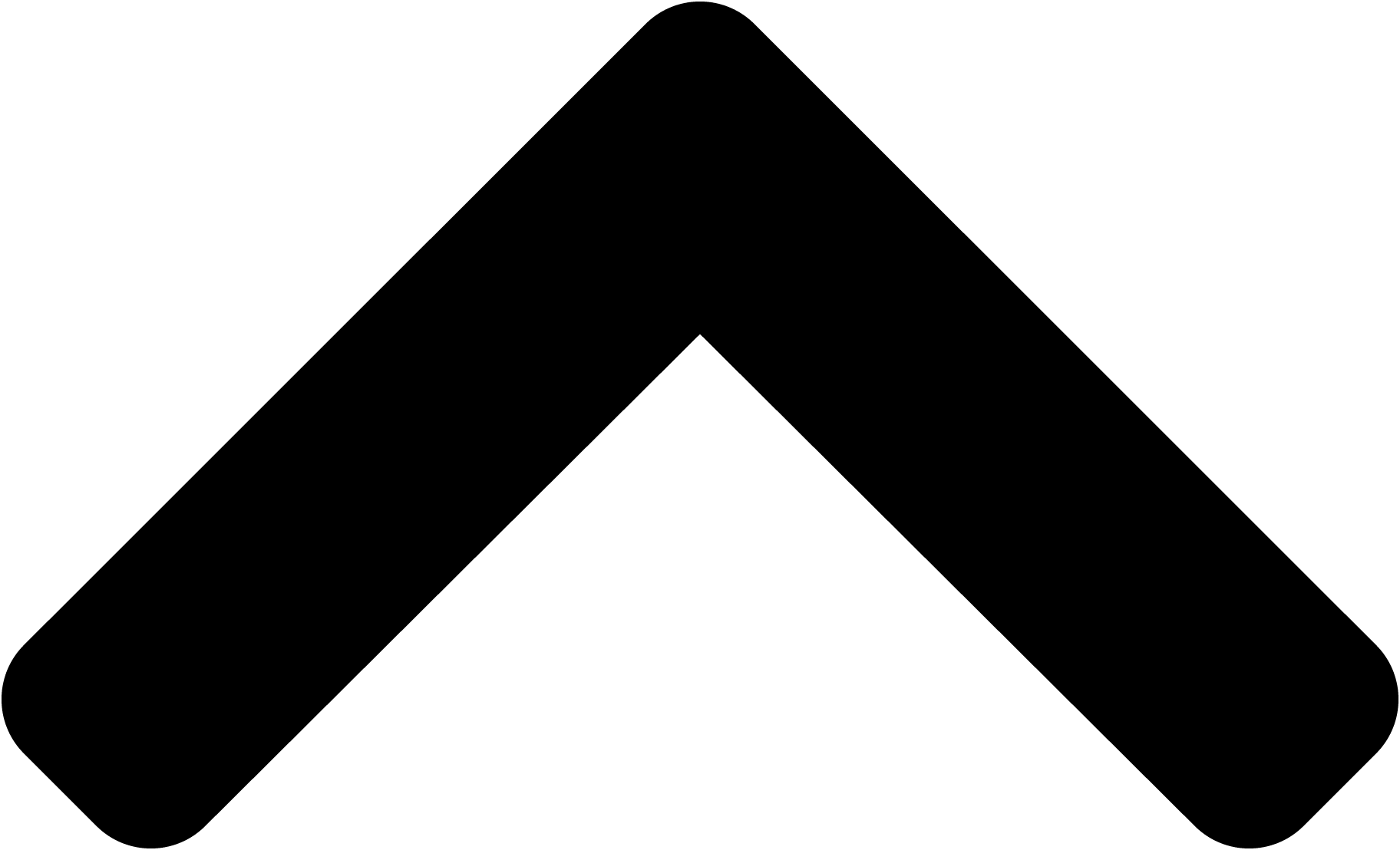
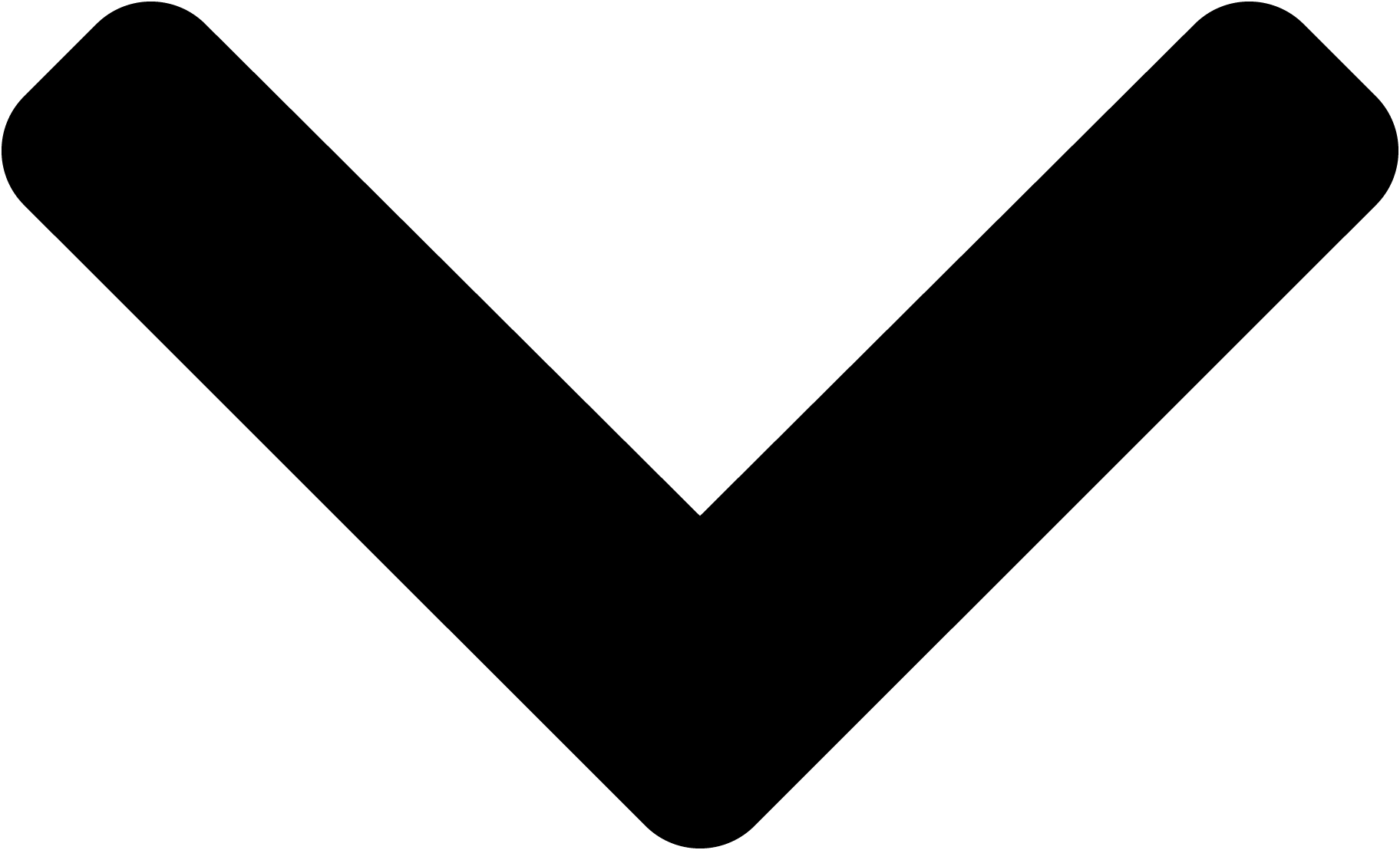
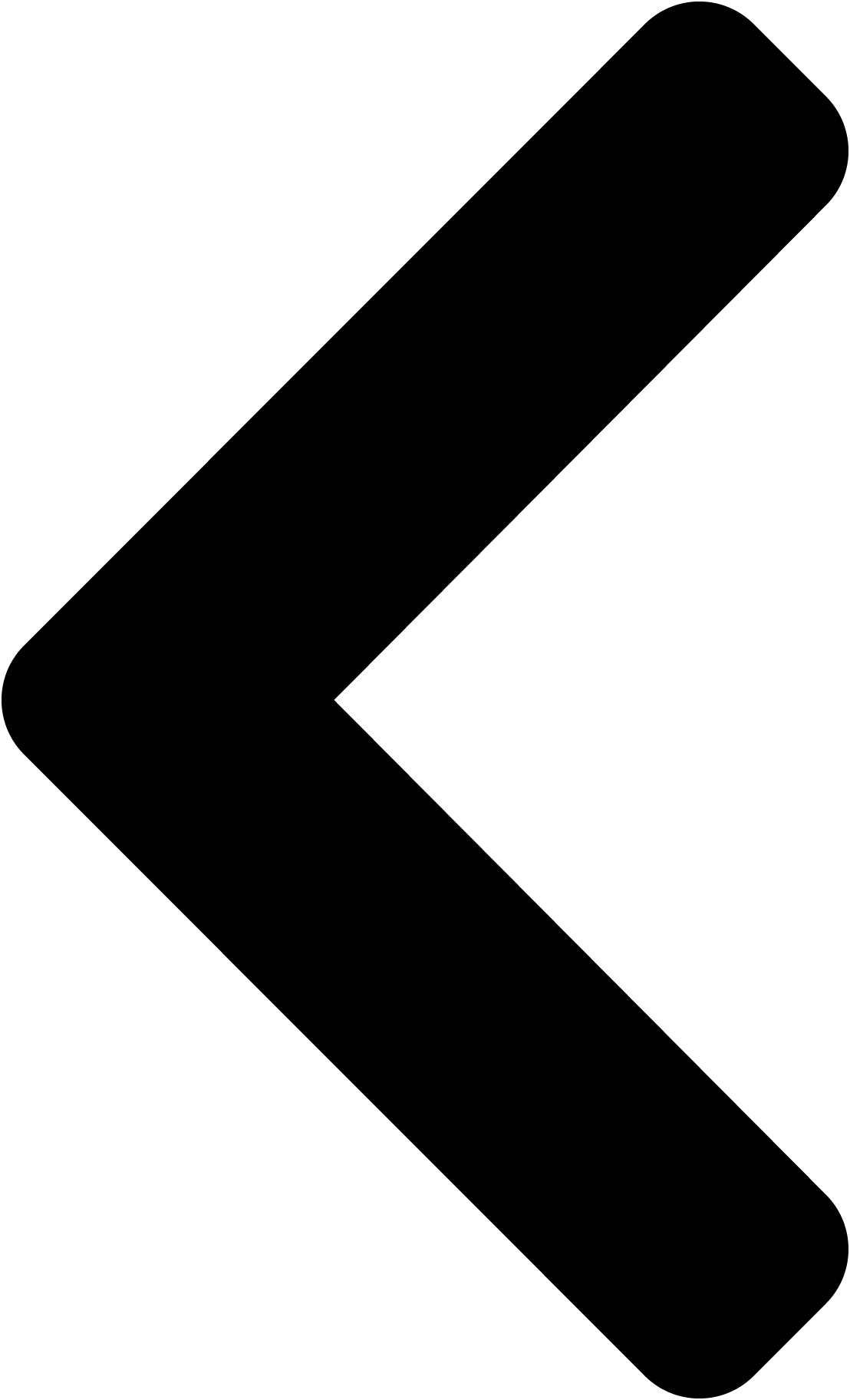
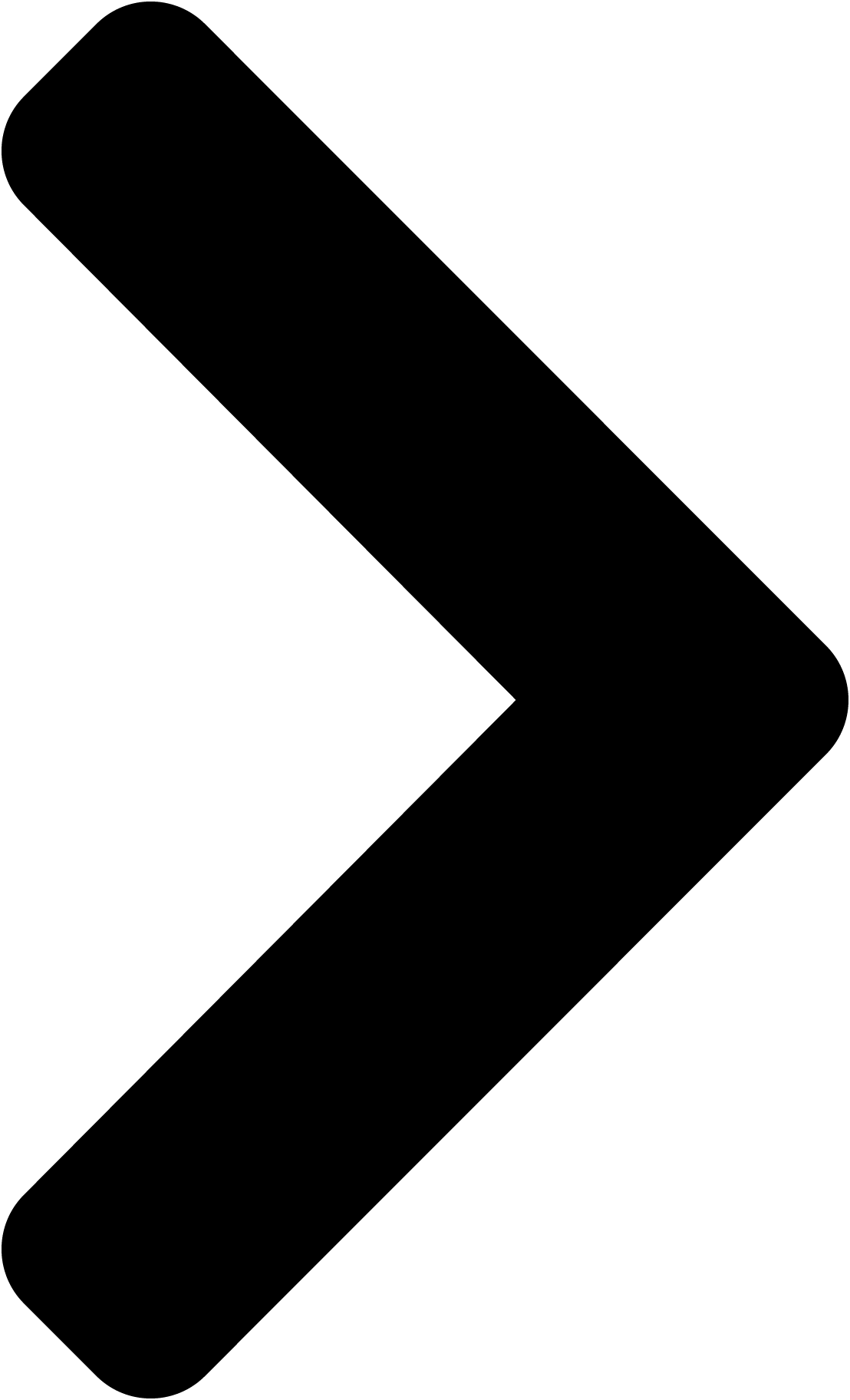
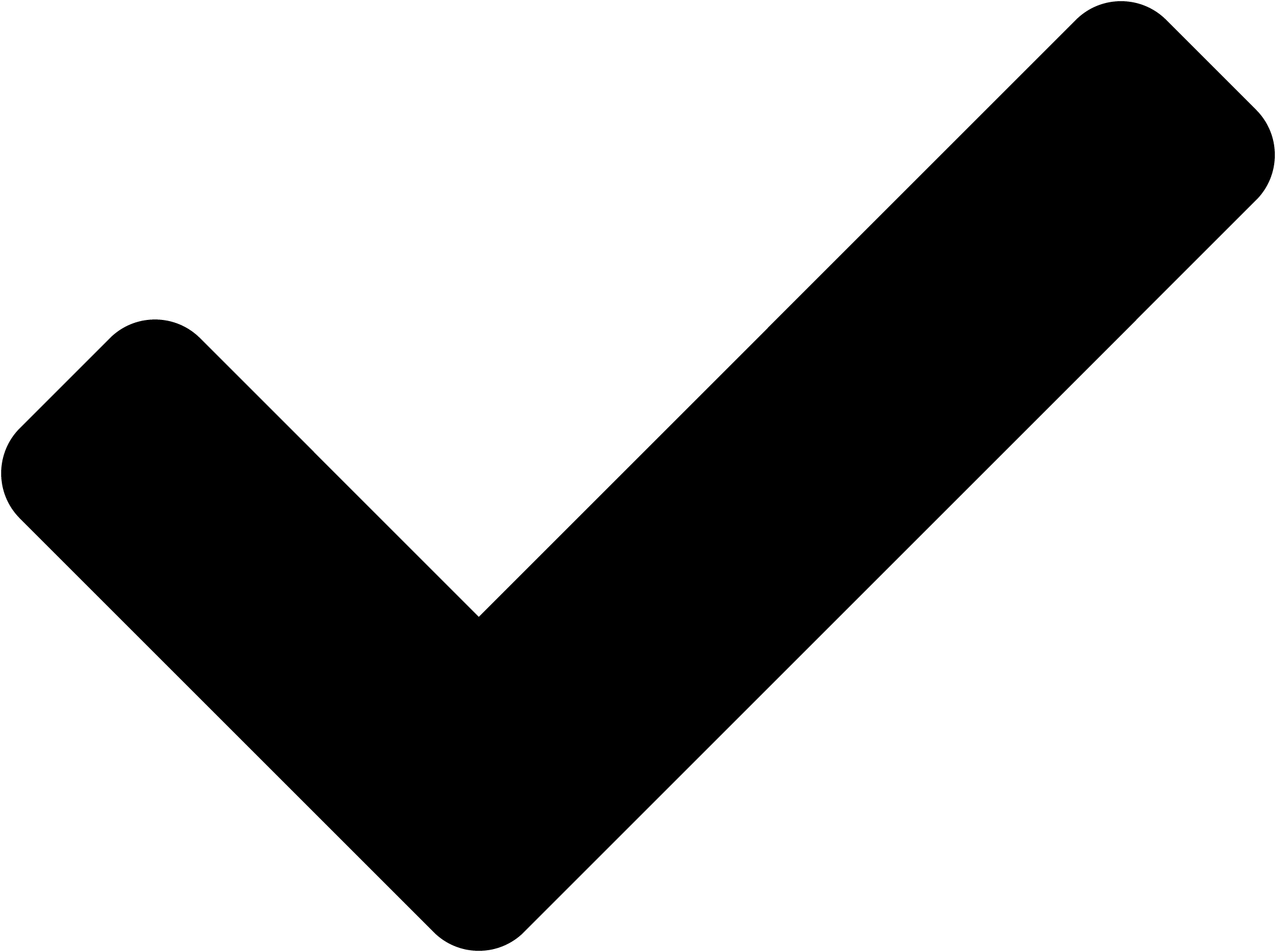
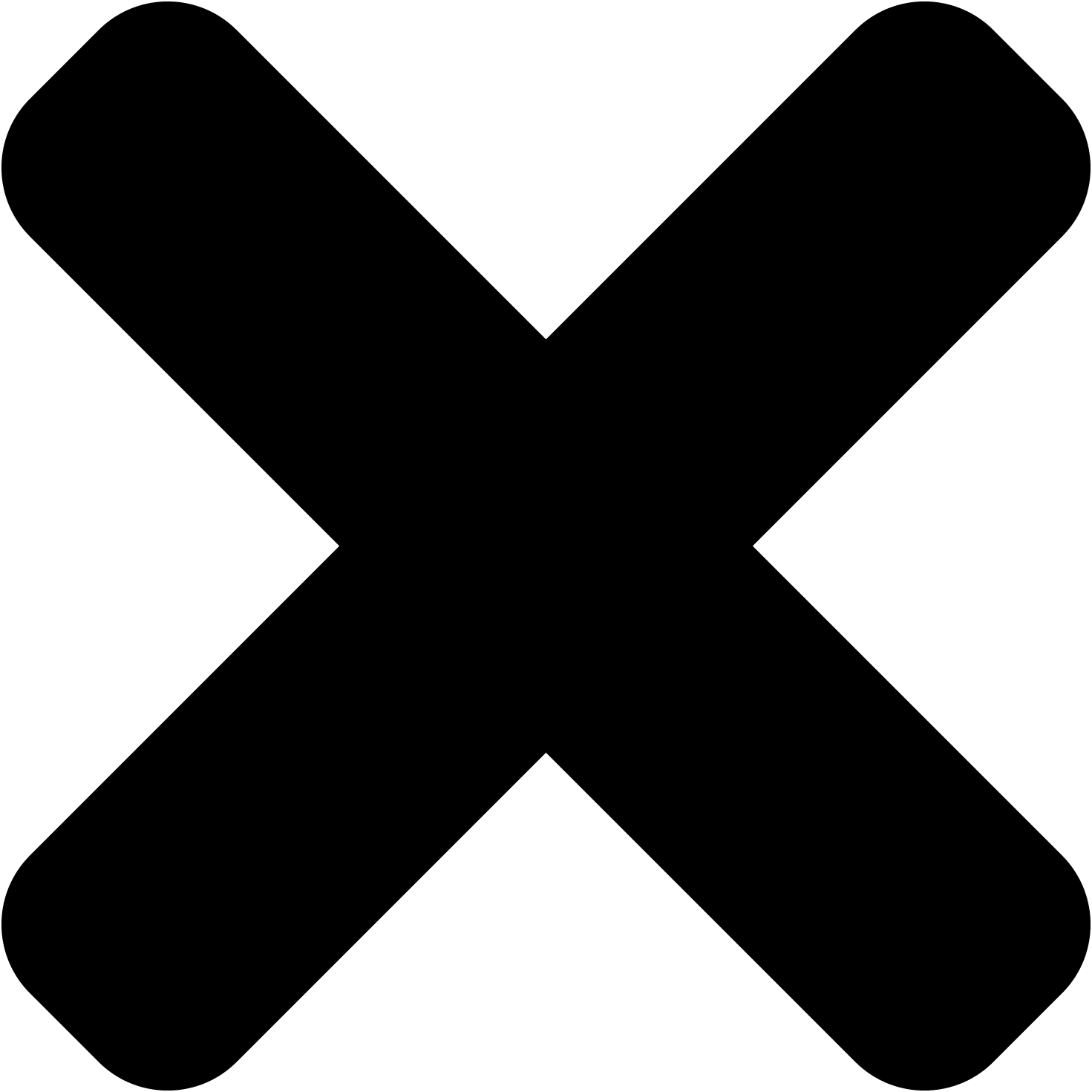
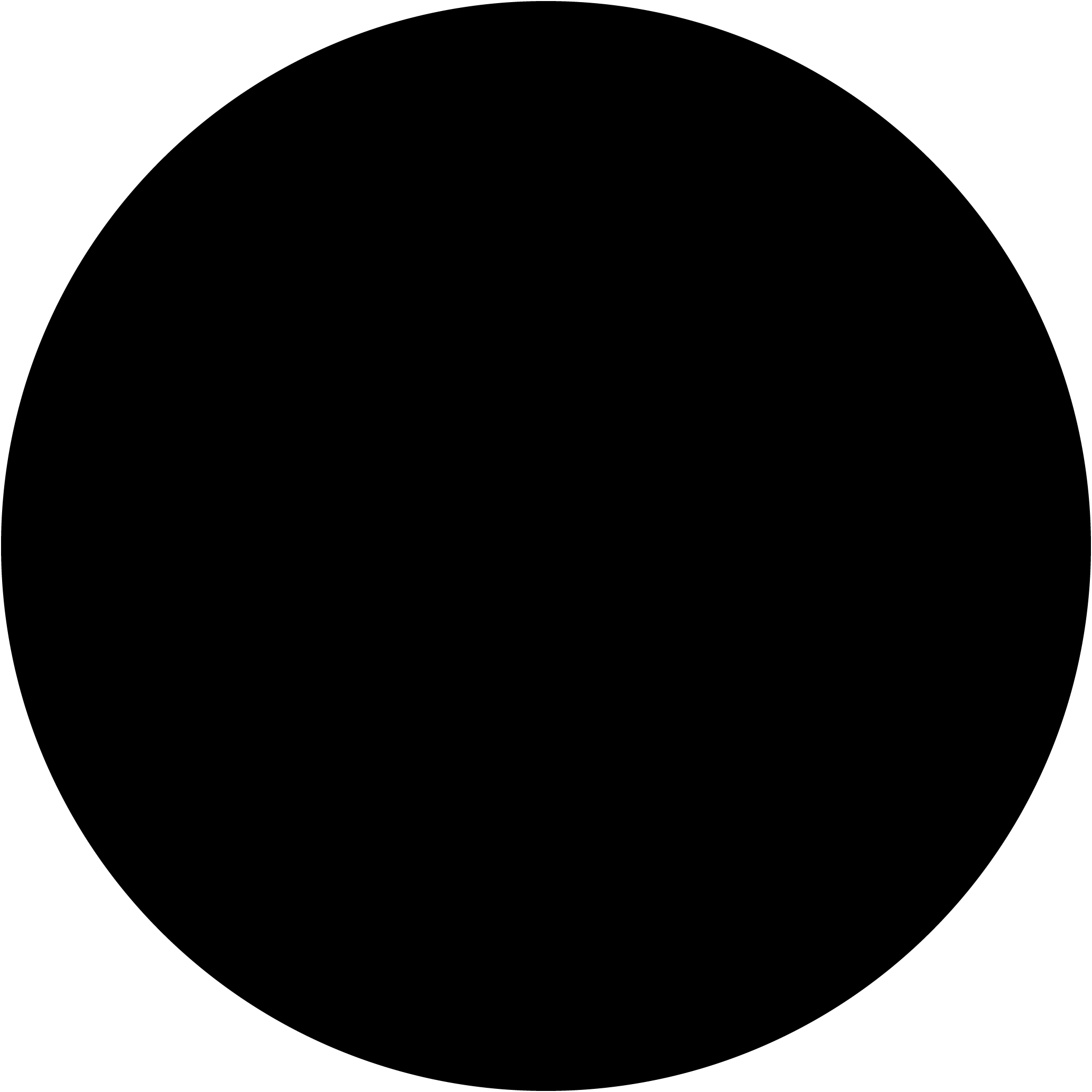
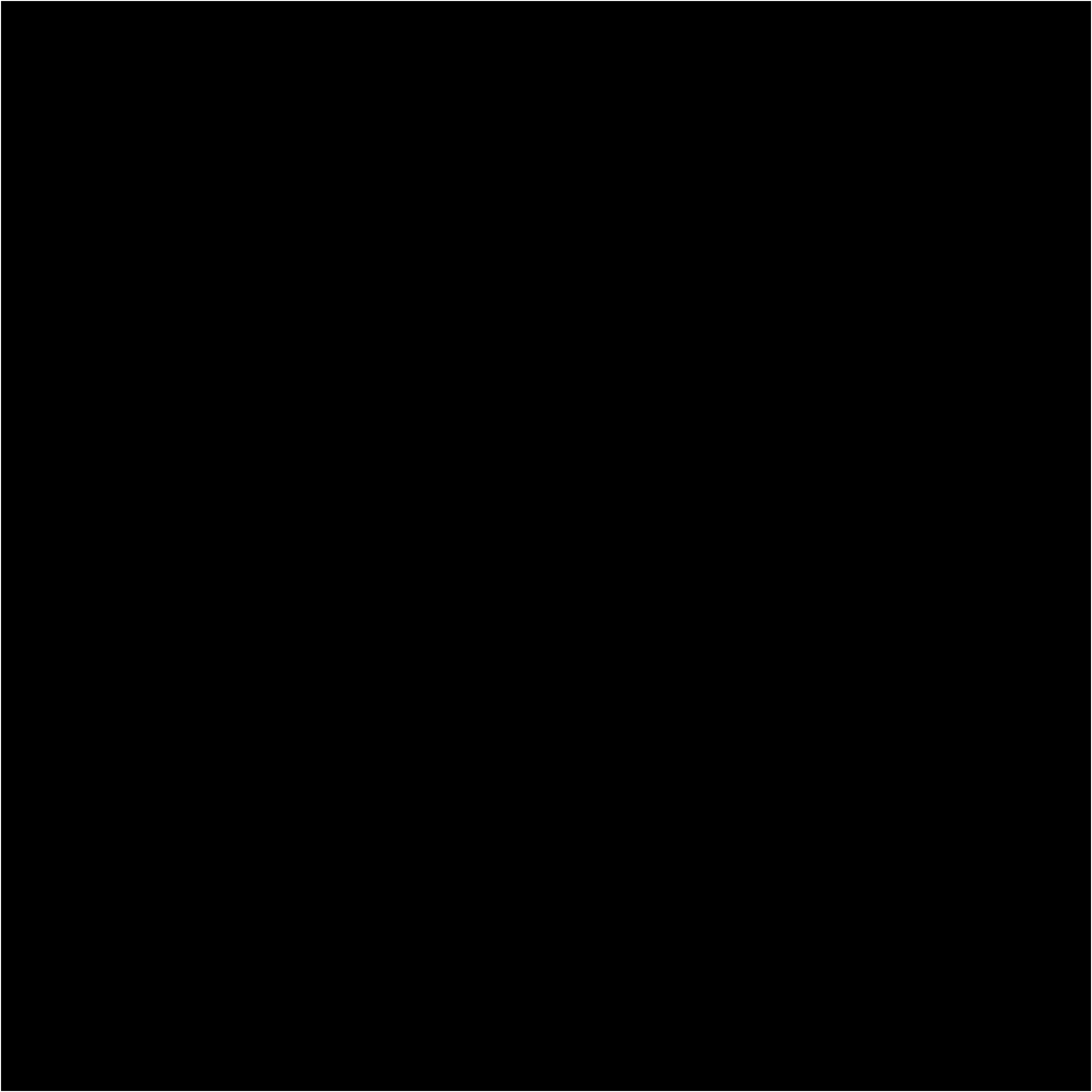
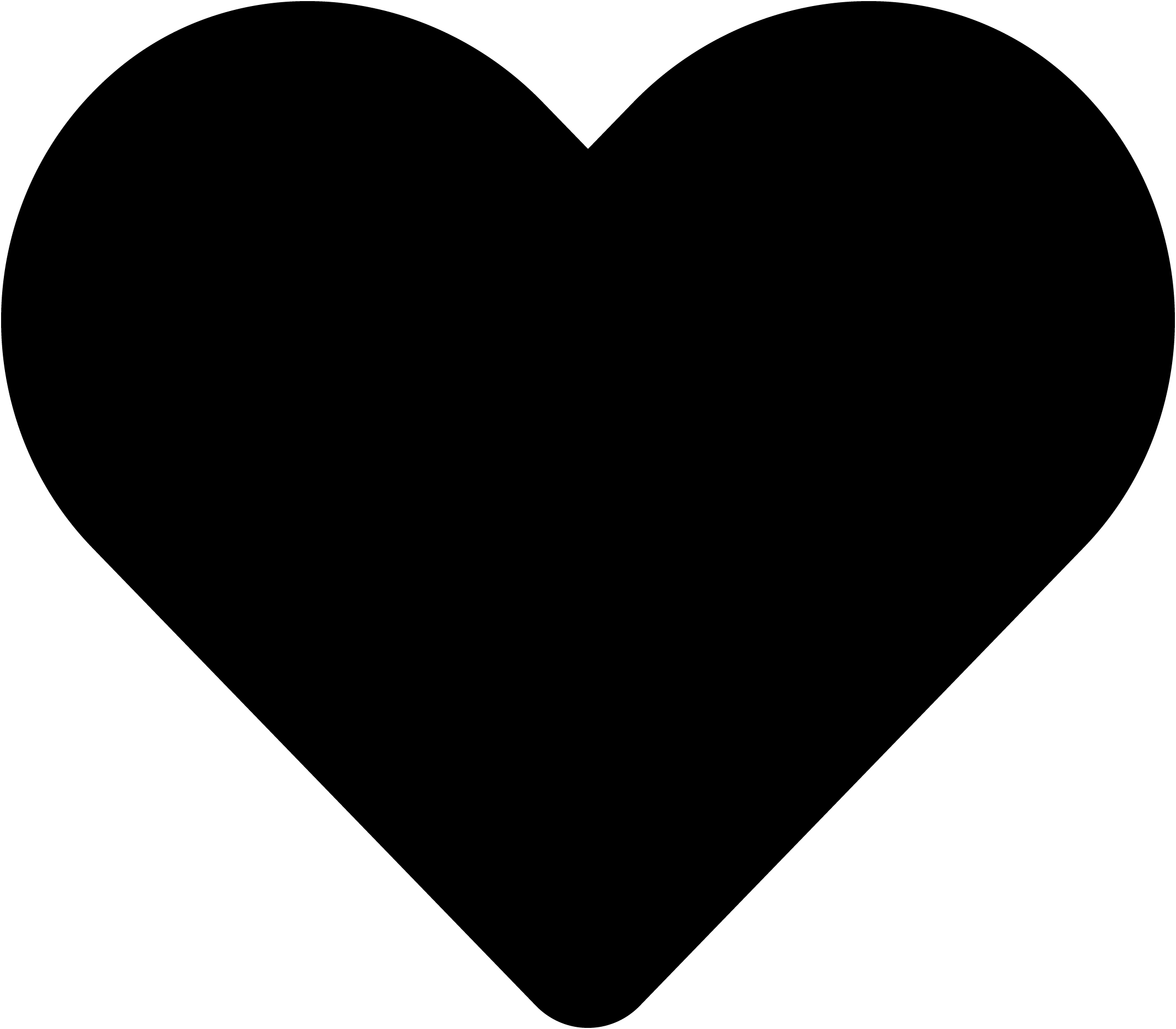
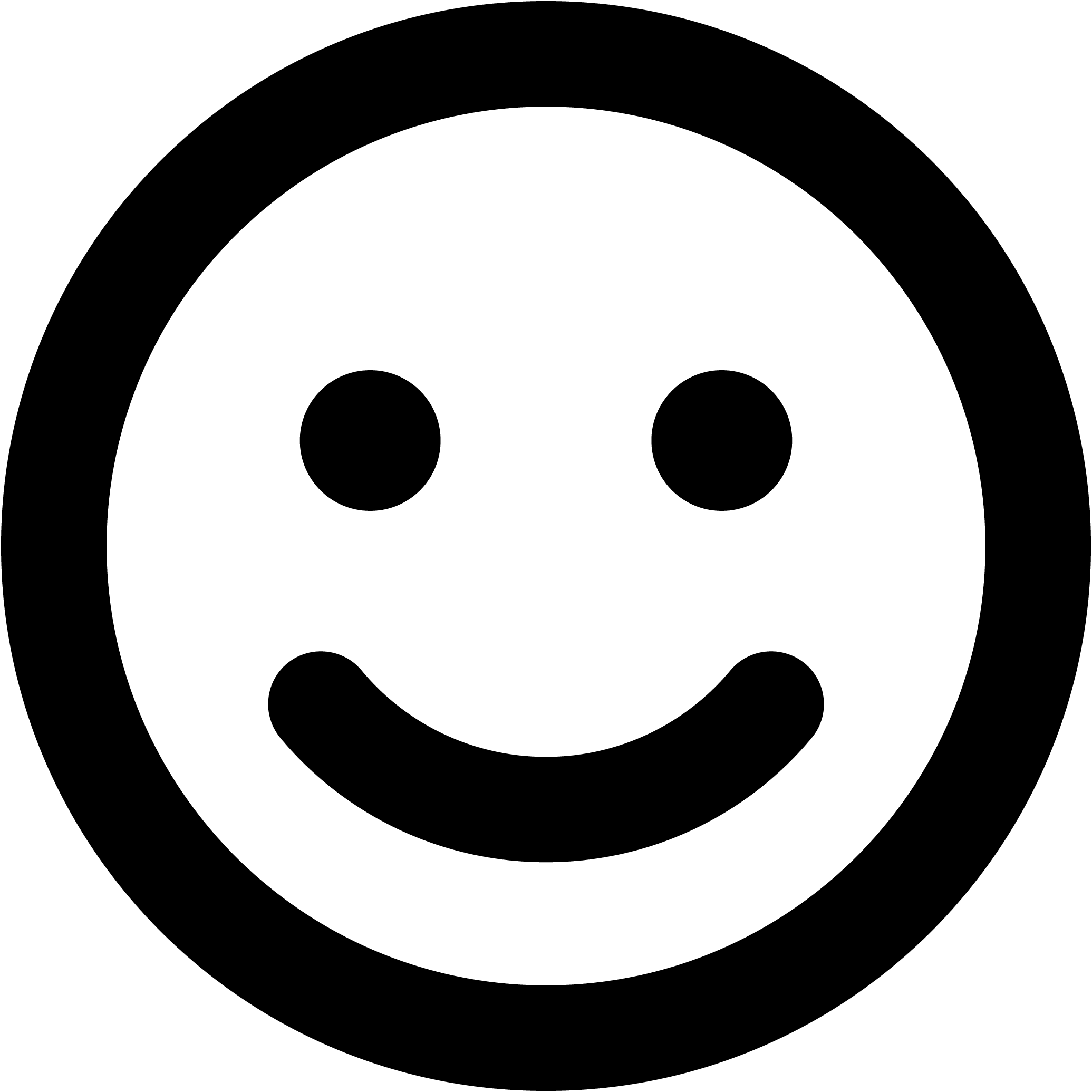
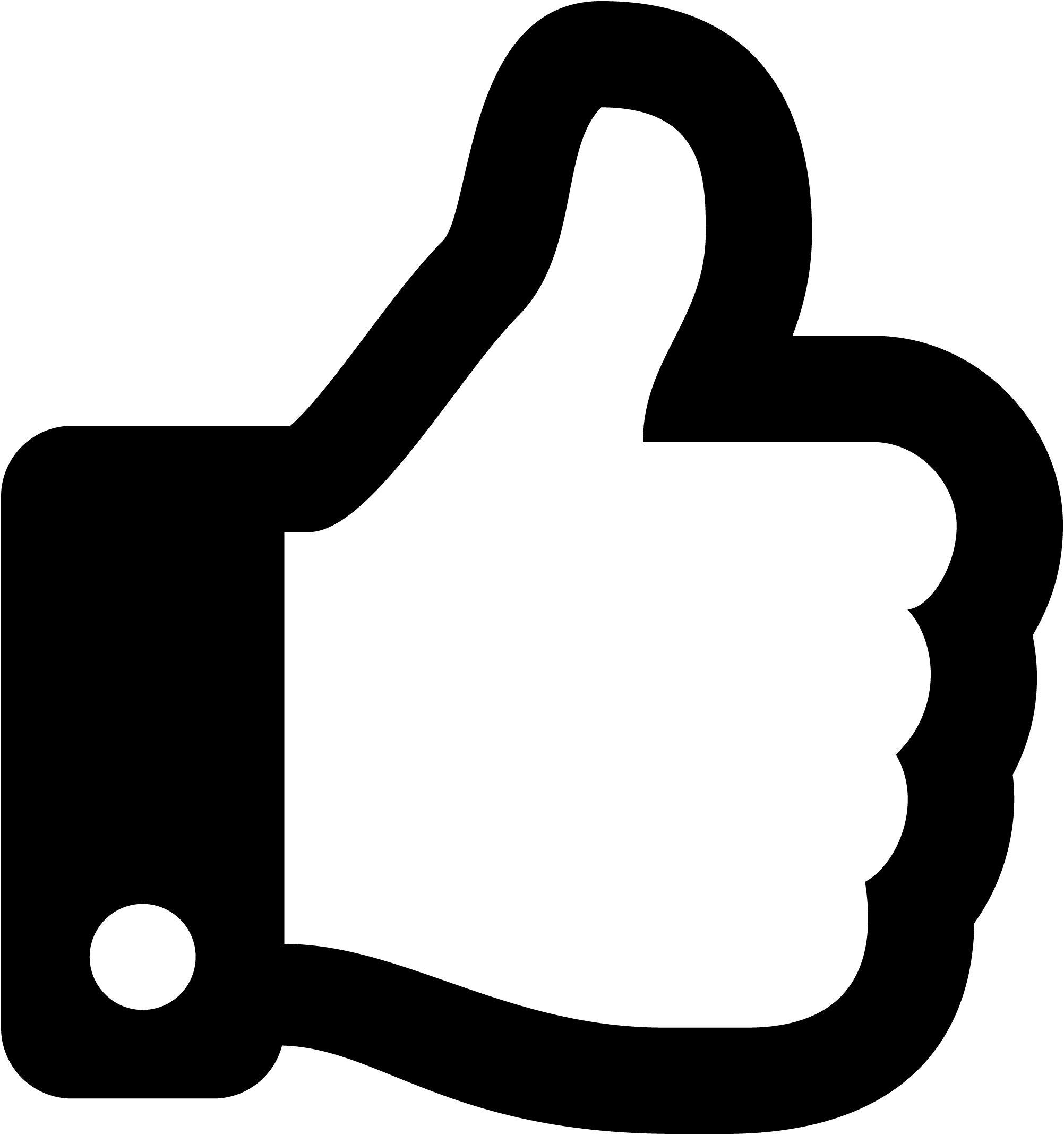
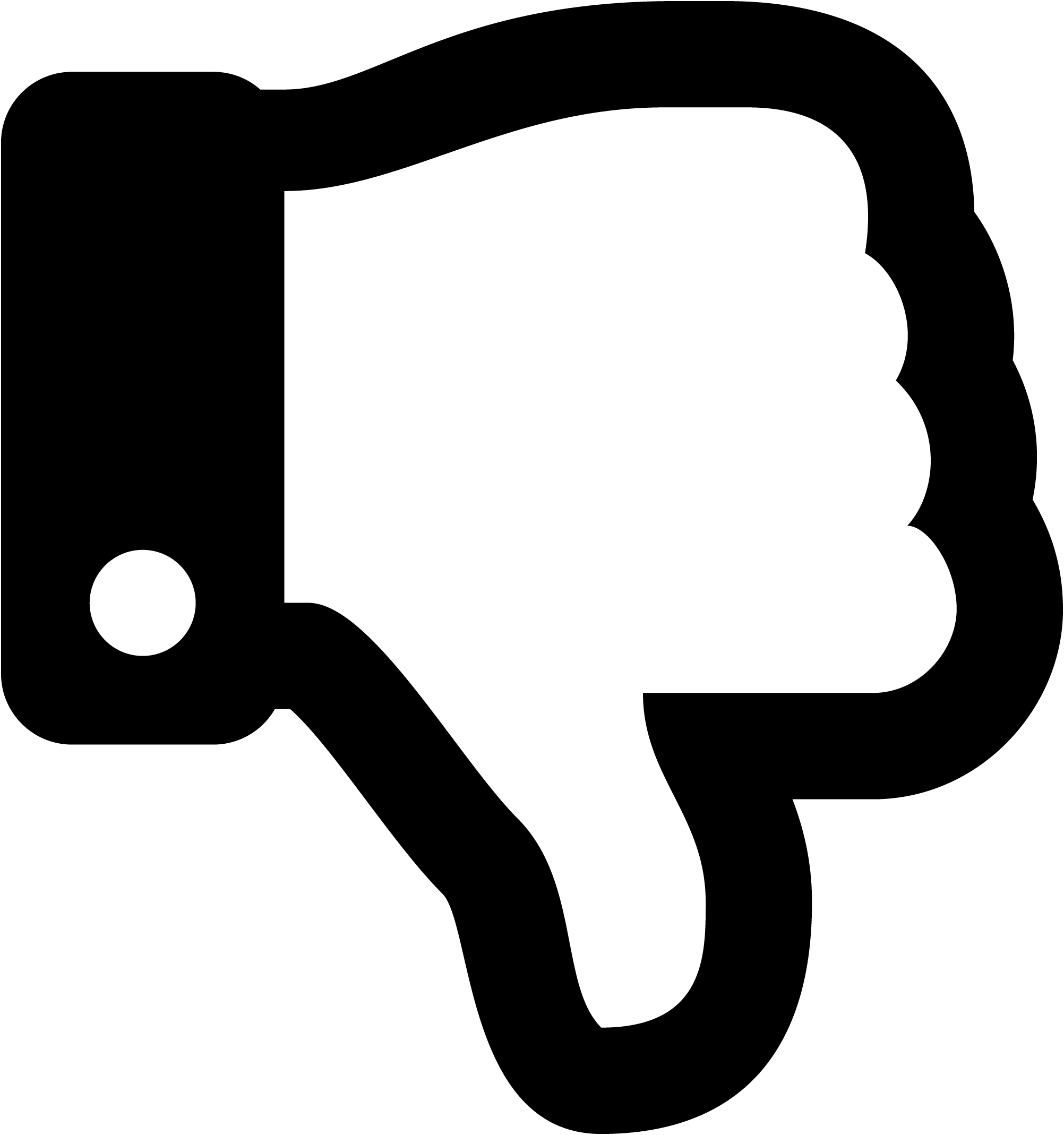
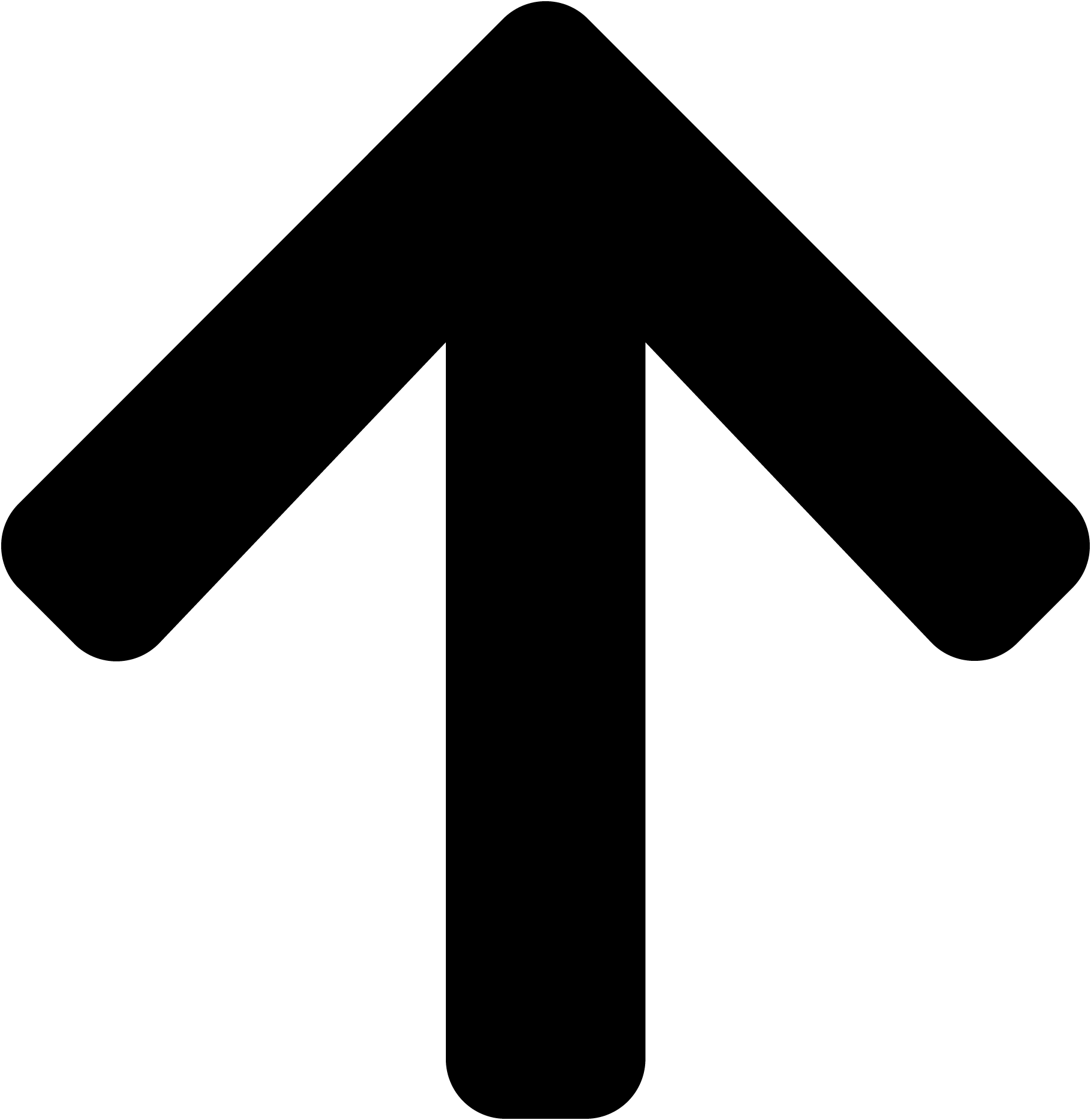
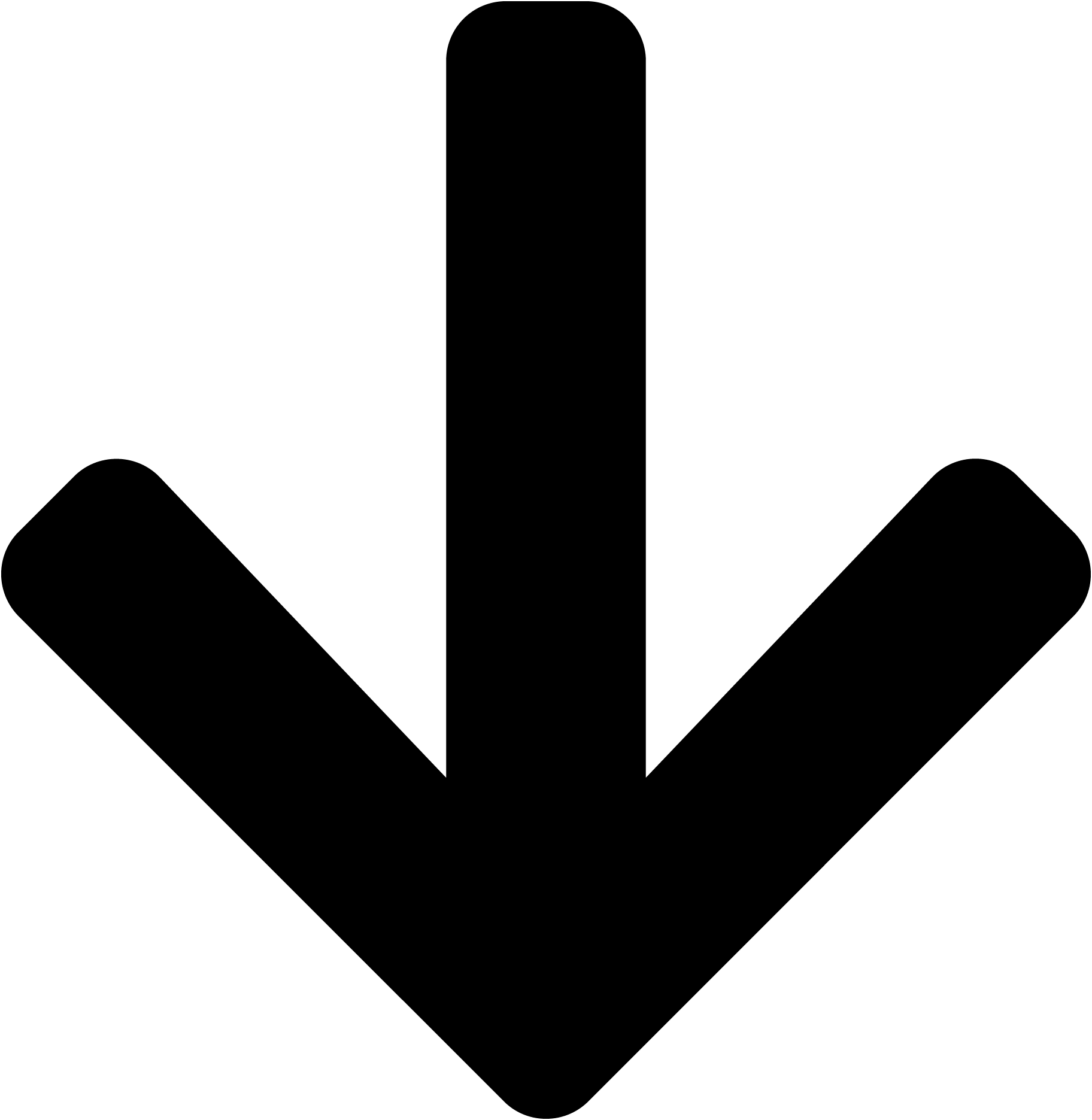
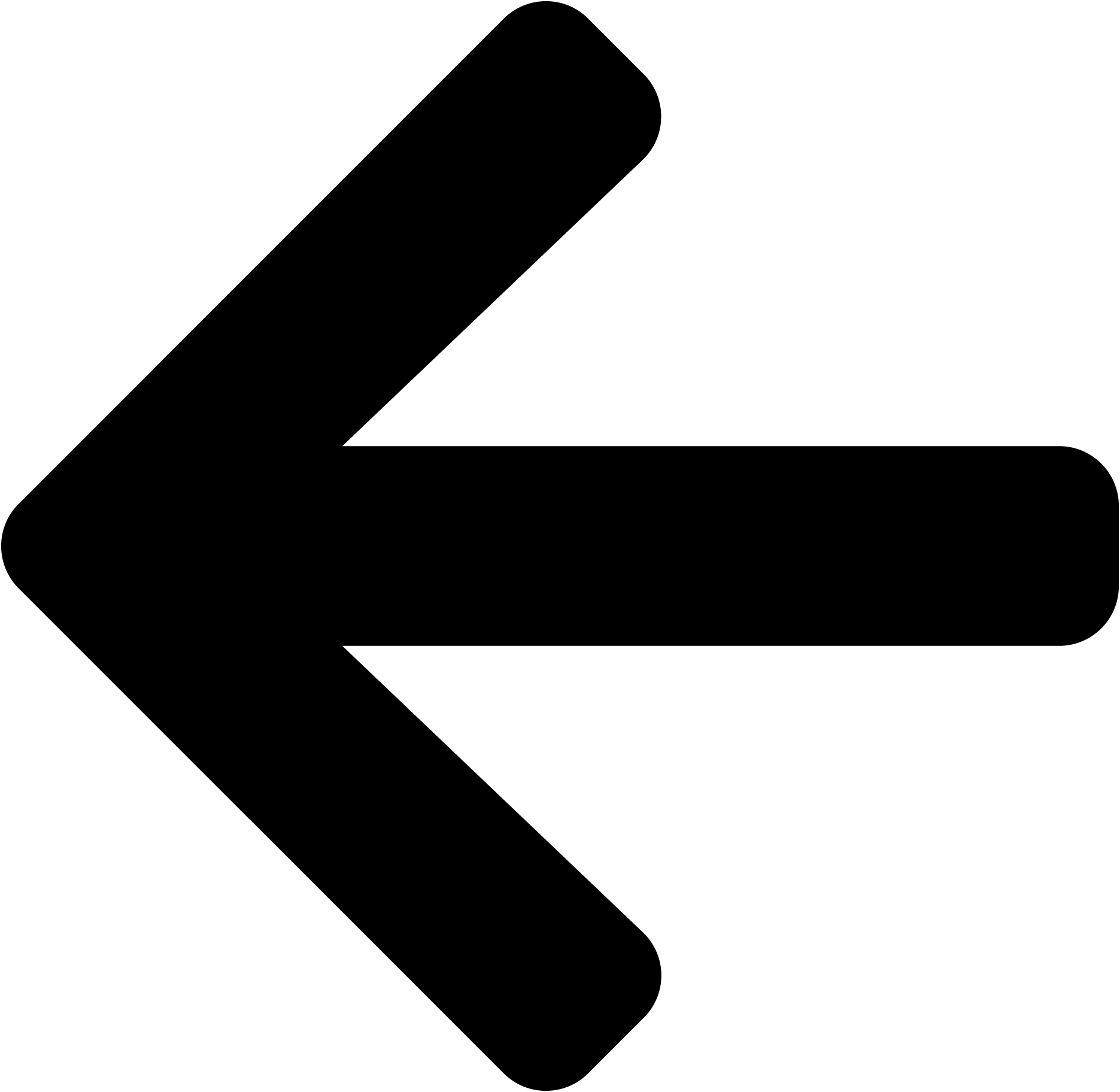
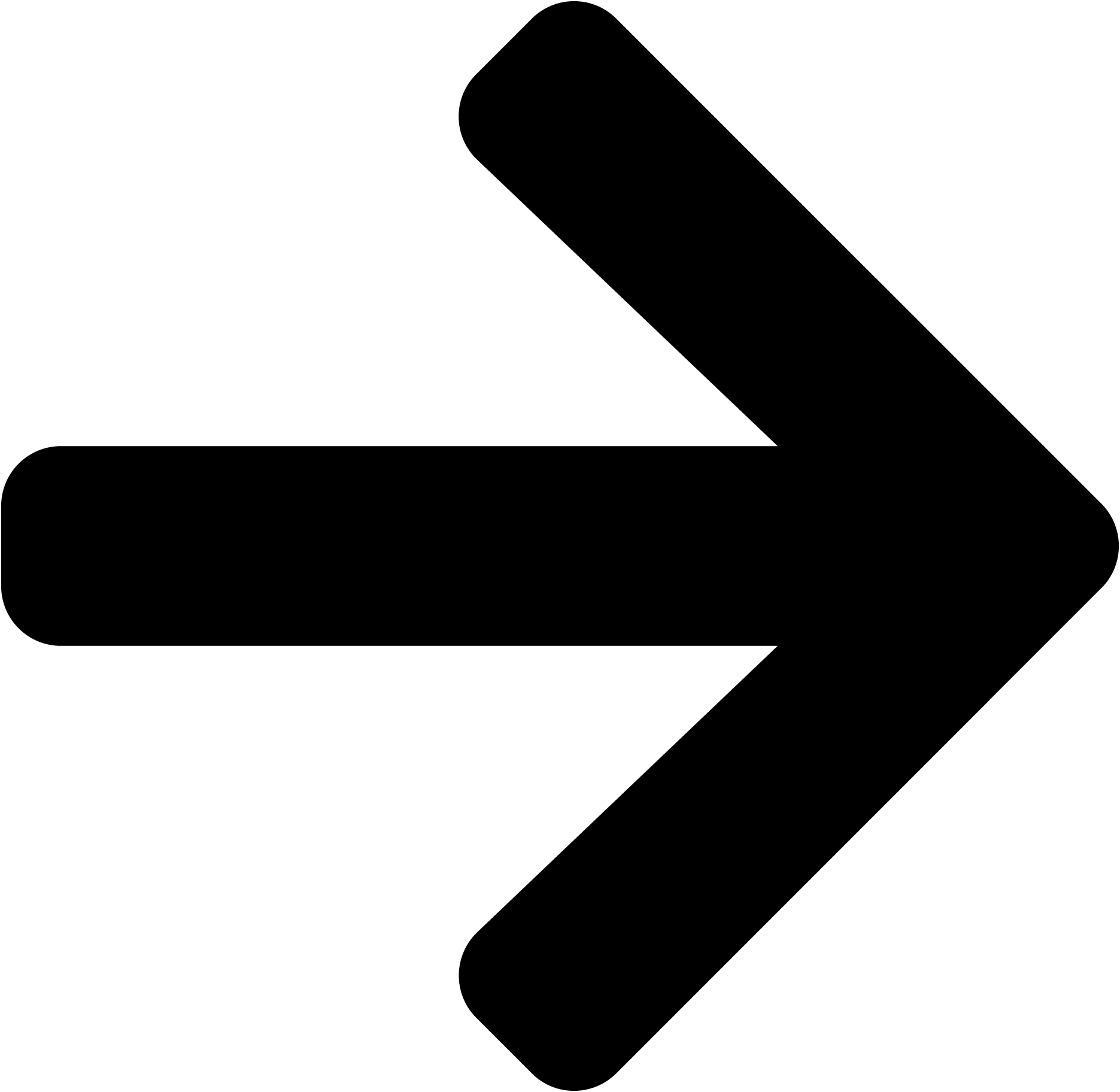
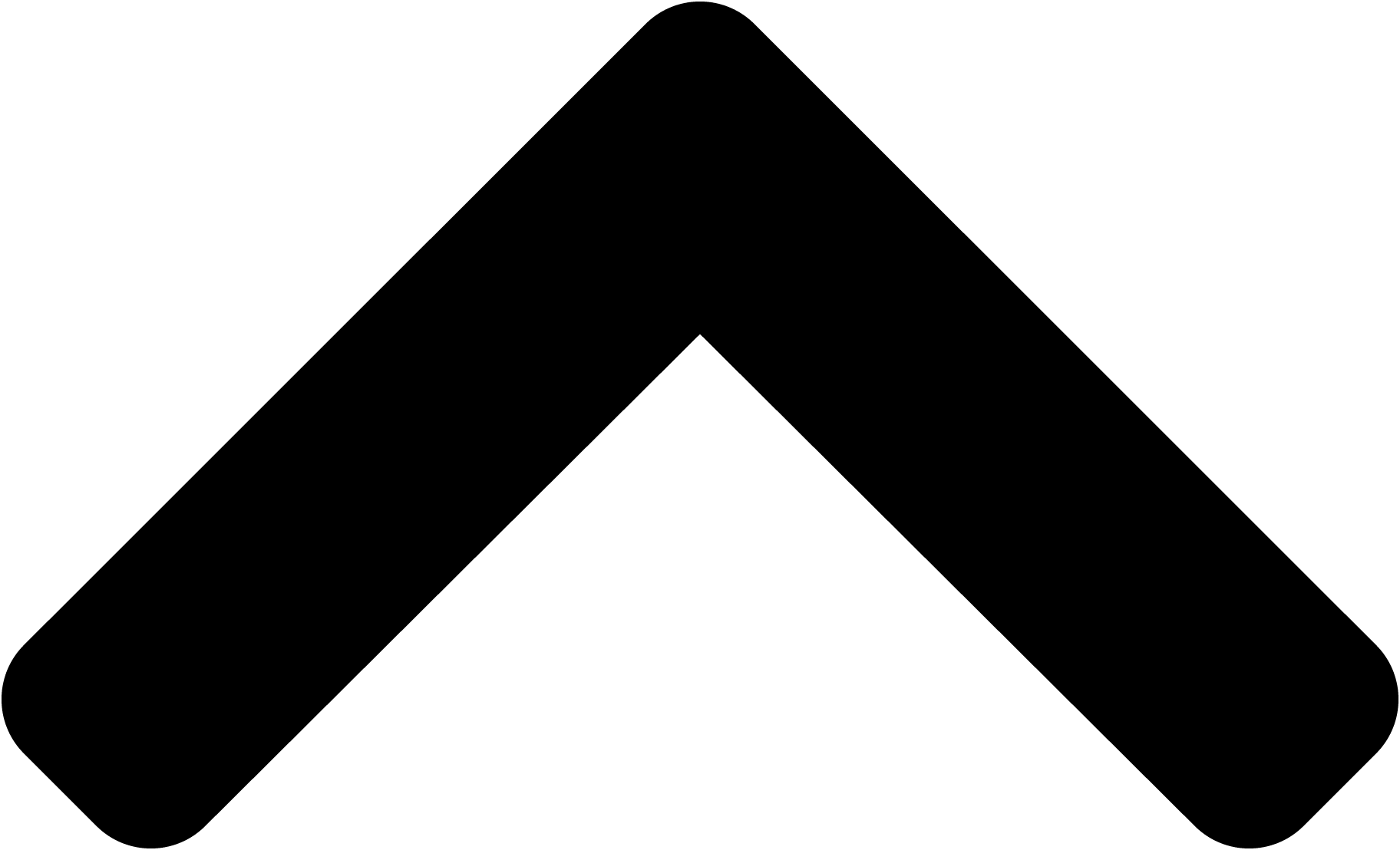
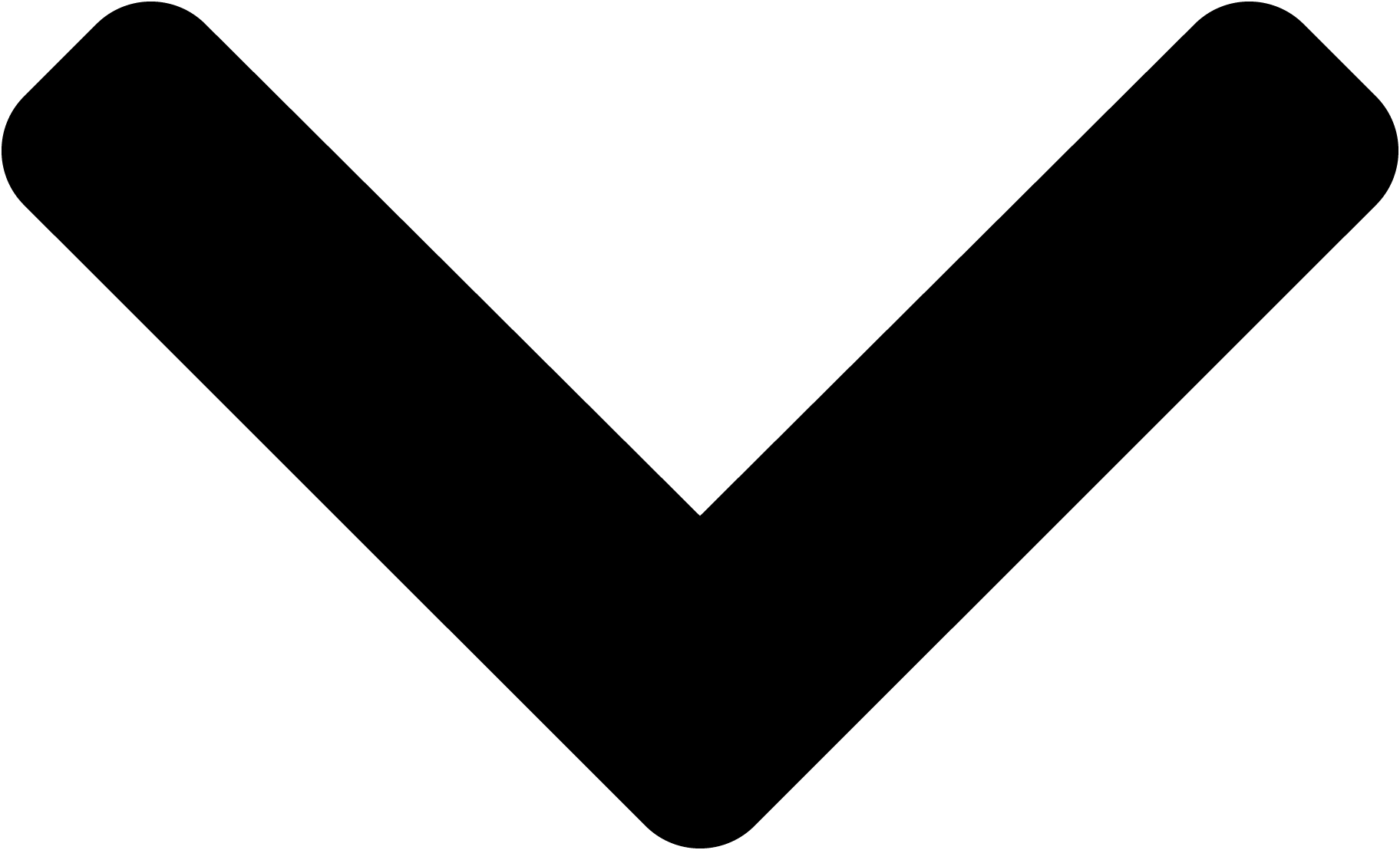
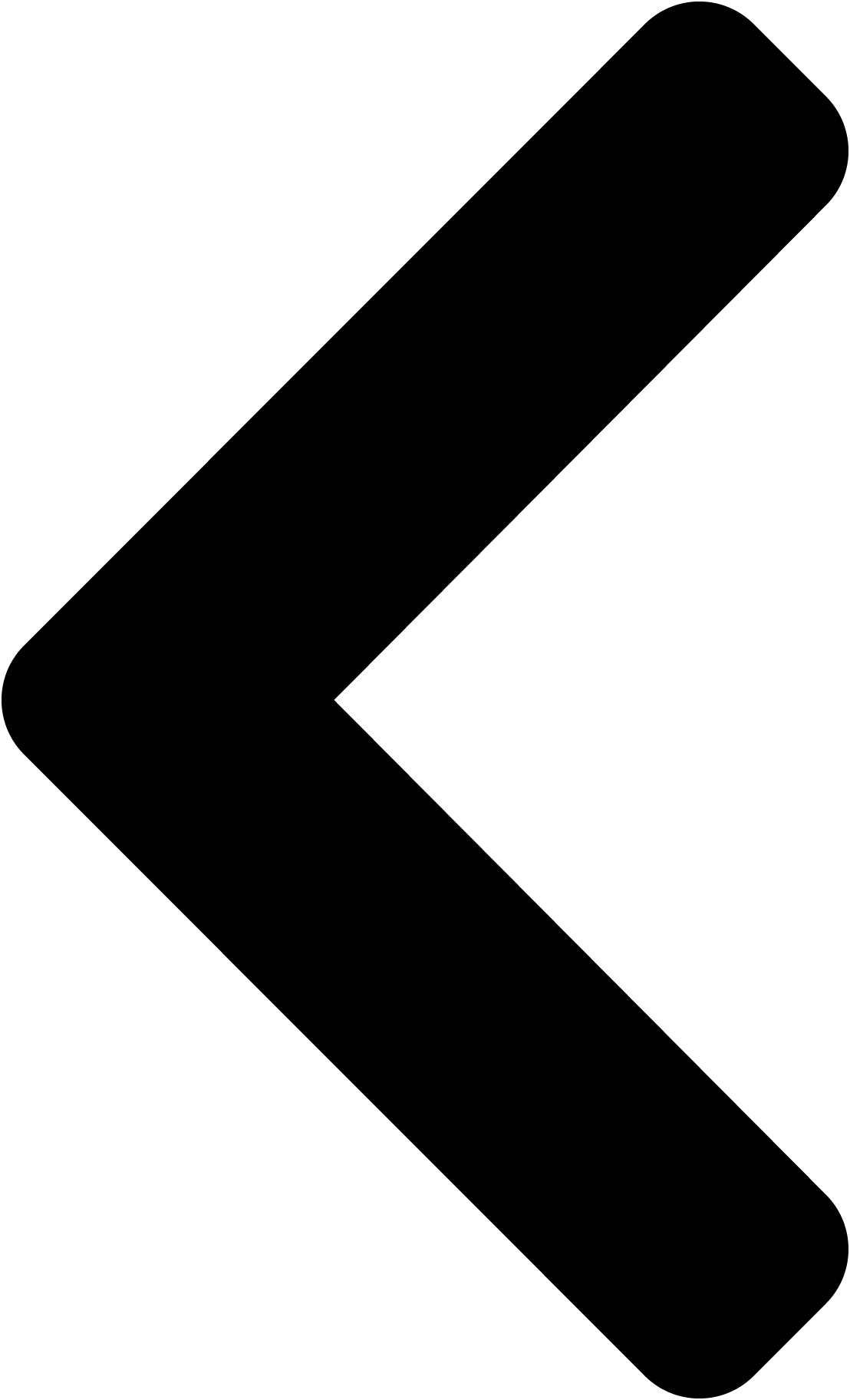
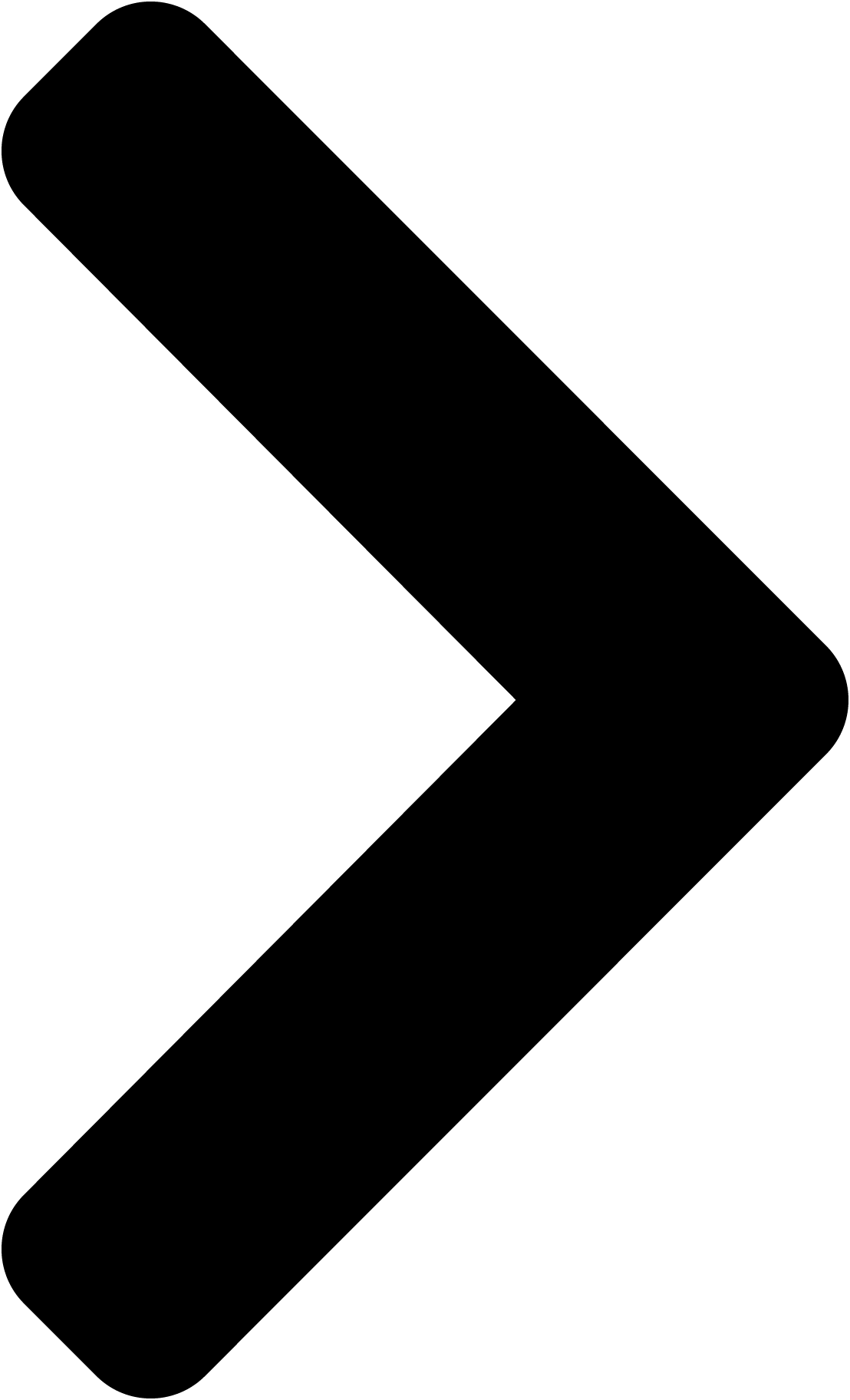
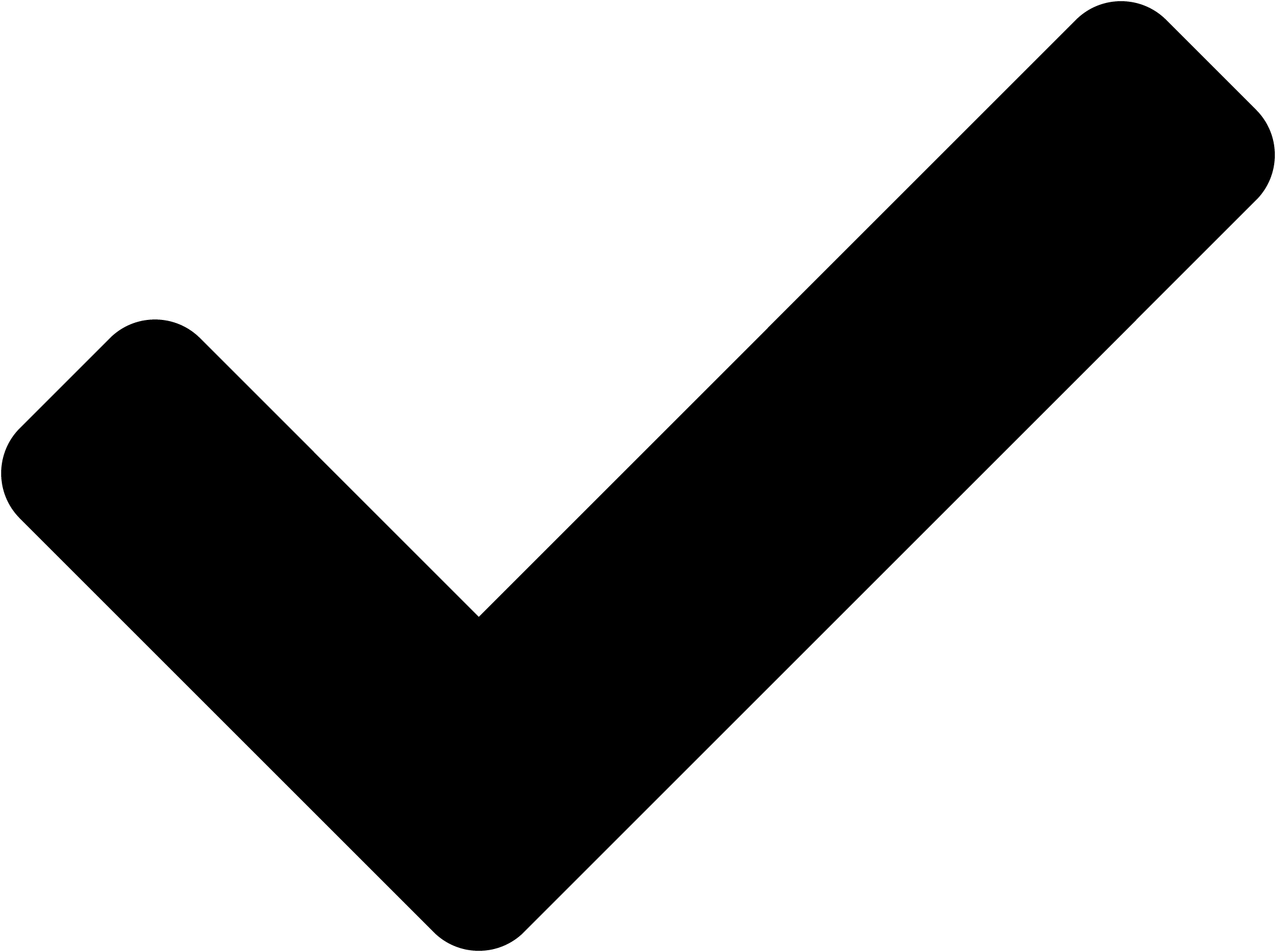
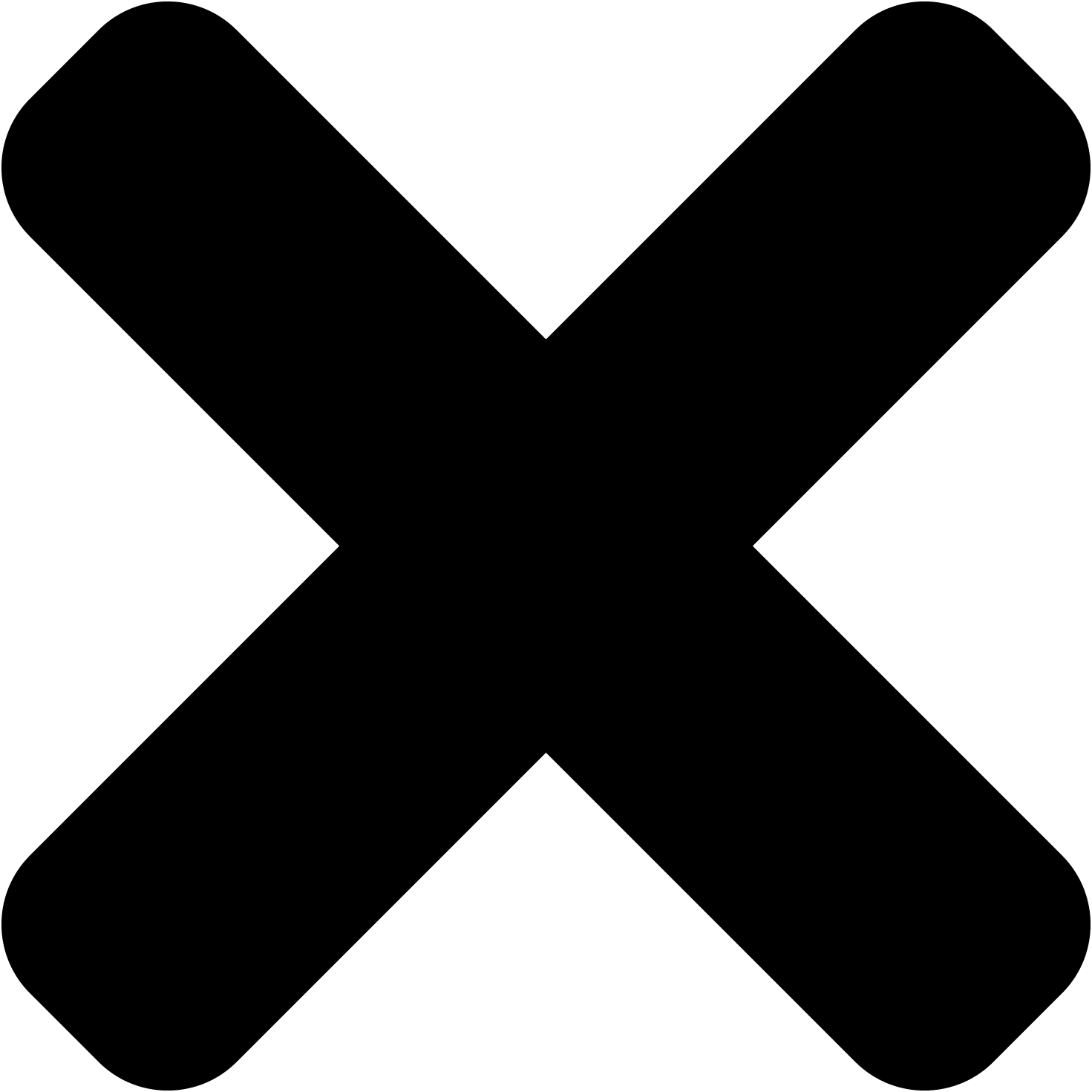
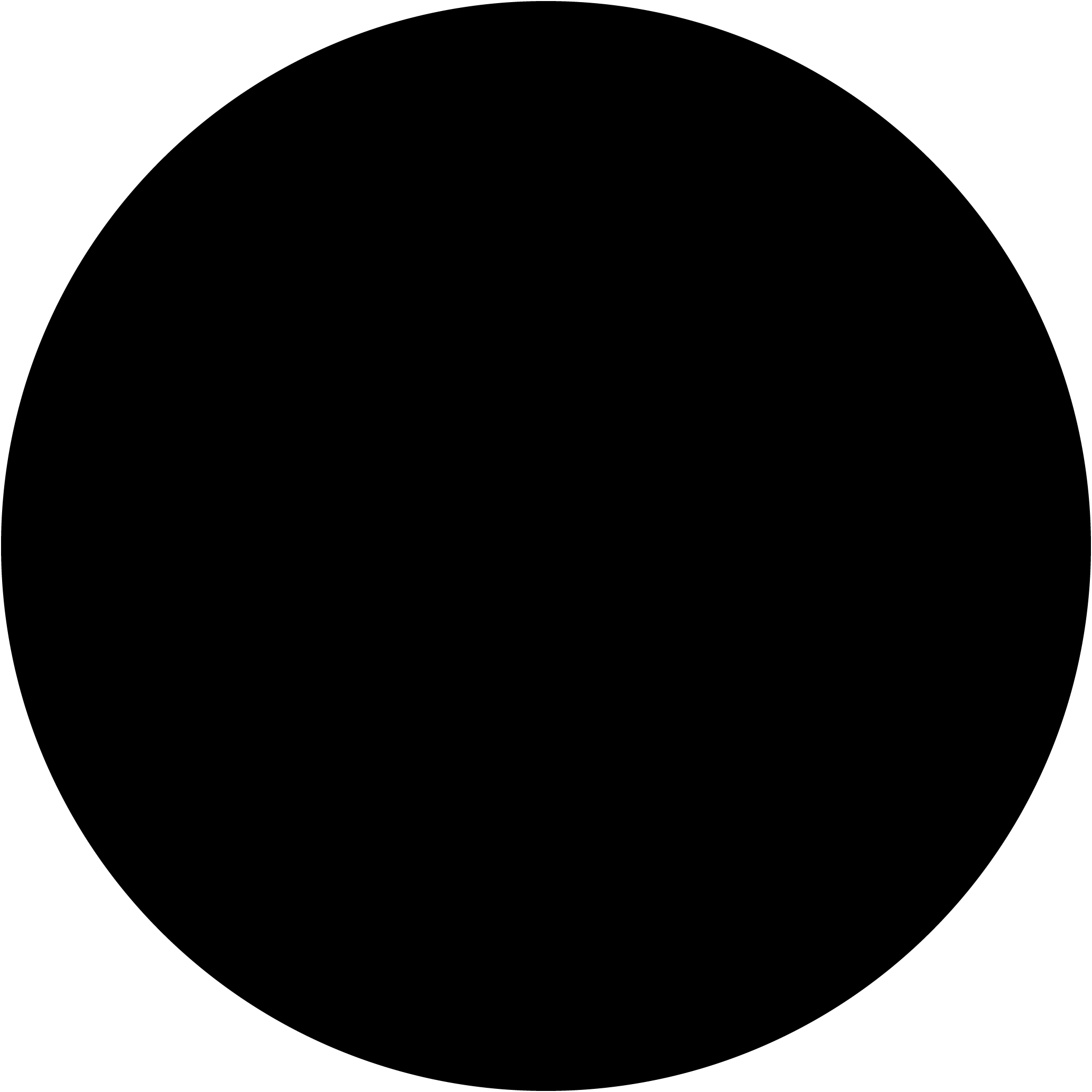
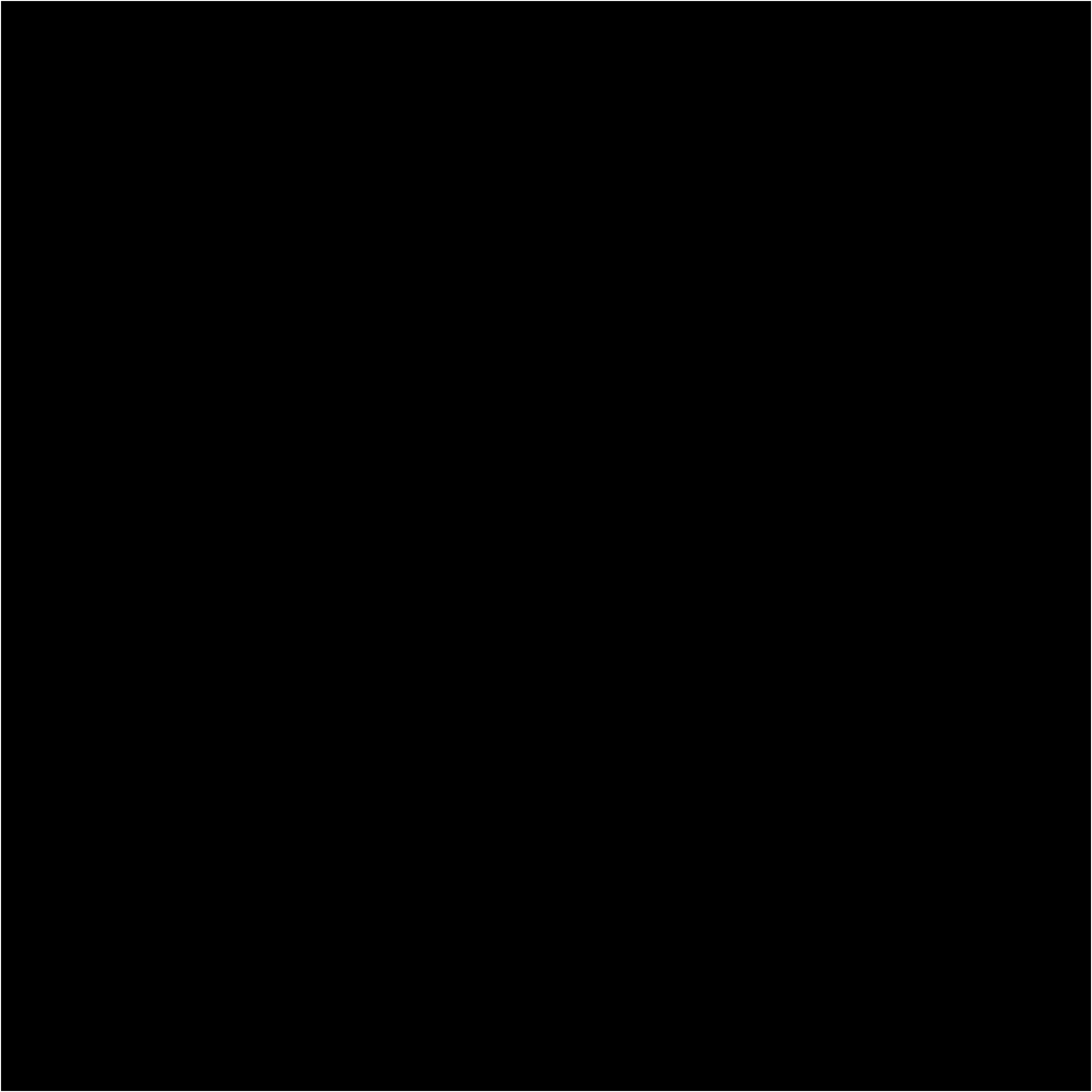
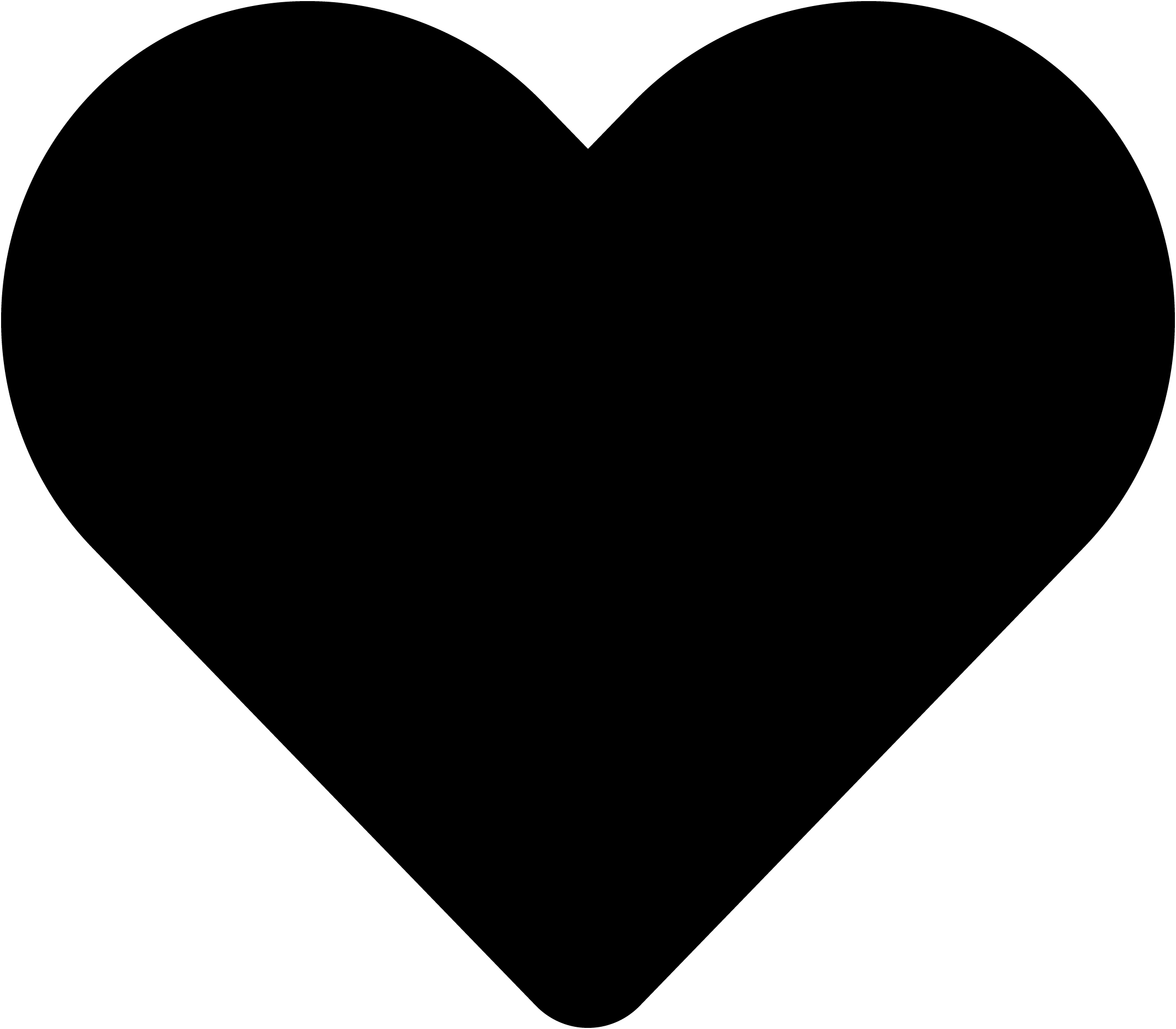
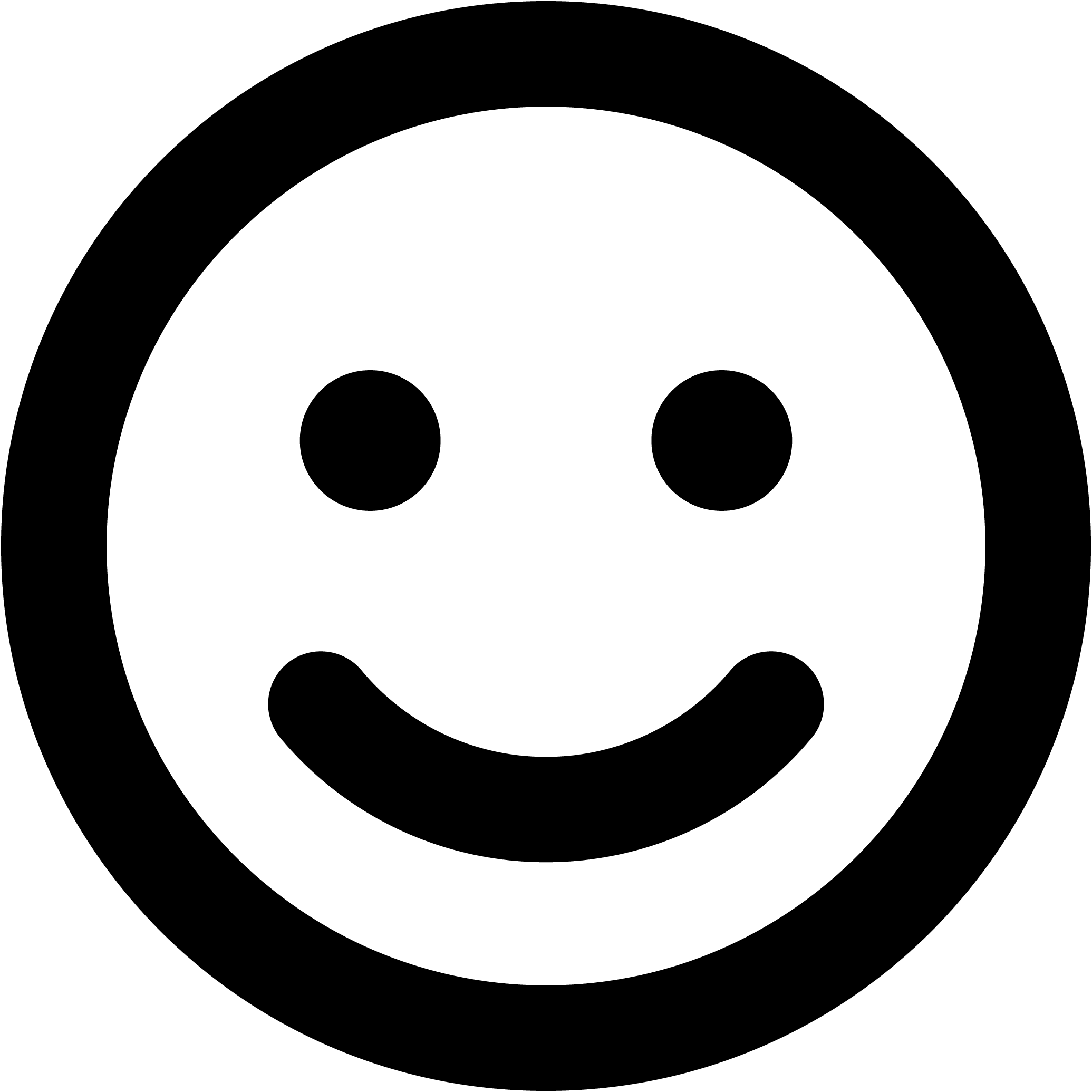
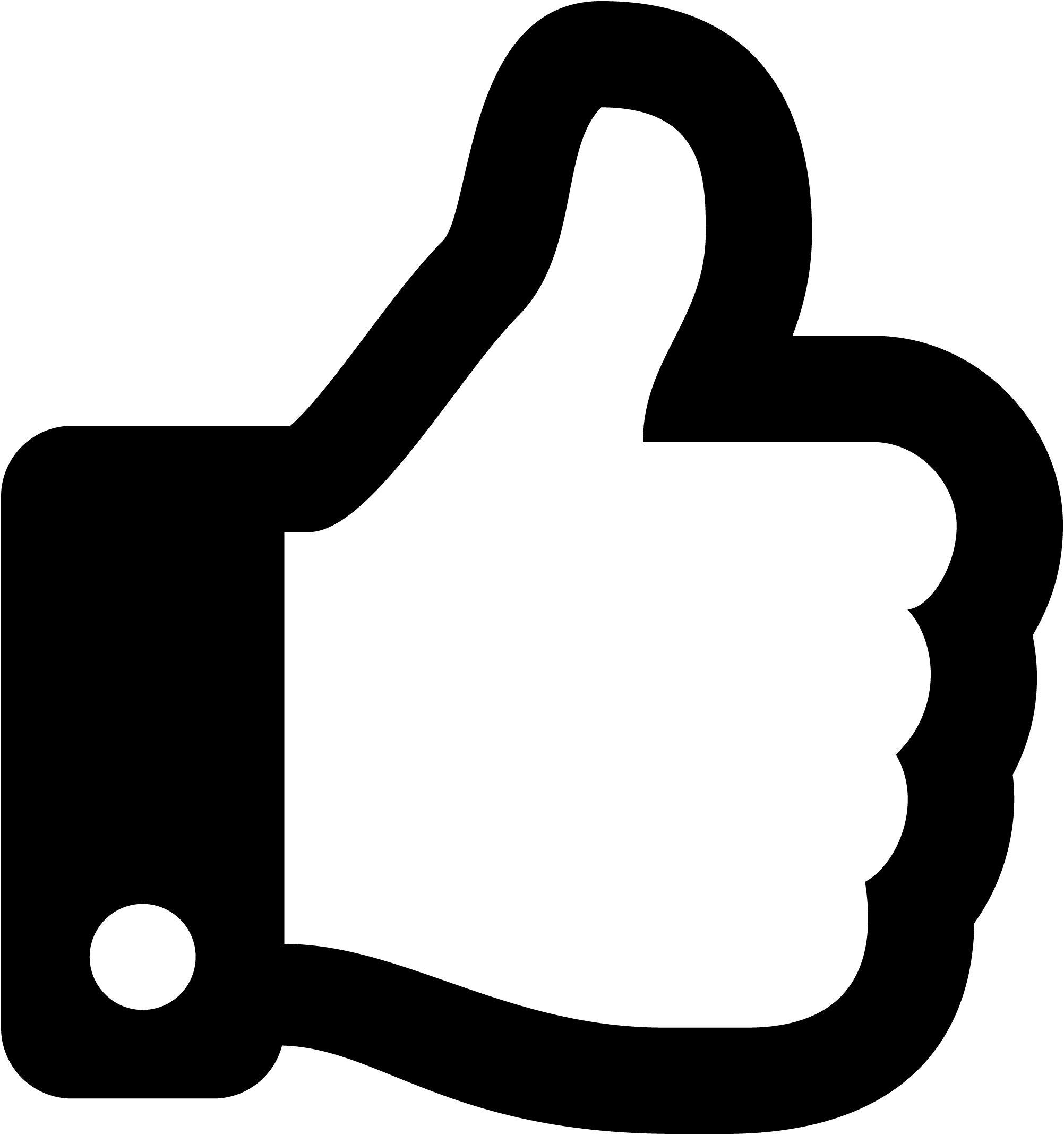
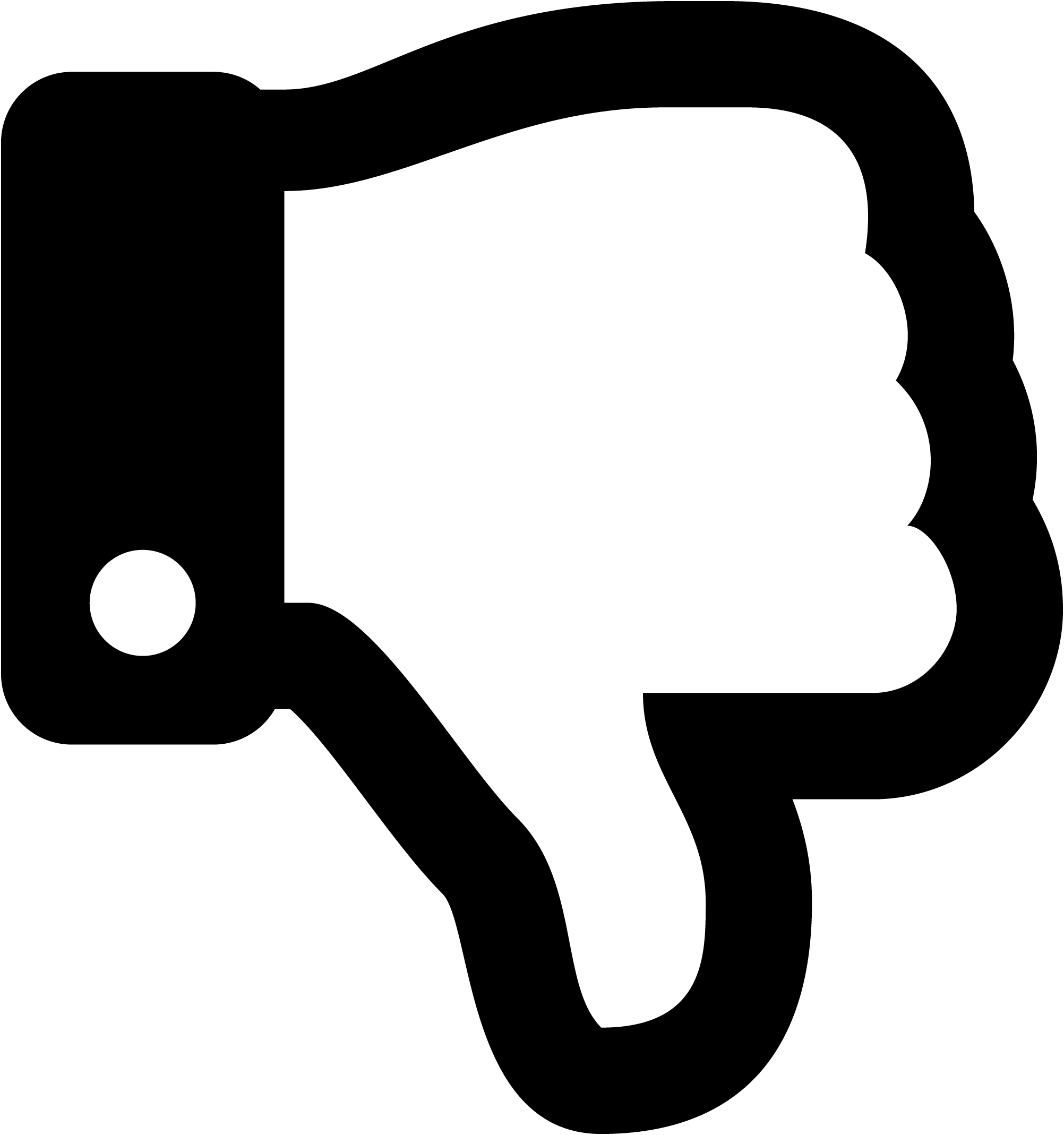
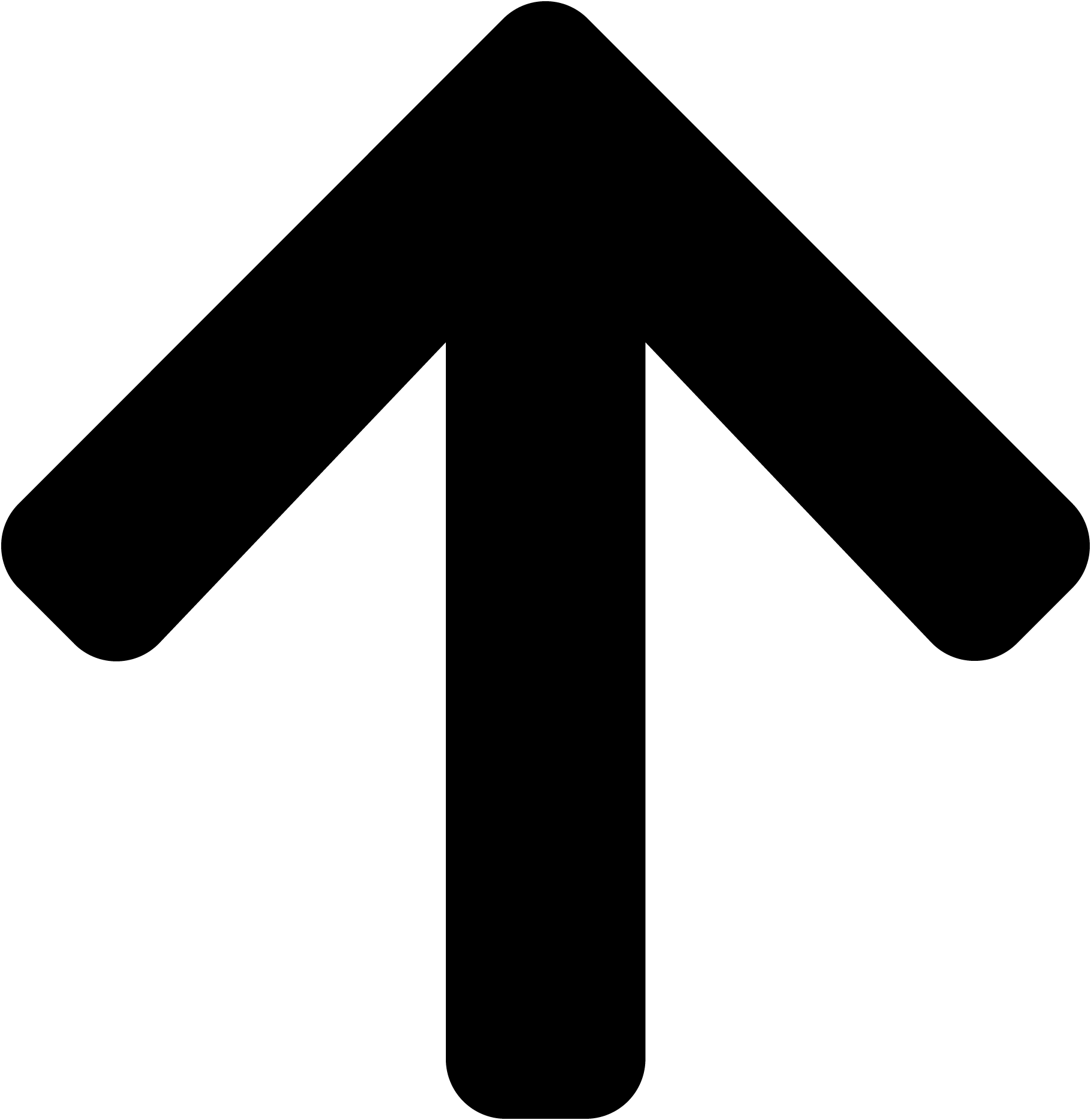
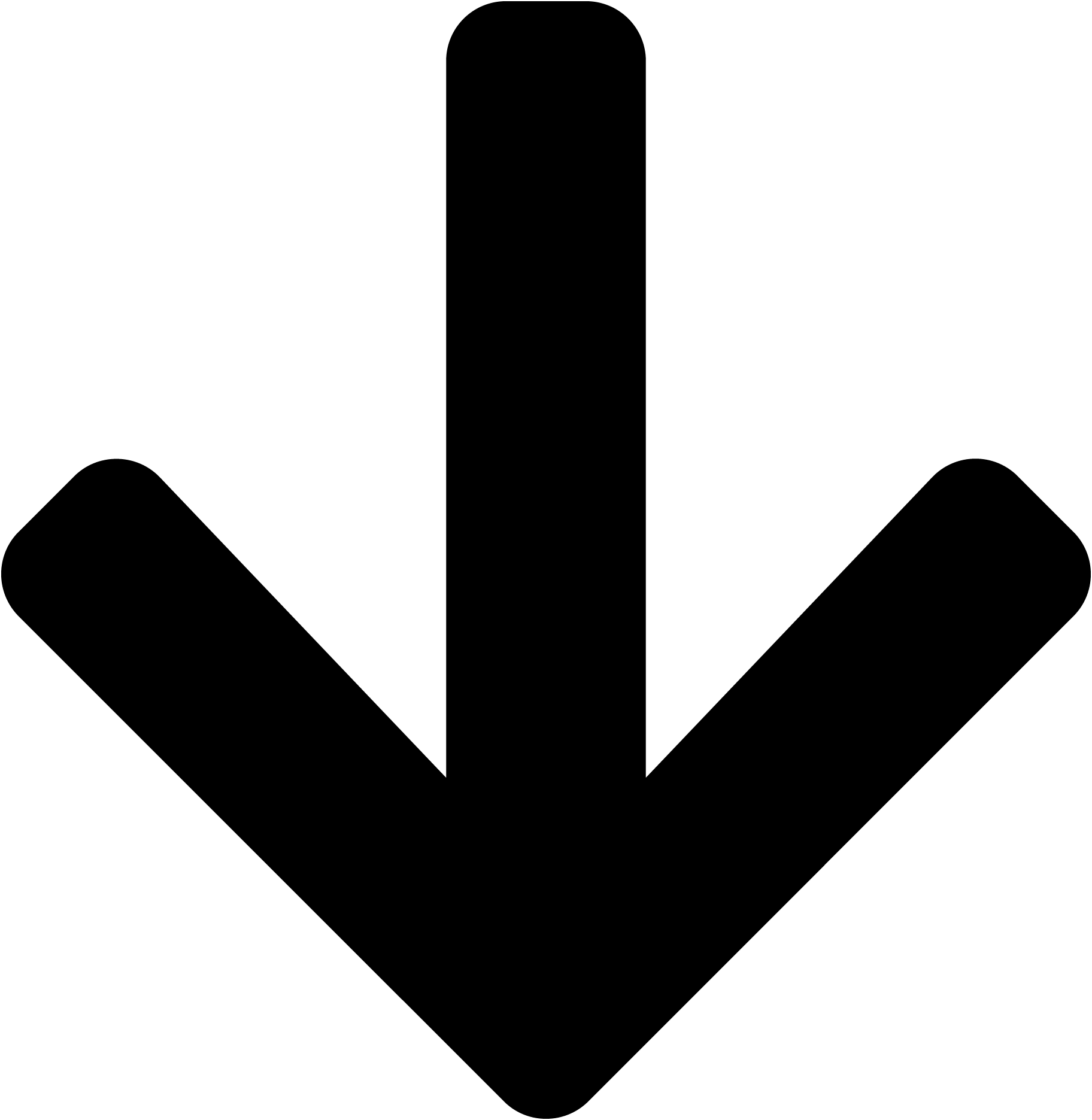
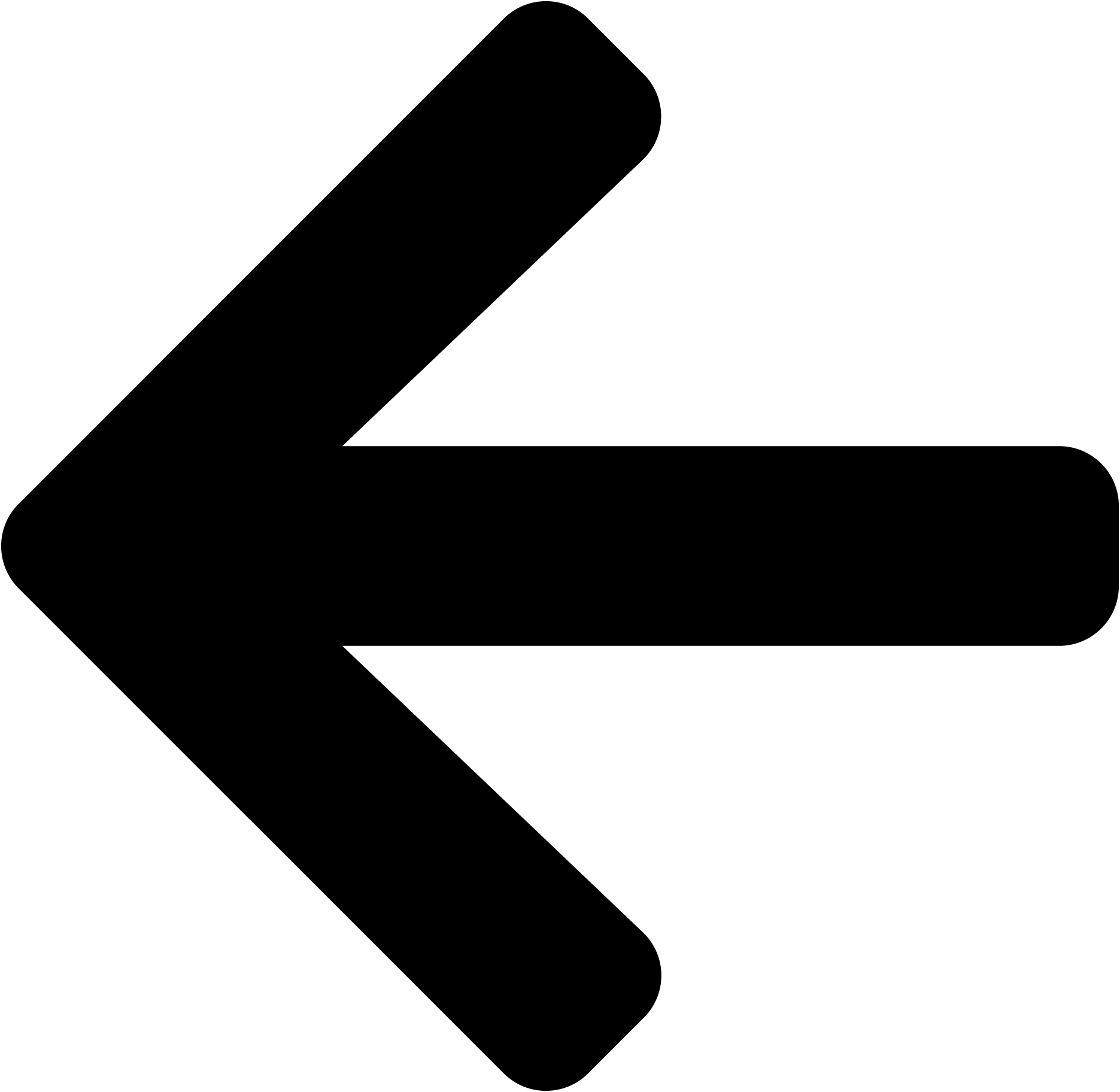
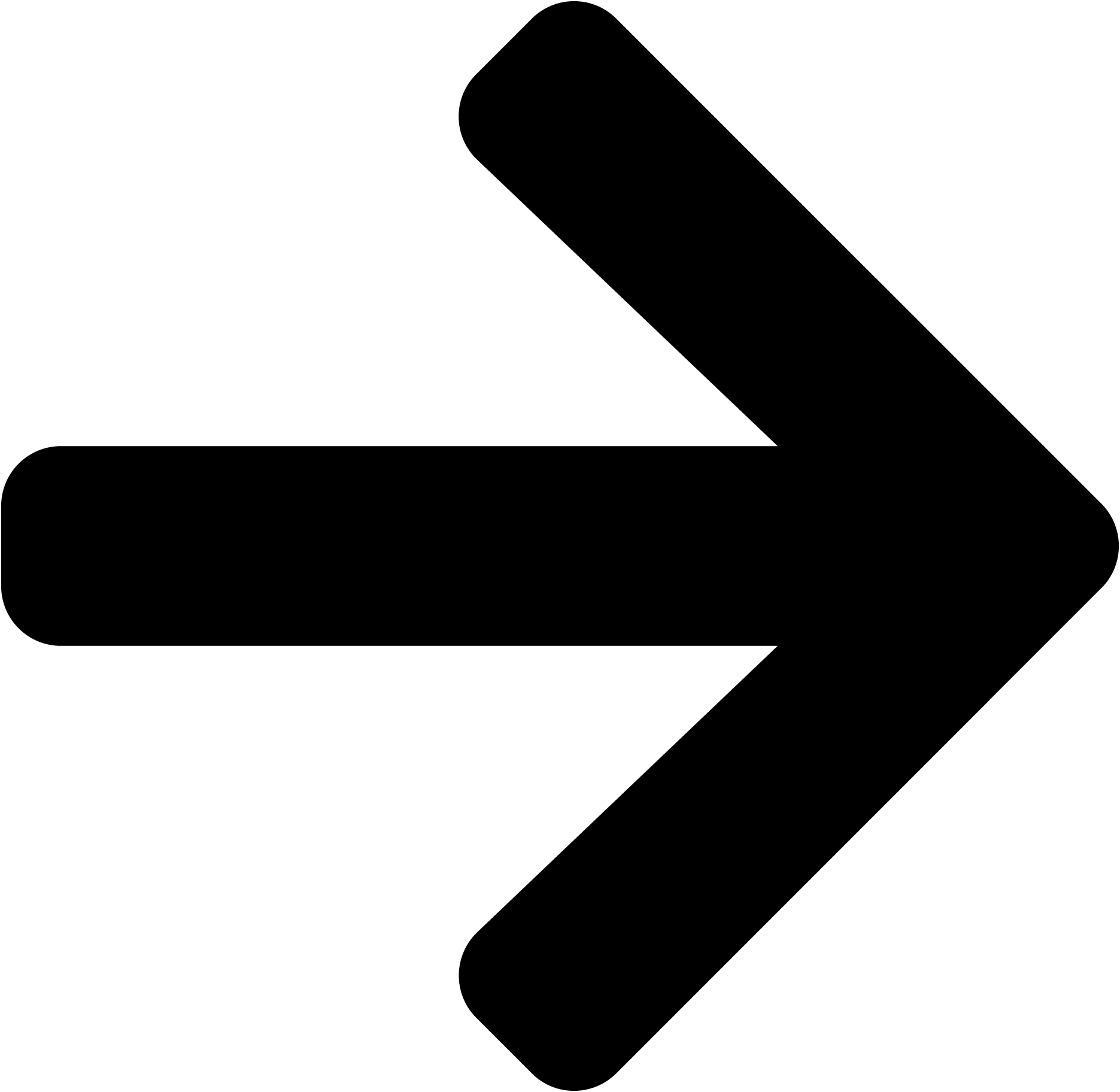
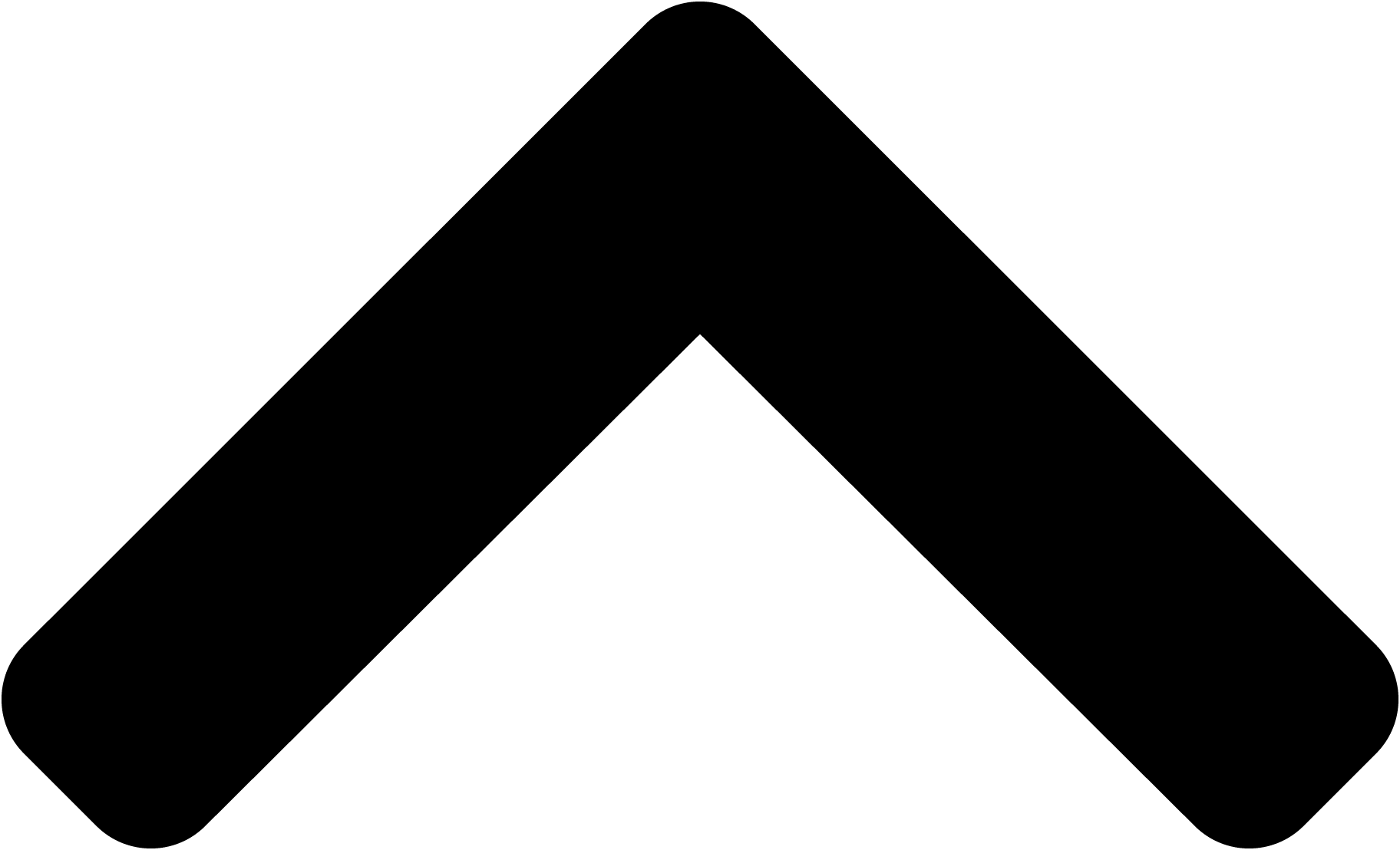
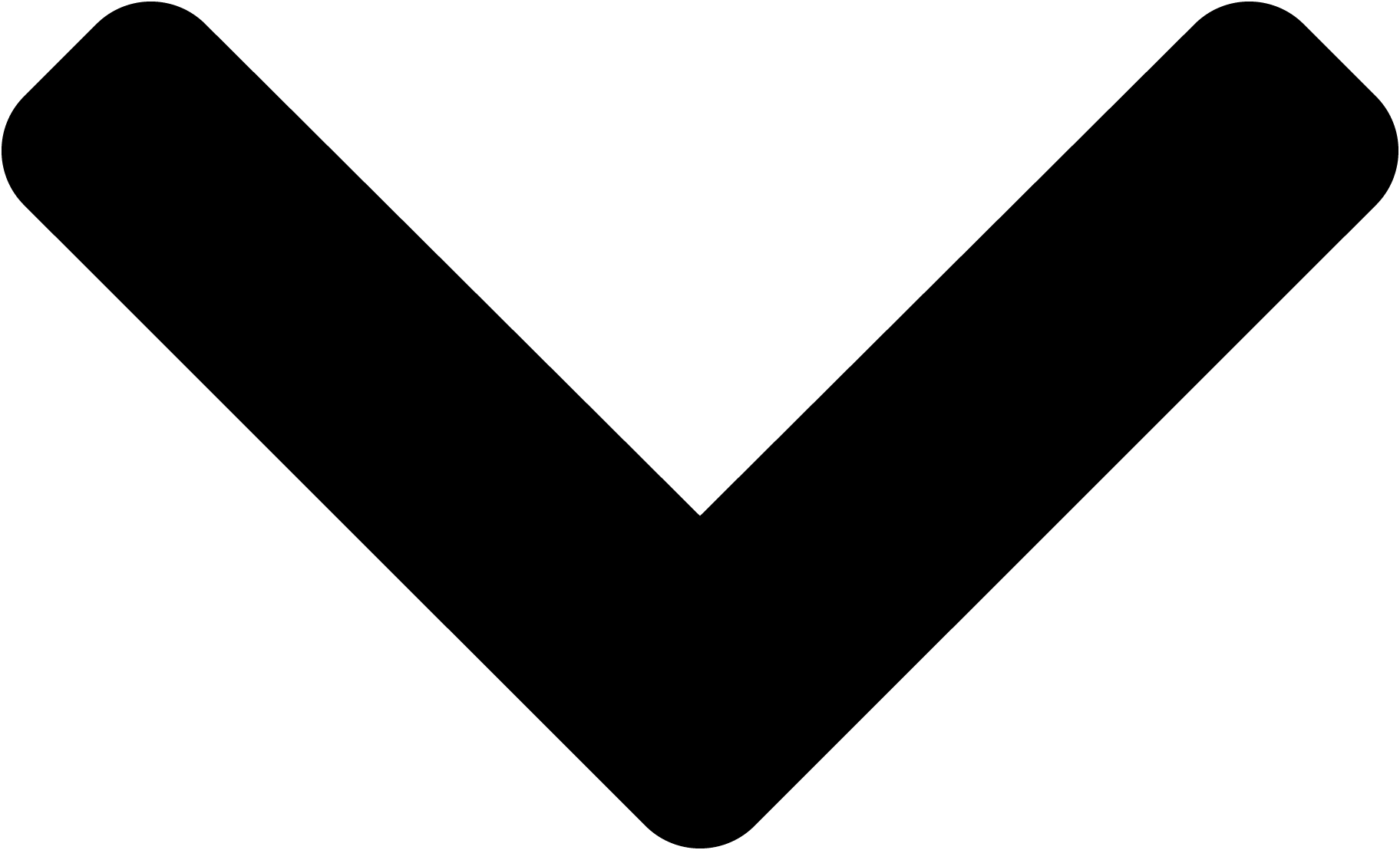
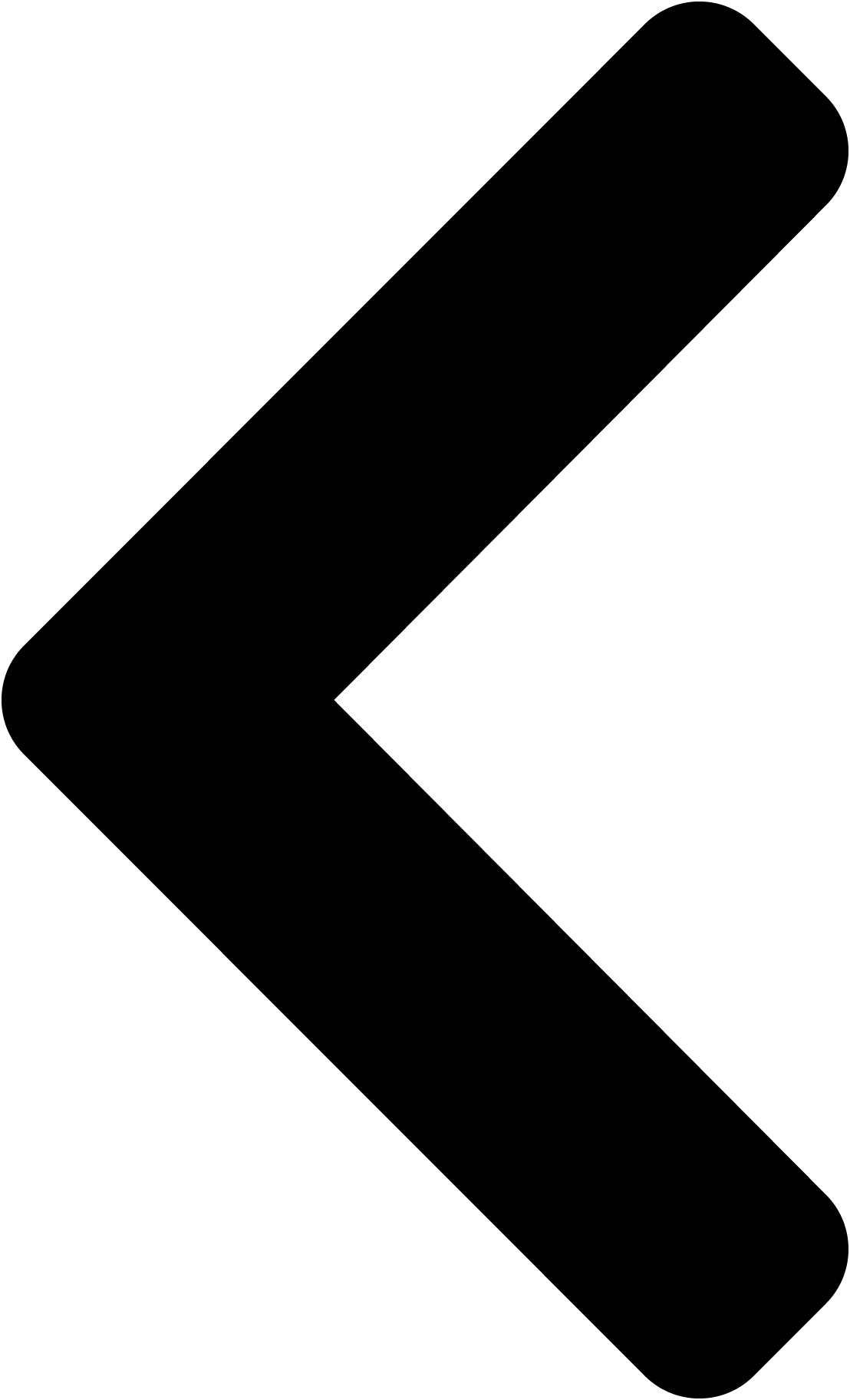
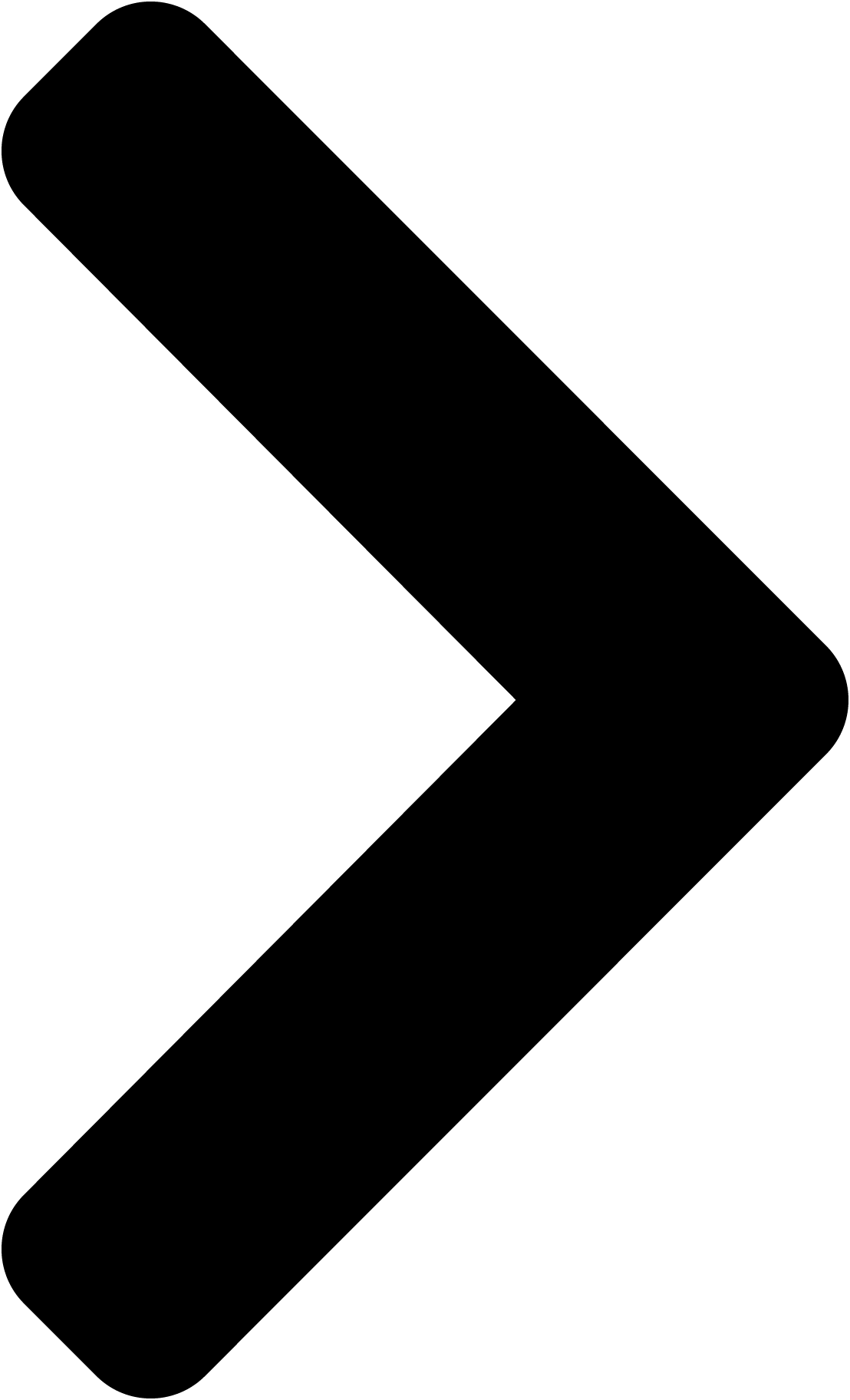
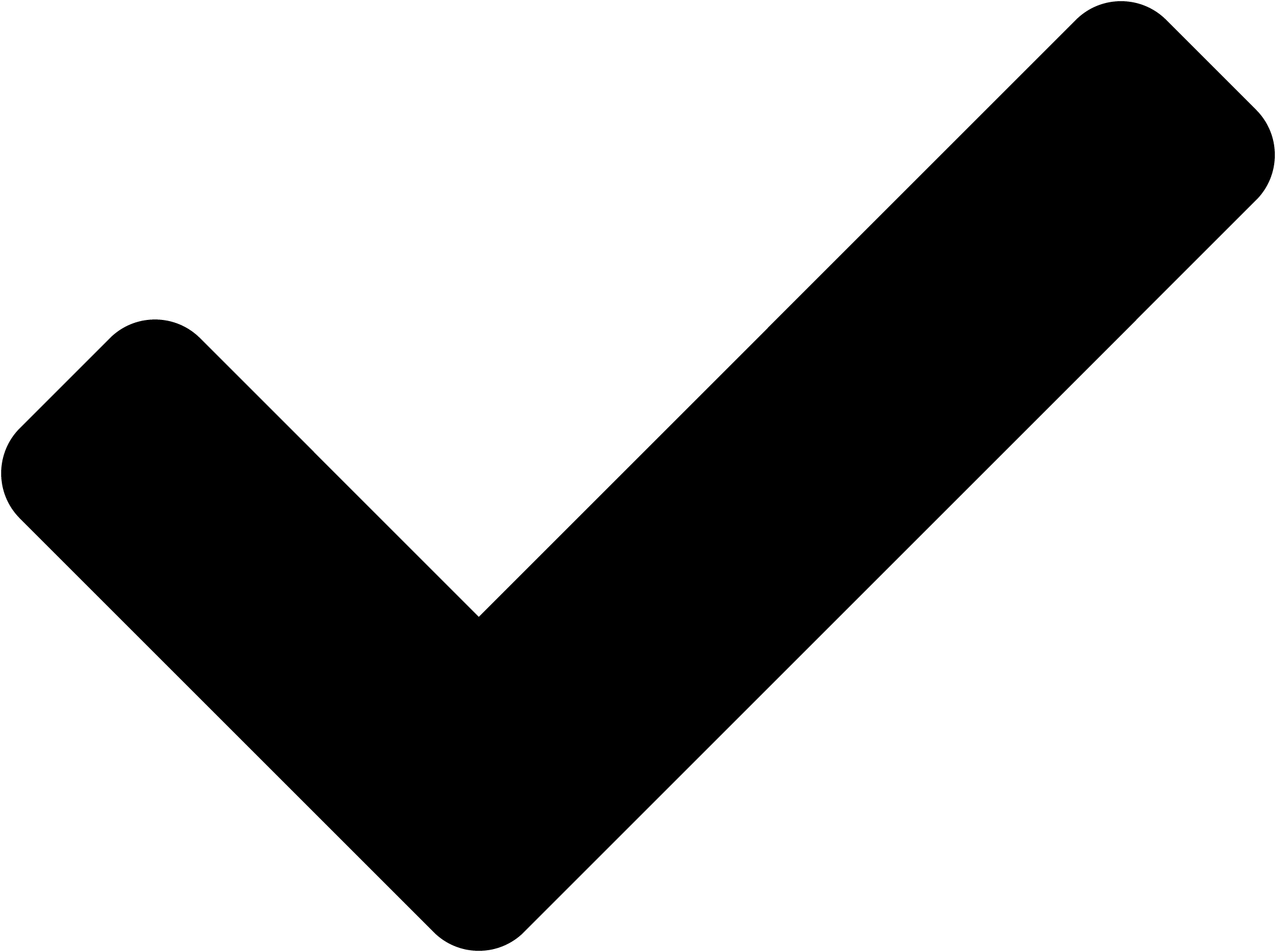
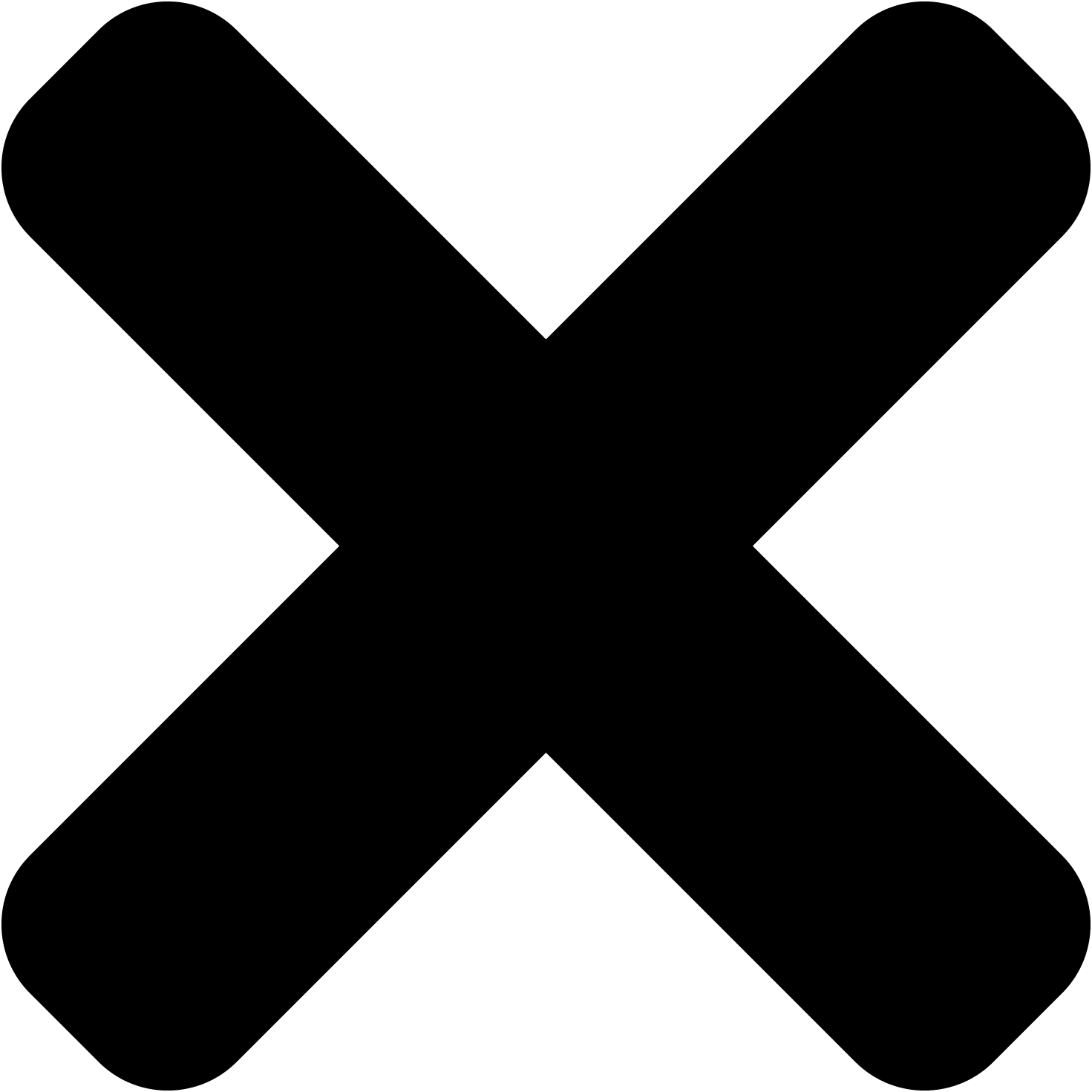
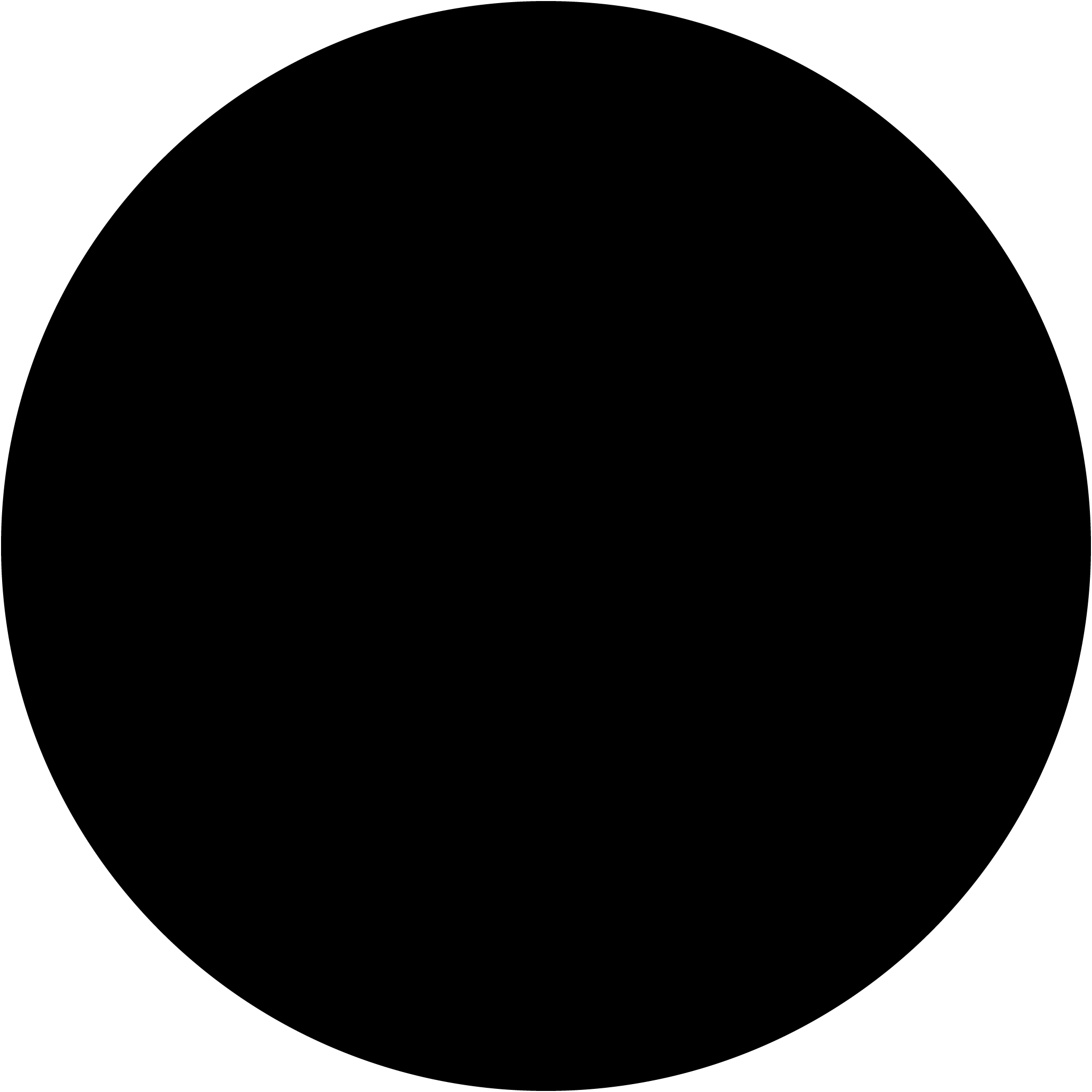
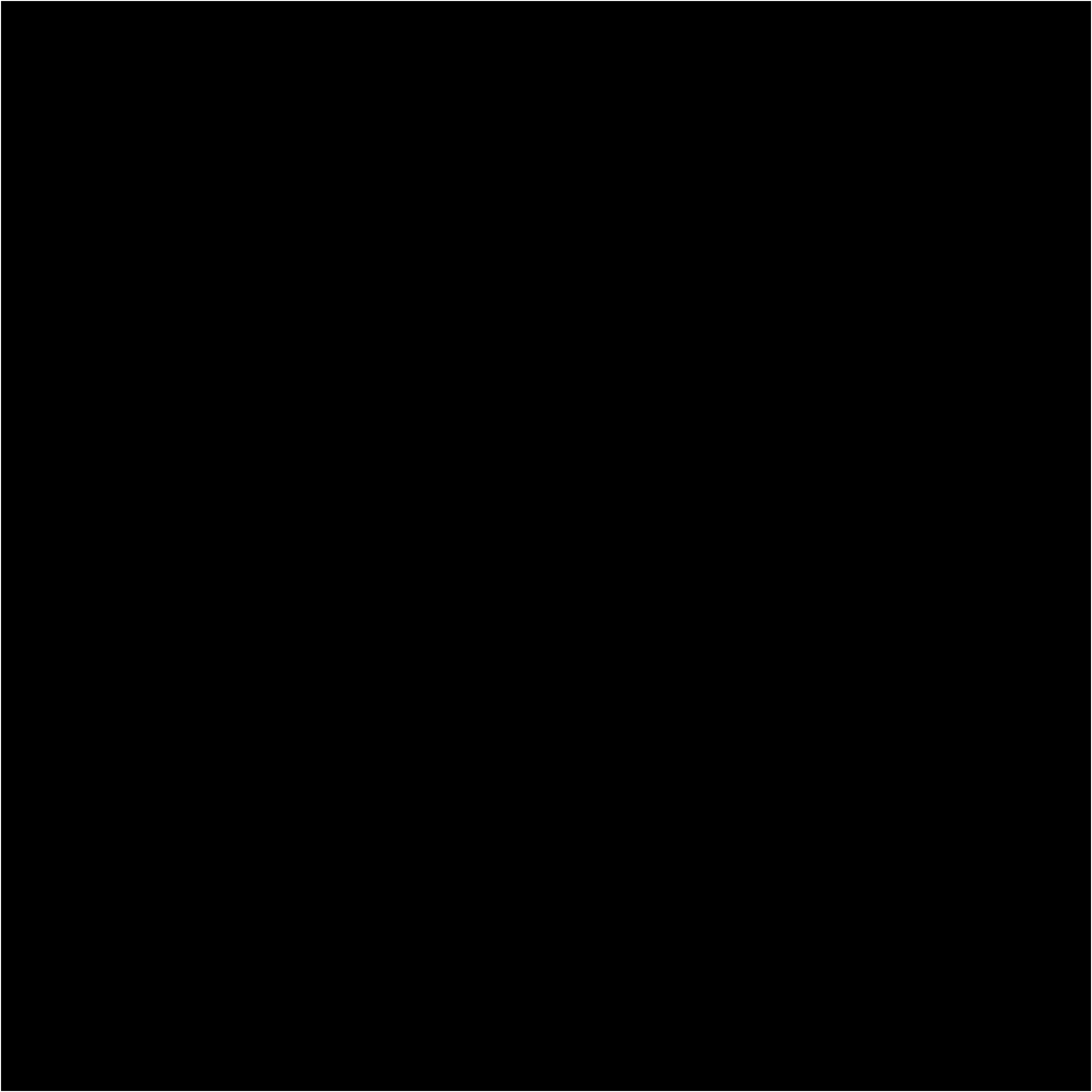
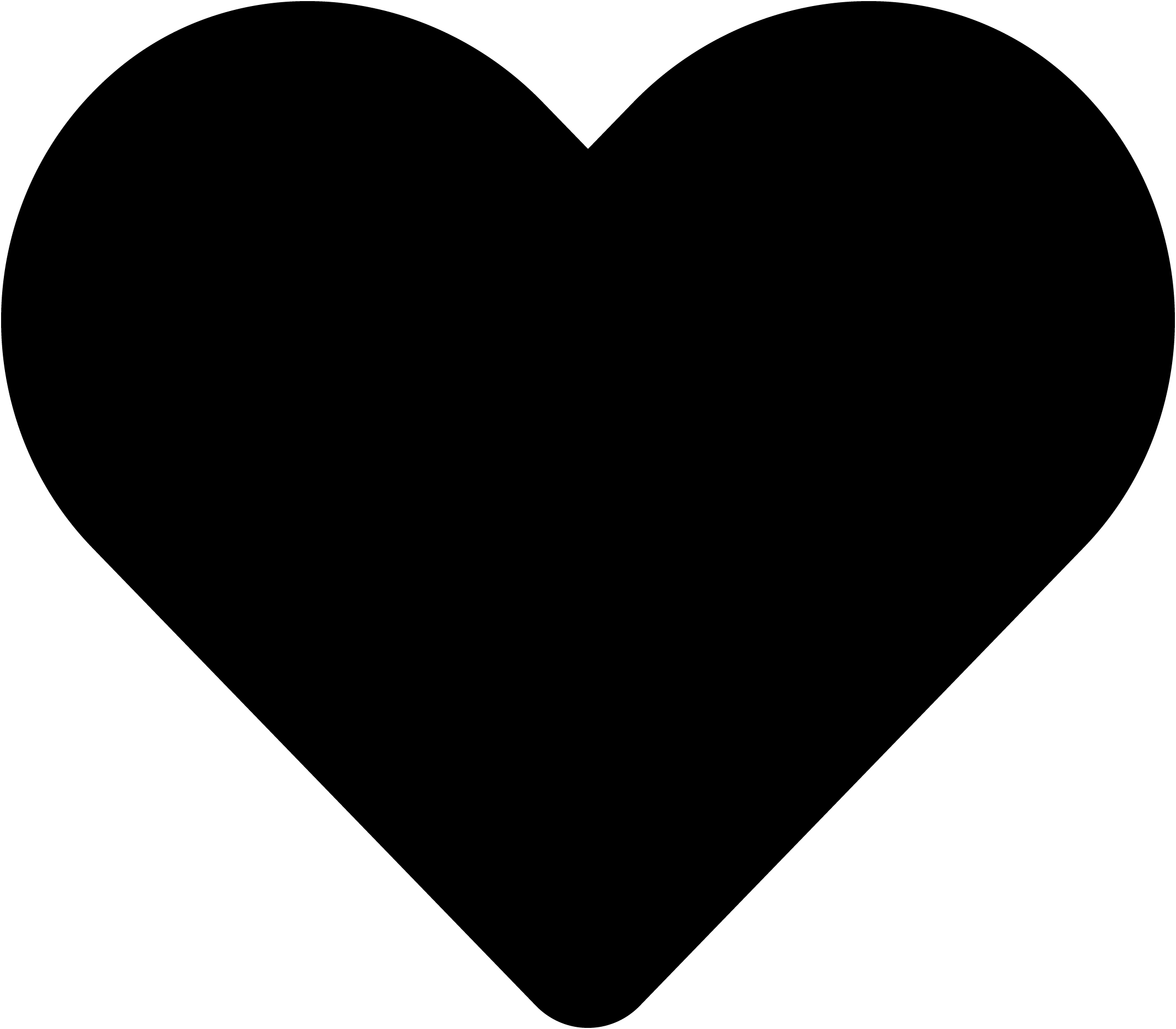
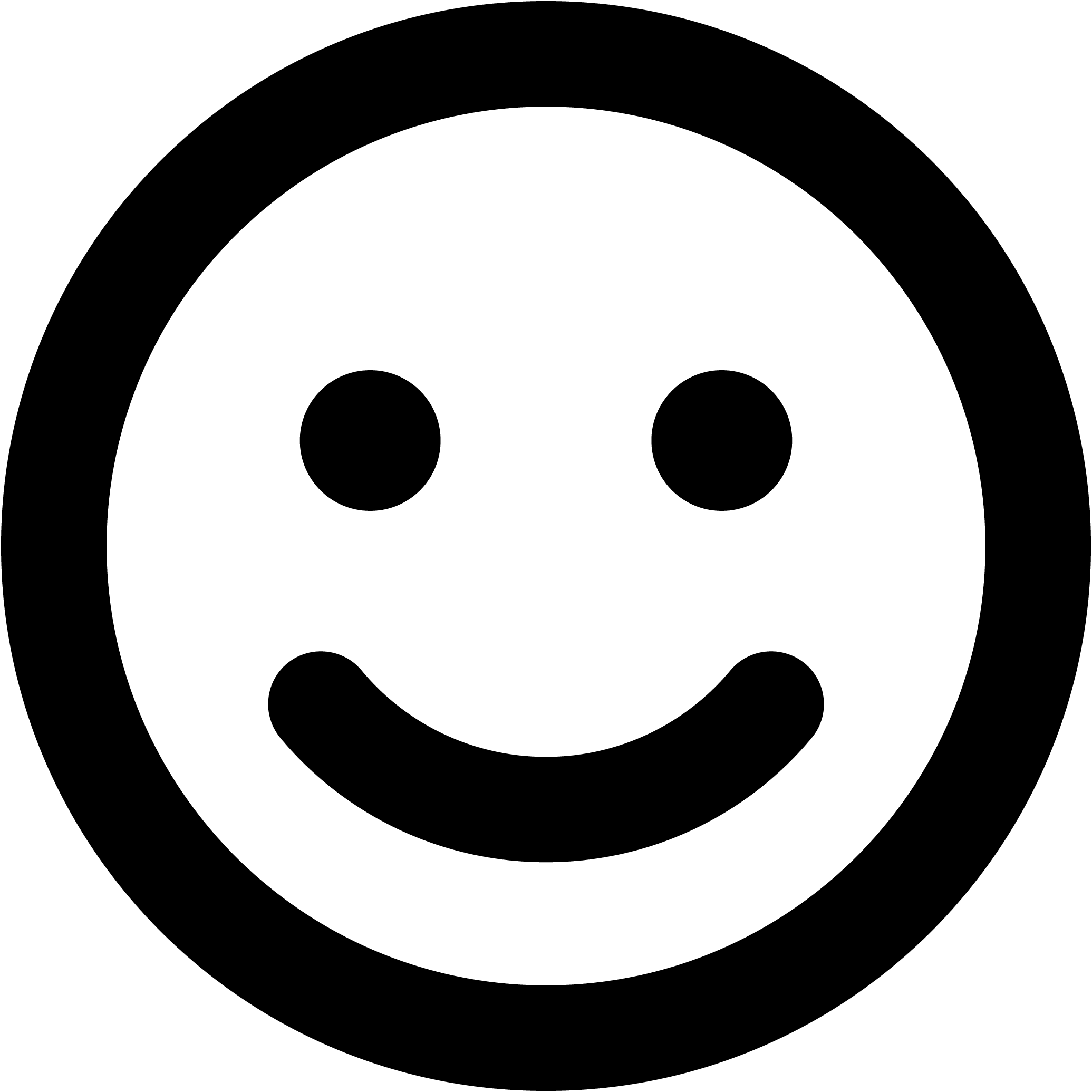
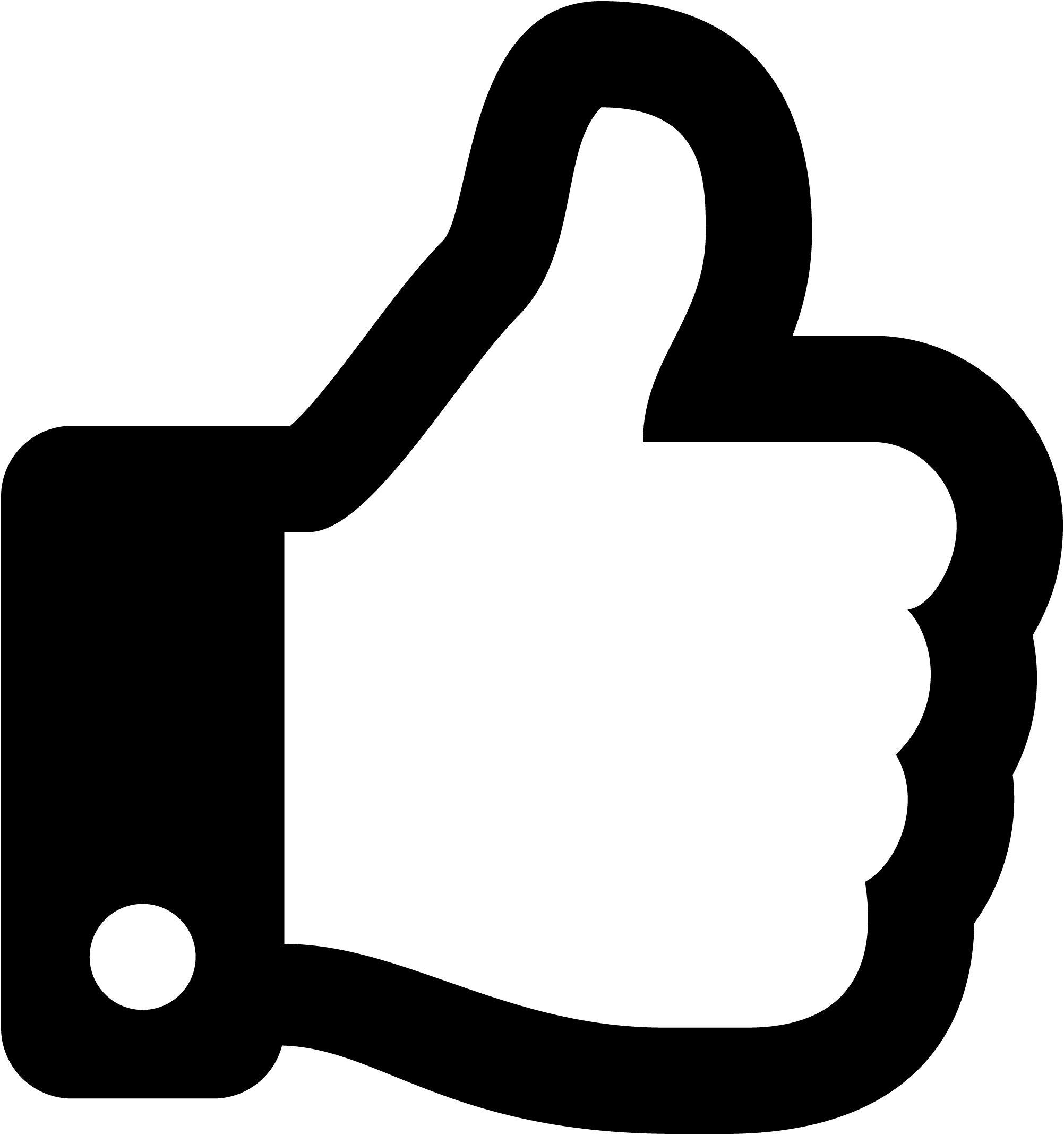
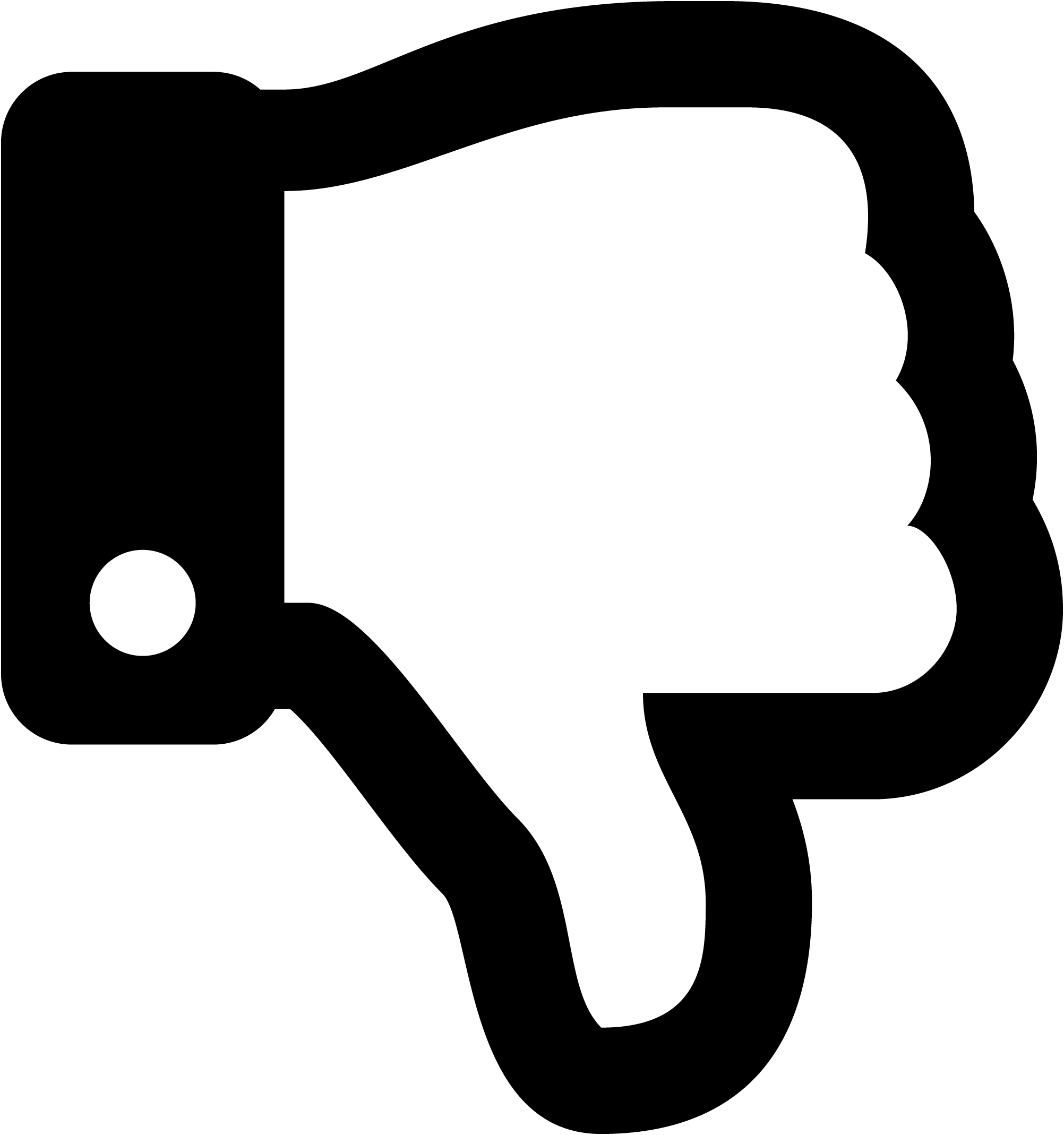
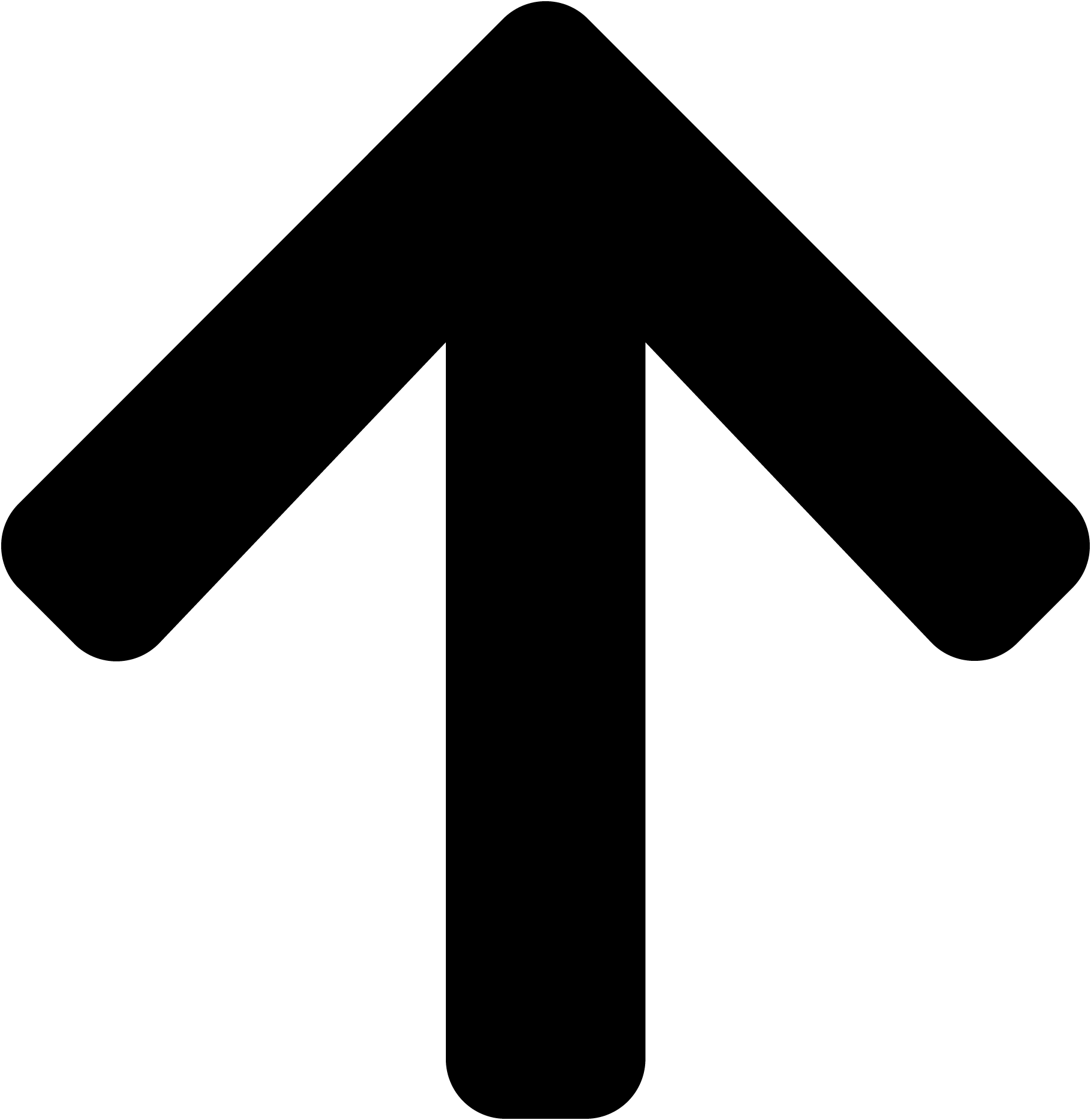
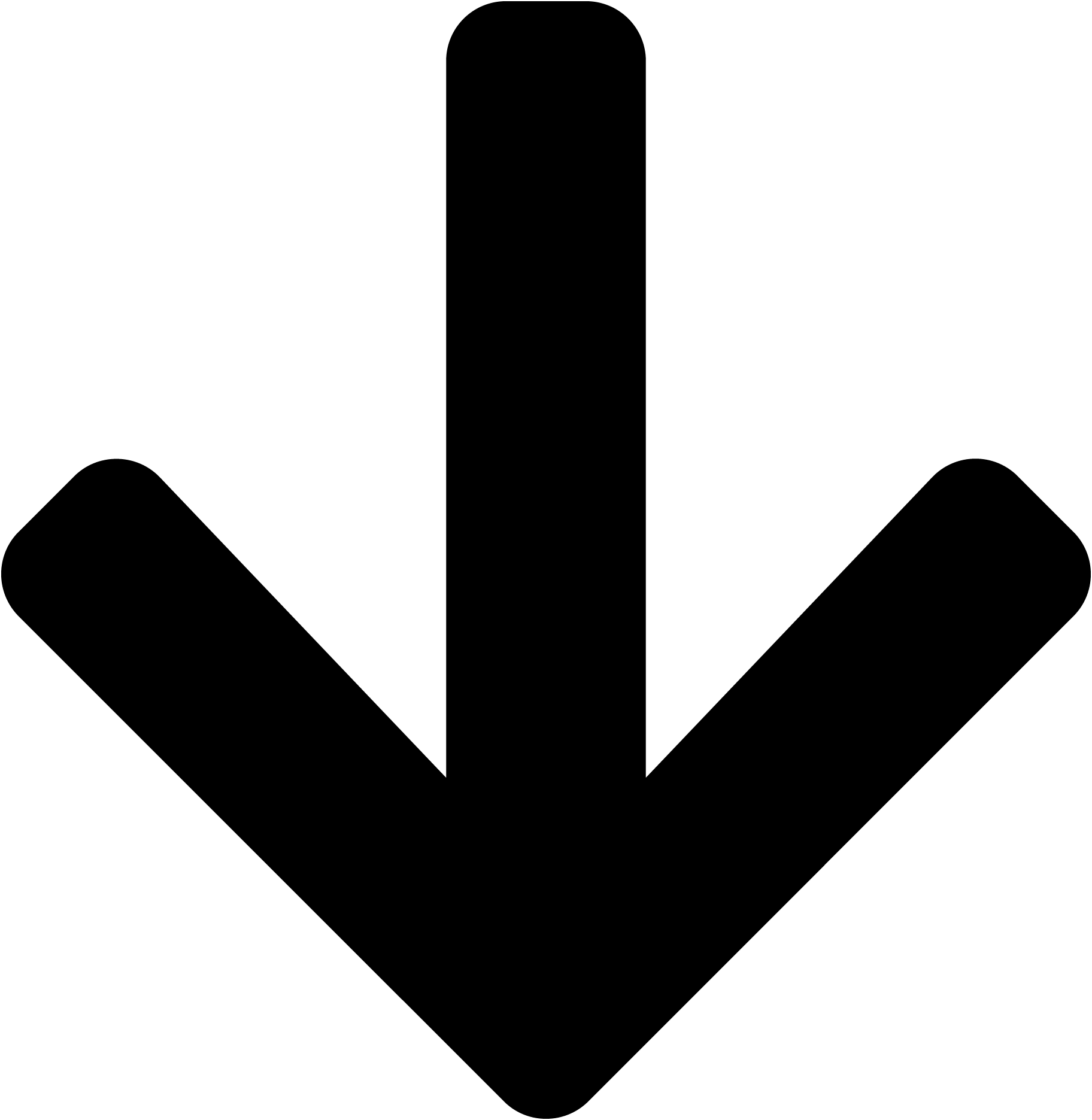
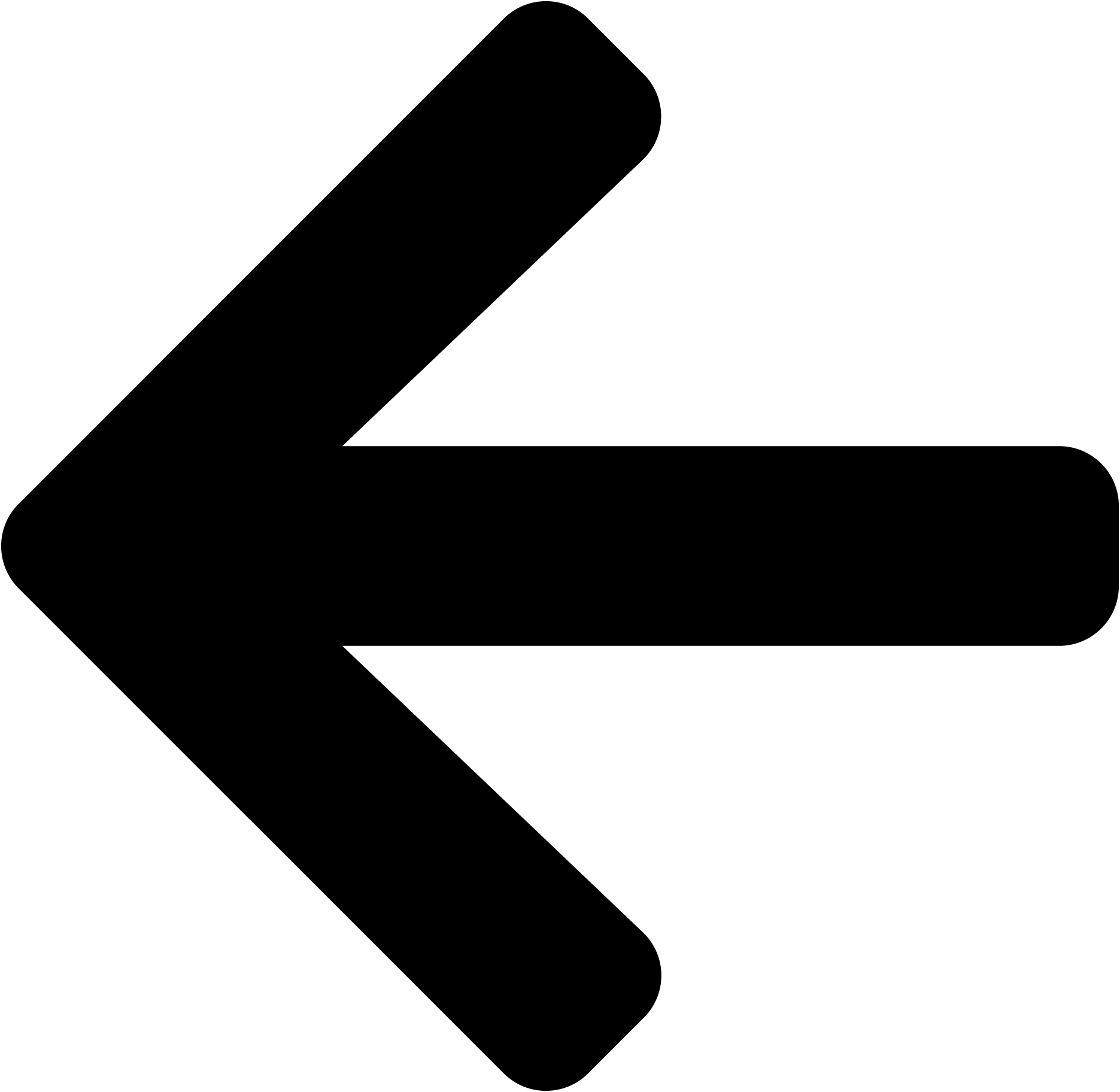
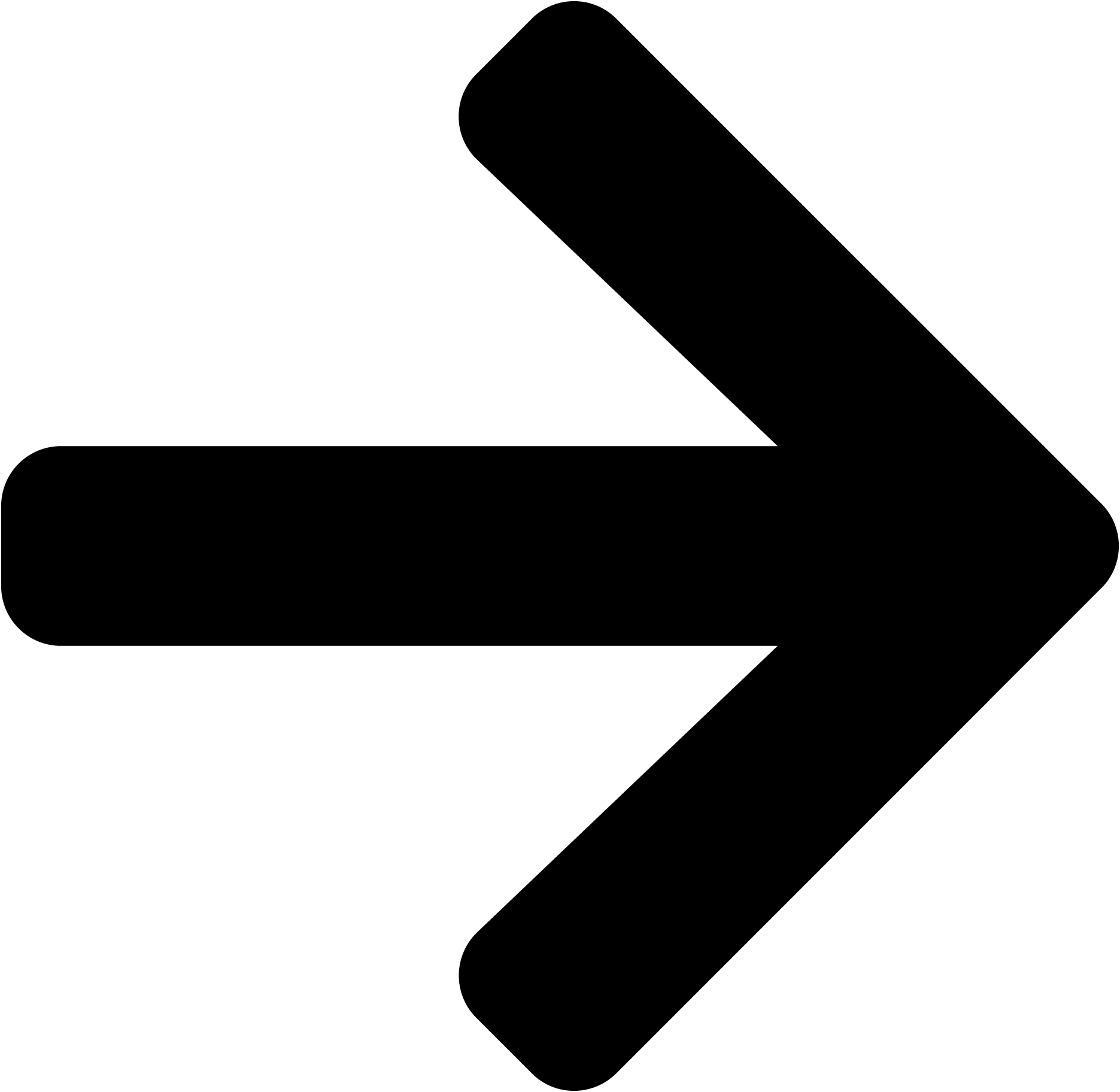
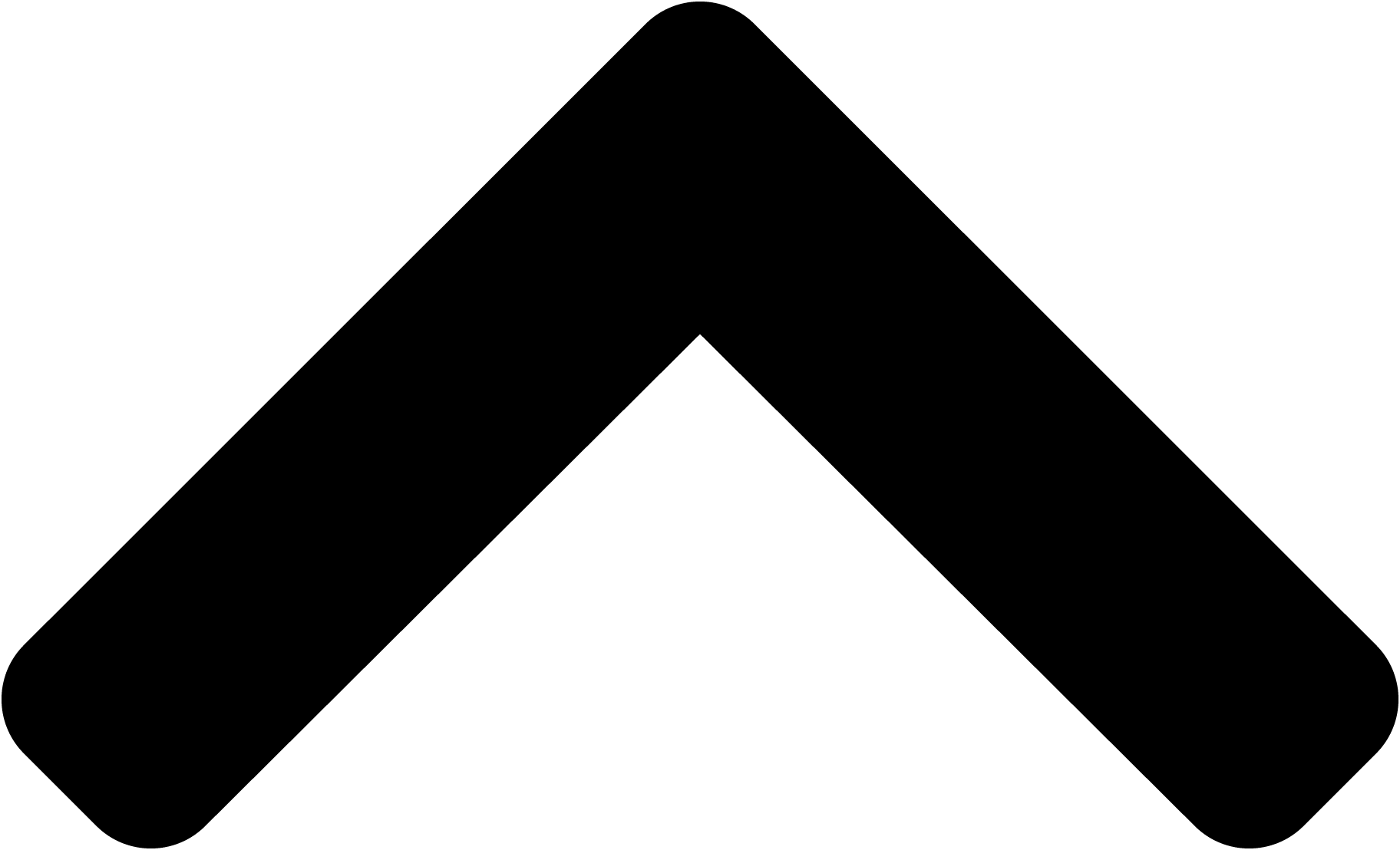
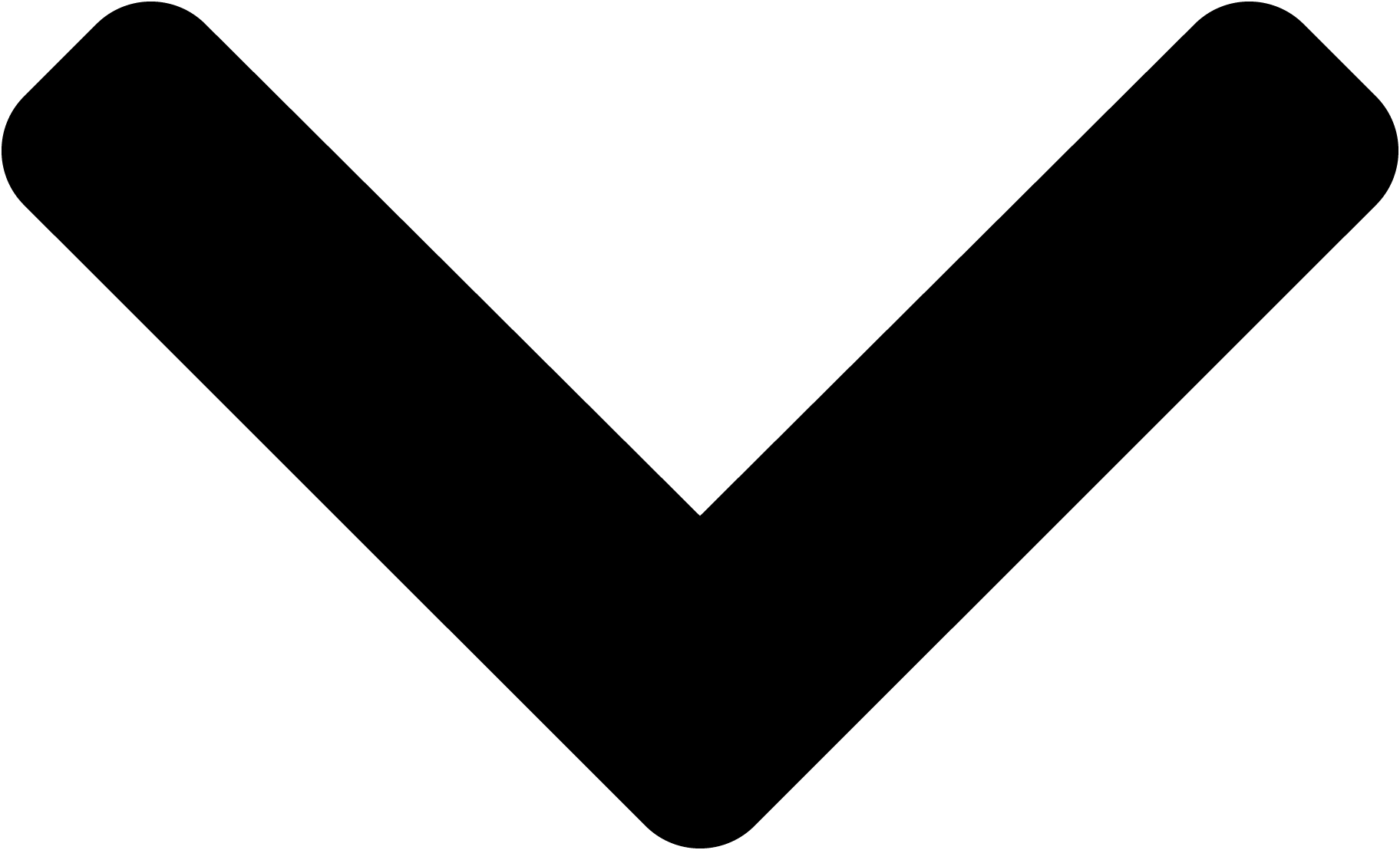
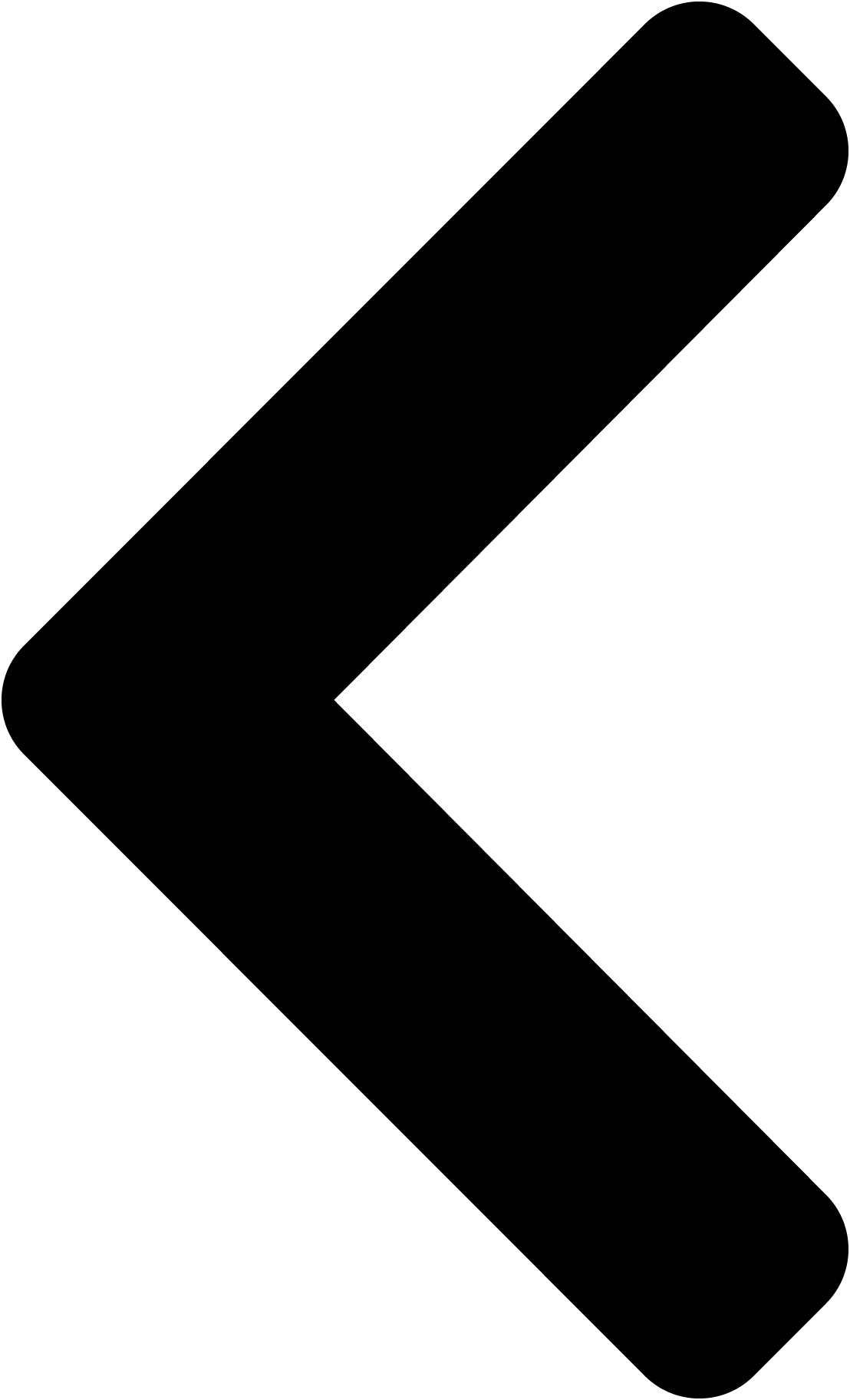
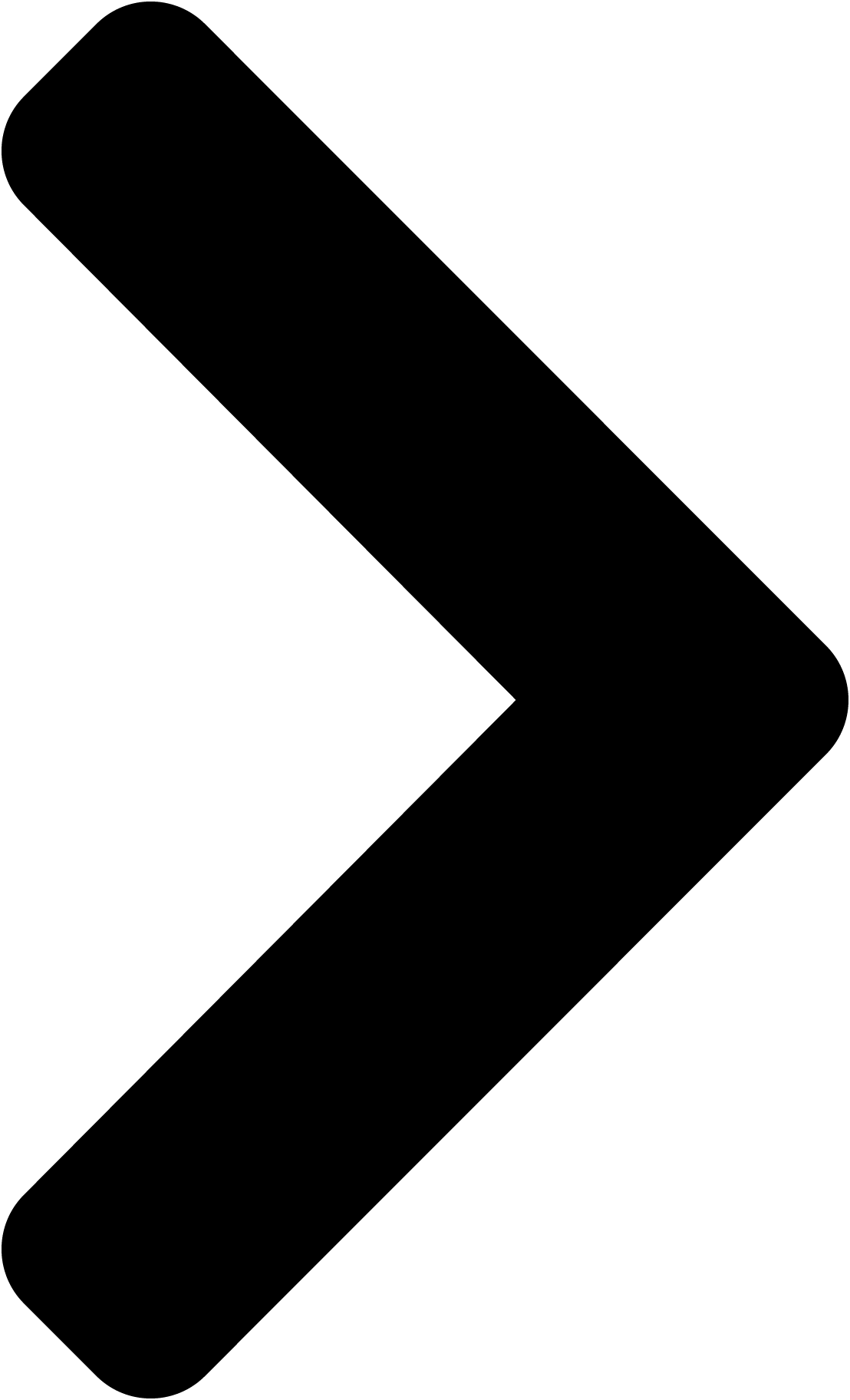
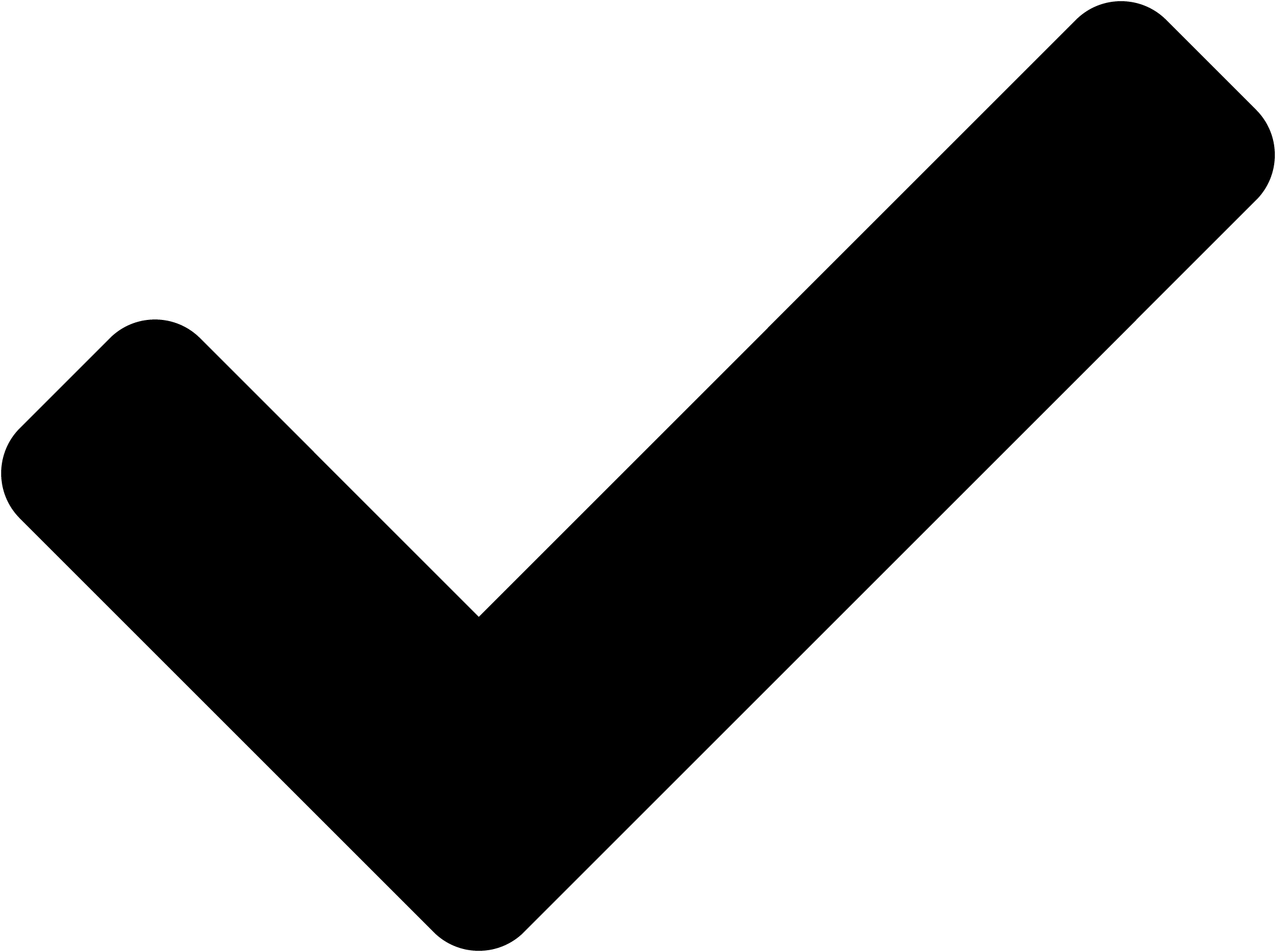
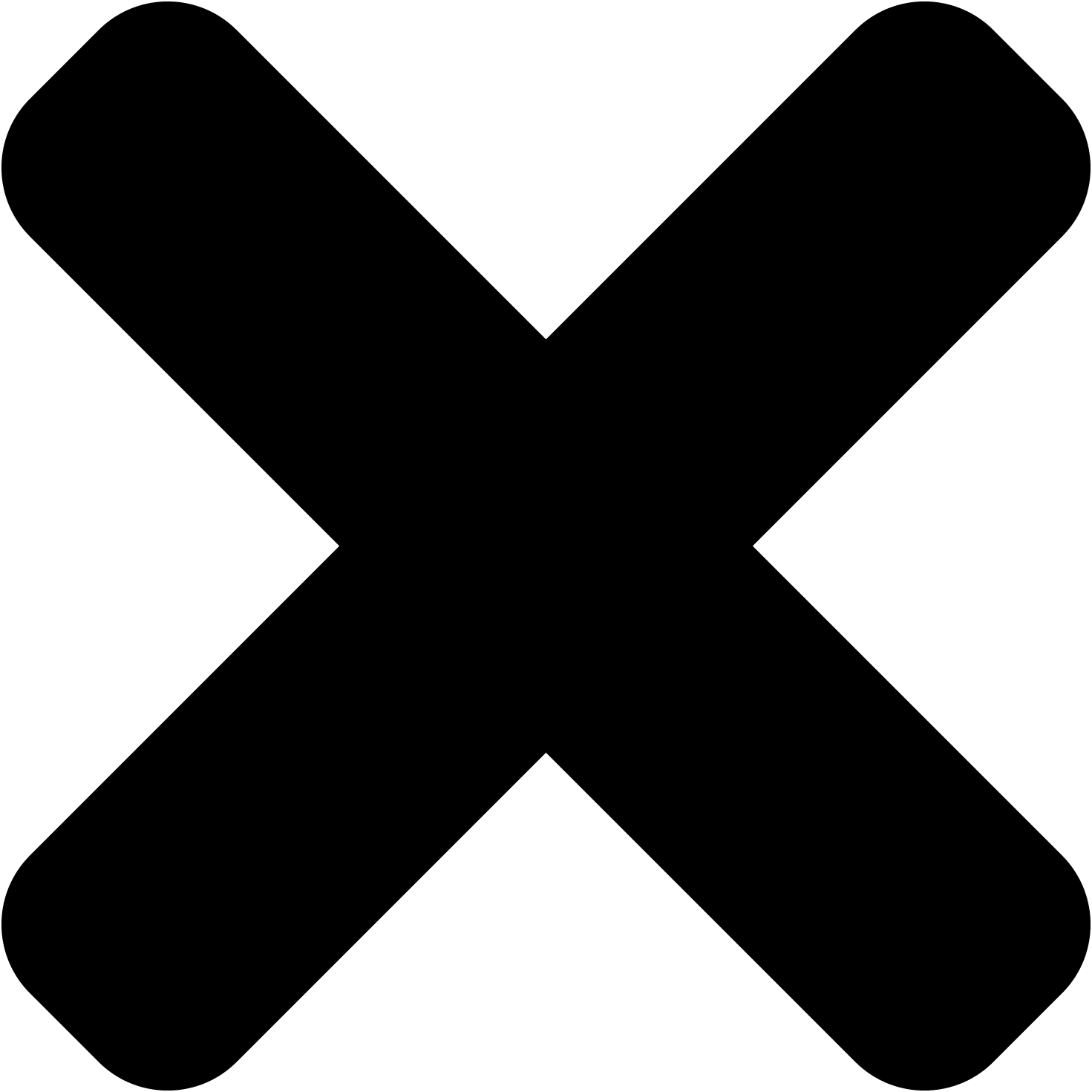
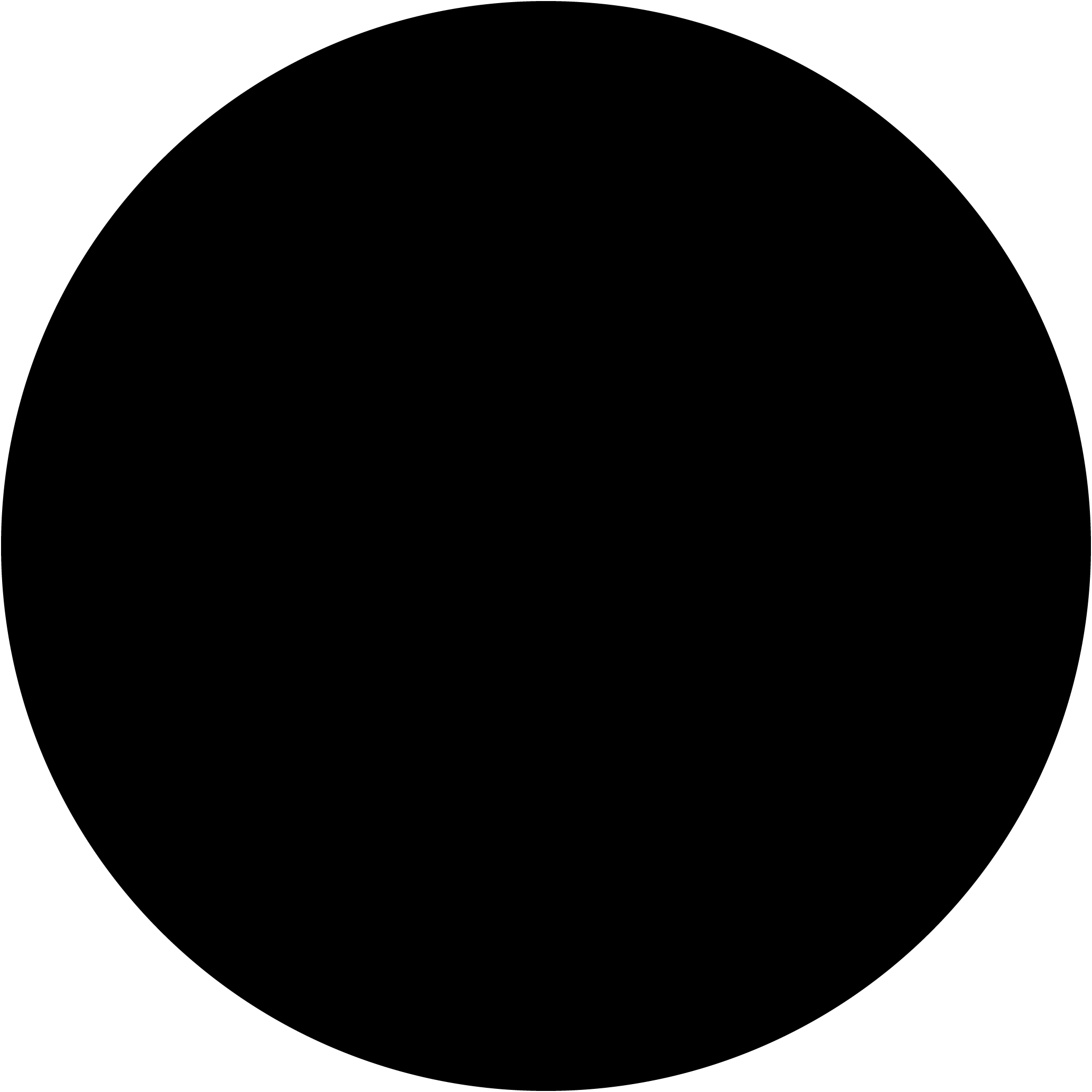
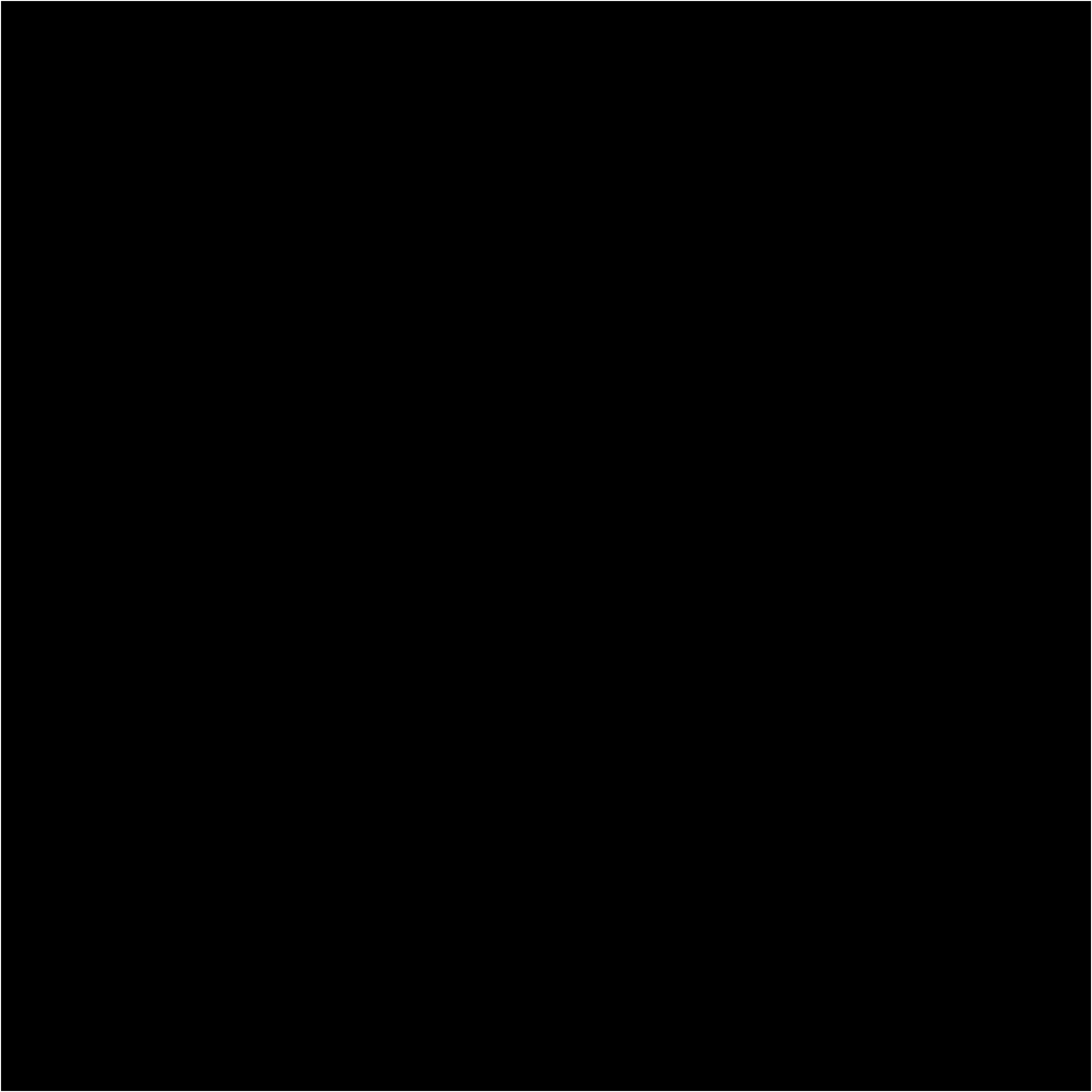
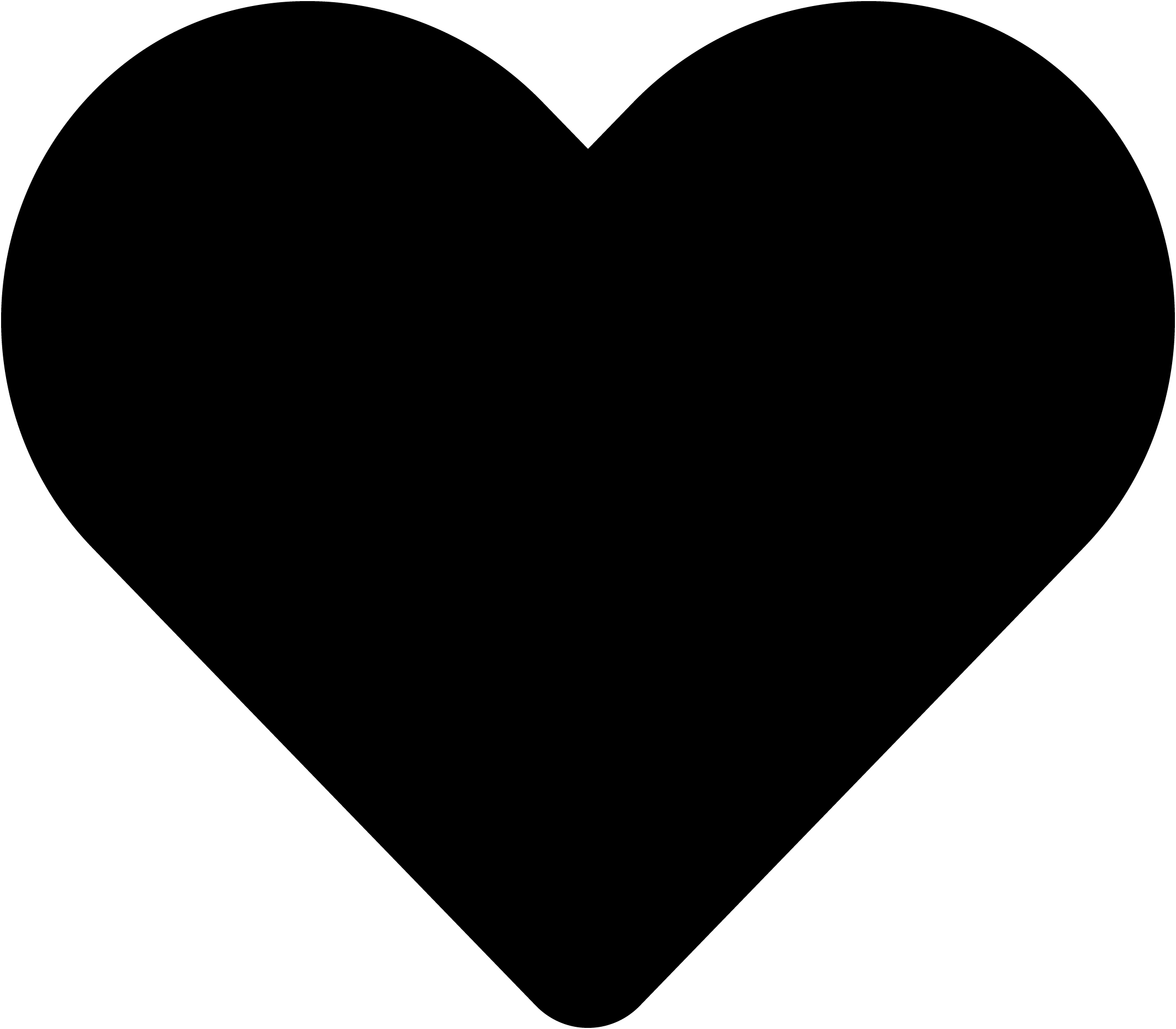
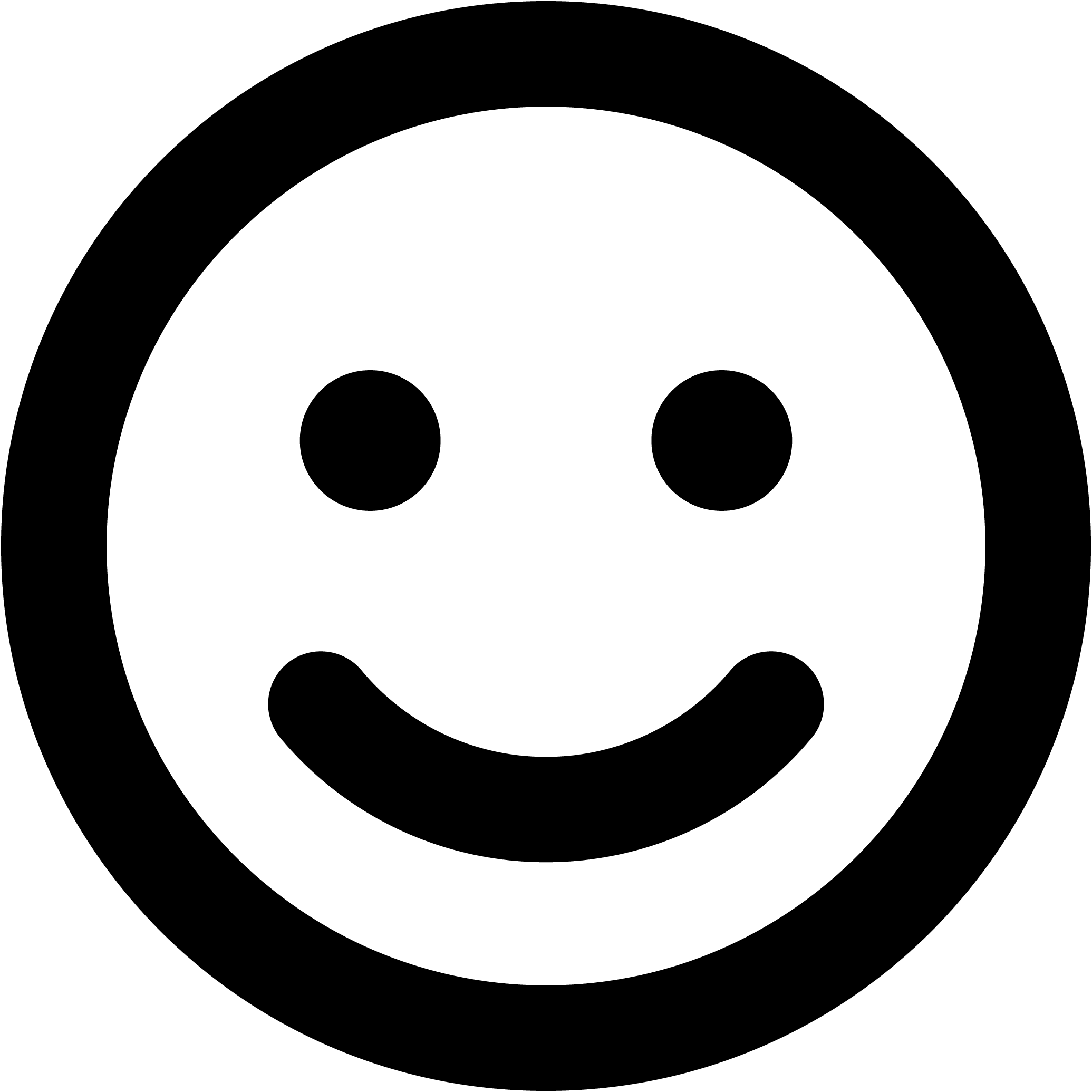
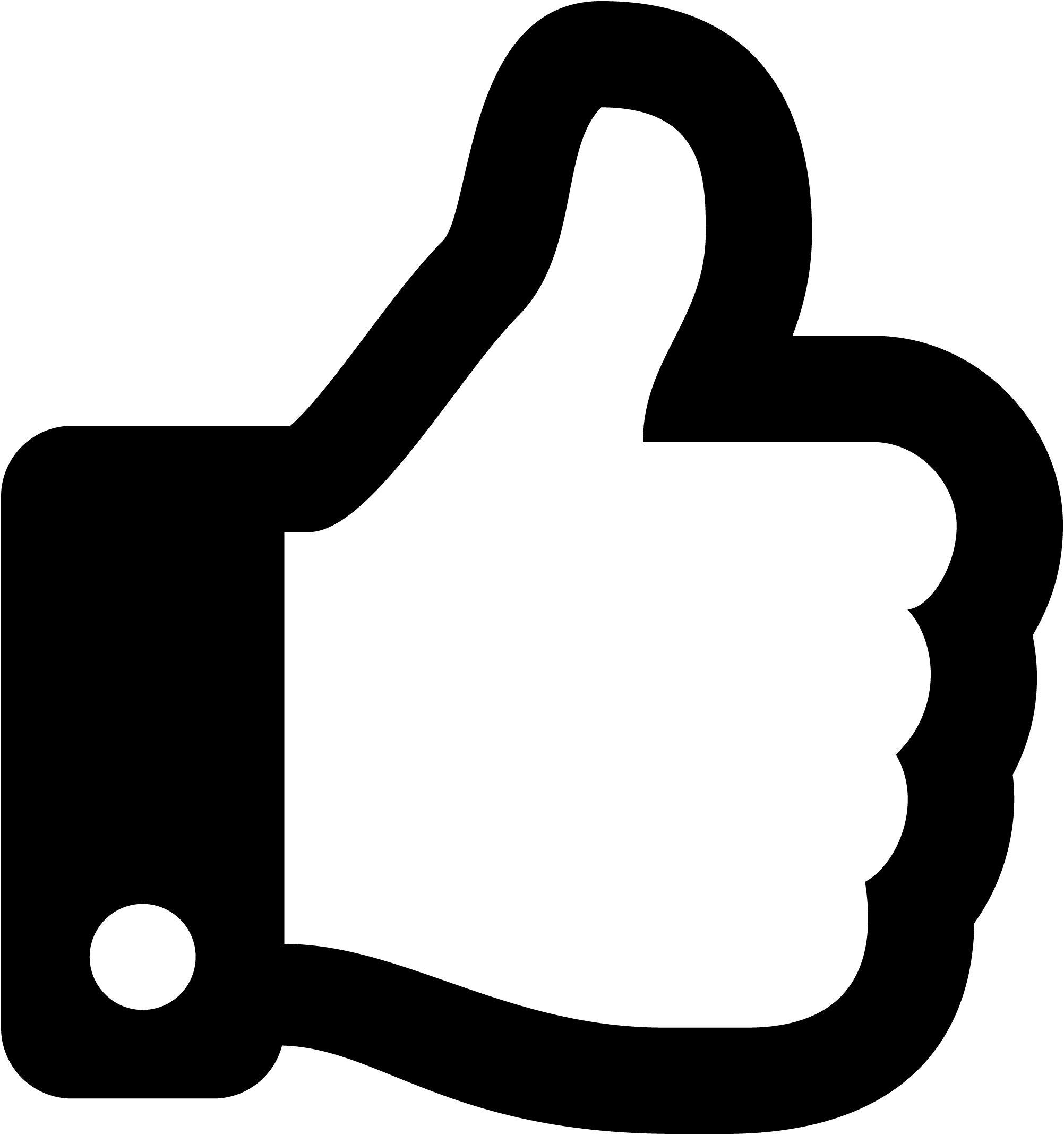
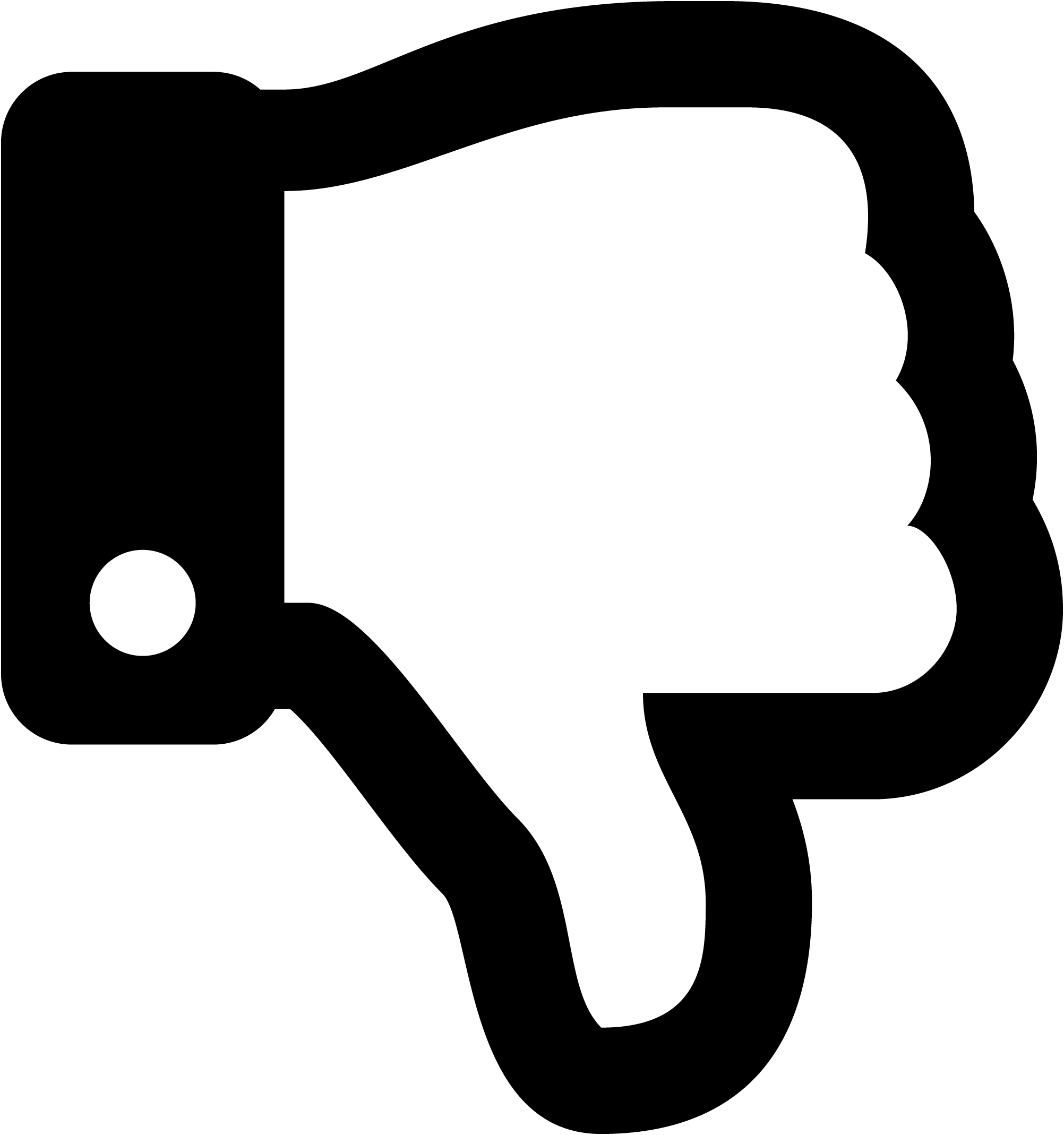
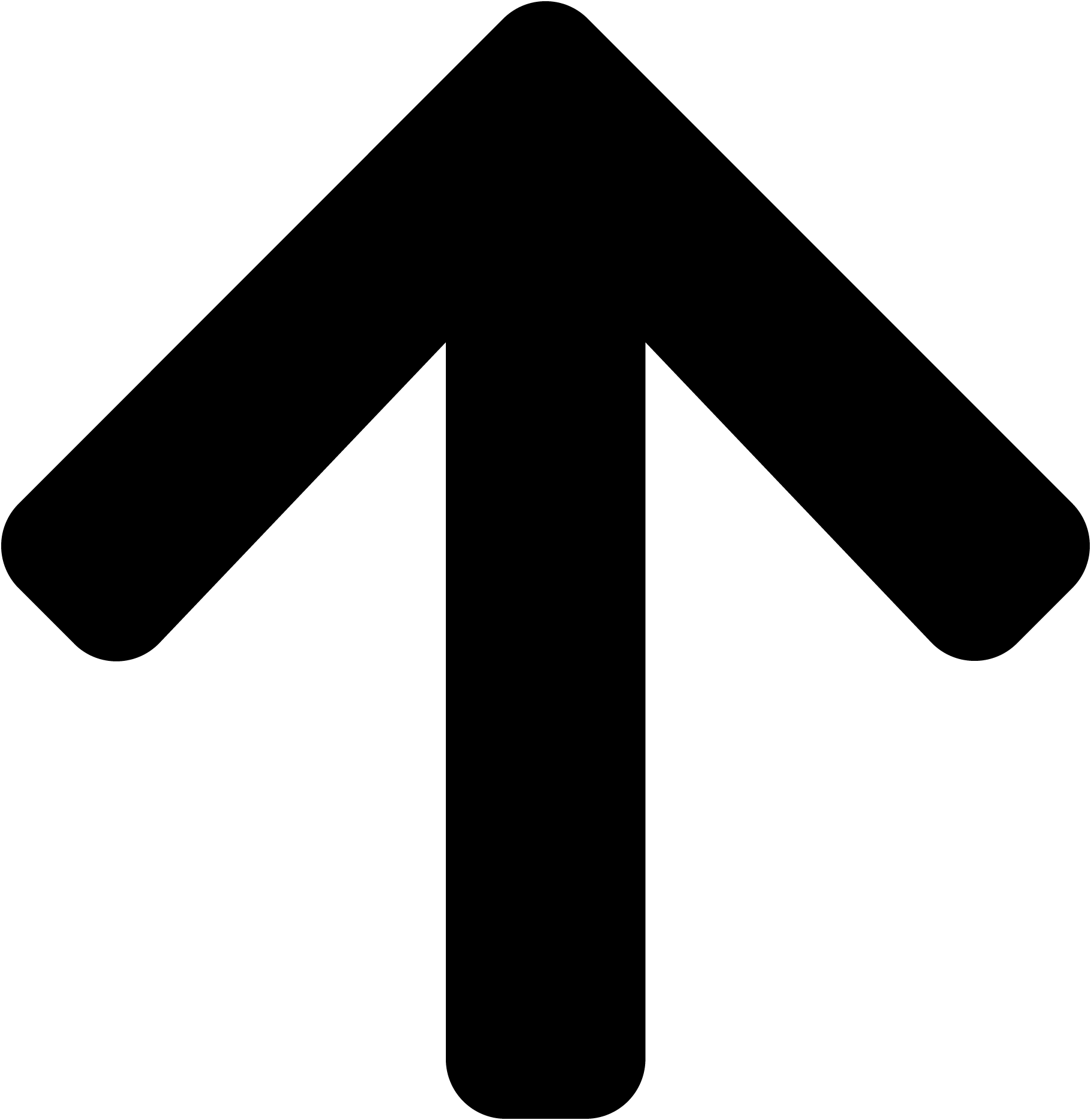
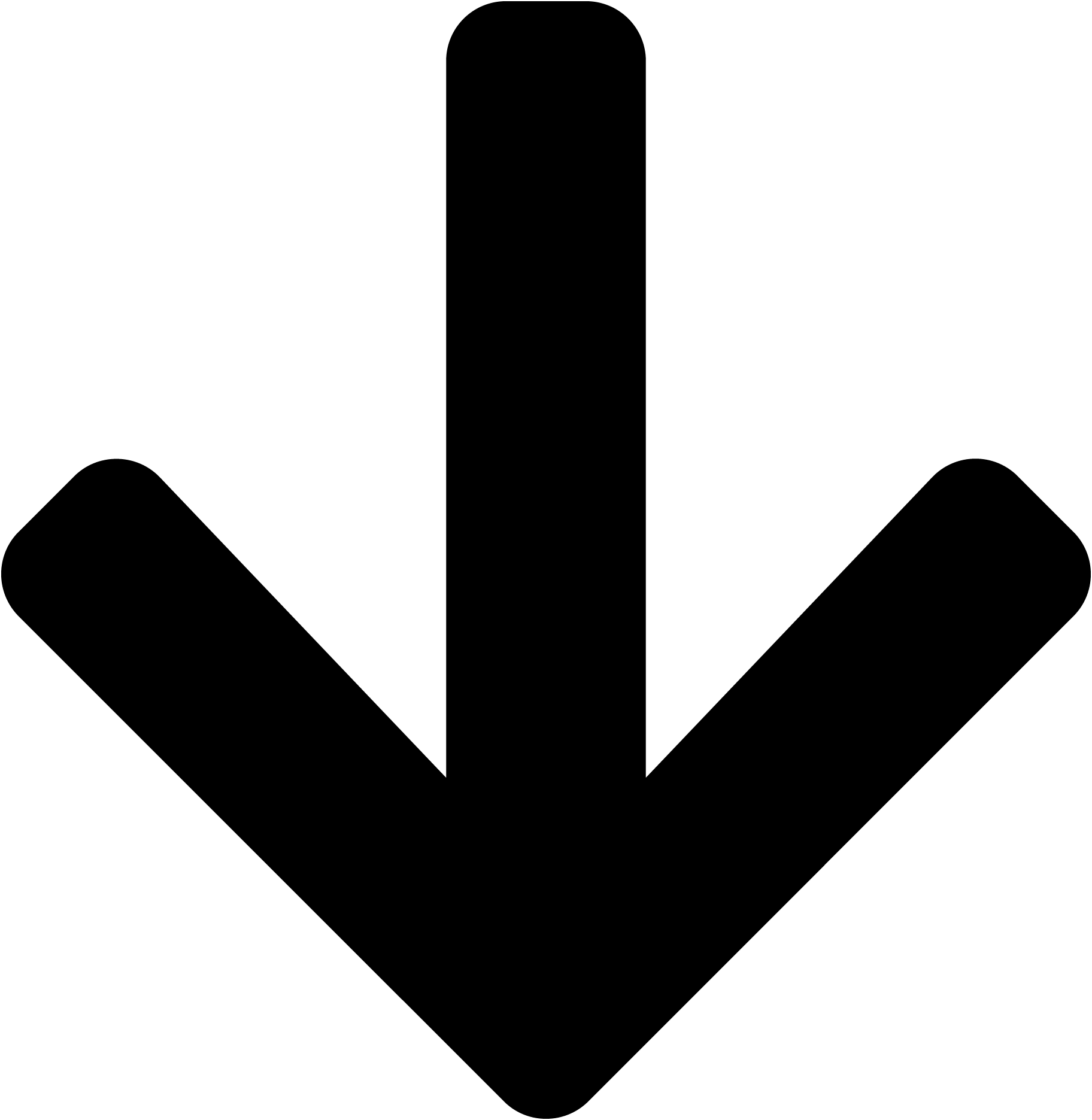
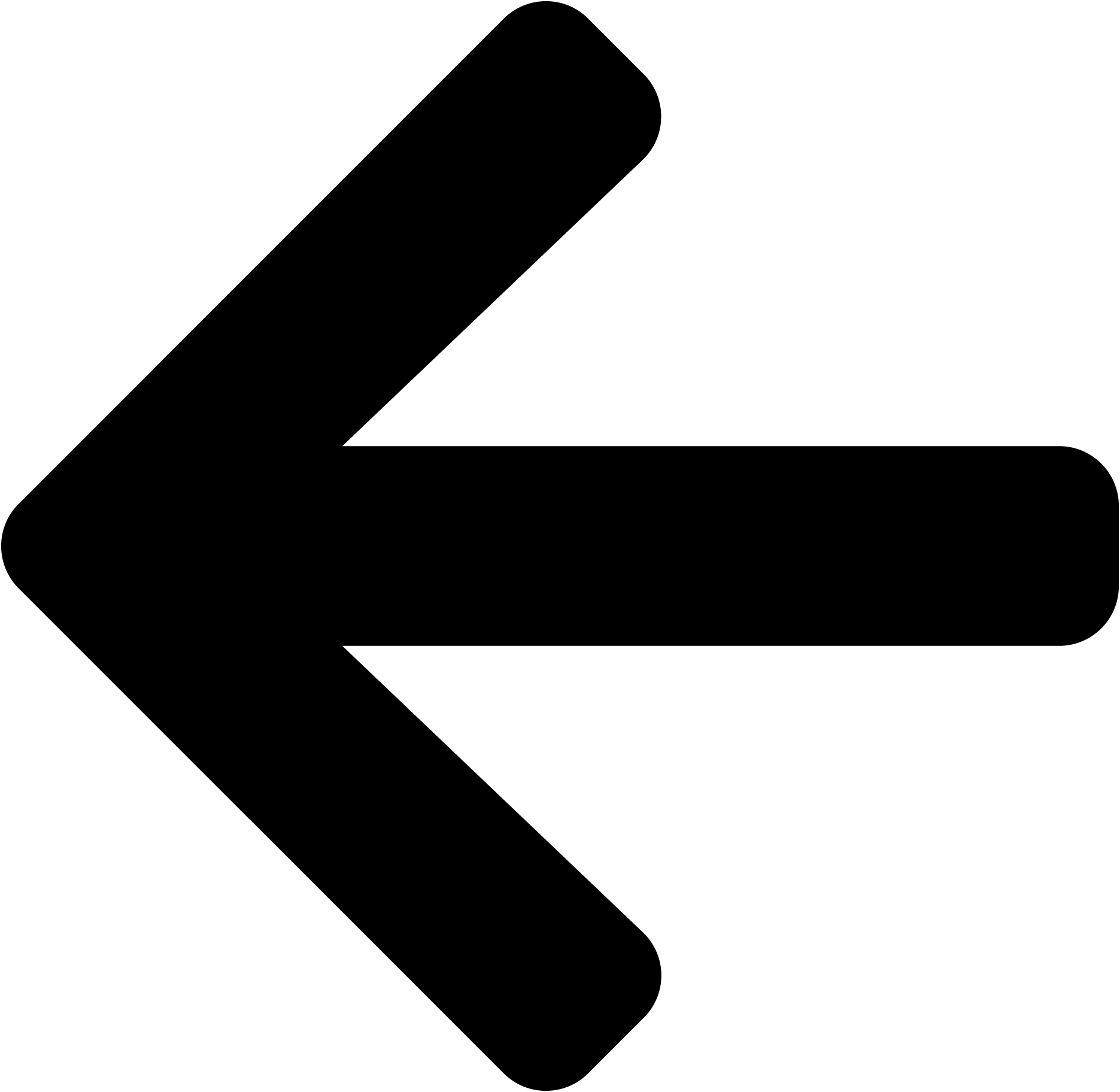
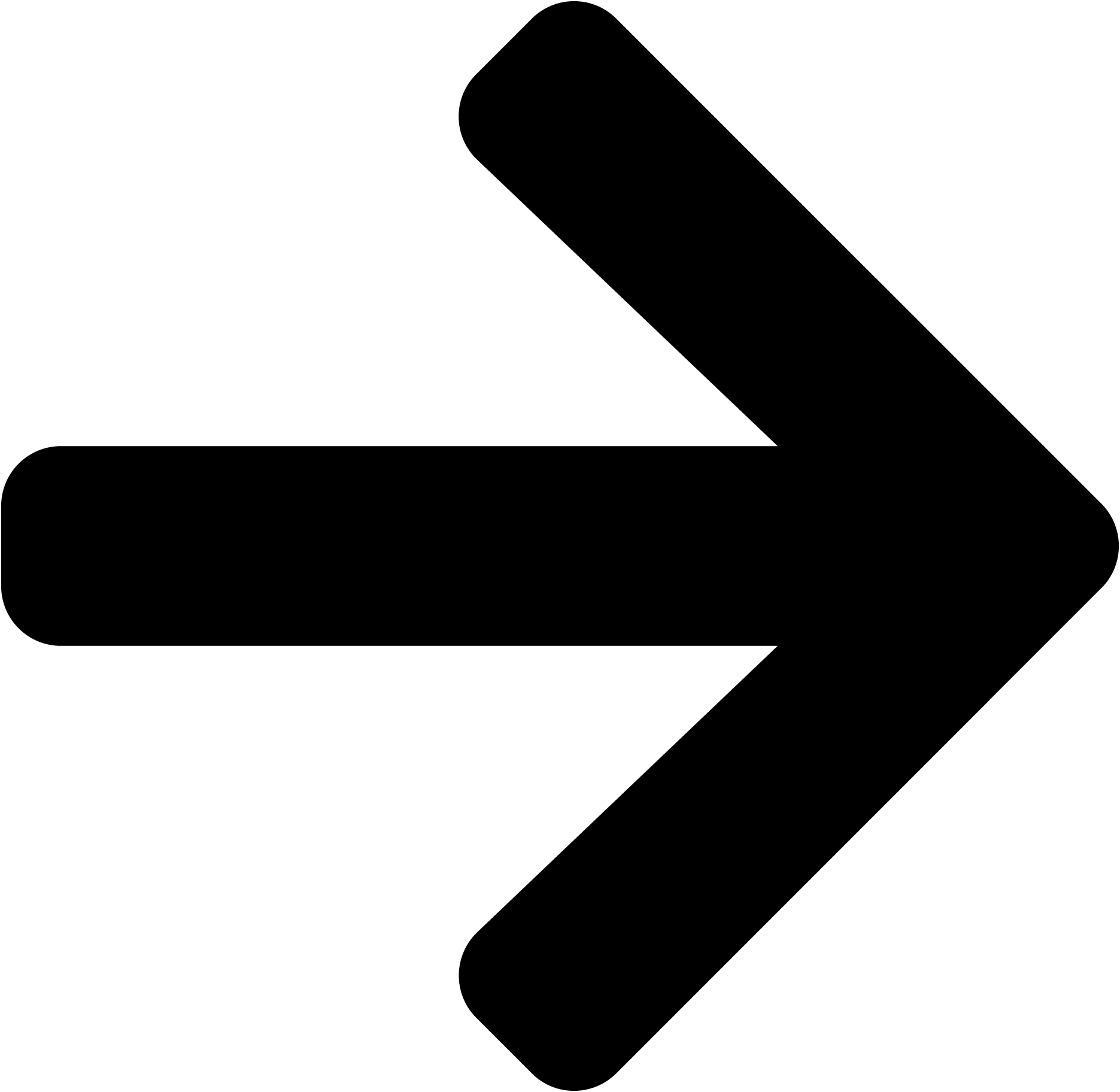
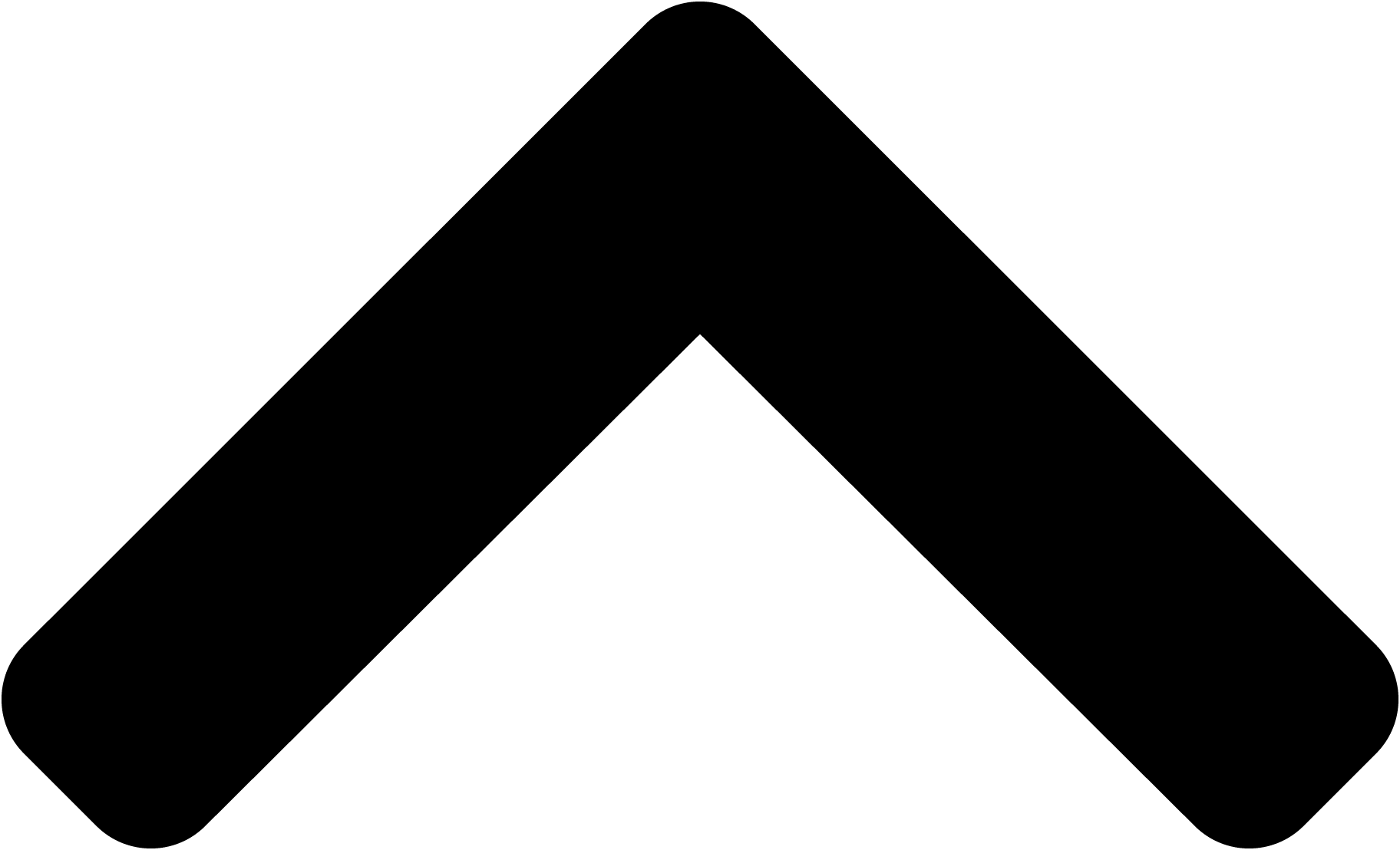
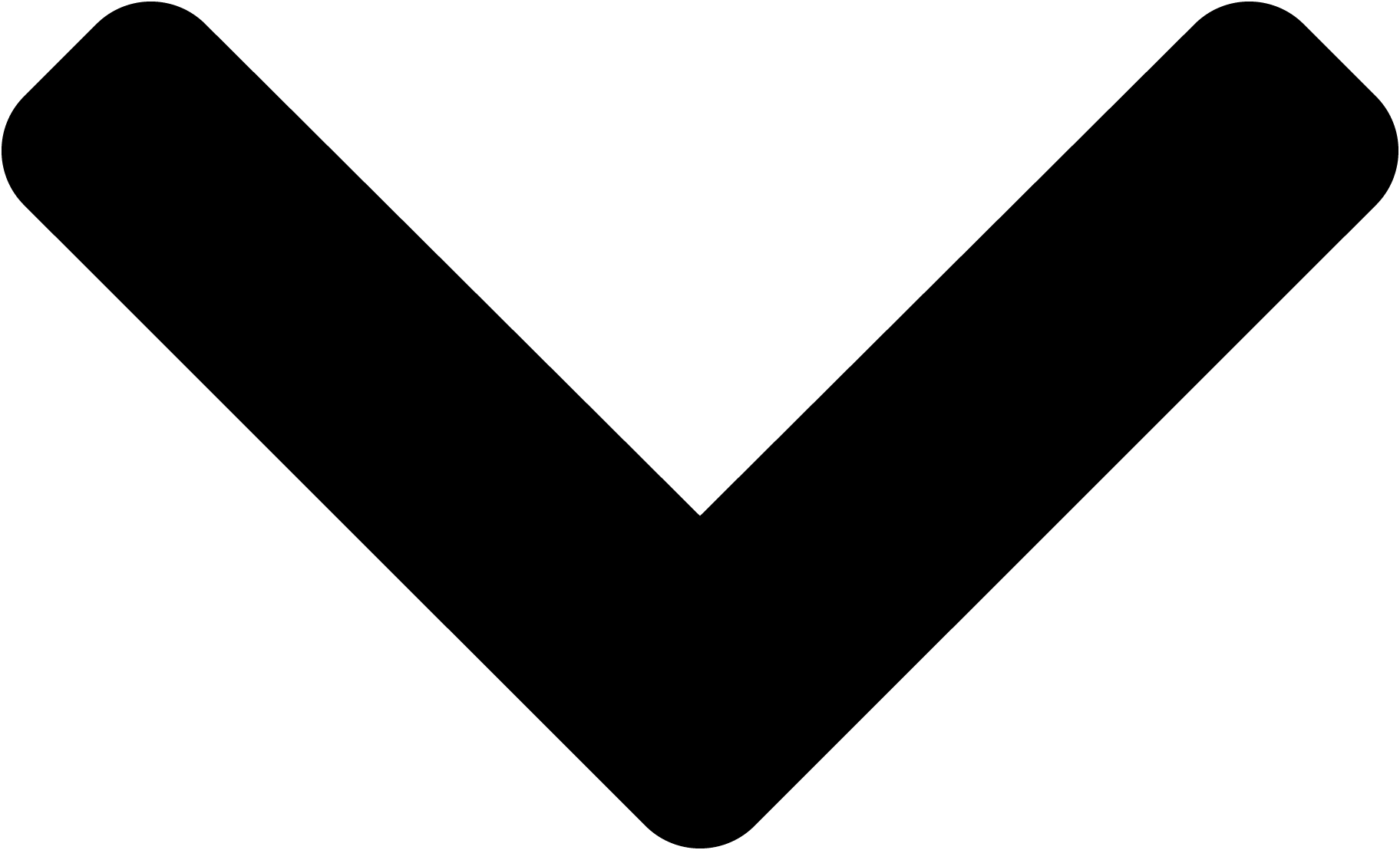
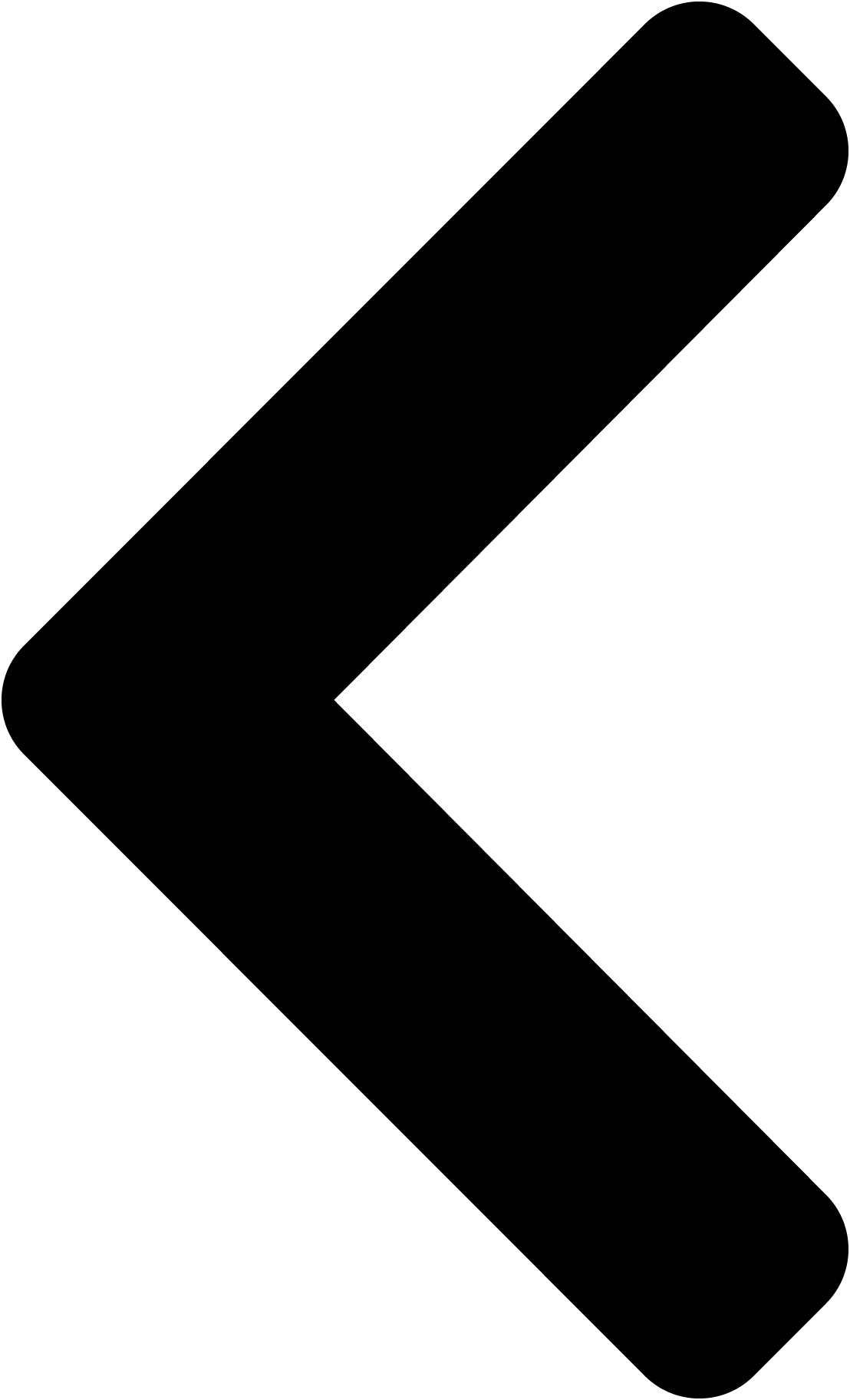
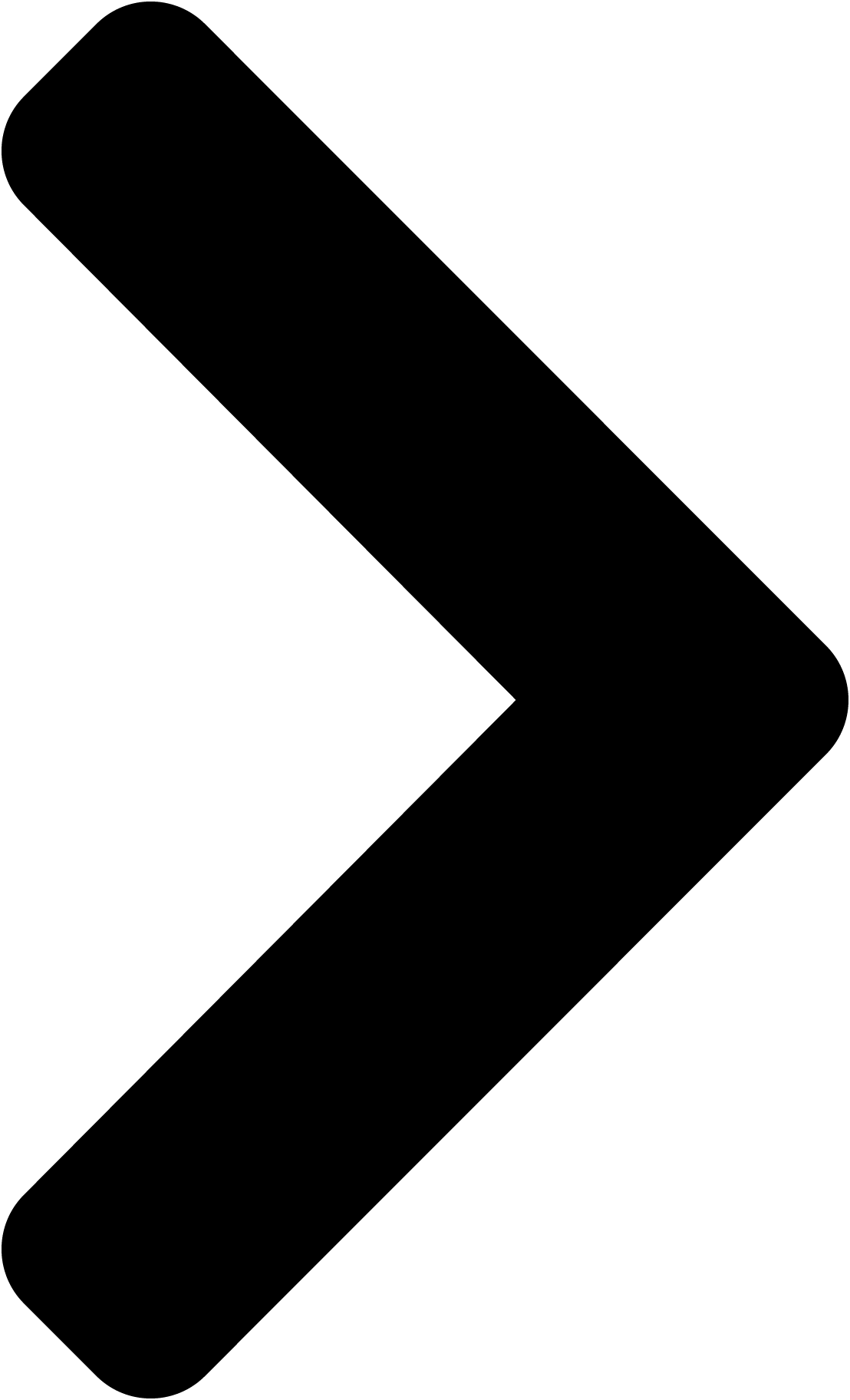
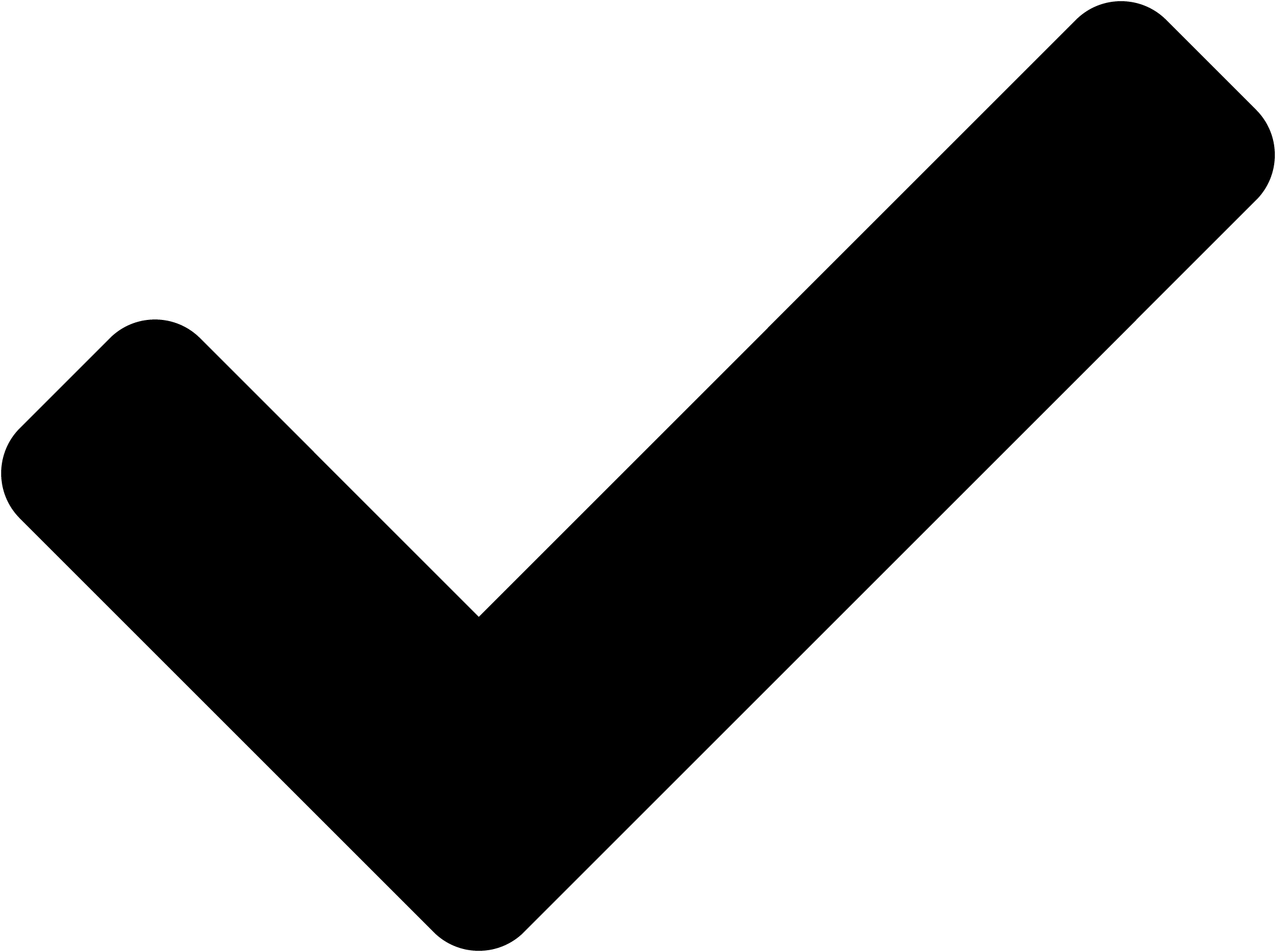
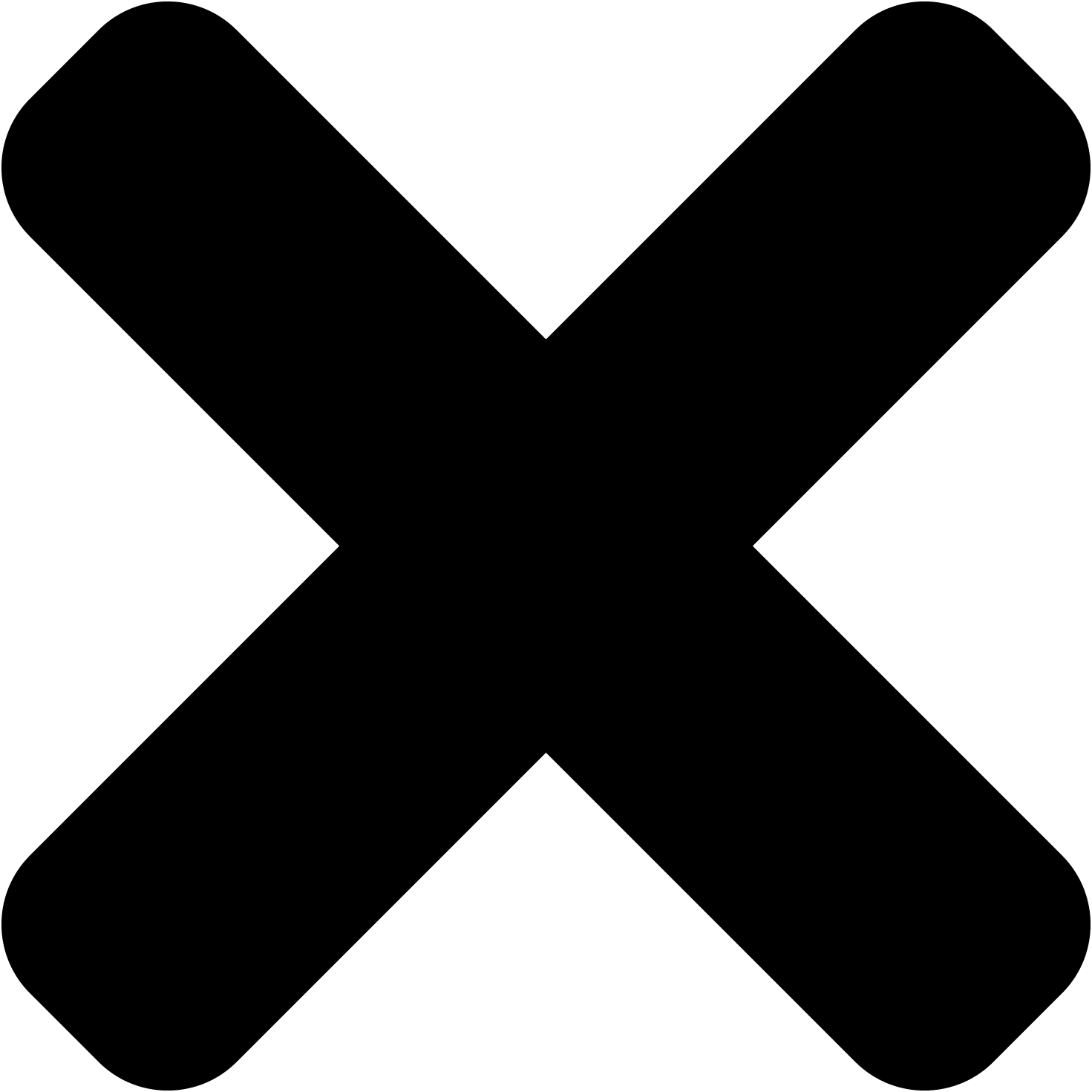
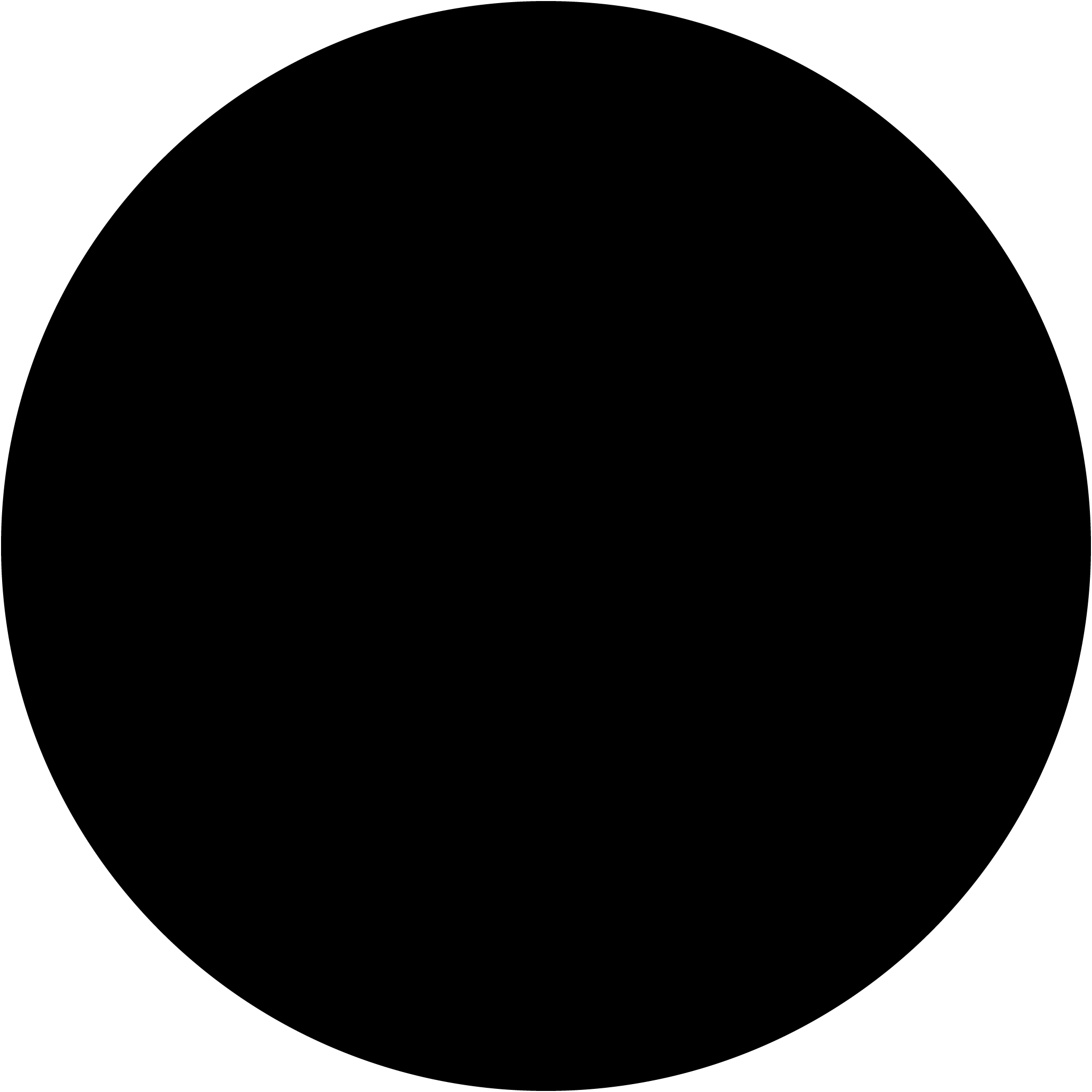
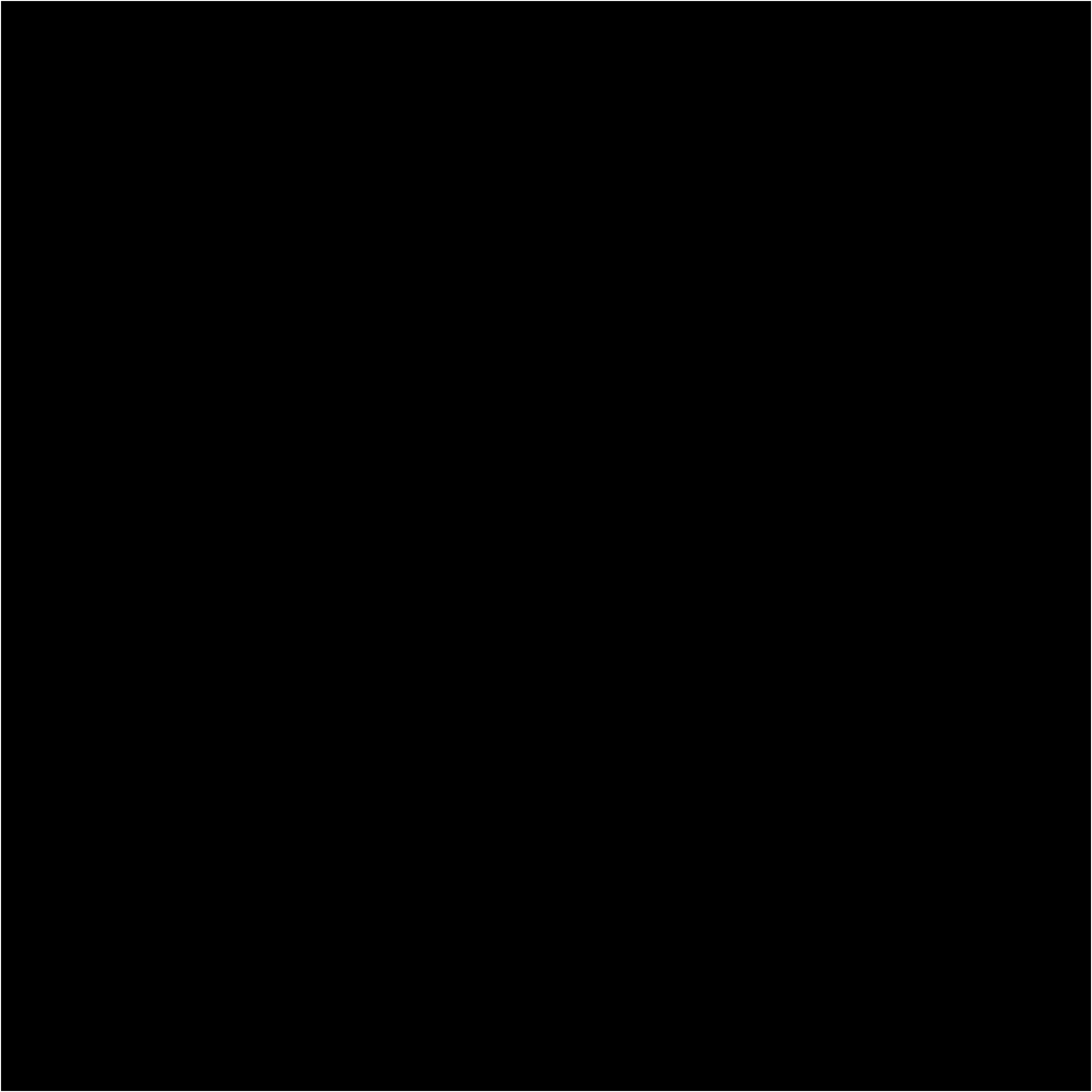
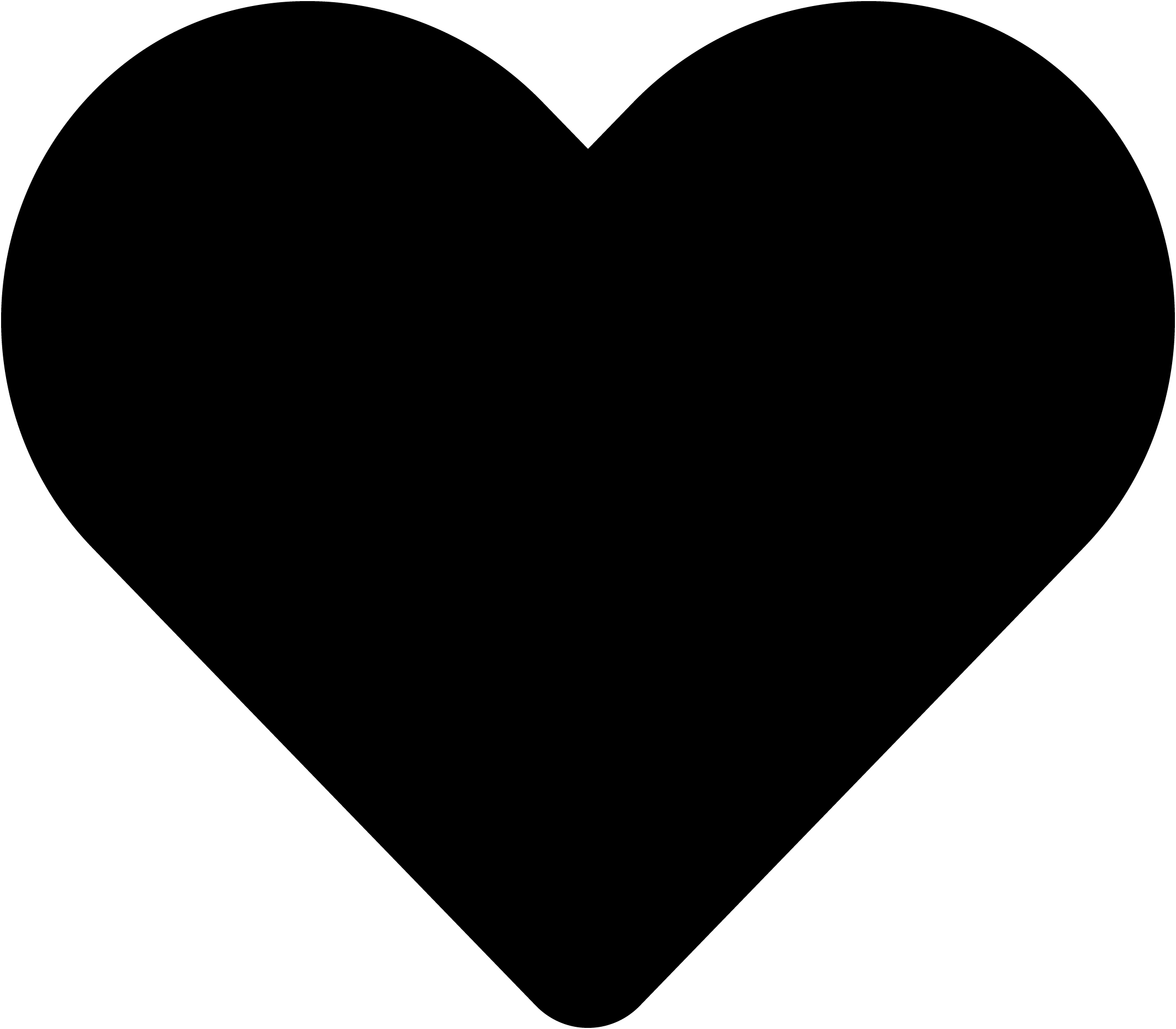
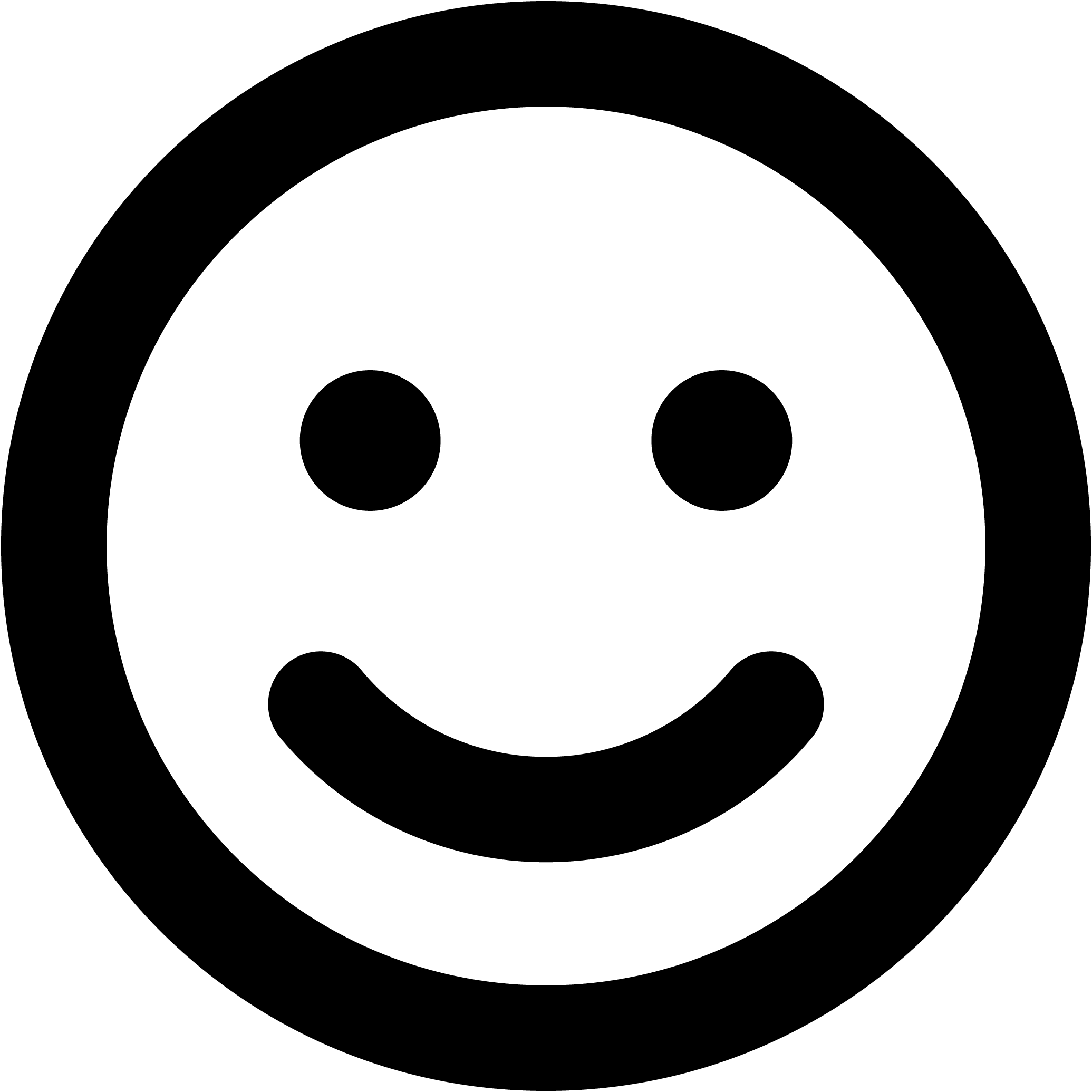
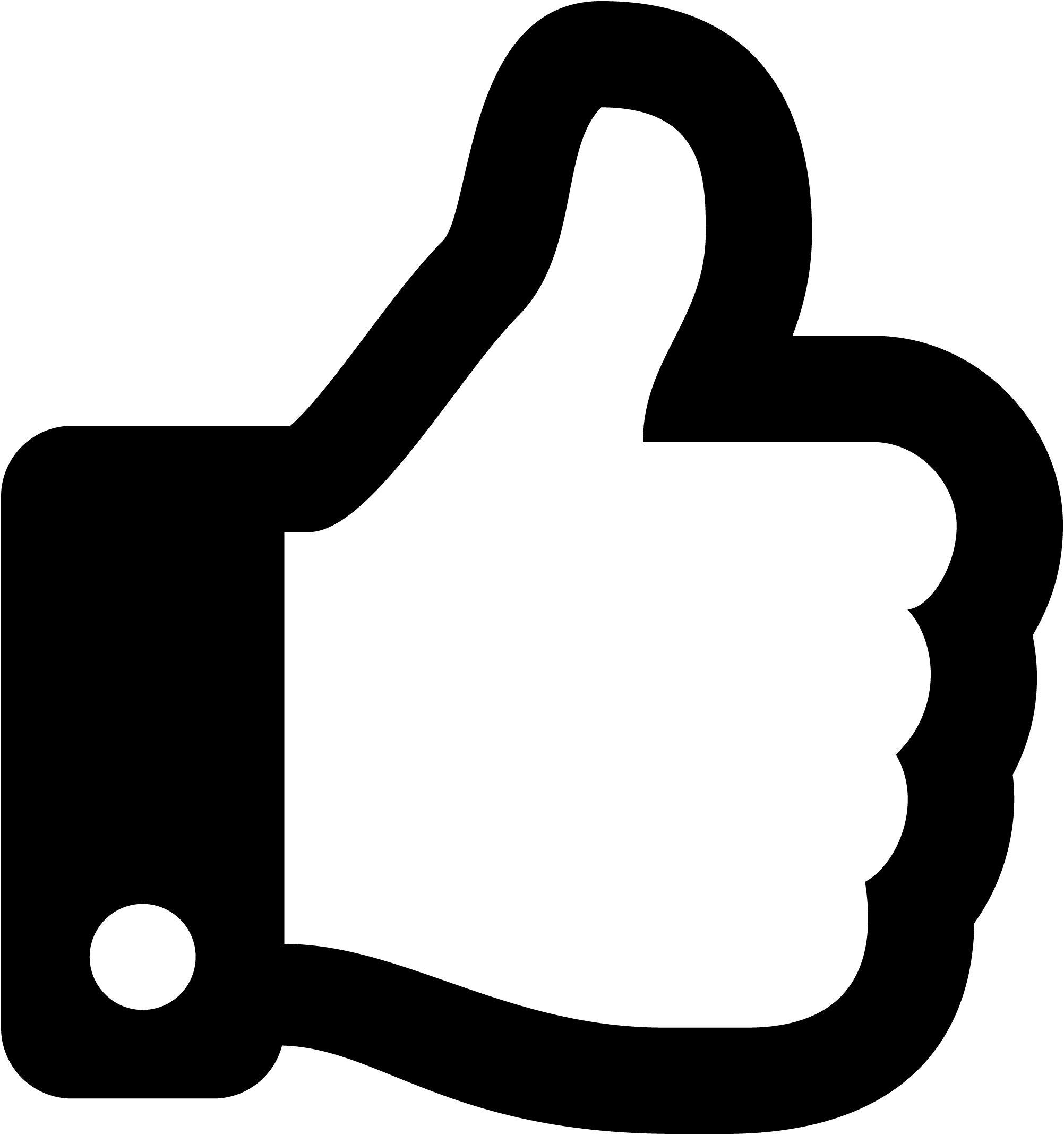
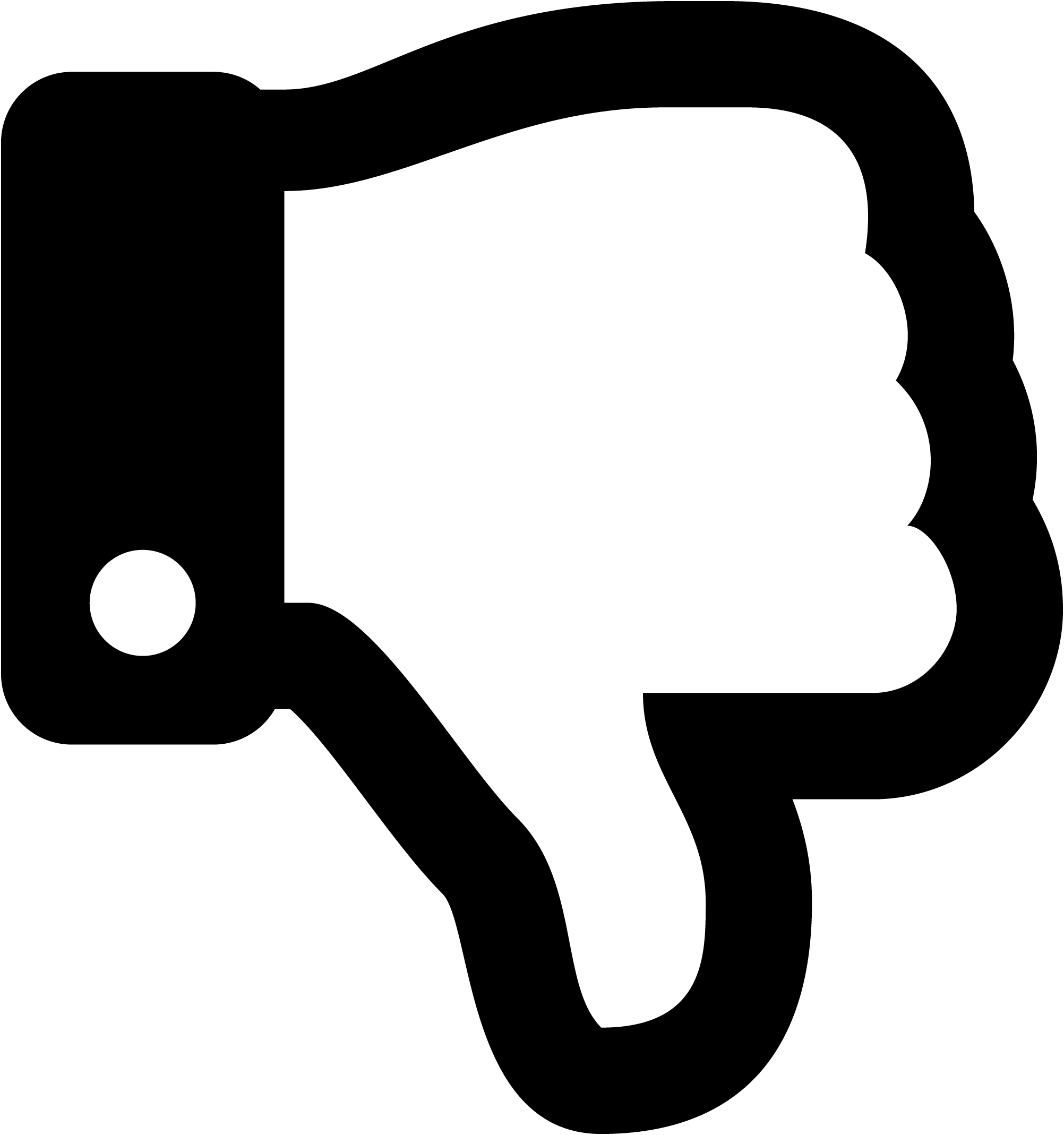